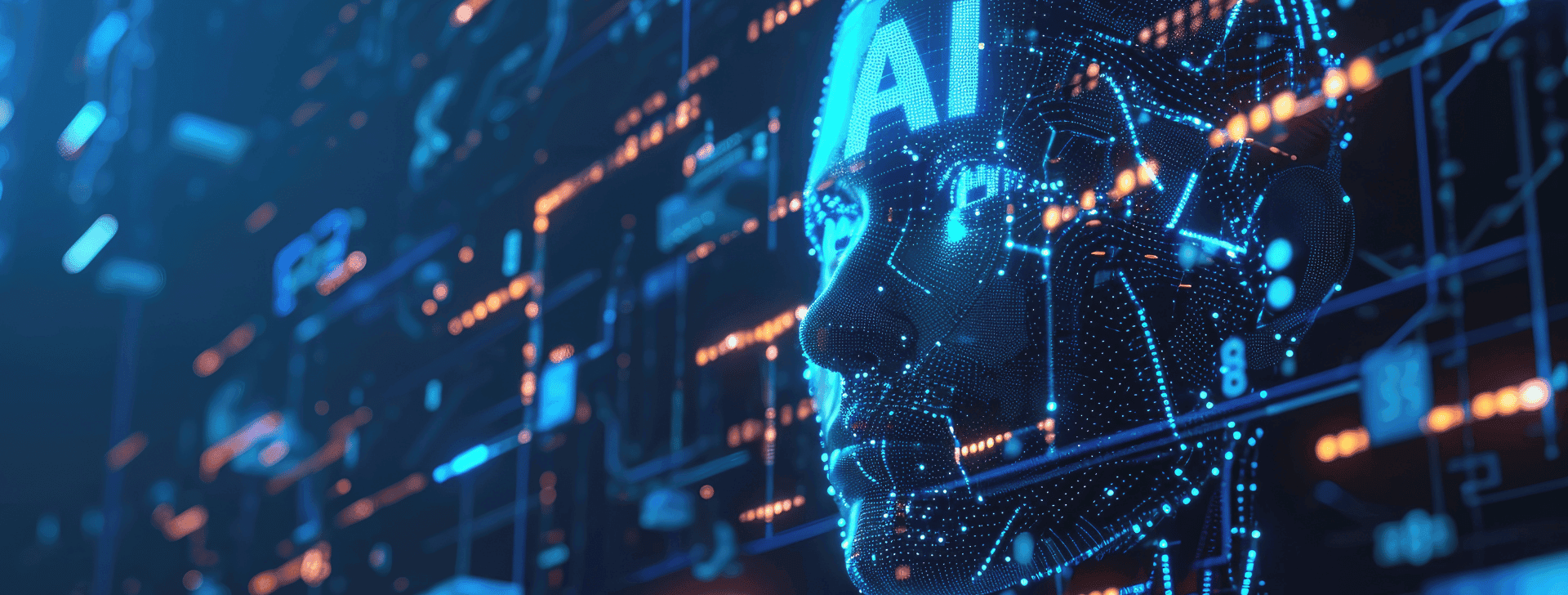
What is AI? An overview of artificial intelligence (Updated 2025)
SEP. 12, 2024
12 Min Read
AI is not a single technology but a collection of technologies that include machine learning (ML), natural language processing (NLP), robotics, and computer vision.
At its core, artificial intelligence (AI) refers to systems and machines capable of performing tasks that would traditionally require human cognition, such as reasoning, learning, problem-solving, perception, and even linguistic understanding.
Artificial intelligence is reshaping industries across the globe by mimicking human intelligence in machines to perform tasks that require governance, learning, and adaptation. As AI technology grows, its ability to process vast amounts of data and improve over time is unlocking unprecedented opportunities for businesses to modernize, innovate, and remain aggressive.
Key takeaways
- 1. AI technology refers to systems and machines capable of performing tasks that traditionally require human cognition, such as reasoning, learning, problem-solving, perception, and understanding.
- 2. AI has evolved from simple systems to sophisticated technologies like machine learning and deep learning, transforming industries with its ability to mimic human intelligence.
- 3. AI offers numerous benefits, including operational efficiency, cost savings, and enhanced decision-making, but it also comes with challenges such as ethical concerns and high implementation costs.
- 4. Narrow AI focuses on specific tasks, while AGI (Artificial General Intelligence) remains theoretical, aiming for human-like intelligence capable of solving broader, more complex problems.
- 5. The AI Bill of Rights was introduced to address concerns over AI's impact on society, focusing on fairness, transparency, and accountability in AI systems.
How does AI work?
AI works by using algorithms to analyze vast amounts of data, recognize patterns, and make predictions or decisions. It encompasses various approaches, including supervised learning (where AI learns from labeled data), unsupervised learning (where AI identifies hidden patterns in data), and reinforcement learning (where AI learns through trial and error).
Key steps in AI operation:
- Data input: AI systems start by ingesting large amounts of data.
- Processing: Algorithms analyze the data, identify patterns, and make predictions or decisions.
- Learning: AI systems continuously learn from new data, refining their outputs for improved accuracy over time.
- Outputs: These systems produce results, which can be predictions, classifications, or actions based on their learning.
By processing data through these steps, AI systems can perform tasks like image recognition, customer service automation, and even autonomous driving.
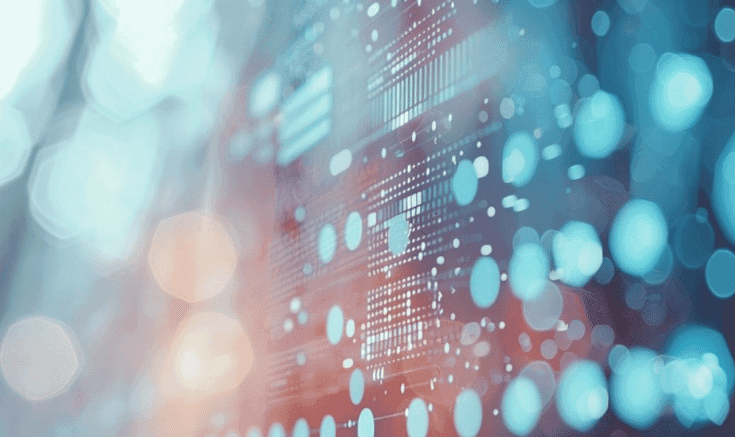
Types of AI
AI can be categorized into different types based on its complexity and functionality:
- Reactive machines: Responds to specific stimuli without memory or learning
- Limited memory AI: Learns from past data to improve decisions over time
- Theory of mind AI: Can understand emotions and mental states (still in development)
- Self-aware AI: Hypothetical AI that possesses consciousness (yet to be realized)
These types of AI represent various stages in the evolution of artificial intelligence, from simple systems that follow predefined rules to more advanced, human-like cognition.
Benefits of AI
AI offers significant advantages across industries, enhancing operational efficiency, evaluation, and customer experience. These benefits can be categorized into the following areas:
1. Operational efficiency
AI optimizes workflows by automating repetitive tasks, allowing employees to focus on more strategic initiatives. For instance, in manufacturing, AI reduces equipment downtime through predictive maintenance, while in retail, AI-led automation streamlines supply chain management.
2. Evaluation
AI's ability to process large datasets enables organizations to make data-based decisions faster and with greater accuracy. For example, AI systems in finance help identify fraud by analyzing real-time transaction data, reducing human error and response time.
3. Cost savings
By automating tasks and improving accuracy, AI significantly reduces operational costs. In sectors like healthcare, AI-led diagnostics lower the costs associated with medical testing and analysis.
4. Personalization
AI enhances customer engagement through personalized experiences. By analyzing consumer behavior, AI algorithms recommend products, tailor marketing messages, and improve customer retention rates.
"Artificial intelligence is reshaping industries across the globe by mimicking human intelligence in machines to perform tasks that require governance, learning, and adaptation."
Limitations of AI
While AI delivers impressive benefits, it is not without its limitations. Several challenges must be addressed to fully realize AI’s potential:
1. Lack of common sense
AI systems lack the ability to understand complex contexts. Despite their advanced data processing capabilities, they cannot yet replicate human intuition and regulation. This limitation makes AI less reliable in scenarios requiring nuanced judgment.
2. Data dependency
AI models rely heavily on high-quality data for training. Poor-quality, biased, or insufficient data can skew AI's results, leading to inaccurate predictions or biased outcomes. This is particularly problematic in applications like hiring or lending, where bias can have significant consequences.
3. Ethical concerns and transparency
The "black box" nature of many AI systems, particularly deep learning models, makes it difficult to understand how decisions are made. In areas like healthcare or finance, this lack of transparency can raise concerns about accountability. Furthermore, AI can perpetuate biases present in its training data, leading to unfair outcomes.
4. High implementation costs
AI solutions can be expensive to develop and implement. Organizations must invest in both, the technology and skilled personnel to manage AI systems, making it challenging for smaller businesses to adopt AI at scale.
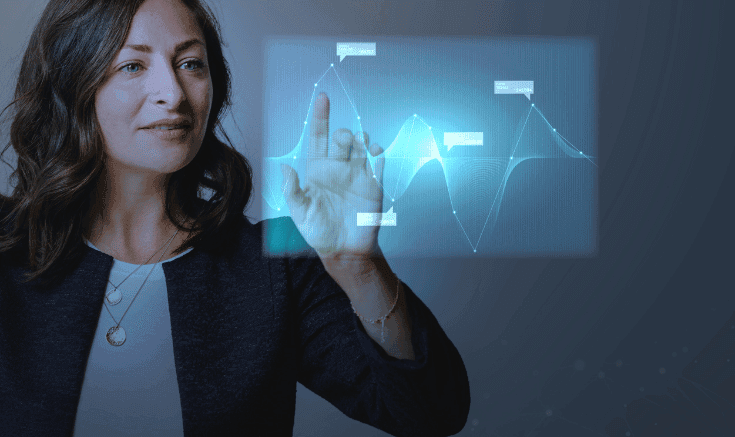
Challenges and risks of AI adoption
Implementing AI presents challenges and risks that organizations must navigate to successfully leverage its potential. Key challenges include:
- Data privacy: As AI systems handle vast amounts of data, ensuring compliance with data protection regulations (e.g., GDPR) becomes critical. Companies must prioritize data security to maintain consumer trust.
- Skill gaps: The shortage of skilled professionals who understand AI technology poses a barrier to adoption. Organizations must invest in training and upskilling their workforce to bridge this gap.
- Regulatory compliance: As AI grows, governments are developing frameworks to regulate its use. Businesses must stay informed of changing regulations and ensure compliance.
AI use cases
Artificial Intelligence (AI) is redefining industries by automating tasks, providing insights, and enhancing management processes. Beyond the examples already mentioned, additional use cases illustrate the broad impact of AI on various sectors:
Healthcare
AI is revolutionizing healthcare through advanced diagnostics, personalized medicine, and operational efficiency. Machine learning models analyze vast datasets, including medical records and genetic information, to assist in early disease detection and precision treatment planning. AI-driven predictive analytics help track disease outbreaks and identify at-risk populations. Additionally, AI-powered robotics assist in surgeries, improving precision and reducing recovery times.
Retail
Retailers leverage AI to enhance customer experiences, optimize operations, and drive profitability. AI-powered recommendation engines analyze customer behavior to deliver personalized shopping experiences. AI is also used in inventory management, minimizing waste by predicting demand fluctuations. Dynamic pricing algorithms adjust prices in real-time based on market trends, competitor pricing, and consumer demand, helping businesses maximize revenue.
Energy
AI plays a critical role in energy optimization and sustainability. In power grids, AI predicts consumption patterns and dynamically adjusts distribution to prevent outages and reduce waste. Smart grid technology uses AI to balance supply and demand efficiently. In the renewable energy sector, AI-driven forecasting models analyze weather patterns to maximize solar and wind energy generation, improving overall grid stability.
Agriculture
AI is reshaping modern farming with precision agriculture technologies. AI-powered drones and satellite imagery assess crop health, detect diseases early, and optimize water and fertilizer use. Machine learning models analyze soil conditions and weather data to provide farmers with actionable insights, enhancing yield predictions and reducing resource waste. Automated machinery, such as AI-driven harvesters, increases efficiency and reduces labor costs.
Transportation
AI is driving efficiency in transportation and logistics through predictive analytics and automation. Autonomous vehicles and AI-powered traffic management systems optimize routes, reducing congestion and fuel consumption. AI-based fleet management enables logistics companies to track vehicle conditions, predict maintenance needs, and minimize downtime. In supply chain logistics, AI enhances demand forecasting and warehouse automation, ensuring timely deliveries and cost reductions.
As AI technology continues to advance, its applications will only grow more diverse. From improving healthcare outcomes to optimizing supply chains, AI is becoming an indispensable tool for industries looking to stay ambitious.
What is machine learning & deep learning?
Machine learning and deep learning are two core technologies underpinning the advancement of artificial intelligence. These fields have grown exponentially in recent years, driving innovations in sectors ranging from healthcare and finance to autonomous driving and customer service. While both fall under the umbrella of AI, machine learning and deep learning differ in their complexity, methodologies, and applications. Understanding these distinctions is crucial for businesses looking to leverage AI for improved evaluation, automation, and personalized services.
Machine learning
Machine Learning refers to systems that learn from data without requiring explicit programming. Rather than being fed specific instructions, ML algorithms identify patterns in datasets and make predictions based on statistical techniques. This flexibility makes ML suitable for a wide array of tasks, such as recommendation engines, fraud detection, and customer segmentation.
A classic example of ML in action is its use in e-commerce. Platforms like Amazon or Netflix use ML to recommend products or shows by analyzing user behavior and preferences. Similarly, in the financial industry, ML-powered algorithms can detect fraudulent transactions by spotting anomalies in spending patterns. Unlike traditional algorithms, which operate based on predefined rules, machine learning develops and improves as it processes more data, resulting in accurate outputs.
ML is typically divided into three types:
- Supervised learning: The system is trained on labeled data, learning from examples to predict outcomes based on input data.
- Unsupervised learning: The algorithm identifies hidden patterns in unlabeled data, commonly used in customer segmentation and clustering.
- Reinforcement learning: The algorithm learns by trial and error, receiving feedback through rewards or penalties to maximize performance. This is crucial for applications like autonomous vehicles.
Deep learning
Deep learning is a specialized subset of machine learning that mimics the neural networks of the human brain to analyze and process data. Using multi-layered neural networks, deep learning can model complex patterns in data, making it especially useful for image recognition, speech processing, and language translation. It powers advanced technologies like facial recognition systems, virtual assistants (e.g., Alexa or Siri), and autonomous driving systems.
One of the primary reasons deep learning has surged in popularity is its ability to perform tasks at a scale and accuracy level that traditional ML models cannot match. For instance, deep learning models can process vast amounts of unstructured data—like images or videos—much faster and with higher precision. The algorithm gets stronger as it learns from large datasets, thanks to the multiple layers within its neural network that allow for higher levels of abstraction and complexity.
In actual applications, deep learning has revolutionized industries like healthcare, where it is used to detect diseases in medical images more accurately than human doctors. It's also integral to natural language processing (NLP), allowing machines to understand and respond to human language in customer service chatbots or virtual assistants.
Machine learning and deep learning both play critical roles in the development of AI, permits businesses to use the power of data for enhanced regulation, customer experiences, and operational efficiency. As AI continues to advance, the capabilities of ML and deep learning will expand, opening up new possibilities for innovation.
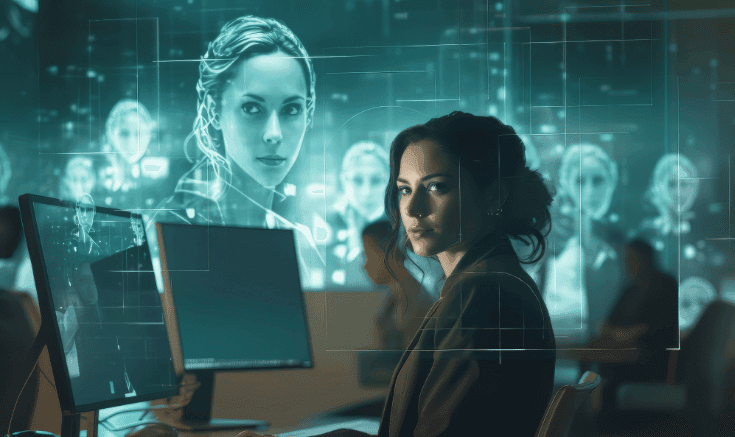
What is generative AI (Gen AI) & artificial general intelligence (AGI)?
Artificial intelligence is constantly changing, and two emerging areas capturing significant attention are generative AI and artificial general intelligence. Generative AI focuses on creating new content using algorithms, while AGI is a more theoretical concept involving AI systems that possess the same intellectual capabilities as humans. Both fields are pivotal in shaping the future of AI, although they have distinct objectives and challenges.
Generative AI
Generative AI refers to algorithms designed to create new content, such as text, images, music, or even videos, based on input data. These AI models are capable of generating outputs that mimic human creativity, using vast datasets to learn patterns and apply them in innovative ways. Some well-known generative AI systems include ChatGPT and DALL-E, both of which utilize deep learning models to produce text and images, respectively.
Generative AI has applications across industries:
- Content creation: In marketing, generative AI is used to produce personalized marketing content, helping companies connect more effectively with their audiences. Automated content generators can draft emails, articles, or even scripts, saving time and resources for businesses.
- Design and art: Tools like DALL-E enable designers to create images from textual descriptions, modifying fields such as graphic design, fashion, and animation. Designers can generate creative concepts or visual elements without needing to manually sketch or prototype ideas.
- Customer service: AI models like ChatGPT provide real-time responses to customer queries, simulating human-like conversation for a more engaging customer experience.
The potential of generative AI is vast, but it also poses ethical challenges, particularly around issues like intellectual property, misinformation, and the spread of deepfakes. As this technology becomes more sophisticated, businesses will need to address these concerns while utilizing its benefits.
Artificial general intelligence
Artificial General Intelligence, often referred to as strong AI, represents a more ambitious frontier of AI development. Unlike narrow AI, which is designed to perform specific tasks, AGI would theoretically have the ability to perform any cognitive task a human can, including reasoning, problem-solving, and abstract thinking. While AGI remains largely speculative, researchers are exploring how to develop AI systems that possess true cognitive flexibility.
Achieving AGI would require breakthroughs in multiple fields, including neuroscience, computer science, and cognitive psychology. Unlike generative AI, which already has practical applications, AGI is still in its infancy, with most experts predicting that it could take decades, if not centuries, to realize.
One of the major ethical considerations surrounding AGI is the potential impact it could have on society. If AGI were to reach a level of intelligence surpassing human capabilities, it could challenge existing social, economic, and legal systems. Some experts advocate for the development of strict regulatory frameworks to manage the risks posed by AGI.
While generative AI is already redeveloping industries by enhancing creativity and automation, AGI remains a theoretical concept with potentially far-reaching consequences. Businesses should stay informed about developments in both fields to understand how they may impact the future of AI and its role in society.
Narrow AI vs. artificial general intelligence
Artificial intelligence can be broadly classified into two categories: Narrow AI and Artificial General Intelligence. Narrow AI is already prevalent in various industries, designed to perform specific tasks such as image recognition, language processing, and customer service. In contrast, AGI remains a theoretical concept, with the goal of achieving human-level intelligence across multiple domains.
Narrow AI
- Task scope: Performs specific, predefined tasks
- Current use: Common in applications like chatbots, recommendation engines
- Flexibility: Limited to the task it was designed for
- Decision-making: Based on algorithms for particular domains
Artificial General Intelligence (AGI)
- Task scope: Capable of performing a wide range of cognitive tasks
- Current use: Still theoretical, not yet realized
- Flexibility: Able to learn and adapt to new tasks like humans
- Decision-making: Expected to reason, learn, and adapt across domains
While narrow AI is making a tangible impact in areas like customer service and logistics, AGI holds the potential to revolutionize every facet of human life, though its realization is still a long way off. Companies should focus on using narrow AI capabilities today while keeping an eye on the future possibilities of AGI.
History of AI
AI, or artificial intelligence, has a rich history that spans decades of innovation, breakthroughs, and periods of stagnation.
The origins of AI can be traced back to the 1950s, when British mathematician Alan Turing proposed the idea that machines could simulate any aspect of human intelligence. This idea led to the development of the Turing Test, a criterion to determine whether a machine could exhibit intelligent behavior indistinguishable from that of a human.
Key milestones in AI development:
- 1956 - The birth of AI: The term "Artificial Intelligence" was first coined at the Dartmouth Conference, which brought together leading minds to explore the potential of machines simulating human intelligence.
- 1960s-1980s - Expert systems and robotics: This period saw the rise of expert systems, where AI programs were used to solve specific problems in fields like medical diagnosis. Robotics also began to make strides during this time.
- 1980s-1990s - Neural networks: The development of neural networks revolutionized AI, allowing machines to learn from data and make more accurate predictions. These early networks laid the foundation for modern deep learning techniques.
- 2000s - Machine learning revolution: As computing power increased, AI systems could process massive datasets more efficiently. Machine learning emerged as a powerful subfield of AI, allowing more advanced data analysis and automation.
- 2010s - Deep learning and AI integration: The explosion of deep learning enabled AI systems to achieve higher levels of accuracy in tasks like image recognition, natural language processing, and autonomous driving.
- Present day - Generative AI and AGI aspirations: With the rise of generative AI, which creates new content, and ongoing discussions about artificial general intelligence, AI continues to push boundaries across industries.
AI has come a long way since its inception, and as new technologies emerge, its future potential only continues to grow. From expert systems to deep learning and beyond, AI will remain at the forefront of technological evolution, reshaping industries and society at large.
What does AI mean for businesses?
AI is shaping how businesses operate, offering solutions ranging from automating routine tasks to enhancing customer engagement. The integration of AI into business processes is no longer optional but a necessity for companies looking to remain aggressive in a digital setting.
- Customer service: Chatbots providing 24/7 support
- Operations: Predictive maintenance reducing downtime
- Marketing: Personalization through recommendation engines
- Finance: Fraud detection using real-time data analysis
- Supply chain: Optimizing logistics and inventory management
AI is a catalyst for digital transformation, helping businesses achieve operational efficiency, improve customer satisfaction, and stay eager. As AI technologies continue to advance, companies that adopt and integrate AI into their strategies will see the most significant benefits in both the short and long term.
"AI's ability to process large datasets enables organizations to make data-based decisions faster and with greater accuracy."
How is the use of AI expanding?
The use of AI is growing as businesses across industries recognize its potential to enhance operational efficiency, drive innovation, and unlock new opportunities. From automating repetitive tasks to offering real-time management capabilities, AI is shaping the way companies operate and serve their customers.
Key drivers of AI expansion include:
- Automation: AI streamlines workflows, automating repetitive tasks and allowing human workers to focus on strategic initiatives.
- Enhanced decision-making: AI systems analyze data faster than humans, allowing companies to make informed decisions based on real-time insights.
- Scalability: AI systems can be scaled to tackle complex problems without requiring proportional resource increases.
- Personalization: By analyzing behavioral data, AI personalizes customer experiences in sectors like e-commerce and entertainment. As AI continues to expand, its role in shaping the future of business cannot be understated. Companies that embrace AI today will be better positioned to innovate, scale, and adapt to the changing market setting of tomorrow.
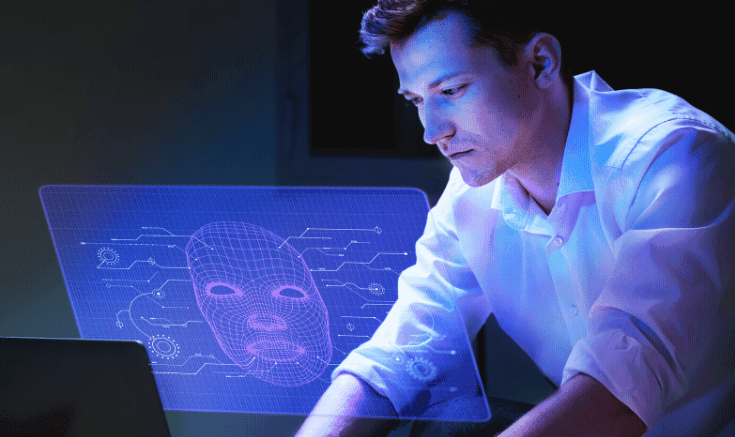
AI ethics and trust
As AI becomes more integrated into daily life, ethical considerations around transparency, bias, and accountability are critical. AI systems, particularly those that influence human welfare, must be designed with fairness in mind. This includes ensuring that AI models are not biased based on race, gender, or socioeconomic factors, and that management processes remain transparent.
For example, AI systems used in recruitment must be carefully monitored to avoid unfair biases in hiring decisions. Regulatory frameworks like the AI Bill of Rights are also being developed to ensure AI technologies align with societal values.
What is the AI Bill of Rights?
The AI Bill of Rights was introduced as a framework to ensure that AI systems are developed and deployed responsibly, with attention to fairness, transparency, and accountability. As AI becomes more pervasive, concerns have emerged about its ethical use, particularly in areas such as privacy, bias, and data security.
Key principles of the AI Bill of Rights include:
- Fairness: AI systems should not perpetuate bias or discrimination, and their decisions should be equitable across all demographic groups.
- Transparency: AI systems should be transparent, allowing users to understand how decisions are made.
- Accountability: There should be mechanisms in place to hold AI developers and users accountable for the outcomes produced by AI systems.
This initiative aims to safeguard human rights in the age of AI and ensure that technological progress benefits society as a whole.
What is AI's future potential?
As AI technology advances, its potential seems limitless. From autonomous vehicles to smart cities, AI will continue to drive innovation across industries. Advances in deep learning and natural language processing are already reshaping fields such as healthcare and finance, while more specialized AI applications, like AI-backed drug discovery, are expected to make significant contributions in the coming decades.
AI applications across industries modernize how businesses operate, innovate, and grow. While AI offers numerous benefits, including operational efficiency and improved evaluation, businesses must also address the ethical and technical challenges associated with its use.
For companies, the question is no longer what is AI, but how can AI be integrated into business strategy to maintain an advantage.
Table of contents
- How AI works
- Types & benefits of AI
- Limitations & challenges of AI
- AI use cases
- What is machine learning and deep learning?
- Generative AI, artificial general intelligence, and Narrow AI
- History of AI
- What AI means for businesses & How it is expanding
- AI ethics & trust
- Future potential of AI
- Common questions about AI
Common questions about artificial intelligence
What is AI, and how does it work?
What does AI stand for, and what are its main types?
What is AI technology capable of doing in business?
What are the differences between AI and machine learning?
What can AI do that traditional software cannot?
What is artificial intelligence used for today?
What are the key benefits of AI for businesses?
What challenges are associated with AI adoption in business?
What is artificial general intelligence (AGI), and how is it different from current AI?
What is the future of AI in business?
Want to learn how artificial intelligence can bring more transparency and trust to your operations?