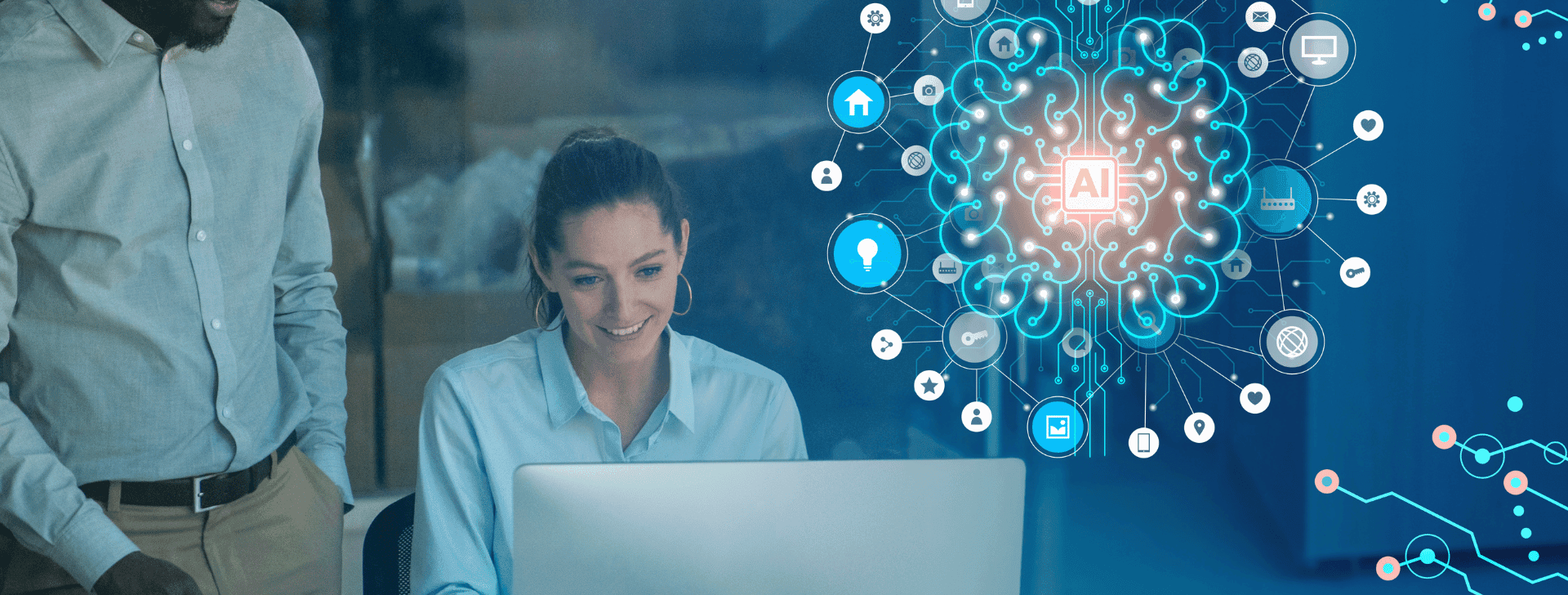
What is generative AI? How it’s shaping the future of technology (Updated 2025)
SEP. 10, 2024
9 Min Read
Generative AI has quickly become one of the most discussed technologies in recent years, fundamentally reshaping the way businesses operate and innovate.
But what exactly is generative AI? At its core, generative AI refers to systems that can create new content—be it text, images, music, or even code—based on patterns and data they have previously learned. This capability distinguishes it from traditional AI, which typically performs tasks based on rules or patterns without generating entirely new outputs.
As organizations look to integrate more advanced AI into their operations, understanding how generative AI works and its potential applications becomes essential. In addition to exploring what generative AI is, we’ll also look at how this transformative technology differs from other AI systems, such as artificial general intelligence (AGI), and how it’s reshaping industries across the globe.
Key takeaways
- 1. Generative AI can create new content such as text, images, or music by using patterns learned from data, distinguishing it from traditional AI that follows pre-defined rules.
- 2. Generative AI models like GPT-4, DALL·E, and Codex are transforming industries by automating content creation, coding, and design processes, boosting efficiency and innovation.
- 3. The distinction between AGI and generative AI is important; while generative AI is task-specific, AGI would possess the ability to learn and perform any intellectual task like a human.
- 4. Generative AI offers benefits such as increased personalization, automation of creative tasks, and cost reduction, though it comes with challenges like data quality, ethical concerns, and high computational costs.
- 5. The future of generative AI will see advancements in model efficiency and multi-modal models, broadening its applications while addressing ethical use and regulatory considerations.
What is generative AI?
Generative AI models rely on machine learning algorithms that are capable of processing massive amounts of data to generate new, unique content. These models, such as GPT-4, which can write text, or DALL·E, which generates images, are trained on vast datasets and then create content based on the patterns they’ve absorbed.
For example, when you ask a generative AI model to write a story, it doesn’t just pull existing sentences from a database—it crafts entirely new sentences based on its training. This makes generative AI incredibly powerful for applications ranging from creative arts to scientific research and product development.
Generative AI differs from traditional machine learning systems, which focus on recognizing patterns, making classifications, or providing predictive outputs. Instead of following predefined rules, generative AI models can perform tasks such as:
- Generating natural language text (e.g., chatbots, virtual assistants)
- Creating realistic images (e.g., art or design)
- Composing music
- Producing synthetic data for research purposes
Generative AI versus AGI: What’s the difference?
Generative AI and AGI (Artificial General Intelligence) are often confused, but they refer to different types of AI systems. While generative AI focuses on creating content based on specific data, AGI refers to an advanced form of AI that can perform any intellectual task that a human can. AGI has not yet been achieved, but researchers are working towards this goal.
What is AGI in artificial intelligence?
AGI represents a major leap beyond generative AI. While generative AI excels at specific tasks such as generating text or images, AGI would possess the ability to reason, learn from experience, and apply that knowledge across a wide variety of domains—just like a human. AGI could, in theory, solve complex problems without needing specific training data or rules for each task.
While generative AI systems are becoming more sophisticated, AGI remains theoretical for now. Achieving AGI would require significant advances in machine learning, cognitive science, and other fields, but the prospect of AGI continues to drive research in the AI space.
What is AGI versus AI?
The key distinction between AGI and narrow AI (such as generative AI) lies in their scope. Narrow AI systems are built for specific functions, like recognizing faces or generating music. AGI, on the other hand, would have the flexibility to perform any intellectual task, adapting to new situations and learning independently. Generative AI can be seen as a highly advanced form of narrow AI, but it’s not equivalent to AGI.
Generative AI vs. AGI
Artificial Intelligence is changing with distinct capabilities that define its types, such as Generative AI and Artificial General Intelligence (AGI). Below is a comparison of these two types:
Scope
- Generative AI: Focuses on narrow, task-specific applications, primarily used for creating specific outputs like text or images.
- AGI: Envisions a broad, general-purpose intelligence capable of handling a wide range of tasks, similar to human capabilities.
Capabilities
- Generative AI: Specializes in generating new content, such as text, images, or other media formats.
- AGI: Aims to develop the ability to reason, understand, and solve complex problems across various domains.
Current Status
- Generative AI: Already widely implemented in multiple industries, helping with tasks such as content generation, creative design, and data analysis.
- AGI: Remains a theoretical concept that is yet to be realized, with ongoing research to reach human-level cognition.
Learning
- Generative AI: Operates by training on specific datasets, learning patterns to produce relevant outputs.
- AGI: Expected to learn from experience similarly to humans, adapting to new information dynamically.
Example Technologies
- Generative AI: Includes well-known technologies such as GPT-4, DALL-E, and Codex, which are currently influencing various fields from creative arts to programming.
- AGI: Does not yet have any existing technologies as it remains a goal for future AI research and development.
While Generative AI is already making significant strides in practical applications across industries, AGI represents the next frontier in AI development. It aims to achieve human-like intelligence, capable of understanding and learning from the world in ways that go beyond the current limitations of machine learning.
The transition from Generative AI to AGI could revolutionize how machines interact with and influence human life, potentially leading to groundbreaking advancements in technology and society.
How does generative AI work?
Generative AI works by using advanced machine learning techniques to generate new content based on existing data. The most common architecture behind these models is the transformer architecture, which has been instrumental in making generative AI models like GPT-4 highly effective.
At a high level, generative AI models are trained on large datasets, learning to recognize patterns in the data. Once trained, these models can generate new content that resembles the input data without directly copying it. For example, when you input a text prompt, the model predicts and generates the most likely continuation of that prompt based on its training data.
The two primary techniques used in generative AI are:
- Autoregressive models: These models generate content one step at a time, using each previous output as the basis for the next. GPT-4 is an example of an autoregressive model, as it generates text word by word.
- Generative adversarial networks (GANs): GANs use two models—the generator and the discriminator—that work against each other. The generator creates content, while the discriminator tries to identify whether the content is real or fake. This process improves the quality of generated content over time.
The underlying mechanics of generative AI make it a highly versatile tool for applications across industries, from content creation to predictive modeling.
"Generative AI has quickly become one of the most discussed technologies in recent years, fundamentally reshaping the way businesses operate and innovate."
Key applications of generative AI
Generative AI is already being applied across a wide range of industries, delivering transformative outcomes. Here are some of the most impactful uses of this technology today:
1. Content creation
In the media and entertainment industries, generative AI is being used to create new music, art, and even video. AI models are helping creative professionals accelerate the production process by generating high-quality drafts that can be refined by humans. For example, AI-generated visual effects are enhancing films, while AI-driven writing tools are providing creative input for scriptwriters and marketers alike.
2. Healthcare innovation
Generative AI is also making waves in healthcare. Models are being trained to generate synthetic patient data, helping researchers test new medical treatments and drugs without the risk associated with real patient data. In medical imaging, AI can generate detailed visualizations of organs or diseases, supporting early diagnosis and personalized treatment plans.
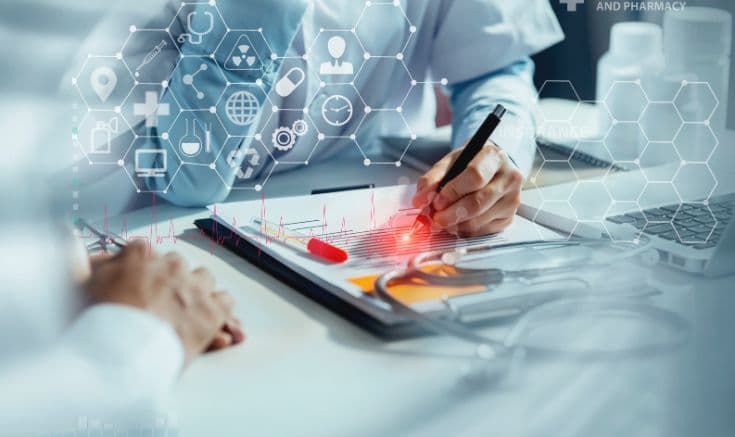
3. Software development
In the tech industry, generative AI tools like OpenAI’s Codex are being used to automate software code generation. These systems can generate entire blocks of code based on simple prompts, drastically reducing the time developers spend on mundane coding tasks and allowing them to focus on higher-level design and problem-solving.
4. Marketing and advertising
Marketing professionals are using generative AI to personalize customer experiences at scale. AI-generated content, such as personalized email campaigns, social media posts, and even custom advertisements, allows businesses to engage more effectively with their audiences. This results in increased engagement rates and better customer experiences overall.
5. Product design and prototyping
Generative design, powered by AI, is being used to create optimized product designs by exploring thousands of variations in a fraction of the time it would take a human designer. This is especially useful in industries like automotive and aerospace, where engineers use AI to design more efficient and innovative products that meet rigorous performance standards.
Types of generative AI
Generative AI is a broad field that encompasses several models and techniques, each designed to generate new content from different types of data. These AI systems are reshaping industries from entertainment to healthcare, with some of the most notable being models like ChatGPT, DALL·E, and Codex. Understanding the different types of generative AI and their applications is crucial for businesses looking to leverage this technology.
Popular Generative AI Models and Their Applications
1. ChatGPT: AI for text generation
ChatGPT, based on the GPT-4 architecture, is one of the most advanced models for natural language generation. It is trained on vast datasets of text and can generate coherent, contextually appropriate responses to user prompts. ChatGPT is widely used for various applications, including:
- Customer support: AI chatbots powered by models like ChatGPT can provide real-time customer service, answering queries, resolving issues, and even assisting in more complex transactions.
- Content creation: Writers and marketers are using ChatGPT to generate blog posts, social media updates, product descriptions, and other forms of content, reducing the time spent on drafting and editing.
- Virtual assistants: AI-powered virtual assistants use ChatGPT to interact with users, manage schedules, set reminders, and even provide technical support.
ChatGPT’s ability to understand context and generate human-like responses has made it an essential tool for businesses looking to improve customer experience and streamline operations.
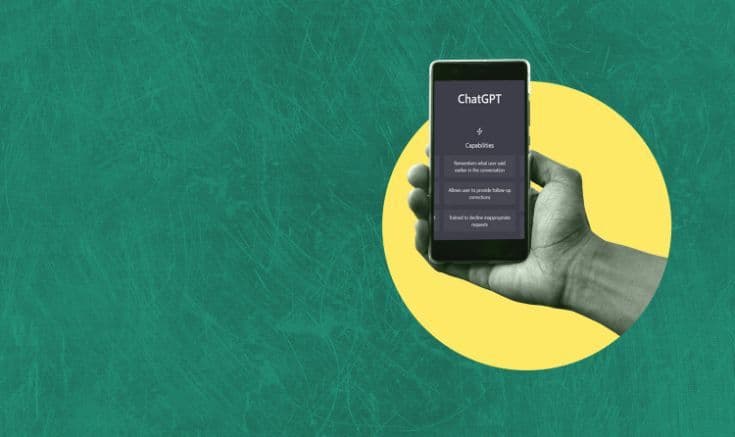
2. DALL·E: AI for image generation
DALL·E, another generative AI model, focuses on generating images from textual descriptions. This model, also based on the GPT architecture, has been trained to understand the relationships between words and visual concepts, enabling it to generate highly creative and realistic images based on prompts.
Applications of DALL·E include:
- Marketing and advertising: Creative teams are using DALL·E to quickly generate custom visuals, reducing the reliance on stock images or expensive photography.
- Design and art: Artists and designers can experiment with DALL·E to create unique artwork or explore new design concepts in a fraction of the time it would take to produce these visuals manually.
- Prototyping: In industries like fashion or product design, DALL·E can generate realistic images of potential designs, helping companies visualize their ideas before committing resources to physical production.
DALL·E represents a major leap in creative AI, allowing businesses to enhance their marketing efforts and reduce production costs in visual content creation.
3. Codex: AI for code generation
Codex is another product of the GPT family, designed to generate code based on natural language prompts. Codex can write entire blocks of code, solve programming challenges, and even debug code, making it a game-changer for software development.
Key applications of Codex include:
- Automating coding tasks: Codex can assist developers by automating repetitive or complex coding tasks, allowing them to focus on more creative aspects of software design.
- Learning and education: Codex is also used in educational platforms, where it helps users learn to code by providing real-time code suggestions, explanations, and solutions to coding problems.
- Prototyping and development: Codex accelerates the software development process by generating code for prototypes or minimum viable products (MVPs), reducing the time it takes to go from concept to execution.
With its ability to understand and write code in several programming languages, Codex is modifying the way developers work, improving productivity and reducing time-to-market for new software products.
4. MusicLM: AI for music generation
MusicLM is an AI model specifically designed for generating music based on text descriptions. This model can compose songs, generate melodies, and even create entire musical compositions based on the user’s input, making it a valuable tool for the music and entertainment industries.
Applications of MusicLM include:
- Music production: Music producers can use MusicLM to quickly generate backing tracks, melodies, or entire songs, speeding up the production process and reducing costs.
- Entertainment: Film, television, and video game developers use MusicLM to create custom soundtracks and musical scores that match the tone and mood of their projects.
- Personalized music: MusicLM can generate personalized compositions based on individual preferences, offering unique experiences for listeners or event planners.
These models demonstrate the versatility of generative AI and its ability to revolutionize a wide array of industries, from content creation to software development and beyond
The impact of various generative AI models
As generative AI continues to change, the number and types of models available will expand, further broadening the range of applications. Models like ChatGPT, DALL·E, Codex, and MusicLM are just the beginning of what’s possible with generative AI. These models are already reshaping industries, making it easier for businesses to automate tasks, enhance creativity, and deliver more personalized experiences.
By understanding the different types of generative AI and their applications, businesses can make informed decisions about how to integrate these technologies into their operations, driving innovation and advantage.
Benefits and challenges of generative AI
Generative AI offers numerous benefits to businesses, but it also comes with challenges that must be addressed for successful adoption.
Benefits of generative AI:
- Automation of creative processes: Generative AI can automate tasks such as content creation, design, and marketing, saving businesses time and resources.
- Increased personalization: By generating personalized recommendations and content, businesses can better engage with their customers, leading to improved satisfaction and loyalty.
- Enhanced productivity: Generative AI tools can automate repetitive tasks, allowing employees to focus on higher-level work, improving overall productivity and efficiency.
- Accelerated innovation: Generative AI can rapidly generate new product designs, code, or even synthetic data, helping businesses speed up research and development. This allows companies to prototype faster and bring products to market more quickly.
- Cost reduction: By automating time-consuming tasks such as content creation, customer service, and software development, generative AI can reduce operational costs. This is particularly useful for businesses looking to streamline workflows and allocate resources more efficiently.
Challenges of generative AI:
- Data quality: Generative AI requires high-quality data to produce reliable and accurate outputs. Poor-quality data can lead to biased or inaccurate results.
- Ethical concerns: The misuse of generative AI for malicious purposes, such as creating deepfakes or fake news, poses significant ethical challenges.
- High computational cost: The training and deployment of generative AI models require significant computational resources, making it difficult for smaller businesses to adopt them.
- Lack of transparency: Generative AI models can sometimes behave like "black boxes," meaning it can be difficult to understand how they arrive at certain resolutions or outputs. This lack of transparency can pose issues when it comes to accountability, especially in regulated industries like healthcare and finance.
- Bias and fairness: If generative AI models are trained on biased datasets, they may produce biased outputs, perpetuating inequalities in areas like hiring, lending, or legal evaluation. Ensuring fairness and avoiding bias in AI-generated outputs is a complex, ongoing challenge.
Despite these challenges, the benefits of generative AI are pushing businesses to invest in and explore the technology’s full potential. By addressing these limitations with the right strategies, companies can unlock new opportunities for growth, innovation, and advantage.
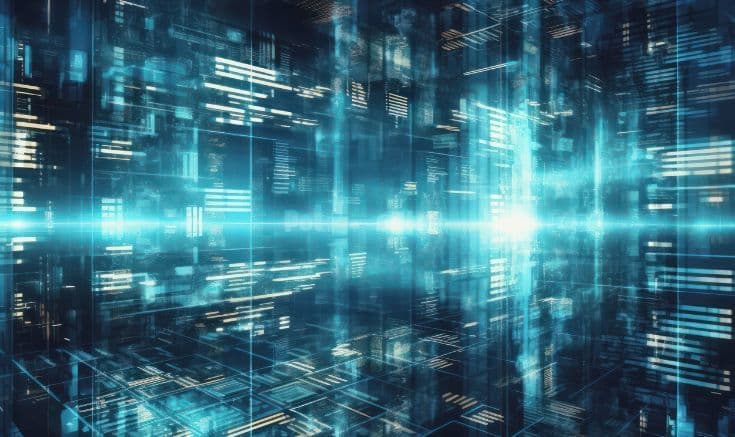
How generative AI is shaping the future of technology
Generative AI is driving the next wave of innovation across industries, enabling businesses to create, design, and deliver products faster than ever before. The ability of these models to generate unique, high-quality content means that industries reliant on creativity, precision, and large-scale data processing are benefiting the most from AI adoption.
1. Accelerating innovation in research and development
In research and development (R&D), generative AI is helping companies reduce costs and increase speed. AI can simulate new product designs or test the efficacy of new drugs in virtual environments before moving to physical trials. This accelerates the innovation pipeline, allowing companies to stay aggressive in developing markets.
2. Driving personalization in customer experiences
In industries like retail and finance, generative AI is enhancing customer interactions. AI models can create personalized recommendations, tailored product suggestions, and targeted marketing campaigns, helping companies deliver better services at scale. As these systems improve, businesses will be able to offer customized experiences, driving higher customer satisfaction and loyalty.
3. Advancing creative fields
Generative AI is renewing creative industries, including fashion, music, and entertainment. By automating routine tasks such as sketching designs, generating music compositions, or editing images, AI allows human creatives to focus on refining and perfecting their work. The collaboration between AI and human creativity is unlocking new possibilities in artistic expression and design.
"The future of generative AI is bright, with potential applications across industries continuing to expand."
What’s next for generative AI?
Generative AI is still in its early stages, but rapid advancements indicate that the technology will play an even more transformative role in the future. Understanding where generative AI is heading can help businesses anticipate trends and prepare for the future.
One key area of development is improving model efficiency. Researchers are working on creating models that require less data and computational power to deliver high-quality outputs. This is crucial for democratizing access to generative AI, allowing smaller businesses to take advantage of the technology without significant financial or resource investment.
Another major advancement on the horizon is the integration of multi-modal models. Currently, most generative AI models specialize in one form of content (e.g., text or images), but multi-modal models are capable of generating various types of content simultaneously. For example, a single model could generate both text and images for a marketing campaign, drastically improving efficiency and creativity for businesses.
Finally, the ethical use of generative AI will continue to be a priority. As the technology becomes more powerful, ensuring its responsible use will be essential. Organizations will likely see stricter regulatory frameworks emerge around the use of generative AI, particularly in areas like data privacy, misinformation, and bias.
The future of generative AI is bright, with potential applications across industries continuing to expand. By staying informed on these advancements, businesses can position themselves to leverage this transformative technology effectively.
Staying at the forefront of generative AI
Generative AI is a transformative technology that is reshaping industries by automating tasks, improving creativity, and enabling new possibilities in product development and customer interaction. While there are clear benefits to adopting generative AI, businesses need to understand its limitations and challenges to ensure responsible and effective implementation.
Table of contents
- What is generative AI?
- Generative AI versus AGI: What’s the difference?
- How does generative AI work?
- Key applications of generative AI
- Types of generative AI
- Benefits and challenges of generative AI
- How generative AI is shaping the future of technology
- What’s next for generative AI?
- Common questions about generative AI
Common questions about generative AI
What is generative AI and how does it work?
What is the difference between generative AI and AGI?
What are the key benefits of generative AI?
How is generative AI used in industries like healthcare and marketing?
What are the ethical concerns around generative AI?
Want to learn how generative AI can bring more transparency and trust to your operations?