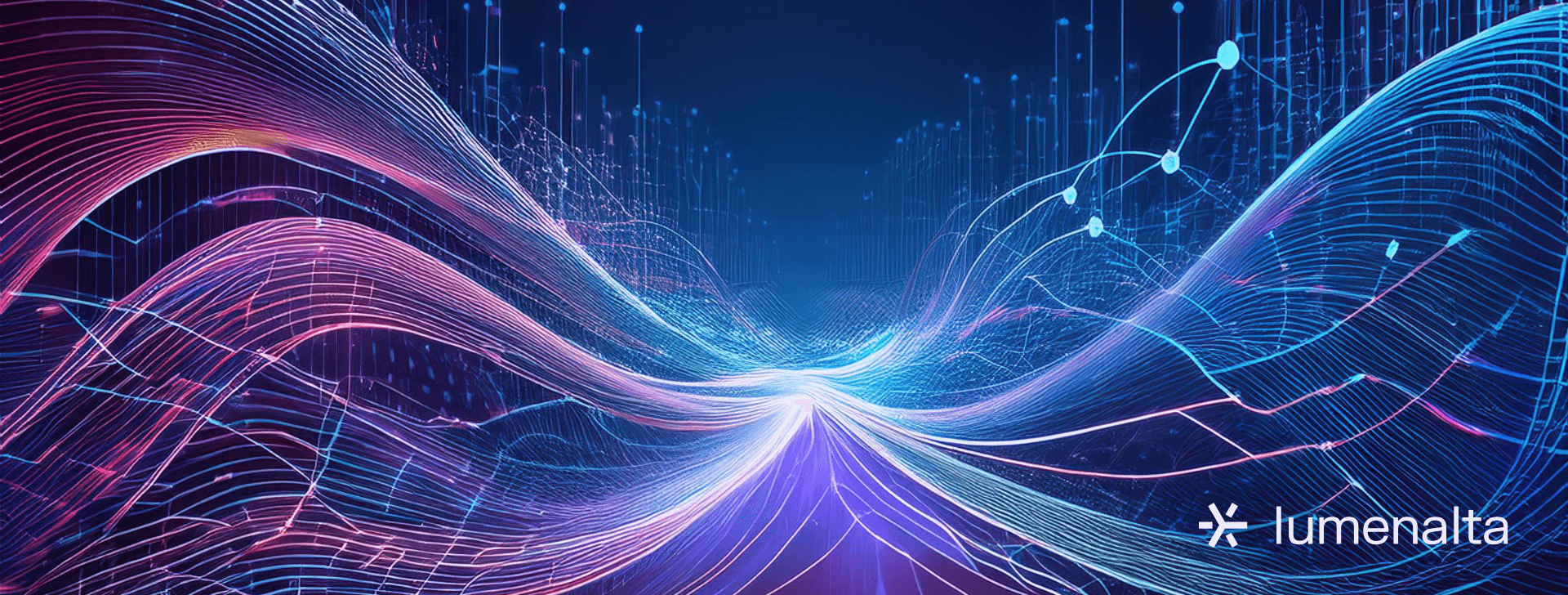
A CEO’s guide to generative AI
OCT. 19, 2024
8 Min Read
Generative AI is reshaping industries by enabling businesses to create content, streamline operations, and drive personalized customer experiences at scale.
CEOs must understand the capabilities and challenges of this technology to harness its value effectively. From automating workflows to creating entirely new business models, generative AI provides significant opportunities. However, effective integration requires thoughtful strategies, responsible governance, and a long-term vision. This guide offers CEOs actionable insights on how generative AI is transforming business operations and what they need to know to stay ahead.
Key takeaways
- 1. Strategic business impact: Generative AI unlocks new opportunities by automating workflows, delivering hyper-personalized customer experiences, and accelerating innovation. CEOs can leverage AI as a key differentiator in a competitive market.
- 2. Industry-wide applicability: Generative AI is transforming retail, healthcare, financial services, and manufacturing by improving efficiency, reducing time-to-market, and enhancing customer satisfaction.
- 3. Integrated AI strategy: Successful AI adoption requires alignment with business objectives, starting with pilot projects, and scaling efficiently across the organization based on early insights.
- 4. Governance and ethics: CEOs must implement robust governance frameworks focusing on data privacy, transparency, and fairness to mitigate risks and maintain stakeholder trust.
- 5. Future-ready infrastructure: To maximize AI's long-term value, organizations should adopt scalable cloud-based platforms, invest in continuous learning, and monitor AI performance with iterative feedback loops.
What is generative AI and why it matters for CEOs
Generative AI refers to artificial intelligence systems capable of creating original content such as text, images, videos, audio, and even code. This is achieved through the model’s ability to learn patterns from vast datasets, generating outputs that mirror human creativity and logical constructs. Unlike traditional AI, which focuses on automation and pattern recognition, generative AI introduces creativity and flexibility. By moving beyond predefined rules, it empowers businesses to deliver personalized experiences at scale, engage customers in new ways, and discover novel solutions across operations.
For CEOs, generative AI is more than just a new technology—it is a strategic tool that reshapes business models. AI-generated solutions streamline operations, create engaging marketing campaigns, and develop new products faster, offering businesses a critical edge in highly competitive markets. Generative AI enables organizations to evolve from reactive operations to proactive, insight-driven business models. CEOs who understand this technology’s capabilities can position their companies to achieve exponential growth, enhance efficiency, and drive sustainable innovation.
Key aspects of generative AI relevant to CEOs:
- Content creation and automation: Generative AI systems can autonomously create assets like product descriptions, blog posts, or marketing visuals, allowing businesses to reduce time-to-market for campaigns.
- Cost savings and productivity: By automating labor-intensive processes, generative AI lowers operational costs while ensuring high-quality output across functions.
- New revenue streams: AI models can introduce innovative products or services, such as virtual assistants or AI-generated products, creating new revenue opportunities.
- Personalization at scale: AI solutions provide hyper-personalized customer interactions in real time, enhancing customer engagement and loyalty.
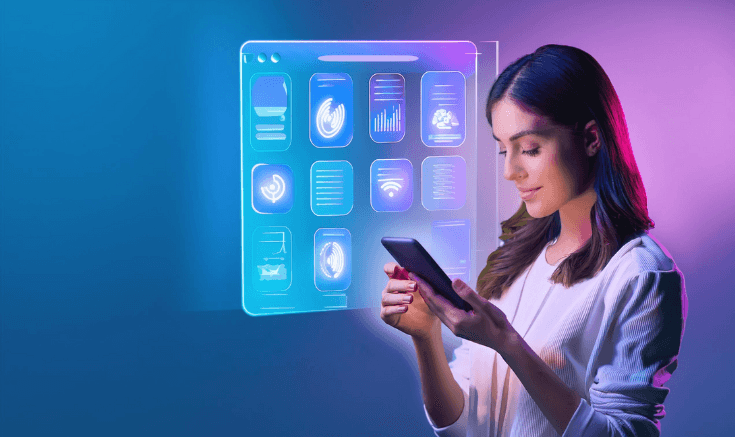
- Continuous learning and adaptation: AI models improve over time, adapting to changing customer behavior or operational needs, making them valuable long-term assets.
For CEOs, adopting generative AI isn't optional—it’s imperative to remain competitive. Early adoption not only enhances current operations but also ensures organizations are ready for emerging trends that will shape the future business landscape.
Types of generative AI
Generative AI encompasses several models and techniques, each suited to unique applications across industries. Below is a list of prominent types, showcasing their functionality and business relevance:
- ChatGPT (Text generation)
- Utilizes generative pre-trained transformer (GPT) models to generate coherent responses for applications such as virtual assistants and automated customer support.
- DALL·E (Image generation)
- Transforms textual descriptions into creative visual content, enhancing marketing, design, and product prototyping processes.
- Codex (Code ceneration)
- Automates programming tasks, speeding up software development and supporting learning environments through code suggestions.
- GANs (Generative adversarial networks)
- Creates realistic images, audio, or video content by pitting two networks against each other—useful in gaming, deepfakes, and product visualization.
- MusicLM (Music composition)
- Generates music based on user input, accelerating the production of custom soundtracks and personalized audio experiences.
Each model offers distinct advantages, driving innovation and operational efficiency across sectors like healthcare, retail, and entertainment. By understanding these generative AI types, businesses can leverage them strategically for improved productivity and market differentiation.
“Generative AI enables organizations to evolve from reactive operations to proactive, insight-driven business models.”
How generative AI unlocks value across industries
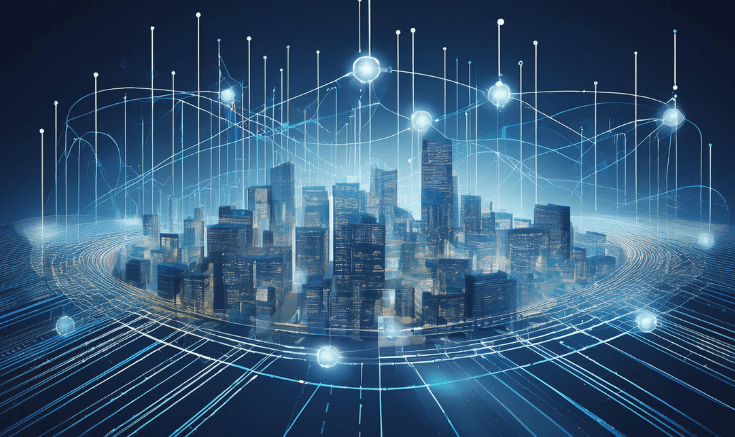
Retail and e-commerce
In retail, AI-generated product descriptions, recommendations, and promotional content improve customer engagement and drive conversions. Retailers use AI to predict trends, tailor campaigns for specific customer segments, and generate personalized shopping experiences. Dynamic content, such as product recommendations based on browsing history, enhances conversion rates by providing hyper-relevant suggestions in real time.
Example: AI-powered platforms can automatically generate tailored email campaigns and product pages that reflect each customer’s preferences, increasing revenue while reducing the need for human input.
Financial services
Generative AI in finance supports chatbots and virtual financial advisors that deliver personalized investment strategies and manage customer inquiries. AI systems analyze customer portfolios and recommend investments aligned with risk tolerance, enabling better financial planning. Additionally, AI models ensure regulatory compliance by automating document generation and risk assessments, saving significant time and resources.
Example: AI tools generate personalized savings plans and alert customers to suspicious transactions, helping banks improve customer trust while streamlining operations.
Healthcare
In healthcare, AI-powered tools accelerate drug discovery by analyzing clinical trial data and medical literature, significantly shortening development timelines. AI also enables personalized treatment plans by evaluating patient data, offering precision medicine tailored to individual needs. Moreover, AI chatbots assist with routine tasks like patient check-ins, appointment scheduling, and answering FAQs, reducing the workload on healthcare staff.
Example: Generative AI models simulate molecular compositions, helping researchers discover new drugs faster and at lower costs. Virtual assistants, meanwhile, ensure patient engagement through timely reminders and personalized care plans.
Media and entertainment
AI transforms the media and entertainment industry by automating content generation. This includes creating articles, social media posts, scripts, and even music compositions. AI helps brands produce engaging content faster while maintaining a consistent tone and style. In addition to speeding up production timelines, generative AI tools enable real-time audience engagement through chat-based interact mmmmmmmmmmmmmmmmmmmmmmmions and interactive media experiences.
Example: Streaming services use AI to generate personalized recommendations and playlists, enhancing user satisfaction and boosting retention rates.
Manufacturing
Generative AI optimizes manufacturing by streamlining product design and prototyping. AI algorithms generate innovative product blueprints and test multiple design variations before production, reducing the time and cost associated with traditional prototyping. AI also plays a key role in predictive maintenance by analyzing machine data and forecasting potential failures to prevent downtime.
Example: AI-generated designs allow companies to create lightweight yet durable components for industries like automotive or aerospace, accelerating the development of cutting-edge products.
Generative AI offers immense value across industries by reducing time-to-market, enhancing personalization, and driving operational efficiency. Whether creating innovative products, transforming customer engagement, or streamlining internal operations, AI is a powerful tool for leaders to unlock new business value and maintain a competitive advantage.
Generative AI use cases every CEO should know
Generative AI enables businesses to unlock new capabilities across multiple operational areas, improving efficiency and fostering innovation. Below is a detailed exploration of some of the most impactful use cases CEOs should be aware of.
Automated content creation
Generative AI accelerates content production by automatically generating high-quality text, images, and videos, significantly reducing the time and resources required. From drafting marketing emails, ad copy, and social media posts to writing news articles, AI-powered tools streamline workflows.
AI-based platforms can also maintain brand consistency by adhering to tone, style, and formatting rules set by the company. This allows businesses to scale content efforts efficiently during promotional campaigns and meet growing audience demands without expanding the workforce.
Examples of automated content creation:
- E-commerce: AI generates product descriptions tailored to each audience segment, enhancing relevance and improving conversion rates.
- Media: News outlets use AI to draft articles on routine topics like financial reports or sports events, freeing human reporters for in-depth journalism.
Product design and prototyping
Generative AI enhances product development by creating multiple design iterations rapidly, enabling teams to test and refine ideas more efficiently. These AI systems analyze historical product data and market trends to propose innovative designs that align with user preferences.
Using AI-generated models, companies can identify the most efficient, cost-effective, and aesthetically appealing prototypes before physical production begins. AI tools also simulate product performance, helping manufacturers reduce errors and ensure quality.
Examples of AI in product design:
- Automotive industry: AI tools generate lightweight vehicle components to improve fuel efficiency.
- Fashion and apparel: AI assists in developing sustainable clothing designs by evaluating material options.
Customer service automation
Customer service departments benefit from AI-powered chatbots and virtual assistants that provide round-the-clock support. These systems handle high volumes of customer inquiries, answering routine questions related to order tracking, billing, and account management.
More advanced AI solutions manage contextual conversations, resolving issues seamlessly or escalating more complex cases to human agents when necessary. By handling inquiries automatically, businesses can reduce response times and increase customer satisfaction while lowering operational costs.
Key benefits:
- Scalability: AI systems handle thousands of interactions simultaneously without delays.
- Omnichannel support: Chatbots operate across websites, messaging platforms, and mobile apps, ensuring a consistent customer experience.
Predictive analytics and forecasting
Generative AI enhances decision-making by analyzing large datasets to generate insights and forecasts for demand, revenue, and market trends. Businesses use predictive models to manage inventory, anticipate fluctuations in demand, and optimize pricing strategies.
In finance and retail, predictive AI tools help manage cash flow, assess risks, and develop data-backed strategies for investment or growth. CEOs benefit from these insights by making proactive, well-informed decisions that align with future market conditions.
Applications of predictive analytics:
- Supply chain management: AI forecasts disruptions and suggests alternate sourcing strategies.
- Retail: AI models predict seasonal sales patterns, ensuring optimized inventory levels.
Software development assistance
Generative AI tools improve software development processes by automatically generating code snippets and suggesting optimizations. AI-based platforms like GitHub Copilot assist developers by offering contextual code completions, reducing the time spent on repetitive coding tasks.
AI also identifies errors early in the development cycle by analyzing code for vulnerabilities, ensuring that products are built faster and with fewer bugs. Teams using AI-assisted development tools experience shorter release cycles and improved software quality, giving businesses a competitive edge.
Examples of AI in software development:
- Startups: AI tools help small teams develop MVPs (minimum viable products) faster by automating basic coding tasks.
- Enterprise IT: AI-driven platforms assist in automating software maintenance and updates, reducing downtime.
Generative AI offers business leaders tools to streamline processes, improve decision-making, and innovate faster. Whether through automating content creation, refining product designs, enhancing customer service, or supporting software development, AI-powered solutions empower businesses to operate efficiently while meeting market demands.
Benefits of generative AI for business leaders
Generative AI offers multiple strategic advantages, enabling business leaders to improve efficiency, foster innovation, and create sustainable growth opportunities. Below are key benefits and deeper insights into how AI empowers businesses.
1. Scalability
Generative AI allows businesses to scale operations without proportionally increasing headcount or resources. By automating repetitive tasks—such as content creation, customer service, and inventory management—AI ensures organizations can manage growing demands with fewer bottlenecks.
For example, AI-generated product descriptions and chatbots enable e-commerce businesses to serve expanding customer bases without adding additional support staff. This scalability also applies to software development and operations, where AI tools automate testing and deployment processes, accelerating the development lifecycle. The ability to scale with minimal additional cost positions businesses for sustainable growth while maintaining profitability.
2. Personalization
AI-driven systems collect and analyze customer data to create hyper-personalized experiences. From targeted marketing campaigns to tailored product recommendations, generative AI enhances customer satisfaction and drives higher conversion rates. Personalized customer interactions also improve brand loyalty, as consumers are more likely to engage with brands that understand their preferences and needs.
For example, in financial services, AI tools analyze spending behavior to recommend personalized credit card offers or savings plans. Retailers can deliver dynamic product recommendations based on past purchases, creating seamless customer journeys across touchpoints. This level of personalization helps businesses build long-term relationships with customers, fostering retention and advocacy.
3. Innovation
Generative AI reduces development timelines, accelerating experimentation and innovation across business units. AI-powered design tools can generate product prototypes and optimize blueprints faster, enabling companies to bring innovative products to market quickly. This is particularly valuable in industries such as manufacturing, where faster iterations can provide a significant competitive edge.
AI also democratizes innovation by lowering barriers to creativity. Even non-experts can use AI tools to generate ideas, code, or marketing content, encouraging cross-functional collaboration. By fostering an innovation-friendly environment, businesses stay ahead of market trends and capitalize on emerging opportunities more effectively.
4. Cost savings
AI-driven automation helps businesses reduce operational costs by minimizing manual labor and improving efficiency. Tasks like data entry, content generation, and administrative support are streamlined, allowing companies to reallocate resources toward higher-value activities. Additionally, AI-powered customer service tools reduce call center workloads, leading to savings in staffing and training expenses.
AI also optimizes supply chain operations, predicting demand fluctuations and recommending inventory levels, which reduces waste and prevents stockouts. These cost savings directly improve profit margins, giving businesses a financial cushion to reinvest in growth and innovation.
“AI-driven systems collect and analyze customer data to create hyper-personalized experiences, enhancing engagement and driving loyalty.”
Challenges in adopting generative AI for business
While generative AI offers immense potential, businesses face several challenges during implementation. Addressing these challenges proactively ensures smooth integration and sustainable AI use.
1. Data privacy and compliance
Generative AI systems rely on vast datasets, often containing personal or sensitive information. Businesses must navigate data privacy regulations, such as GDPR in Europe and CCPA in California, which govern how customer data is collected, stored, and processed. Non-compliance can lead to significant financial penalties and reputational damage.
Implementing encrypted communication and anonymization protocols helps ensure compliance. Companies must also educate employees on responsible data handling practices and establish transparency around how AI models use data to build customer trust.
2. Bias and fairness
Generative AI models can unintentionally introduce biases into decision-making processes if the data used to train them is not representative or contains prejudices. Biased outputs can negatively impact brand reputation, especially in industries like finance and healthcare, where fairness is critical.
To mitigate bias, companies must audit datasets regularly, ensuring diversity and accuracy. Implementing fairness guidelines and monitoring AI outputs for unintended consequences helps businesses maintain trust with customers and stakeholders.
3. Integration complexities
Many companies rely on legacy systems that are not natively compatible with AI technologies, creating integration challenges. Aligning AI tools with existing infrastructure often requires significant technical resources and collaboration between IT and business teams.
Partnering with AI vendors that offer flexible APIs and cloud-based solutions can simplify the integration process. Additionally, adopting modular AI platforms allows businesses to phase in new capabilities gradually, reducing disruption during implementation.
4. Talent shortages
The rapid adoption of generative AI has led to a high demand for skilled professionals, such as data scientists, AI engineers, and machine learning experts. Businesses face challenges in attracting and retaining talent in a competitive labor market.
To address talent shortages, companies should invest in upskilling existing employees and offer training programs to build internal AI expertise. Partnering with universities and research institutions can also create a pipeline of future talent. Furthermore, adopting no-code or low-code AI platforms democratizes access to AI capabilities, allowing non-technical teams to contribute to AI initiatives effectively.
Successfully navigating these challenges positions businesses to fully leverage generative AI’s potential while maintaining ethical practices and operational efficiency. CEOs who proactively address compliance, bias, and talent gaps will be better equipped to lead their organizations through AI-driven transformation.
Navigating the generative AI value chain
To extract the maximum value from generative AI, businesses must develop a comprehensive strategy that spans the entire AI value chain. Each stage—data collection, model development, deployment, and performance monitoring—requires careful planning to align AI initiatives with organizational goals. Below is an in-depth exploration of these key stages and how businesses can successfully navigate the generative AI value chain.
Data collection: Gathering high-quality datasets to train AI models
The quality of an AI model is directly tied to the quality of the data it is trained on. High-quality, diverse, and relevant datasets are essential to ensure that AI systems can generate accurate and meaningful outputs. Generative AI often relies on structured and unstructured data, including text, images, audio, and video. This stage may involve collecting internal data from customer interactions, transaction records, or operational systems, as well as external data sources like public datasets and industry reports.
Key considerations for data collection:
- Data diversity: Ensure datasets reflect various customer demographics and use cases to minimize bias.
- Compliance: Collect and store data in accordance with privacy laws such as GDPR and CCPA to avoid legal risks.
- Data enrichment: Use techniques such as data augmentation and synthetic data generation to enhance datasets, particularly when real-world data is limited.
Model development: Partnering with AI vendors or building proprietary models
Once the data is prepared, businesses can either partner with AI vendors or develop proprietary AI models tailored to their specific needs. Off-the-shelf AI solutions from vendors can accelerate time-to-market, but custom models may offer better alignment with unique business requirements. Choosing between these options depends on the organization’s AI maturity, budget, and internal technical capabilities.
- Vendor partnerships: Pre-trained AI models from vendors offer speed and scalability but may require customization.
- Proprietary model development: Building models in-house ensures greater control and customization but demands investments in talent, infrastructure, and expertise.
- Open-source tools: Companies can also leverage open-source platforms like TensorFlow or PyTorch, enabling faster experimentation and reduced development costs.
Collaboration between data scientists, IT teams, and business units is critical during this stage to ensure the models align with both technical and operational goals.
Deployment: Integrating AI systems into existing workflows
Deploying generative AI solutions successfully requires seamless integration into existing business workflows and systems. Whether it’s integrating AI into CRM platforms, e-commerce websites, or production lines, organizations must ensure that AI tools enhance efficiency without disrupting operations.
Best practices for deployment:
- Modular deployment: Begin with pilot programs in specific areas (e.g., customer service or content creation) before scaling organization-wide.
- Cloud-based solutions: Deploy AI on cloud platforms to leverage scalability and flexibility, minimizing infrastructure investments.
- Workflow automation: Integrate AI outputs directly into business processes, such as routing AI-generated insights to decision-makers or automating customer interactions.
- Change management: Provide training to employees and communicate the benefits of AI adoption to reduce resistance and promote collaboration.
Performance monitoring: Continuously evaluating AI outputs to ensure relevance and accuracy
AI systems must be monitored continuously to ensure they remain relevant and perform accurately over time. This involves tracking both quantitative metrics—such as accuracy, efficiency, and uptime—and qualitative feedback from end-users. Performance monitoring also includes regular audits for biases and errors to maintain trust and alignment with business objectives.
Key aspects of AI performance monitoring:
- Feedback loops: Use feedback from customers and employees to improve AI outputs over time.
- Bias detection and correction: Regular audits help identify and mitigate biases introduced during model development or data collection.
- Model retraining: AI models require retraining periodically using fresh data to adapt to evolving trends and operational needs.
- KPIs and dashboards: Set key performance indicators (KPIs) to measure success and monitor AI activity through real-time dashboards.
Successfully navigating the generative AI value chain requires strategic alignment, cross-functional collaboration, and ongoing optimization. By focusing on high-quality data, effective model development, seamless deployment, and rigorous performance monitoring, organizations can unlock the full potential of generative AI to drive innovation, efficiency, and long-term growth.
Building a generative AI-ready organization
Building a generative AI-ready organization demands more than just technology implementation; it involves a cultural shift, structured collaboration, and continuous learning. CEOs must align business units, IT teams, and data scientists around shared objectives, fostering a culture of innovation that embraces AI's potential. Key to this process is empowering employees through AI upskilling and ensuring seamless communication across functions to maintain momentum and alignment.
Key areas | Action items | Benefits |
---|---|---|
Cultural shift | Foster collaboration between IT, business, and data teams. | Aligns stakeholders on shared goals. |
Upskilling workforce | Provide AI training and tools for employees at all levels. | Increases engagement and AI literacy. |
Infrastructure readiness | Implement scalable cloud-based platforms. | Enhances adaptability to technology changes. |
Data governance | Establish policies for data quality and compliance. | Reduces risks and ensures trust in AI outputs. |
Change management | Monitor AI performance with iterative feedback loops. | Facilitates continuous improvement. |
The success of AI integration hinges on a dynamic interplay of people, technology, and processes. CEOs play a pivotal role in leading this transformation by cultivating a learning environment, ensuring data integrity, and driving strategic alignment. With the right foundation in place, organizations can harness AI to unlock efficiency, innovation, and sustainable business growth.
Implementing generative AI
1. Start small, scale fast
One of the most effective ways to adopt generative AI is by starting with pilot projects. Pilots help businesses test AI capabilities on a smaller scale, identify bottlenecks, and fine-tune workflows before scaling across the organization. These projects provide valuable insights into what works and what doesn’t, minimizing risks during broader deployment.
- Identify low-risk use cases: Choose areas like automated content creation or customer support for initial pilots, as these offer quick wins and measurable outcomes.
- Collect feedback early: Use pilot results to assess performance and identify areas for improvement before expanding to other departments.
- Plan for scalability: Once pilots prove successful, build infrastructure that allows for seamless scaling across different business functions.
2. Align AI with business goals
To maximize the impact of AI initiatives, it is critical that they align with the organization’s broader business strategy. Generative AI should enhance core business objectives—whether that’s increasing efficiency, improving customer experience, or driving revenue growth. CEOs need to collaborate closely with business units to identify high-impact areas where AI can add value.
- Integrate AI into strategic planning: AI initiatives should complement and enhance existing business goals, not operate as standalone projects.
- Define KPIs: Establish clear metrics for success—such as productivity gains or improved customer satisfaction—and track them to measure AI's contribution to business outcomes.
3. Invest in partnerships
Building robust AI systems often requires specialized knowledge and technology that may not exist in-house. Collaborating with AI vendors, technology partners, and consultants helps businesses leverage external expertise while reducing time-to-market.
- Technology partnerships: Engage with cloud providers and AI platform vendors to access cutting-edge tools and infrastructure.
- Industry experts: Consultants can provide strategic guidance on best practices, compliance requirements, and integration challenges.
- Cross-industry collaborations: Partnering with research institutions or industry peers can help businesses stay ahead of evolving AI trends.
4. Foster a learning environment
Given the rapid pace of AI innovation, organizations must foster a culture of continuous learning to keep up with new trends and developments. CEOs can create an environment that encourages employees to explore new tools and techniques, fostering innovation across the organization.
- Internal training programs: Offer AI literacy courses to upskill employees and ensure they are comfortable working with AI tools.
- Collaborative innovation labs: Set up cross-functional teams focused on experimentation and rapid prototyping with AI.
Knowledge sharing: Encourage participation in AI forums and industry conferences to stay updated on the latest trends and insights.
“Monitoring AI performance is equally important to maintain operational fairness… continuous tracking helps organizations adapt AI outputs to evolving standards and maintain effectiveness over time.”
Governance and responsible AI: What every CEO should prioritize
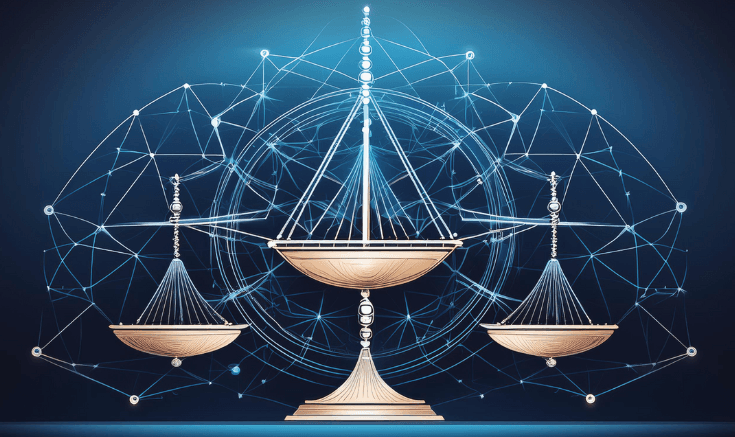
Governance frameworks play a vital role in ensuring that AI systems operate ethically, transparently, and in compliance with regulations. CEOs are responsible for setting clear policies that govern how data is used, managed, and protected within their organizations. These frameworks not only help maintain trust with stakeholders but also safeguard against potential misuse of AI technologies. A well-developed governance structure ensures that AI aligns with the company's mission and values while minimizing risks.
Creating an AI ethics framework is essential for embedding principles of fairness, accountability, and transparency into the system’s design and application. This framework guides decisions on how AI should be developed and deployed, ensuring that automated systems reflect the organization’s ethical commitments. In parallel, robust data governance policies are necessary to ensure compliance with privacy laws, such as GDPR and CCPA. These policies establish guidelines for data collection, storage, and usage, helping organizations detect and address any privacy violations through regular audits and evaluations.
Monitoring AI performance is equally important to maintain operational fairness. CEOs need to implement mechanisms that identify and mitigate bias, ensuring that AI models do not produce discriminatory outcomes. Continuous tracking of performance helps organizations adapt AI outputs to evolving standards and maintain effectiveness over time. Regular performance reviews and bias audits foster public trust, ensuring that AI initiatives remain aligned with the company’s strategic and ethical goals.
Ethical considerations and responsible AI practices
Ethical AI adoption is essential to building trust with customers and stakeholders. Responsible AI practices go beyond compliance by ensuring that AI systems align with broader social and ethical standards.
- Transparency and communication: Companies should clearly communicate how AI systems work and provide users with the ability to control their data.
- Bias mitigation: Regular audits and testing ensure AI systems remain unbiased and do not reinforce harmful stereotypes or unintended outcomes.
- Data protection: Organizations must adopt robust data governance policies, including encryption and anonymization, to safeguard personal information.
- Long-term accountability: CEOs should establish internal governance boards to oversee AI initiatives and ensure systems remain aligned with both regulatory requirements and company values.
By prioritizing governance and responsible AI practices, CEOs can ensure that AI adoption drives sustainable innovation while maintaining public trust and mitigating risks.
Future trends in generative AI for business leaders
Emerging trends in generative AI include real-time personalization, where AI systems tailor interactions as they occur. Additionally, advancements in multimodal AI—capable of processing both text and images—are expanding the scope of AI applications. Voice-driven AI is also gaining momentum, enabling more natural interactions between businesses and customers.
Generative AI holds immense potential to reshape business operations and create new opportunities for growth. CEOs who understand the strategic value of AI and adopt a responsible, well-planned approach will position their organizations for long-term success in the evolving business landscape.
Lumenalta’s generative AI solutions empower organizations to unlock new levels of efficiency, innovation, and personalized customer engagement. Through our collaborative approach, we help businesses build AI-ready infrastructure, integrate scalable AI solutions, and drive sustainable growth. With expertise across multiple industries, we guide CEOs in aligning AI with strategic goals, ensuring governance, and navigating the evolving landscape of AI opportunities.
table-of-contents
- What is generative AI and why it matters for CEOs
- How generative AI unlocks value across industries
- Generative AI use cases every CEO should know
- Benefits of generative AI for business leaders
- Challenges in adopting generative AI for business
- Navigating the generative AI value chain
- Building a generative AI-ready organization
- Implementing generative AI
- Governance and responsible AI: What every CEO should prioritize
- Future trends in generative AI for business leaders
- Common questions about guide to generative AI
Common questions about guide to generative AI
1. What is generative AI, and how does it differ from traditional AI?
How can CEOs align generative AI initiatives with business goals?
What industries benefit the most from generative AI?
What are the primary challenges in adopting generative AI?
How does generative AI ensure scalability and long-term value for businesses?
Want to learn how artificial intelligence can bring more transparency and trust to your operations?