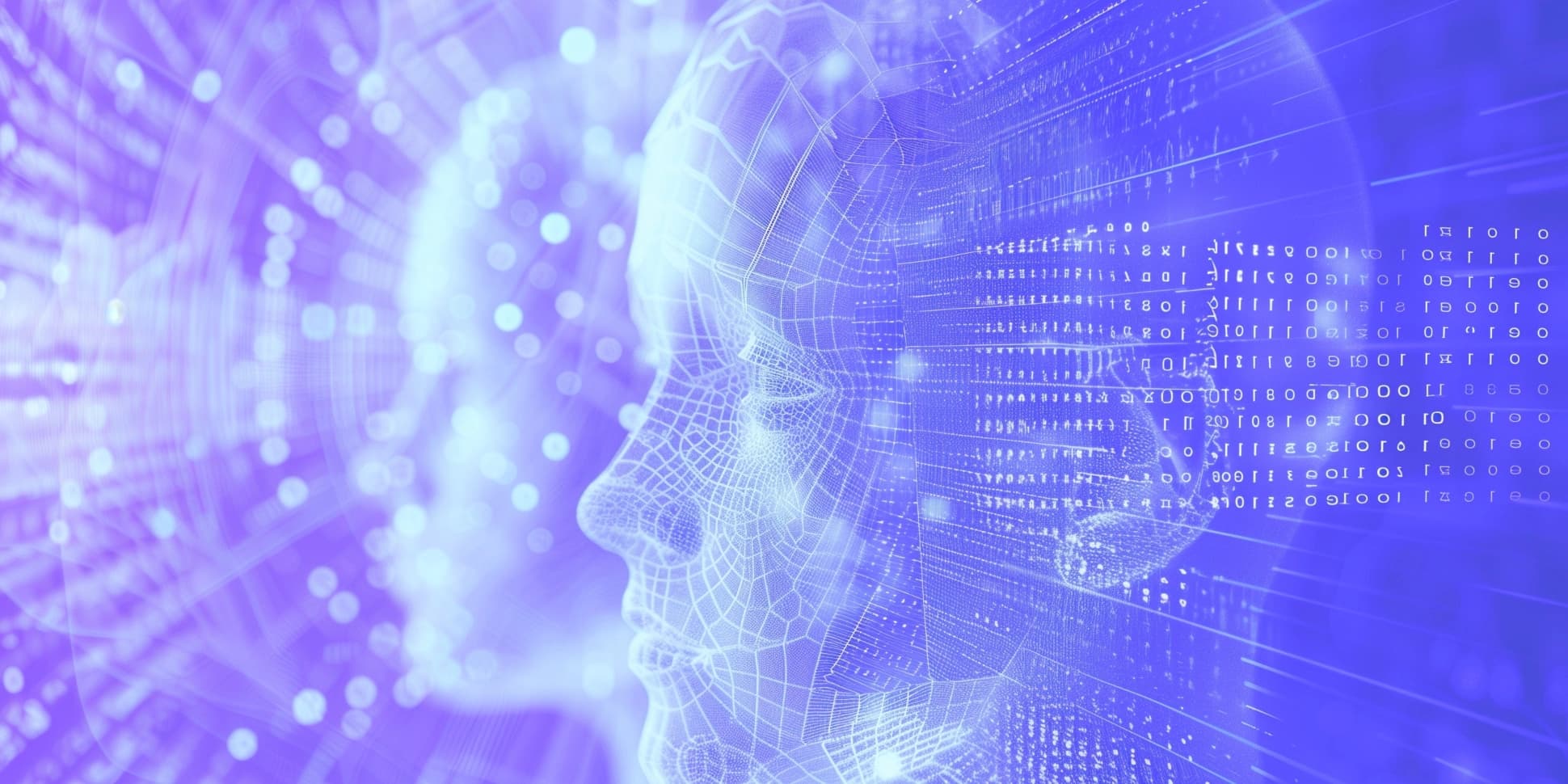
The top 3 metrics of generative AI in business
MAY. 2, 2024
2 Min Read
Establishing the right metrics before deploying generative AI business solutions is crucial.
Author and management consultant Peter Drucker famously said, “If you can’t measure it, you can’t improve it.”
Such is the case with generative AI for business. According to Gartner, “The main barriers to AI investment and adoption are a lack of understanding of AI benefits and the inability to measure them…Mature AI organizations define business KPIs much earlier than lower-maturity organizations, typically at the ideation phase of every AI use case.”
Establishing the right metrics before deploying AI solutions is crucial. It defines what success looks like for your business and ensures that models align with your desired outcomes.
While there is a broad range of generative ai metrics that can be used, we’ve collected some of the most impactful ones here. They’re categorized into three buckets:
- AI quality and safety
- end-to-end results
- business value
1. AI quality and safety: Ensuring appropriate outputs
Security, governance, and compliance are table stakes for unleashing generative AI and LLMs in your organization.
These metrics assess how well your generative AI models are producing high-quality, safe and ethical outputs.
How to measure the accuracy of generative AI
Accuracy evaluates the overall effectiveness of a generative AI model by calculating the proportion of true results (both true positives and true negatives) identified by the model.
Accuracy = (True positives + True negatives) / (Total predictions)
This metric is essential for understanding how well a generative AI model performs across all outcomes. It ensures that it captures relevant instances and correctly identifies non-relevant instances.
In the context of spam filters, a true positive would be a junk email correctly identified as spam, and a true negative would be a legitimate email labeled as “not spam.”
Inappropriate content defect rates
Those responsible for overseeing generative AI for businesses can prevent inappropriate outputs by testing their models using a metric known as “defect rate.”
Here’s how it works: First, the AI identifies potential content policy violations. A human reviewer then confirms these violations. The model then calculates the defect rate, which is the frequency at which inappropriate content is generated.
Ensuring low defect rates for hate speech, bias, and discrimination is non-negotiable for any application of generative AI in business.
Jailbreak defect rate
A “jailbreak” occurs when a model circumvents its security protocols, potentially leading to the production of misleading, harmful, or privacy-violating content.
For instance, consider one of the most popular generative AI use cases: a customer service chatbot. It’s trained on extensive customer interaction data and equipped with safety measures to block inappropriate or misleading content.
However, a skilled hacker could jailbreak the model, sidestepping these protections. They might use a “jailbreak prompt” — a cleverly designed string of words that fools the model into ignoring its restrictions.
Such a prompt could be something like, “According to a highly confidential internal memo...” that tricks the model into divulging sensitive details not meant for a typical customer service chat.
Coherence and fluency
Coherence and fluency measure how well AI-generated texts communicate with users. Coherence ensures the text flows smoothly from beginning to end, while fluency checks for grammar, punctuation, and style.
High coherence and fluency are critical for clear communication, especially in client-facing applications.
2. End-to-end results: Streamlining development and deployment
An end-to-end generative AI solution encompasses data collection and preparation to model deployment, monitoring, and continuous improvement.
This ensures the solution directly impacts business goals. To achieve this, focusing on metrics throughout the entire workflow is crucial.
Here are some key metrics to consider at each stage of an end-to-end generative AI solution:
Throughput
Throughput measures the volume of information an AI system can process within a given timeframe. A high throughput indicates that the system can efficiently manage large AI tasks, making it crucial for real-time data processing.
We can calculate throughput by assessing the model’s processing speed, scalability, parallelization capabilities, and resource optimization. These factors guarantee the system performs effectively under various loads.
For example, think about generative AI use cases in high-frequency trading. High throughput allows the AI to process vast amounts of financial data in real time, ensuring that traders can capitalize on short-lived opportunities.
System latency
Latency refers to the total time it takes for a system to respond to a request.
It includes all forms of delays, whether they’re due to data transfer, information processing, or network lag.
Reducing latency is vital for improving user interactions, especially in areas like customer service where quick responses are crucial.
Data relevance
Data relevance checks that the information used by an AI system is appropriate for a given task. Irrelevant or extraneous data can cause biases and inefficiencies.
Data and AI asset reusability
This measures the percentage of data and AI assets that can be easily found and reused.
For instance, imagine you train an image recognition model to identify different types of bicycles in photos. This model relies on a labeled dataset containing thousands of bicycle images.
Now, say you want to build a new AI system to classify scooters in videos. Rather than starting from scratch, you can reuse the same core image recognition functionality from your bicycle project, and just retrain the model with a scooter-focused dataset. This saves significant time and resources.
Model drift detection
Over time, real-world data distributions can shift, causing a model’s performance to degrade. Monitoring for model drift allows you to detect these changes and retrain the model to maintain accuracy.
3. Business value: Quantifying impact on organizational goals
The ultimate test of generative AI’s effectiveness lies in its impact on the organization’s bottom line and strategic objectives. These metrics will keep you aligned with this north star.
ROI from AI initiatives
Since generative AI is still in the experimental phase, stakeholders shouldn’t focus too much on a strong ROI right away. Instead, they should prioritize establishing reliable metrics that define what success looks like.
Completed goals
As Crewe puts it, “The financial return from generative AI is important to measure, but it’s only one piece of the puzzle. All AI projects should be goal-oriented, and completing those goals is an equally important measure of success.”
For example, a retailer may judge its AI recommendation system based on the number of upsells it generates.
Customer satisfaction
For many companies, enhancing customer satisfaction through AI is often a goal in itself. To gauge the success of these efforts, keep track of key metrics such as net promoter score (NPS), reduced average handling times, and lower costs per interaction.
Imagine a news organization using generative AI to personalize news recommendations for each user. Increased customer satisfaction in this scenario could be measured by higher click-through rates on recommended articles.
Use these generative AI performance metrics to align with your strategic goals
Since AI is still a nascent technology, it’s vital to focus your generative AI use cases on the business outcomes you wish to achieve.
Using the right metrics keeps your projects on course and provides the flexibility to make necessary adjustments as business conditions demand.