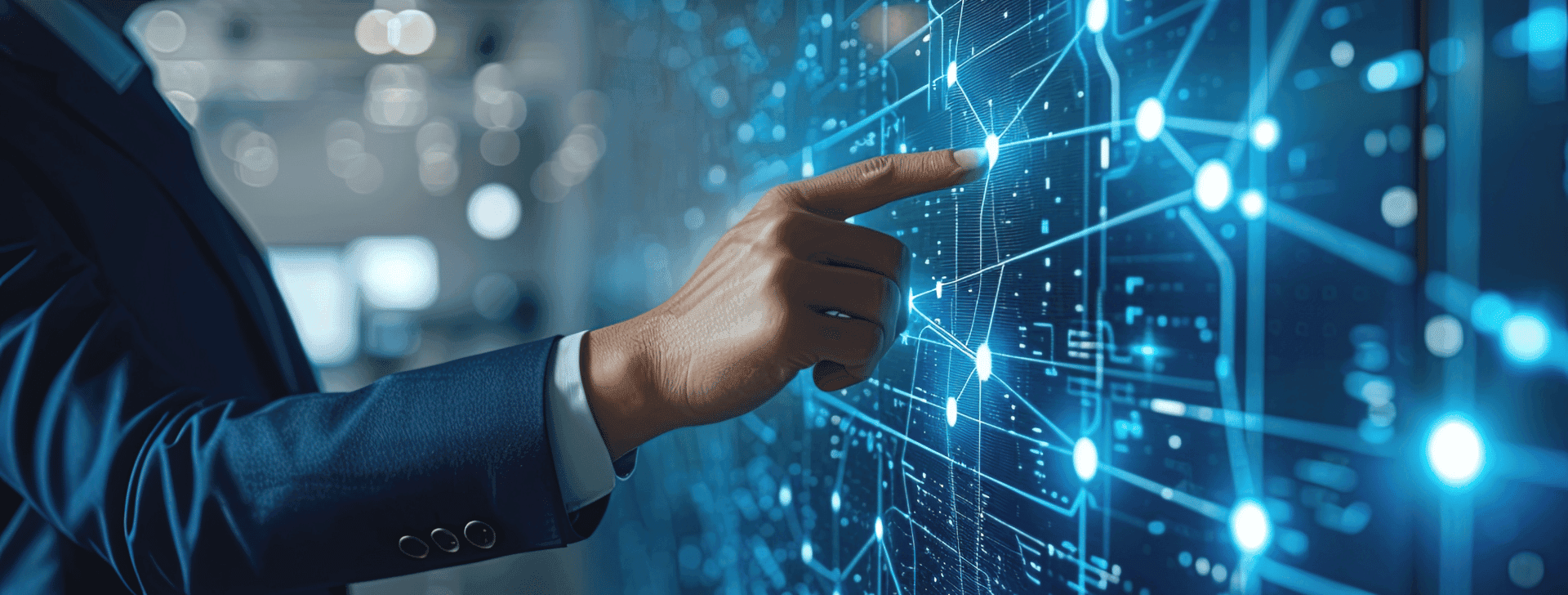
9 AI problems in 2025: Common challenges and solutions
AUG. 20, 2024
9 Min Read
Artificial intelligence (AI) offers tremendous potential, but it also comes with its own set of challenges.
As businesses increasingly adopt AI technologies, addressing these issues becomes crucial to achieving long-term success. Whether dealing with the lack of transparency in AI models, ethical concerns, or difficulties with data management, the obstacles are significant but not insurmountable. Understanding these AI problems and finding appropriate solutions is essential to maximizing the value of artificial intelligence.
Key takeaways
- 1. Lack of transparency in AI models can be solved with Explainable AI (XAI).
- 2. Data privacy concerns are addressed by technologies like privacy-preserving AI.
- 3. Bias in AI models is reduced through fairness-aware algorithms and data audits.
- 4. Upskilling staff and using AI-as-a-Service platforms can meet the demand for AI skills.
- 5. Hybrid models bridge the gap between legacy systems and AI technologies.
Below are the most common artificial intelligence problems in 2025 and the practical solutions companies can adopt to overcome them, ensuring that the age of artificial intelligence delivers on its promises.
1. Lack of transparency in AI models
One of the biggest problems of AI is the lack of transparency in how models make decisions. This issue, often referred to as the “black box” problem, arises when AI systems—particularly those using machine learning and deep learning algorithms—operate in ways that are not easily explainable to human users. For industries like healthcare, finance, and law, understanding how decisions are made is critical for compliance and trust.
- The challenge: Business leaders and regulators require clear, explainable models, especially in critical industries such as healthcare, finance, and legal sectors. Lack of transparency can erode trust and make it harder for organizations to comply with regulations.
- The solution: Investing in explainable AI (XAI) systems can solve this issue. XAI improves the interpretability of machine learning models, allowing stakeholders to understand AI-driven decisions. By incorporating interpretability into AI development, businesses can ensure that their models are more transparent and meet regulatory standards.
By prioritizing explainable AI solutions, businesses can unlock the potential of AI while maintaining accountability and regulatory compliance. This sets the stage for AI systems that not only perform well but are also trusted by users and regulators alike.
"Lack of transparency in AI models can erode trust and make it harder for organizations to comply with regulations."
2. Data privacy concerns
Data privacy is one of the most critical issues and challenges in artificial intelligence. AI systems often require vast amounts of data, but collecting, storing, and processing sensitive information can lead to security risks.
Data privacy is a crucial issue in today's digital landscape, especially as AI models rely on vast amounts of personal and sensitive data. With the rising number of data breaches and the introduction of stringent regulations like GDPR, ensuring that AI systems respect privacy is more important than ever. Companies face a complex balancing act between harnessing data for AI advancements and maintaining compliance with data protection laws.
- The challenge: AI models may inadvertently breach privacy regulations, especially with personal data, potentially leading to legal penalties and damaged reputation. As more regions implement stringent data laws like GDPR, businesses face increasing pressure to ensure compliance.
- The solution: To mitigate these risks, companies must prioritize data governance frameworks. This includes data encryption, anonymization, and adhering to privacy standards. Implementing privacy-preserving AI technologies such as federated learning allows businesses to train AI models without sharing sensitive data, safeguarding both user privacy and model performance.
To overcome these privacy concerns, adopting privacy-preserving AI strategies such as federated learning and data anonymization can help companies achieve compliance without compromising the effectiveness of their AI systems. With these strategies in place, AI can be used responsibly and securely.
3. Bias in AI models
Artificial intelligence problems and solutions often center on bias. Bias in AI models is a critical issue that can have far-reaching implications for fairness and equality.
When AI is trained on biased or unrepresentative data, it can perpetuate or even exacerbate discrimination in areas like hiring, lending, and criminal justice. Addressing bias is essential for ensuring that AI systems are ethical and provide equitable outcomes across all demographics.
- The challenge: Biased AI systems can perpetuate inequality, leading to unfair outcomes in areas like hiring, lending, and law enforcement. The reliance on biased data can damage the credibility of AI, making it hard to trust automated decisions.
- The solution: To reduce bias, businesses should implement rigorous bias detection and mitigation strategies during the development phase. Regular auditing of data sources, training diverse datasets, and using fairness-aware machine learning algorithms can help reduce bias and promote fairness in AI systems.
By investing in bias detection tools, using diverse datasets, and adopting fairness-aware algorithms, companies can reduce the risks associated with biased AI. Building more ethical AI systems will improve trust and ensure that these technologies work for everyone.
4. The high demand for AI skills
The rapid progress in artificial intelligence has resulted in a demand for artificial intelligence skills that far outstrips supply. Many organizations are eager to implement AI but struggle to find qualified professionals with expertise in machine learning, data science, and AI engineering.
This shortage can stall AI adoption, limit innovation, and prevent companies from realizing the full potential of AI.
- The challenge: Many companies face difficulties hiring the right talent, from data scientists to machine learning engineers, which slows down AI adoption and implementation.
- The solution: Companies can bridge the talent gap by investing in upskilling and reskilling programs. Collaborating with universities, offering internships, and providing ongoing AI training for existing staff can help meet the demand for AI expertise. In addition, adopting AI-as-a-Service platforms allows businesses to leverage AI technologies without needing an in-house team of experts.
Bridging the AI talent gap through upskilling and reskilling programs, partnerships with educational institutions, and leveraging AI-as-a-Service platforms can empower organizations to build AI solutions without the need for an in-house team of AI specialists. This approach allows businesses to stay competitive in the age of artificial intelligence.
5. Integration with legacy systems
Many organizations rely on legacy systems that are not compatible with modern AI technologies. These outdated systems often lack the flexibility and scalability needed to handle AI workloads, creating a significant barrier to AI adoption. For businesses, the challenge is finding ways to integrate AI into their existing infrastructure without overhauling the entire system.
- The challenge: Many enterprises still rely on outdated systems that cannot effectively handle AI technologies, leading to delays and complications in AI deployment.
- The solution: Businesses need to adopt hybrid models that blend legacy systems with new AI infrastructure. Modern APIs, cloud services, and middleware solutions can act as a bridge between old and new technologies, allowing for smoother AI integration without the need for a complete overhaul of existing systems.
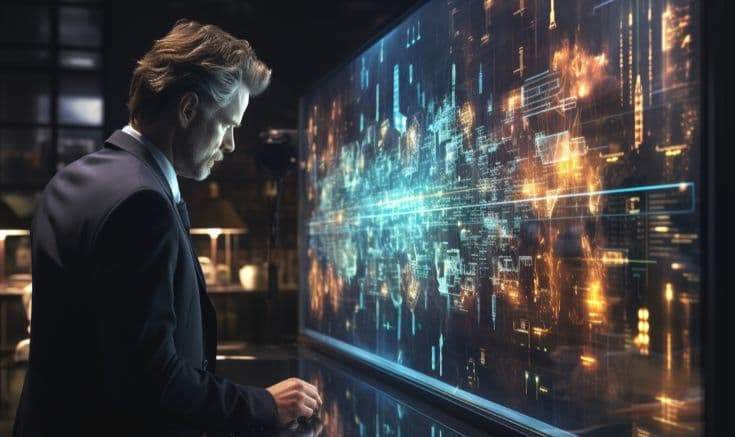
To overcome this, businesses should adopt hybrid models that connect legacy systems with modern AI solutions through APIs and middleware. This approach enables companies to benefit from AI without incurring the high costs and disruptions associated with completely replacing legacy systems.
"Bias in AI models is a critical issue that can have far-reaching implications for fairness and equality."
6. The ethics of AI usage
As AI becomes more powerful and widespread, ethical concerns around its usage have come to the forefront. Issues like data privacy, bias, and accountability raise serious questions about the ethical implications of AI in decision-making. Businesses must ensure that their AI systems are aligned with ethical standards to maintain trust and avoid misuse.
- The challenge: Without ethical AI frameworks, businesses risk using AI in ways that violate human rights, promote inequality, or erode consumer trust.
- The solution: Companies must develop AI ethics guidelines to govern the use of AI technologies. This includes creating ethical committees, ensuring transparency in AI decision-making, and adhering to international ethical standards for AI use. By adopting responsible AI practices, businesses can mitigate ethical risks and build trust with users and customers.
Developing a robust framework for AI ethics guidelines and governance is essential for responsible AI deployment. By taking steps to implement transparent and ethical AI practices, companies can lead the way in creating technologies that benefit society while minimizing risks.
7. High costs of AI implementation
The financial barrier to AI adoption remains a significant challenge for many companies, particularly small to medium-sized enterprises (SMEs). The costs associated with AI infrastructure, talent acquisition, and data management can be prohibitive, preventing businesses from fully embracing AI technologies. Finding a cost-effective way to implement AI is crucial for companies that want to stay competitive without breaking the bank.
- The challenge: AI technologies require substantial investment in infrastructure, talent, and data management, which can be prohibitively expensive for small to medium-sized enterprises.
- The solution: To overcome cost challenges, businesses can start small with pilot AI projects before scaling up. Leveraging cloud-based AI services also provides cost-effective access to AI capabilities without the need for heavy capital investment in infrastructure.
Starting with small-scale pilot AI projects and using cloud-based AI services can significantly reduce the upfront costs of AI implementation. These strategies allow businesses to explore AI's benefits in a cost-effective way, scaling up as they see positive returns on their investment.
8. AI in physical intelligence
Physical intelligence AI focuses on enabling machines to perform tasks that require physical interaction with the environment, such as robotics and autonomous vehicles. However, many challenges arise when AI systems must adapt to unpredictable real-world conditions, making physical intelligence one of the more complex areas of AI development.
- The challenge: Physical intelligence AI faces limitations in areas like robotics, where machines must navigate and interact with unpredictable environments. The complexity of physical tasks often requires AI systems to adapt in real-time, which can be difficult for current AI technologies.
- The solution: Improving AI capabilities in robotics and other physical domains requires advancements in sensor technologies and adaptive learning algorithms. By integrating machine learning with advanced robotics, companies can develop AI systems capable of handling more complex physical tasks.
Advances in sensor technology and adaptive learning algorithms are essential to improving AI’s physical capabilities. By continuing to invest in these technologies, businesses can unlock new opportunities for AI-driven robotics and automation in industries ranging from manufacturing to healthcare.
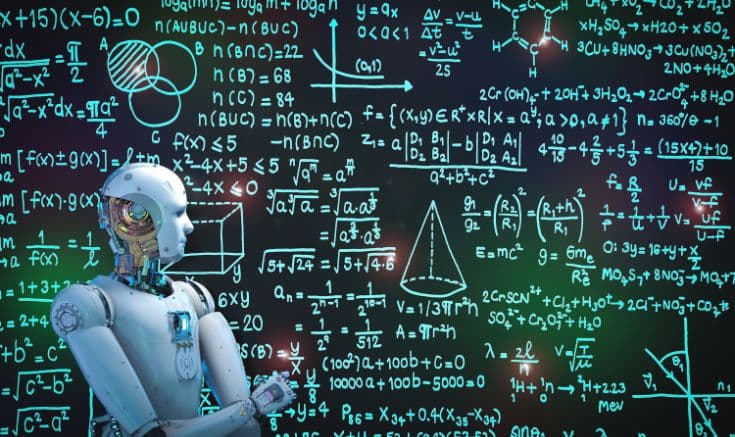
9. Resistance to AI adoption
Internal resistance to AI adoption is a common challenge for organizations, often stemming from fears of job displacement or uncertainty about how AI will impact workflows. Without buy-in from employees and leadership, AI projects can stall or fail to achieve their full potential.
- The challenge: Resistance to AI within organizations can slow down implementation, particularly when employees are concerned about automation leading to job loss.
- The solution: To ease resistance, businesses should focus on change management strategies that highlight AI’s role in augmenting human work rather than replacing it. Clear communication about how AI will complement and enhance jobs, rather than eliminate them, can help employees embrace AI adoption.
Addressing these concerns through transparent communication and change management strategies can help ease resistance. By highlighting how AI can augment, rather than replace, human roles, businesses can foster a culture that embraces AI adoption and innovation.
The problems of artificial intelligence are significant, but by adopting the right strategies, businesses can overcome these challenges. Whether addressing transparency issues, ethical concerns, or data privacy, finding the right solutions ensures that AI continues to evolve in a way that benefits both organizations and society. As the age of artificial intelligence progresses, overcoming these hurdles will be key to unlocking AI’s full potential.
Common questions about AI problems
What are the common problems of artificial intelligence today?
How do businesses handle bias in AI models?
What are the solutions to AI problems in data privacy?
How can AI be integrated with legacy systems?
What are the ethical concerns in AI development?
How can small businesses overcome the high costs of AI implementation?
Want to learn how artificial intelligence can bring more transparency and trust to your operations?