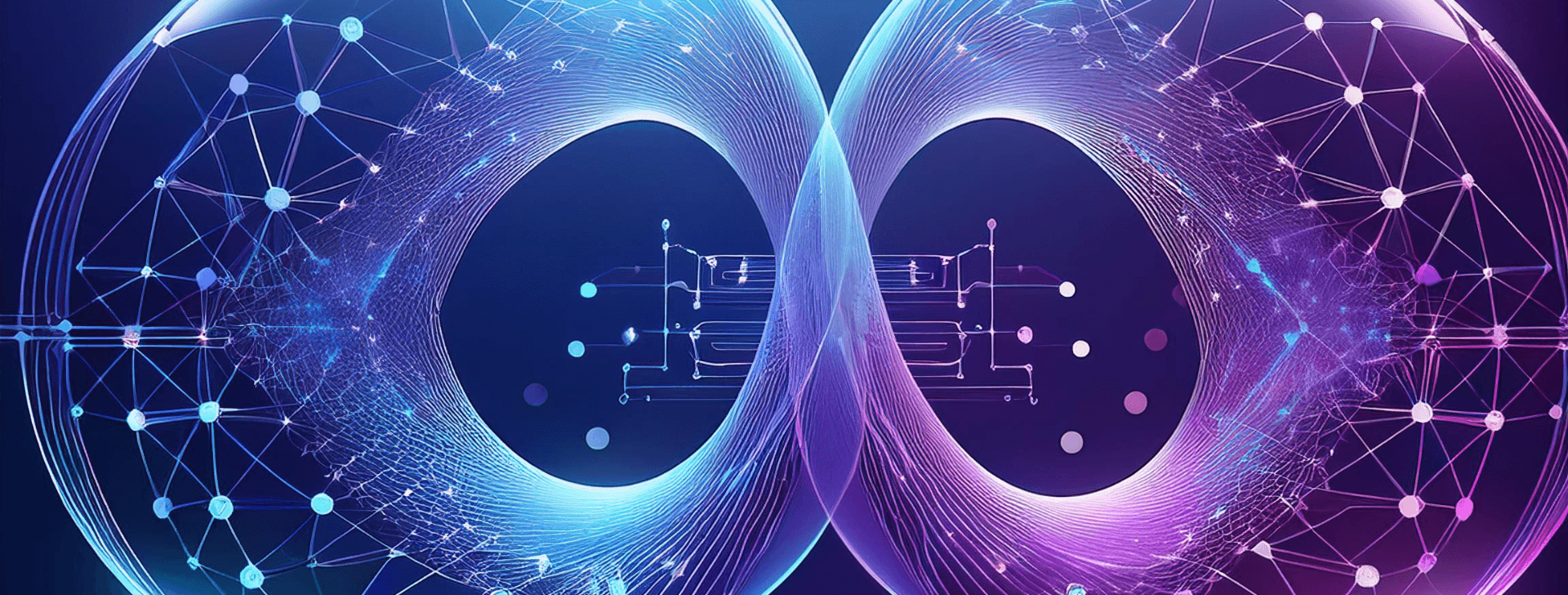
9 differences of machine learning vs generative AI
DEC. 14, 2024
5 Min Read
Machine learning and generative AI are shifting how organizations optimize operations, innovate processes, and achieve measurable outcomes.
These technologies offer distinct yet complementary approaches to solving complex problems, empowering businesses to scale efficiently, discover new opportunities, and deliver meaningful results. Whether the goal is operational excellence or groundbreaking creativity, understanding their unique applications is critical to driving success.
Key takeaways
- 1. Machine learning enhances operational efficiency and improves decisions by analyzing data for predictions and classifications.
- 2. Generative AI enables creative outputs like text, images, and audio, driving innovation and enhancing customer engagement.
- 3. Effective implementation of these technologies requires clear objectives, scalable infrastructure, and strong governance frameworks.
- 4. Both machine learning and generative AI provide measurable business value, from cost efficiency to revenue growth.
- 5. Combining these technologies allows businesses to unlock new opportunities and future-proof their operations for long-term success.
Understanding machine learning and generative AI
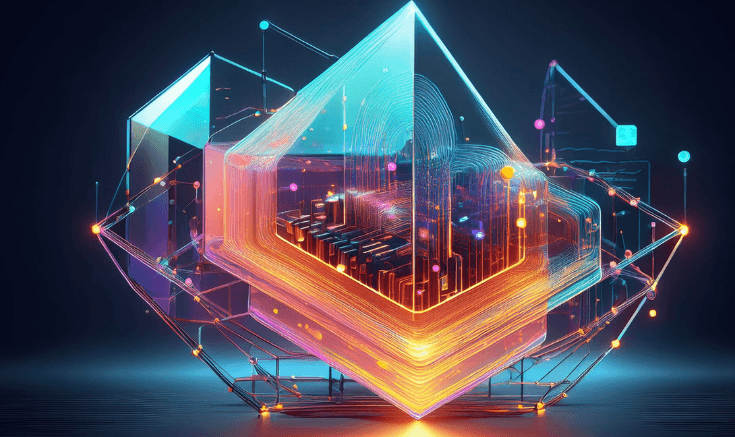
Machine learning and generative AI are transformative technologies shaping industries worldwide. While both rely on algorithms and data to deliver insights or create outputs, they serve distinct purposes. Machine learning focuses on analyzing data patterns to make predictions or improve decision-making processes. Generative AI, however, is specialized in creating new data—such as text, images, or sounds—that closely mimics human-like creativity.
The distinctions between these technologies lie in their underlying methods and applications. Machine learning powers systems like recommendation engines, fraud detection, and predictive maintenance, while generative AI underpins innovations such as realistic image generation, conversational agents, and content creation. Understanding these differences is crucial for selecting the right approach to meet specific business objectives, optimize processes, or generate measurable ROI.
"Machine learning delivers measurable value by enhancing efficiency and optimizing processes, allowing companies to scale operations while reducing costs."
9 key differences of machine learning vs generative AI
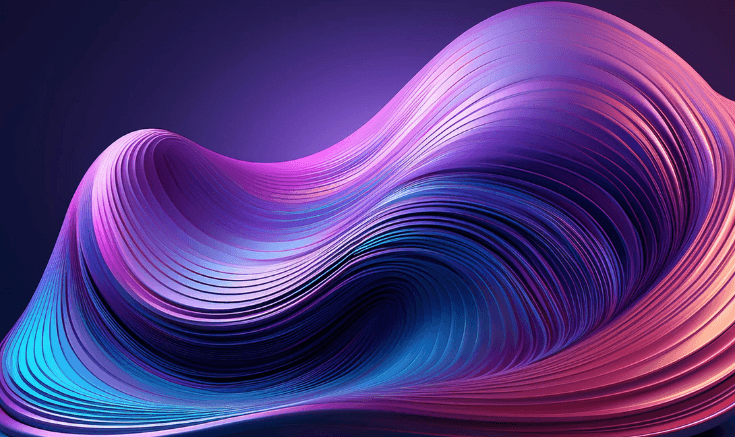
The main differences between machine learning vs generative AI are centered on their unique goals, learning methods, and operational capabilities. Machine learning excels in recognizing patterns and making accurate predictions based on data, while generative AI focuses on creating new, original outputs. Both offer immense business potential but cater to distinct use cases, making it important to identify which aligns with your goals. Let’s explore these differences in depth.
1. Purpose and functionality
Machine learning is designed to analyze data and draw actionable insights, typically optimizing performance. Its algorithms identify trends, classify information, and make accurate forecasts across various scenarios. This makes it ideal for applications like customer segmentation, fraud detection, and predictive analytics.
Generative AI, on the other hand, produces entirely new content based on existing patterns. Rather than identifying trends, it synthesizes new data, mimicking human creativity. This capability is transformative in areas like creative content generation, conversational AI, and dynamic visualizations.
Understanding the purpose of each allows you to leverage machine learning for operational efficiency and generative AI for innovative outputs that capture user attention and engagement.
2. Data requirements
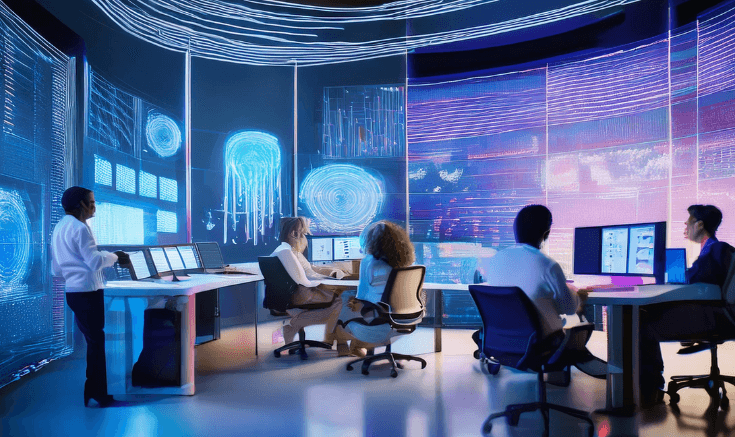
Machine learning relies heavily on structured datasets, often requiring labeled data for supervised learning tasks. The quality and quantity of input data directly impact its effectiveness. Preprocessing and curating datasets are critical steps to ensure reliable predictions or classifications.
Generative AI demands a multitude of datasets rich in context, as it needs extensive input to train models capable of creating realistic and nuanced outputs. Data diversity and context improve the quality of generative models, ensuring outputs align with user expectations and market demands.
Recognizing the data dependencies of both technologies helps businesses streamline preparation processes, reducing costs and accelerating deployment timelines.
3. Output type
Machine learning provides outputs in the form of predictions, classifications, or numerical values. These results guide decision-making, offering clear and actionable insights into specific problems.
Generative AI generates new outputs that mirror the patterns in its training data. Examples include images, text, and audio, all created to resemble real-world examples while introducing novel variations.
Selecting the right technology depends on whether your goal is to enhance operational clarity with predictions or to innovate with new, creative outputs.
4. Learning approach
Machine learning adopts various techniques, such as supervised, unsupervised, and reinforcement learning. Supervised learning uses labeled data, while unsupervised learning identifies patterns in unstructured data. Reinforcement learning focuses on optimizing actions through trial and error.
Generative AI often employs deep learning models, particularly neural networks like generative adversarial networks (GANs) or transformer-based architectures. These models learn complex data relationships, enabling the creation of highly realistic outputs.
Tailoring your choice of technology to align with your team’s expertise and resources ensures a smoother adoption curve and faster ROI.
5. Model complexity
Machine learning models vary in complexity, ranging from simple regression algorithms to sophisticated ensemble methods. These models balance computational efficiency with performance accuracy, depending on the task.
Generative AI models tend to be more complex, often requiring higher computational resources and extended training times. Models like GANs involve intricate processes, such as pitting generator and discriminator networks against each other to refine outputs.
Evaluating the complexity of models ensures you allocate the right resources to achieve desired outcomes without overburdening your infrastructure.
6. Application scope
Machine learning has a broad application scope, excelling in predictive analytics, recommendation systems, and anomaly detection. Its adaptability makes it a go-to solution across industries like healthcare, finance, and logistics.
Generative AI, while more specialized, shines in creative and immersive applications. It powers virtual assistants, digital art, and synthetic data generation, offering unique opportunities for innovation.
Understanding the scope of each ensures alignment with strategic priorities, optimizing outcomes for both efficiency and creativity.
7. Handling uncertainty
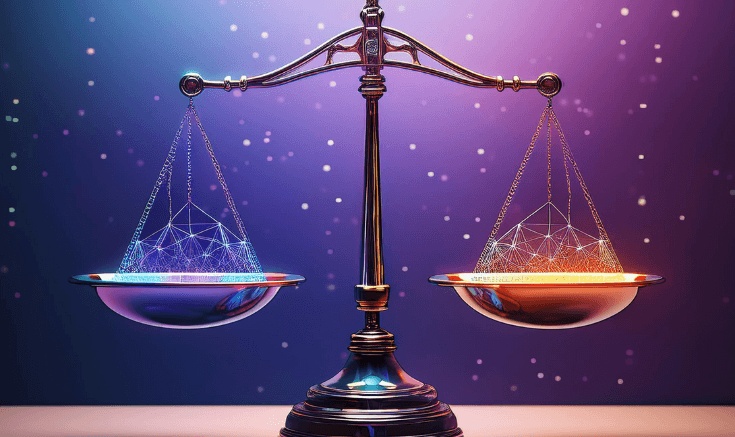
Machine learning thrives in scenarios where data is incomplete or ambiguous, providing probabilistic insights and confidence intervals to guide decisions. Its adaptability enables it to handle real-world uncertainties effectively.
Generative AI is less suited to dealing with uncertainty, as it focuses on producing outputs rather than analyzing probabilities. However, it can aid in scenarios like simulating future scenarios or generating varying solutions.
Selecting the right approach for uncertainty management can enhance risk mitigation and foster more informed decisions.
8. Interpretability
Machine learning models often emphasize interpretability, allowing users to understand why certain predictions were made. This transparency is crucial for applications in regulated industries like healthcare or finance.
Generative AI models are typically less interpretable due to their complexity. While they deliver impressive outputs, explaining the processes behind them can be challenging, limiting their use in sensitive areas requiring clear accountability.
Balancing the need for interpretability with your business goals ensures trust and alignment with compliance requirements.
9. Autonomy and interaction
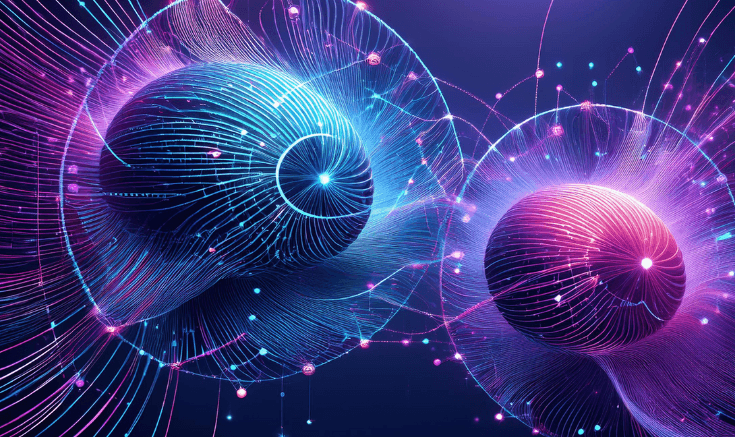
Machine learning systems typically operate autonomously, requiring minimal user interaction once deployed. They focus on automating processes and optimizing decision-making frameworks.
Generative AI often encourages user interaction, especially in creative domains. It enables businesses to collaborate with AI to refine outputs, delivering tailored and engaging results.
Evaluating the level of autonomy or interaction you need can clarify which technology better suits your operational model.
Both machine learning and generative AI offer transformative potential, each tailored to specific use cases and objectives. Businesses seeking efficiency, scalability, and measurable impact may lean toward machine learning, while those prioritizing innovation and creative engagement can explore generative AI. Choosing the right approach is not just a matter of technical preference but a strategic decision aligned with your long-term vision.
"Generative AI unlocks untapped creative potential, empowering businesses to produce innovative solutions that resonate with customers or stakeholders."
Business impact of machine learning and generative AI
Machine learning and generative AI are not just transformative technologies—they are strategic enablers that reshape how businesses operate, compete, and innovate. Machine learning delivers measurable value by enhancing efficiency and optimizing processes, allowing companies to scale operations while reducing costs. Generative AI, on the other hand, unlocks untapped creative potential, empowering businesses to produce innovative solutions, from personalized customer experiences to entirely new product categories.
Both technologies drive significant ROI by aligning with core business priorities. Machine learning accelerates time-to-value through predictive analytics and intelligent automation, while generative AI enables future-proof strategies by addressing emerging demands with agility and innovation. Regardless of if your focus is operational optimization or creative transformation, these technologies provide the tools to achieve lasting competitive advantage.
Their impact extends beyond immediate gains. Machine learning strengthens data and decisions, helping organizations anticipate challenges and mitigate risks. Generative AI fosters deeper customer engagement and opens doors to new revenue streams. Together, they allow businesses to not only address current challenges but also strategically position themselves for long-term growth and market leadership.
Implementing machine learning and generative AI strategies
Achieving the full potential of machine learning and generative AI requires a strategic approach tailored to specific business needs. These technologies demand more than just technical expertise; they necessitate alignment across teams, robust infrastructure, and a focus on measurable outcomes. Whether improving efficiency, uncovering new revenue streams, or enhancing customer experiences, a clear implementation strategy is essential.
Establishing measurable objectives and aligning stakeholders
A successful strategy begins with defining clear, measurable objectives. Businesses must pinpoint the processes or outcomes they aim to improve, ensuring alignment with broader goals such as scalability, cost efficiency, or enhanced decisions. These objectives guide every stage of implementation, from model selection to performance evaluation.
Stakeholder alignment is equally critical. Collaboration across departments ensures clarity, minimizes resistance, and fosters support. Engaging leaders, technical teams, and operational staff early can streamline adoption while promoting accountability.
Building scalable infrastructure for sustainable growth
Scalability is a cornerstone of any AI initiative. Machine learning and generative AI often require significant computing power, robust data pipelines, and flexible cloud solutions to meet both current and future demands. Investing in scalable infrastructure ensures systems can handle increasing complexity and larger data volumes over time.
Integrated platforms and modular solutions are key to sustainable growth. These tools allow businesses to adapt to evolving needs without overhauling existing systems, ensuring faster time-to-market and greater agility in response to new opportunities.
Implementing governance and ensuring transparency
Governance frameworks are essential for managing the risks and complexities associated with machine learning and generative AI. These frameworks address compliance with regulations, data security, and ethical considerations, building trust among stakeholders and customers.
Transparency is another critical component. Businesses must ensure that machine learning models and generative AI outputs are explainable and aligned with organizational values. This fosters confidence while minimizing risks related to bias, misinterpretation, or regulatory challenges.
Iterating for impact and driving continuous optimization
A results-driven approach ensures that AI initiatives deliver tangible benefits. Machine learning can streamline operations and improve decisions, while generative AI fosters innovation and customer engagement. Monitoring key performance indicators and analyzing real-time feedback enable ongoing refinement.
Continuous optimization is vital to maintaining the relevance and effectiveness of AI solutions. Iterative updates based on business goals ensure that models evolve alongside market demands, unlocking further opportunities for growth and innovation.
Tailoring implementation strategies to align with organizational goals maximizes the business impact of machine learning and generative AI. These efforts not only support operational efficiency and creativity but also enable future-proof strategies that drive sustainable success.
Machine learning and generative AI are redefining what’s possible, from operational efficiencies to transformative innovation. At Lumenalta, we specialize in crafting AI-driven strategies and solutions that align with your business objectives, delivering measurable impact and long-term value.
Let’s shape the future together.
Table of contents
- Understanding machine learning and generative AI
- 9 key differences of machine learning vs generative AI
- 1. Purpose and functionality
- 2. Data requirements
- 3. Output type
- 4. Learning approach
- 5. Model complexity
- 6. Application scope
- 7. Handling uncertainty
- 8. Interpretability
- 9. Autonomy and interaction
- Business impact of machine learning and generative AI
- Implementing machine learning and generative AI strategies
- Common questions about machine learning vs generative AI
Common questions about machine learning vs generative AI
What is the main difference between machine learning and generative AI?
How do machine learning and generative AI benefit businesses?
What industries can benefit most from machine learning and generative AI?
How can businesses implement machine learning or generative AI effectively?
Are machine learning and generative AI future-proof technologies?
Want to learn how machine learning & generative AI can bring more transparency and trust to your operations?