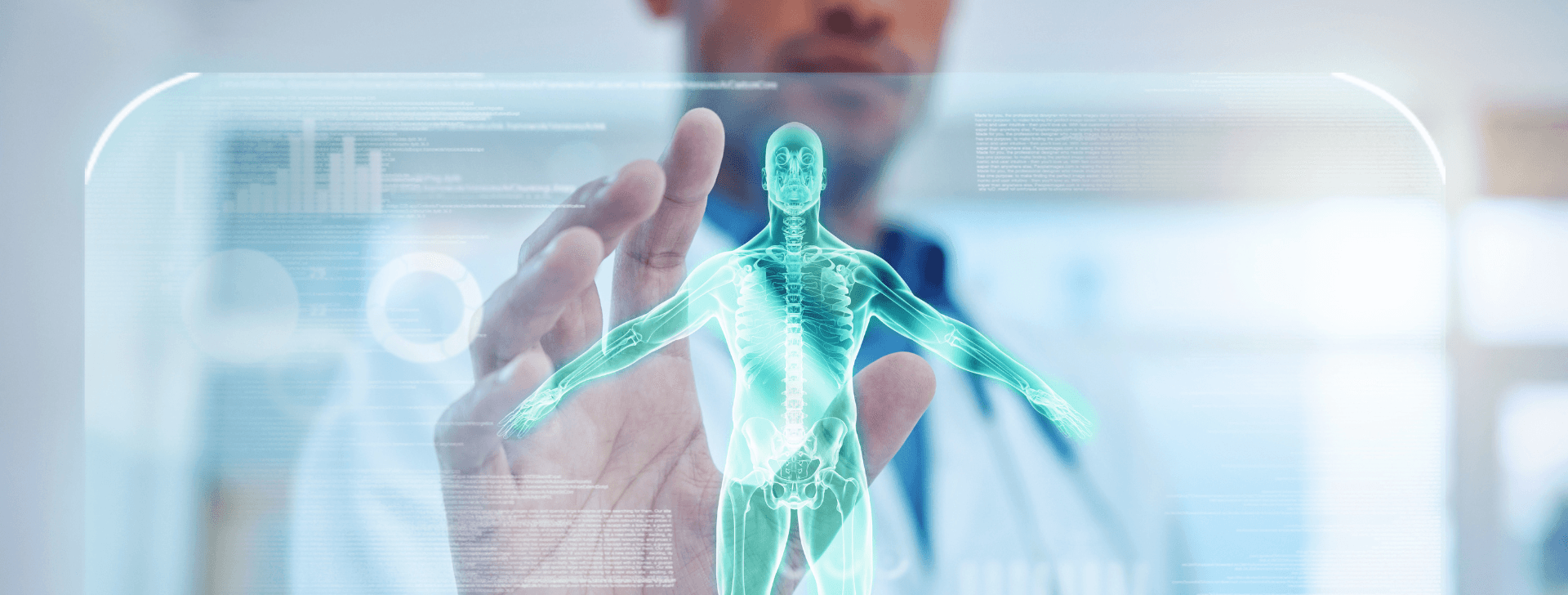
Healthcare innovations powered by generative AI
OCT. 10, 2024
8 Min Read
The healthcare industry is undergoing rapid transformation, driven by the integration of cutting-edge technologies.
One of the most impactful innovations is generative AI, which is not only changing how data is processed but also how healthcare services are delivered. Generative AI in healthcare has the potential to significantly enhance operational efficiency, improve diagnostics, and personalize patient care in ways previously unimaginable.
By understanding how generative AI for healthcare is evolving, decision-makers can leverage these insights to guide their own healthcare systems toward greater efficiency and improved patient outcomes.
Key takeaways
- 1. Generative AI in healthcare offers transformative benefits, from automating administrative tasks to improving diagnostics and personalizing treatment plans.
- 2. AI-driven applications, such as predictive analytics and virtual health assistants, enhance operational efficiency and improve patient care outcomes.
- 3. Key challenges include data privacy, regulatory compliance, and the need to address algorithmic bias to ensure equitable treatment across all demographics.
- 4. Collaborative partnerships between healthcare organizations and AI vendors are essential for building tailored AI solutions that meet specific operational needs.
- 5. To successfully implement generative AI, healthcare organizations must start with pilot projects, ensure data governance, and invest in staff training to maximize AI’s potential.
What is generative AI in healthcare?
Generative AI refers to the use of artificial intelligence (AI) models that can create new data, such as text, images, or predictions, based on the patterns they have learned from vast datasets. In the context of healthcare, generative AI enables the analysis of large volumes of medical data, such as patient records, imaging scans, and genomic information, to generate insights, automate tasks, and even simulate outcomes. The core capability of generative AI lies in its ability to generate new, contextually relevant information that aids in decision-making, diagnosis, and treatment.
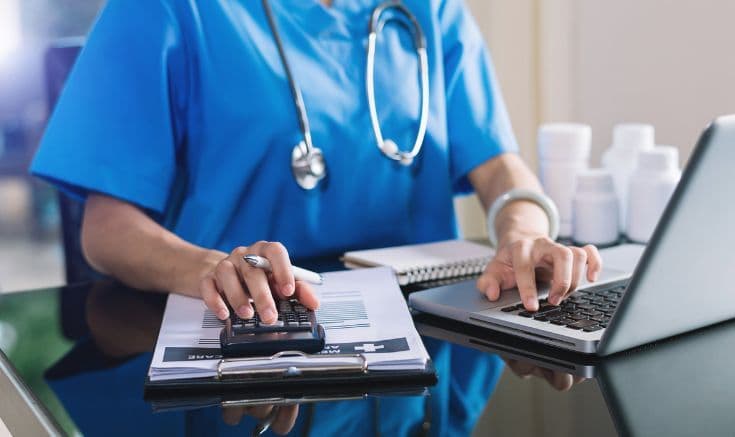
- AI-driven medical insights: Generative AI models are designed to assist healthcare providers by offering real-time insights derived from a combination of patient history, medical literature, and current data. This helps clinicians in making faster, more informed decisions about patient care.
- Automation of clinical tasks: Generative AI excels in automating repetitive and labor-intensive tasks like generating medical documentation, summarizing patient records, or creating discharge instructions in multiple languages. This reduces administrative burdens on healthcare professionals and allows them to focus more on patient care.
- Predictive modeling: One of the most powerful applications of generative AI in healthcare is its ability to create predictive models. By analyzing past data and generating potential future scenarios, AI can forecast patient outcomes, anticipate disease progression, and suggest preventive measures tailored to individual patients.
- Personalized treatment and care: Generative AI can analyze a patient’s unique genetic and medical data to recommend personalized treatment plans. This ensures that patients receive care that is specifically tailored to their needs, improving outcomes and reducing the likelihood of adverse reactions to treatments.
Generative AI in healthcare is not just a tool for automation but a transformative technology that can enhance the accuracy of diagnostics, streamline operations, and provide more personalized and effective patient care.
Generative AI healthcare use cases
Generative AI is revolutionizing healthcare across a diverse range of applications, from automating administrative tasks to assisting with complex diagnostics and personalized treatment plans. By leveraging machine learning models, healthcare organizations can optimize workflows, improve accuracy, and enhance patient outcomes. The following are key generative AI healthcare use cases that are already demonstrating significant value.
Administrative efficiency
One of the most immediate applications of generative AI is streamlining administrative tasks such as scheduling, billing, and medical documentation. These systems can extract critical information from unstructured data, such as handwritten medical notes and patient histories, significantly reducing manual work. This automation not only cuts down on clerical errors but also allows healthcare professionals to allocate more time to patient care. In some cases, AI systems can even assist with coding and billing processes, improving accuracy and minimizing delays in reimbursements.
Clinical decision support
AI algorithms can analyze vast datasets of medical records, lab results, and imaging data to provide decision-support tools that assist physicians in diagnosing and treating patients. By identifying patterns that may not be obvious to human clinicians, generative AI helps in the early detection of diseases such as cancer and heart disease. These AI-driven insights enable healthcare providers to make more informed decisions, thereby increasing diagnostic accuracy and improving patient outcomes. For instance, AI can flag abnormal patterns in medical images, supporting radiologists in making quicker and more precise diagnoses.
Drug discovery and development
The drug discovery process is being revolutionized by generative AI’s ability to simulate molecular interactions and predict how different compounds might behave. This allows pharmaceutical companies to accelerate the identification of promising drug candidates and reduce the time and cost involved in bringing new treatments to market. AI models can sift through vast libraries of chemical compounds and suggest those most likely to interact with disease-causing proteins, streamlining the experimental process. Additionally, AI can predict how different populations of patients may respond to specific medications, contributing to the development of more targeted therapies.
Personalized treatment
One of the most powerful applications of generative AI in healthcare is its ability to create personalized treatment plans based on a patient’s unique genetic profile, medical history, and lifestyle. For example, in oncology, AI can recommend tailored treatment options that account for a patient’s genetic markers, improving the effectiveness of cancer therapies and reducing the likelihood of adverse side effects. This level of personalization ensures that treatments are optimized for each individual, enhancing both the efficacy and safety of medical interventions.
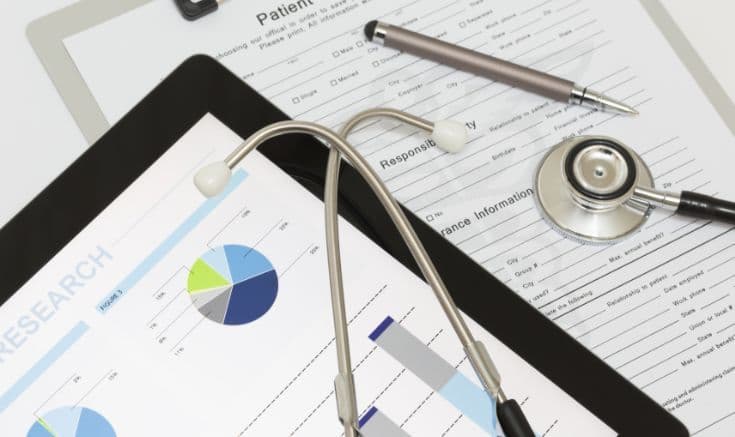
Telemedicine and virtual health assistants
AI-powered virtual health assistants and chatbots are transforming patient engagement by offering round-the-clock support for medical inquiries, appointment scheduling, and even initial diagnoses. These systems improve accessibility by providing real-time responses to patient questions and reducing the burden on healthcare staff. In telemedicine, AI enhances remote consultations by analyzing patient symptoms and recommending next steps, such as scheduling follow-ups or prescribing medications. Virtual health assistants are particularly valuable for managing chronic diseases, where continuous monitoring and timely interventions can prevent complications.
Predictive analytics
Generative AI’s predictive capabilities allow healthcare providers to anticipate patient needs, predict disease progression, and recommend preventive measures. This is especially important for managing chronic conditions such as diabetes, hypertension, and heart disease. By analyzing data from patient records, wearable devices, and health monitoring systems, AI can forecast potential health crises and enable proactive care. For example, AI can alert healthcare providers to signs of worsening conditions, prompting early intervention and potentially preventing hospitalizations.
Medical imaging analysis
Generative AI models are capable of analyzing medical images such as X-rays, MRIs, and CT scans with a high degree of accuracy. These models assist radiologists in identifying abnormalities, detecting diseases at earlier stages, and providing second opinions to reduce diagnostic errors. The use of AI in medical imaging is not limited to detection; it can also predict how diseases may progress based on image data, offering clinicians additional insights to guide treatment plans.
Operational forecasting
AI can also be used to optimize hospital operations, from predicting patient inflows to managing staff and resource allocation. By analyzing historical data, AI can forecast demand for hospital beds, medical supplies, and staffing needs. This leads to more efficient resource management, reducing wait times and ensuring that patients receive timely care. Hospitals that integrate generative AI into their operations report improvements in both operational efficiency and patient satisfaction.
Generative AI continues to expand its role in healthcare, offering innovations that are transforming the way medical services are delivered. From streamlining administrative tasks to personalizing treatments, AI is creating a more responsive, efficient, and patient-centered healthcare system. As these technologies continue to evolve, their impact on healthcare will only grow, bringing new opportunities to improve both clinical and operational outcomes.
Challenges and risks of generative AI in healthcare
While generative AI holds great promise for healthcare, there are several significant challenges and risks that need to be managed. One of the primary concerns is data privacy and security. Healthcare organizations handle highly sensitive patient data, and AI systems require access to large datasets to function effectively. Ensuring compliance with stringent regulations like HIPAA, while safeguarding against potential data breaches, is critical to maintaining patient trust and confidentiality. Additionally, the issue of algorithmic bias poses a substantial risk, as AI models trained on biased data can lead to unequal treatment outcomes, particularly across different demographic groups. Healthcare providers must ensure that AI systems are regularly audited and trained on diverse datasets to avoid these disparities.
Regulatory compliance also presents a significant challenge, as healthcare is one of the most heavily regulated industries. AI systems must meet strict safety and efficacy standards before being deployed at scale, making the approval and integration process complex. Another critical challenge lies in the lack of transparency often associated with AI models. Many generative AI systems, particularly those using deep learning, function as "black boxes," making it difficult for clinicians to understand and explain the decision-making process. This lack of explainability can hinder adoption in healthcare, where trust and accountability are paramount.
The integration of generative AI into existing healthcare infrastructures is another hurdle. Many healthcare organizations operate on legacy systems that may not be compatible with modern AI technologies, requiring substantial investments in both technology and training. Finally, there are ethical concerns about the role AI will play in healthcare, particularly in situations where life-altering decisions are made. While AI can enhance decision-making, it is essential to ensure that these technologies complement rather than replace human judgment in critical care scenarios. Addressing these challenges with a thoughtful, patient-centered approach is key to the responsible and effective use of generative AI in healthcare.
"Generative AI enables the analysis of large volumes of medical data, such as patient records, imaging scans, and genomic information, to generate insights, automate tasks, and even simulate outcomes."
How to implement generative AI for healthcare
Successfully implementing generative AI in healthcare requires a strategic approach that balances innovation with practicality. Here are key steps for healthcare organizations looking to adopt AI technologies:
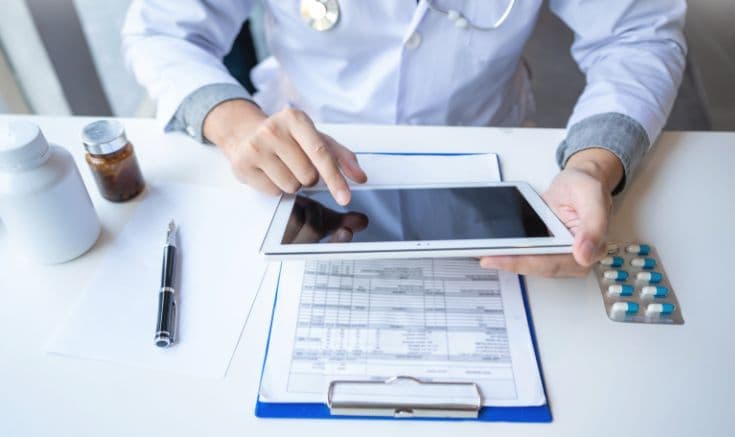
- Assess organizational readiness: Before implementing AI solutions, healthcare leaders must evaluate their organization's current technology infrastructure, data management capabilities, and staff expertise. This assessment will help identify any gaps that need to be addressed, such as upgrading IT systems or investing in staff training.
- Start with pilot projects: Implementing generative AI on a small scale through pilot programs allows healthcare organizations to test the technology in a controlled environment. These pilot projects can focus on specific areas such as medical imaging, administrative automation, or clinical decision support. Starting small helps identify challenges early and allows for adjustments before scaling the technology across the organization.
- Foster cross-functional collaboration: Collaboration between healthcare professionals, data scientists, and IT specialists is essential for the successful integration of AI. By bringing together diverse teams, organizations can ensure that AI models are designed with practical, real-world applications in mind. This collaborative approach also helps in addressing any concerns related to workflow changes or data handling.
- Ensure data quality and governance: The accuracy and reliability of AI models depend on the quality of the data they are trained on. Healthcare organizations must prioritize data governance by ensuring that patient data is accurate, up-to-date, and properly anonymized to protect privacy. Implementing strict data governance policies also helps in ensuring compliance with regulations and reducing the risk of data breaches.
- Develop clear use cases: Generative AI is a versatile tool, but its implementation should be driven by specific use cases that align with the organization’s strategic goals. Whether the aim is to improve diagnostic accuracy, reduce operational costs, or enhance patient outcomes, having clear objectives will guide the AI implementation process and help measure its success.
- Invest in training and education: Generative AI is a powerful tool, but healthcare professionals need to be equipped with the skills to use it effectively. Ongoing training and education programs are essential to ensure that staff understand how AI works, how to interpret AI-generated insights, and how to integrate these insights into patient care.
- Monitor and evaluate outcomes: Once generative AI is implemented, it’s important to continuously monitor its performance and evaluate outcomes. This includes tracking improvements in efficiency, cost savings, and patient outcomes, as well as addressing any issues related to algorithmic bias or system integration. Regular evaluations ensure that the AI system remains effective and aligned with organizational goals.
By following these steps, healthcare organizations can implement generative AI solutions that not only enhance operational efficiency but also improve patient care outcomes in a responsible and sustainable manner.
ROI of generative AI in healthcare
The return on investment (ROI) for generative AI healthcare applications is becoming an increasingly important consideration for healthcare organizations. While the technology is still in its early stages, the potential for cost savings and improved patient outcomes is already evident.
- Operational cost savings: Automating administrative tasks such as scheduling, billing, and medical documentation reduces the need for manual labor, leading to significant cost savings. Hospitals and clinics can reallocate these resources to more patient-centric activities, improving overall service delivery.
- Efficiency gains: AI’s ability to analyze vast amounts of data in real time enables healthcare providers to make quicker, more accurate decisions. This reduces the time patients spend in hospitals, lowers readmission rates, and enhances the overall efficiency of care delivery.
- Reduced diagnostic errors: Generative AI improves diagnostic accuracy by identifying patterns in medical images and patient data that may be overlooked by human clinicians. This leads to earlier detection of diseases and more effective treatment plans, ultimately lowering the long-term costs of care.
- Accelerated drug discovery: AI-powered drug discovery shortens the time it takes to bring new treatments to market, reducing research and development costs. This acceleration also means that life-saving treatments can reach patients faster, improving health outcomes and generating revenue more quickly.
- Patient satisfaction and outcomes: By enabling more personalized treatment plans and providing real-time monitoring, AI improves patient satisfaction and outcomes. Patients receive more tailored care, experience fewer complications, and are more likely to adhere to their treatment plans.
The ROI of generative AI in healthcare is both measurable and transformative. As more healthcare organizations begin to implement AI solutions, the financial and clinical benefits will become even more apparent.
Generative AI adoption trends
The adoption of generative AI in healthcare is accelerating, with many organizations in the early stages of proof-of-concept projects and pilot programs. However, while the promise of generative AI is significant, healthcare providers are approaching its implementation with a focus on strategic priorities and risk management.
- Proof-of-concept testing: Many healthcare organizations are currently testing AI solutions in controlled environments to assess their effectiveness and scalability. These initiatives help stakeholders understand the potential returns, risks, and operational challenges associated with deploying AI at scale.
- Strategic partnerships: A growing number of healthcare providers are collaborating with third-party vendors to co-develop AI solutions. These partnerships allow organizations to leverage external expertise while customizing AI tools to fit their specific needs.
- In-house development vs. third-party solutions: While some organizations are building AI capabilities in-house, others are opting to purchase off-the-shelf solutions. The decision largely depends on the complexity of the use case, the organization’s technological maturity, and the level of customization required.
- Adoption challenges: Despite the excitement around generative AI, some organizations are taking a wait-and-see approach due to concerns about data security, regulatory compliance, and the potential for algorithmic bias. Addressing these concerns is crucial for widespread adoption.
Healthcare providers adopting generative AI are positioning themselves to lead the industry in innovation and efficiency. By starting with proof-of-concept projects and fostering strategic partnerships, these organizations can build the necessary infrastructure to scale AI solutions effectively.
"Generative AI’s predictive capabilities allow healthcare providers to anticipate patient needs, predict disease progression, and recommend preventive measures."
Collaborative partnerships driving AI innovation
Collaborative partnerships are playing a key role in the development and deployment of generative AI for healthcare. By working with technology vendors, academic institutions, and other healthcare providers, organizations can accelerate the adoption of AI and ensure that it is tailored to meet their specific needs.
- Vendor partnerships: Many healthcare organizations are partnering with AI vendors to develop custom solutions that address specific operational challenges. These collaborations allow healthcare providers to leverage the expertise of AI specialists while retaining control over the implementation and scaling of AI systems.
- Academic collaborations: Research institutions are working with healthcare providers to test and validate AI models. These collaborations help ensure that AI solutions are safe, effective, and capable of delivering real-world results in clinical settings.
- Cross-industry partnerships: In addition to working with AI vendors and academic institutions, healthcare organizations are forming partnerships with companies in other industries, such as pharmaceuticals and insurance. These cross-industry collaborations are helping to drive innovation by pooling resources and expertise.
- Internal AI teams: Some healthcare providers are building in-house AI teams to develop and maintain their own AI systems. This approach allows organizations to fully control the development process and ensure that AI solutions are tailored to their specific workflows and patient populations.
Collaborative partnerships are essential for the successful implementation of generative AI in healthcare. By leveraging the expertise of external partners, healthcare providers can accelerate innovation and improve patient care.
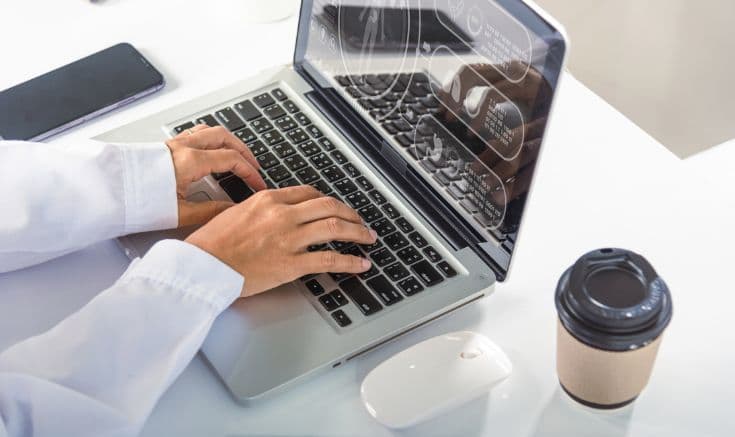
Bringing generative AI to healthcare: What leaders need to know
As generative AI continues to evolve, healthcare leaders must consider several factors to ensure successful implementation. From managing risks to aligning AI initiatives with strategic priorities, healthcare organizations need to take a comprehensive approach to adopting AI technology.
- Evaluate organizational readiness: Before implementing generative AI, healthcare leaders should assess their organization’s technological infrastructure and talent capabilities. Ensuring that the necessary systems and expertise are in place will help avoid common implementation pitfalls.
- Address data privacy and security concerns: AI models require access to vast amounts of sensitive patient data. Healthcare organizations must prioritize data privacy and implement robust security measures to protect against breaches and ensure compliance with regulations.
- Mitigate algorithmic bias: To ensure equitable care, healthcare organizations need to monitor AI models for potential biases. This involves regularly auditing algorithms and using diverse data sets to train AI systems.
- Focus on governance and compliance: Healthcare organizations should establish clear governance structures to manage AI initiatives. This includes defining roles and responsibilities, setting up oversight committees, and ensuring that AI solutions comply with regulatory requirements.
- Invest in training and education: As AI becomes more integrated into healthcare, it’s important for healthcare professionals to understand how to use AI tools effectively. Investing in training programs will help ensure that staff are equipped to leverage AI to improve patient care.
By considering these factors, healthcare leaders can position their organizations to successfully integrate generative AI into their operations, paving the way for improved patient outcomes and operational efficiency.
Generative AI holds immense potential to revolutionize healthcare, from operational improvements to enhanced patient care. As healthcare organizations continue to adopt and refine AI solutions, leaders must focus on building the right partnerships, mitigating risks, and aligning AI initiatives with their strategic goals. If you’re ready to explore how AI-powered solutions can elevate your healthcare services, visit Lumenalta’s healthcare solutions page.
Table of contents
- What is generative AI in healthcare?
- Generative AI healthcare use cases
- Challenges and risks of generative AI in healthcare
- How to implement generative AI for healthcare
- ROI of generative AI in healthcare
- Generative AI adoption trends
- Collaborative partnerships driving AI innovation
- Bringing generative AI to healthcare: What leaders need to know
- Common questions about generative ai in healthcare
Common questions about generative ai in healthcare
What is generative AI in healthcare, and how does it work?
How can generative AI improve healthcare efficiency?
What are the key use cases of generative AI in healthcare?
What are the future trends in AI and data governance?
How do healthcare organizations adopt generative AI?
Want to learn how artificial intelligence can bring more transparency and trust to your operations?