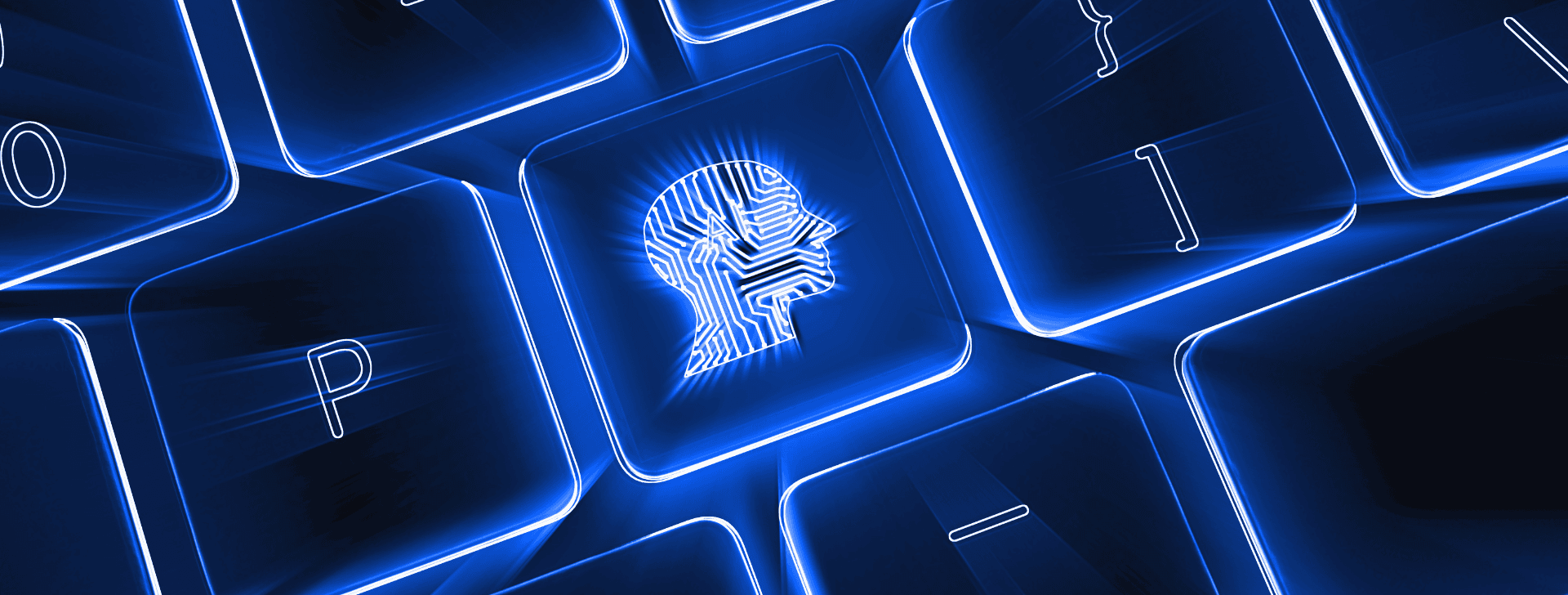
10 key differences between generative AI and predictive AI
OCT. 4, 2024
8 Min Read
Artificial intelligence (AI) has become a cornerstone of modern business strategy, driving innovations in everything from customer service to product design and risk management.
Among the various types of AI, generative AI and predictive AI stand out for their transformative potential, yet they serve different purposes. In this blog, we’ll explore the distinctions between these two forms of AI, their use cases, and how businesses can strategically adopt them to achieve significant operational benefits.
Key takeaways
- 1. Predictive AI is essential for forecasting: It analyzes historical data to predict future outcomes, offering insights that help businesses optimize decision-making and operational efficiency.
- 2. Generative AI enables creativity and content production: It is widely used in industries like marketing and entertainment, where the generation of new, unique content is crucial.
- 3. Different data requirements: Predictive AI needs labeled data to make accurate predictions, while generative AI works with unlabeled data, allowing it to create new outputs based on patterns within the data.
- 4. AI collaboration enhances business capabilities: Businesses can combine predictive AI for data-driven insights with generative AI to develop innovative solutions, enabling faster responses to market needs.
- 5. Explainability is a challenge for generative AI: While predictive AI models are more transparent, generative AI often operates as a black box, making its outputs more difficult to interpret in certain industries.
What is predictive AI?
Predictive AI refers to the use of machine learning algorithms and statistical models to analyze historical data and make informed predictions about future events or outcomes. It is typically applied in scenarios where forecasting and decision-making are critical, offering businesses insights that drive efficiency and mitigate risks.
Predictive AI uses techniques such as regression models, time series analysis, and classification methods. Industries like finance, healthcare, and logistics rely heavily on predictive AI to optimize processes, reduce costs, and improve forecasting accuracy. By analyzing large datasets, predictive AI uncovers hidden patterns and trends that businesses can use to make data-driven decisions.
In short, predictive AI is about anticipating the future based on the past. Its strength lies in its ability to model various scenarios and offer accurate predictions that inform everything from inventory management to fraud detection.
What is generative AI?
Generative AI, on the other hand, focuses on creating new content from existing data. Rather than analyzing and predicting outcomes, generative AI models are designed to produce novel outputs such as text, images, videos, and even music. These outputs are based on the patterns and relationships within the training data.
Generative AI uses deep learning models like Generative Adversarial Networks (GANs), transformers, and variational autoencoders (VAEs). These models are trained on large datasets, which allows them to generate new content that mimics the style or structure of the original data. For instance, a generative AI model trained on images of landscapes could generate entirely new, yet realistic, landscape images.
Generative AI is widely used in industries such as entertainment, marketing, and software development, where creative solutions are paramount. From AI-powered chatbots to automated content generation, the applications of generative AI are vast and growing.
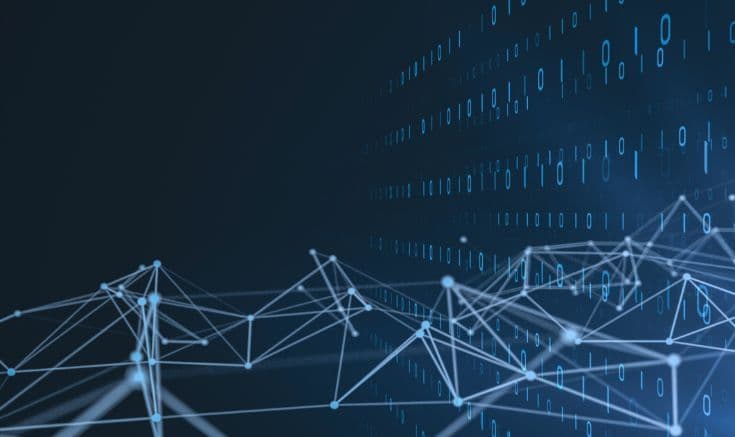
What’s the difference between generative AI and predictive AI?
Generative AI and predictive AI are two distinct technologies, each with a specific purpose and approach. Generative AI specializes in producing new data or content, including things like images, text, and even complete virtual environments. It is commonly used in fields like entertainment, design, and marketing, where originality and creativity are key. On the other hand, predictive AI is designed to analyze historical data to forecast future outcomes, helping businesses optimize processes, plan strategically, and manage risk. These differences in function and application make it important to understand how each type of AI can best serve different business objectives.
1. Primary function: creating vs. predicting
The core difference between generative AI and predictive AI lies in their primary function. Generative AI focuses on creating new data or content, while predictive AI uses historical data to forecast future outcomes. This fundamental distinction shapes how these AI technologies are applied across industries.
- Generative AI: Generative AI creates new content, whether that’s text, images, or even entire virtual worlds. It’s used in industries like entertainment, where creating new and unique experiences is key to engaging customers.
- Predictive AI: Predictive AI, on the other hand, is all about forecasting. By analyzing past data, predictive AI provides businesses with insights into future trends, helping them plan and optimize their strategies accordingly.
Generative AI focuses on originality and innovation, while predictive AI is more about reliability and informed decision-making. Both play vital roles in today’s data-driven world, but they fulfill different needs.
2. Data requirements: labeled vs. unlabeled data
Another critical distinction between generative and predictive AI lies in the type of data they require. The nature of the data directly influences how these AI models are trained and how they perform their respective tasks.
- Generative AI: Generative AI often utilizes unlabeled data and leverages unsupervised learning to generate outputs. For example, it can create images, text, or music without the need for explicitly labeled input data.
- Predictive AI: Predictive AI typically relies on labeled data to learn patterns and make accurate predictions. This form of AI needs clearly defined input and output relationships, such as historical sales data labeled with specific outcomes to predict future trends.
The reliance on labeled vs. unlabeled data underscores the contrasting approaches of generative and predictive AI in solving problems and delivering value.
3. AI models: adversarial networks vs. regression models
The underlying models that power generative AI and predictive AI differ significantly. Understanding the types of models involved helps businesses identify which technology is better suited to their particular challenges.
- Generative AI: Common models in generative AI include generative adversarial networks (GANs) and transformers. These models are designed to create new data that closely mimics real-world examples.
- Predictive AI: Predictive AI often relies on regression models, decision trees, and time series analysis to identify trends and forecast outcomes. These models use statistical techniques to predict future events based on historical data.
While generative AI models are built to produce novel content, predictive AI models focus on analyzing past patterns to make informed predictions about the future.
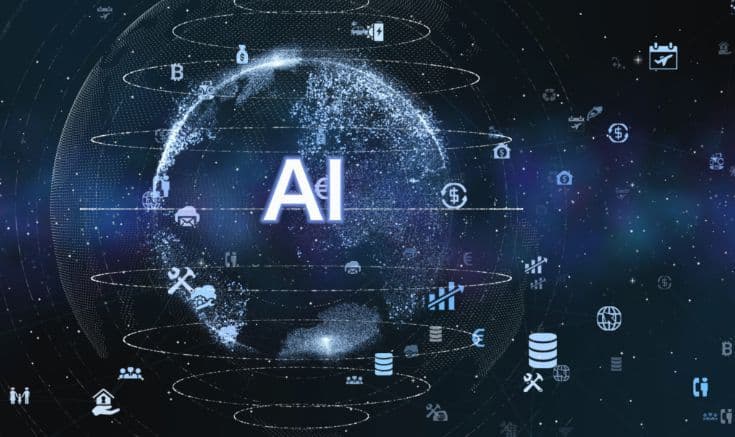
4. Use cases: creativity vs. optimization
The use cases for generative AI and predictive AI are as varied as their capabilities. Each form of AI is uniquely suited to addressing specific business needs, from creating content to optimizing operations.
- Generative AI: Generative AI is ideal for industries where creativity is paramount. It’s used in marketing for personalized content creation, in design for generating prototypes, and in entertainment for creating digital assets like art, music, and video.
- Predictive AI: Predictive AI is more focused on optimization. Businesses use predictive AI for demand forecasting, risk management, and operational planning. For instance, it helps supply chain managers predict inventory needs and financial institutions assess credit risks.
Whether a business needs innovation or efficiency, generative AI and predictive AI cater to very different objectives, allowing companies to select the right tool for the job.
5. Interactivity: static outputs vs. dynamic feedback loops
The way these two AI types interact with data and users also highlights a key difference. Generative AI and predictive AI offer contrasting experiences in terms of interactivity and real-time feedback.
- Generative AI: Generative AI typically produces static outputs. Once a model generates an image, a piece of text, or another form of content, that output remains fixed unless prompted for a new generation.
- Predictive AI: Predictive AI often works in dynamic feedback loops. It continuously learns from new data and updates its predictions over time. This is common in applications like fraud detection, where predictive models improve as more cases of fraud are identified.
The distinction between static and dynamic processes further defines the operational roles of generative AI and predictive AI, especially in interactive or evolving business environments.
6. Business impact: innovation vs. efficiency
AI technologies, specifically generative and predictive AI, offer businesses two distinct advantages: driving innovation and enhancing operational efficiency. The application of each depends on a company’s specific goals, whether it's pushing the boundaries of creativity or optimizing day-to-day operations.
Generative AI
Generative AI is instrumental in fostering innovation by creating new products, services, and even entire business models. It enables businesses to tap into fresh ideas that wouldn’t be possible through traditional methods. For example, generative AI can design prototypes for new products, develop personalized customer experiences, and even assist in architectural or industrial design processes. Industries like pharmaceuticals use generative AI to discover new drug compounds, while manufacturers may rely on it for design innovations. Its ability to create content, solutions, or products from minimal input makes it a catalyst for businesses seeking to stay ahead of competitors through differentiation and creativity.
Generative AI's impact on innovation is not limited to creativity; it also empowers businesses to solve complex problems by generating multiple solutions that humans may not have considered. This opens up new avenues for research, development, and disruptive technologies, placing companies at the forefront of their industries.
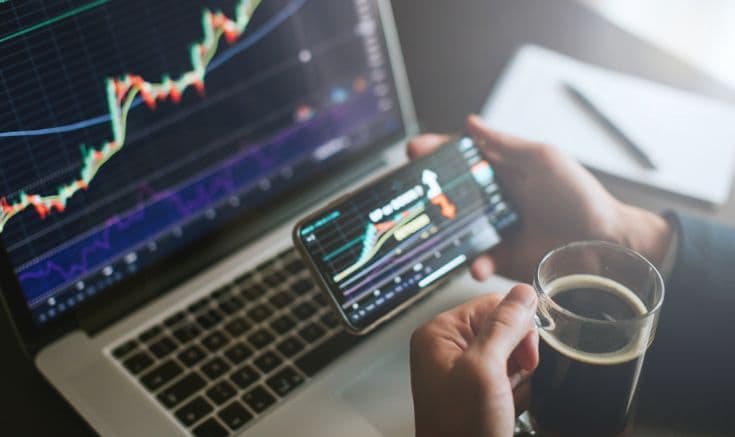
Predictive AI
In contrast, predictive AI drives operational efficiency by analyzing large datasets to forecast future trends and outcomes. This ability helps businesses make informed decisions, reduce risks, and allocate resources more effectively. For example, predictive AI is used in supply chain management to forecast demand, optimize inventory levels, and reduce waste. In finance, it assists in risk management by analyzing market trends to predict potential downturns or credit risks. Healthcare providers use predictive models to anticipate patient needs and allocate resources like staffing or equipment accordingly.
By providing real-time insights and future projections, predictive AI empowers businesses to optimize workflows, reduce costs, and respond to changes more quickly. Whether it's predicting market demand, identifying potential customer churn, or optimizing production schedules, predictive AI enables companies to operate more efficiently with data-driven precision.
Together, generative AI and predictive AI provide a powerful combination. Generative AI fuels forward-looking innovation, helping businesses break new ground, while predictive AI ensures that operations run smoothly, helping them make smarter, more informed decisions. Depending on the organization’s goals—whether focused on creative growth or process optimization—each technology offers a distinct path to achieving measurable business outcomes.
7. Natural language processing (NLP) applications
Natural language processing (NLP) is a critical area where both generative and predictive AI have distinct applications. The difference in how each AI type handles language-based tasks is significant.
- Generative AI: Generative AI excels in creating human-like text. It’s used in chatbots, content generation, and automated report writing. Large language models like GPT-3 demonstrate how AI can generate text that mimics human language.
- Predictive AI: Predictive AI in NLP is more about classifying and interpreting text. It’s used for sentiment analysis, language translation, and text classification, helping businesses understand and respond to customer feedback or analyze large volumes of text data.
Whether generating text or interpreting it, both generative and predictive AI play essential roles in advancing NLP applications.
8. Time horizon: long-term creation vs. short-term forecasting
The distinct timeframes in which generative AI and predictive AI operate offer businesses flexibility in addressing both immediate and long-term challenges.
- Generative AI: Generative AI is typically applied to long-term projects where the goal is to create something new that will have sustained value. For example, in product development, generative AI can be used to design innovative products or generate new creative content that will evolve over months or even years. This is especially valuable in industries such as architecture, where long-term planning and creation are essential, or in software development, where new systems or user interfaces are developed over extended periods. By focusing on long-term impact, generative AI provides businesses with a tool for strategic innovation, allowing them to stay ahead of market trends and maintain relevance over time.
- Predictive AI: Predictive AI, in contrast, operates on a shorter time horizon, often focusing on near-term forecasting and decision-making. It helps businesses respond to immediate needs by analyzing current data to predict short-term outcomes. For instance, retail businesses use predictive AI to forecast next quarter’s sales based on consumer trends, allowing them to adjust their inventory and marketing strategies accordingly. In staffing and resource management, predictive AI helps optimize short-term planning, ensuring that businesses are well-prepared to handle fluctuations in demand or market conditions.
The difference in time horizons makes each type of AI suitable for specific business needs. Generative AI is ideal for long-term innovation and creation, supporting strategic planning and sustained growth, while predictive AI is essential for short-term agility and decision-making, helping businesses adapt quickly to changing conditions. By leveraging both AI types, companies can effectively manage their immediate needs while planning for future opportunities.
9. Risk management: uncertainty vs. probability
Managing risk is crucial in any business decision, and artificial intelligence can play a significant role in this process. Generative AI and predictive AI offer different approaches to managing uncertainty and probability.
- Generative AI: Generative AI carries more uncertainty. Since it creates new outputs that may not always be predictable, businesses must carefully assess the risks and benefits of implementing generative AI solutions.
- Predictive AI: Predictive AI reduces uncertainty by using probability-based models. It helps businesses make informed decisions by forecasting potential outcomes based on historical data. Predictive AI is particularly useful in risk-heavy industries like finance and insurance.
While generative AI brings creative possibilities, predictive AI provides a more structured approach to risk management by leveraging data-driven insights.
10. Collaboration potential: enhancing creativity vs. augmenting expertise
Finally, the collaborative potential of generative and predictive AI highlights another key difference. Both AI types can work alongside humans but in very different ways.
- Generative AI: Generative AI enhances human creativity by providing tools that allow for new and innovative ideas. It’s frequently used by artists, designers, and marketers to push the limits of what’s possible.
- Predictive AI: Predictive AI augments human expertise by providing data-driven insights that enhance decision-making. Professionals in fields like healthcare and finance use predictive AI to refine their strategies and improve outcomes.
Both generative and predictive AI empower users, but generative AI focuses on enhancing creativity, while predictive AI supports data-backed expertise.
"Generative AI creates new content, whether that’s text, images, or even entire virtual worlds."
How generative AI and predictive AI complement each other
Generative AI and predictive AI are not mutually exclusive. In fact, they often complement each other in driving business transformation. Predictive AI can analyze patterns in existing data to forecast needs, while generative AI can then create new content or solutions based on those forecasts.
For example, a company may use predictive AI to forecast customer demand and generative AI to create personalized marketing content tailored to those predictions. By combining the strengths of both AI types, businesses can innovate faster and with greater precision.
Examples of generative and predictive AI working together
The integration of generative and predictive AI presents a powerful opportunity for businesses to unlock new efficiencies and innovations. By combining the strengths of both technologies, organizations can achieve results that neither could deliver on its own. Here are some expanded examples of how businesses are using generative and predictive AI in tandem to drive superior outcomes:
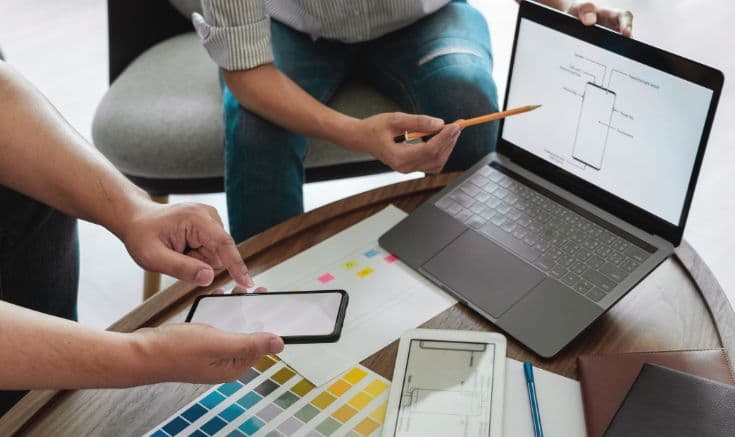
- Product design: In product development, predictive AI analyzes historical sales data, market trends, and customer feedback to forecast future preferences. For example, a fashion brand might use predictive AI to determine emerging styles or color palettes based on past purchase patterns. Once these insights are identified, generative AI can step in to design new product prototypes that align with these predicted trends. This allows companies to create products that not only meet current market demand but are also likely to resonate with future customer desires, shortening the product development cycle and reducing the risk of failure.
- Content marketing: In the realm of content marketing, predictive AI can analyze data from previous campaigns, identifying which types of content (e.g., blogs, videos, social media posts) perform best with specific target audiences. It can even predict optimal times for publishing content to maximize engagement. Generative AI then takes this insight and produces content tailored to those preferences, whether it’s generating personalized email campaigns, blog articles, or social media content. By combining predictive insights with generative capabilities, businesses can create high-performing content at scale, improving customer engagement and marketing ROI.
- Supply chain optimization: Predictive AI is widely used in supply chain management to forecast demand for specific products based on factors such as seasonal trends, market fluctuations, and historical sales data. Once demand forecasts are established, generative AI can simulate different logistics and distribution strategies. It can generate optimized models for inventory management, shipping routes, and warehouse layouts, offering businesses a range of scenarios to improve efficiency, reduce costs, and enhance service delivery. This combination helps businesses not only prepare for demand fluctuations but also execute logistics strategies that are both innovative and cost-effective.
- Customer service: Many companies rely on predictive AI to analyze historical customer interactions and identify the most common queries or issues customers face. These insights enable businesses to predict which customer concerns are most likely to arise. Generative AI can then be used to power chatbots or virtual assistants that provide real-time, personalized responses to those inquiries. The generative models enable the creation of highly interactive, human-like responses, enhancing the customer experience. By blending predictive AI’s ability to foresee customer needs with generative AI’s capability to deliver personalized responses at scale, businesses can offer more efficient, responsive, and proactive customer service.
By integrating both generative AI and predictive AI, businesses can create systems that are more agile, responsive, and tailored to market conditions. Predictive AI offers data-driven insights that help businesses anticipate needs, while generative AI acts on those insights to create new products, services, or content in real time. This synergy allows businesses to not only react to changes in their environment but also actively shape the future, giving them a significant competitive advantage in an increasingly dynamic market.
"Predictive AI enhances efficiency by streamlining decision-making processes, helping companies optimize supply chains, manage resources, and reduce risks."
AI explainability and interpretability
One of the challenges of AI, especially generative AI, is the lack of explainability. Generative AI models, such as generative adversarial networks (GANs), often function as "black boxes," producing outputs without clearly revealing the decision-making process behind them. This lack of transparency can be a challenge for businesses that require traceability and accountability.
Predictive AI, on the other hand, offers greater explainability. Since it relies on statistical models and probability, its outputs are more transparent and easier to interpret. Businesses can understand how and why a specific prediction was made, making predictive AI more suitable for risk-sensitive applications like finance and healthcare.
However, even predictive AI is not entirely free of challenges. Human interpretation of its outputs can introduce errors, making it essential for organizations to pair AI-driven insights with expert analysis.
AI business considerations and adoption strategies
When deciding between generative AI and predictive AI, businesses need to consider their specific objectives. Both technologies offer distinct benefits, but choosing the right one—or strategically combining them—can yield the best results.
1. Define your business goals: Are you looking to drive innovation with new products, or do you need to optimize existing processes? Your goals will determine whether generative or predictive AI is more appropriate.
2. Evaluate data availability: Predictive AI requires high-quality historical data, whereas generative AI can often work with unlabeled or less structured datasets. Ensure your data aligns with your chosen AI model.
3. Consider ethical implications: Both types of AI raise ethical concerns, from bias in predictive models to the misuse of generative content. It’s important to have governance structures in place to mitigate these risks.
4. Start small, then scale: Begin by implementing AI in a pilot project to evaluate its effectiveness. Once proven, scale up your AI initiatives to cover more areas of your business.
By thoughtfully integrating generative and predictive AI, businesses can position themselves for long-term success, driving both innovation and operational efficiency.
Understanding and utilizing both generative AI and predictive AI enables businesses to tap into the full potential of artificial intelligence, applying it to innovate and optimize across multiple facets of their operations. At Lumenalta, we specialize in helping companies deploy AI solutions tailored to their specific needs.
Table of contents
- What is predictive AI?
- What is generative AI?
- What’s the difference between generative AI and predictive AI?
- How generative AI and predictive AI complement each other
- AI explainability and interpretability
- AI business considerations and adoption strategies
- Common questions about generative AI and predictive AI
Common questions about generative ai vs predictive ai
How do businesses benefit from generative AI vs. predictive AI?
What industries use generative AI and predictive AI most frequently?
What types of data are needed for generative AI and predictive AI?
What are the challenges of explainability in generative AI?
How can businesses combine generative AI and predictive AI for better outcomes?
Want to learn how artificial intelligence can bring more transparency and trust to your operations?