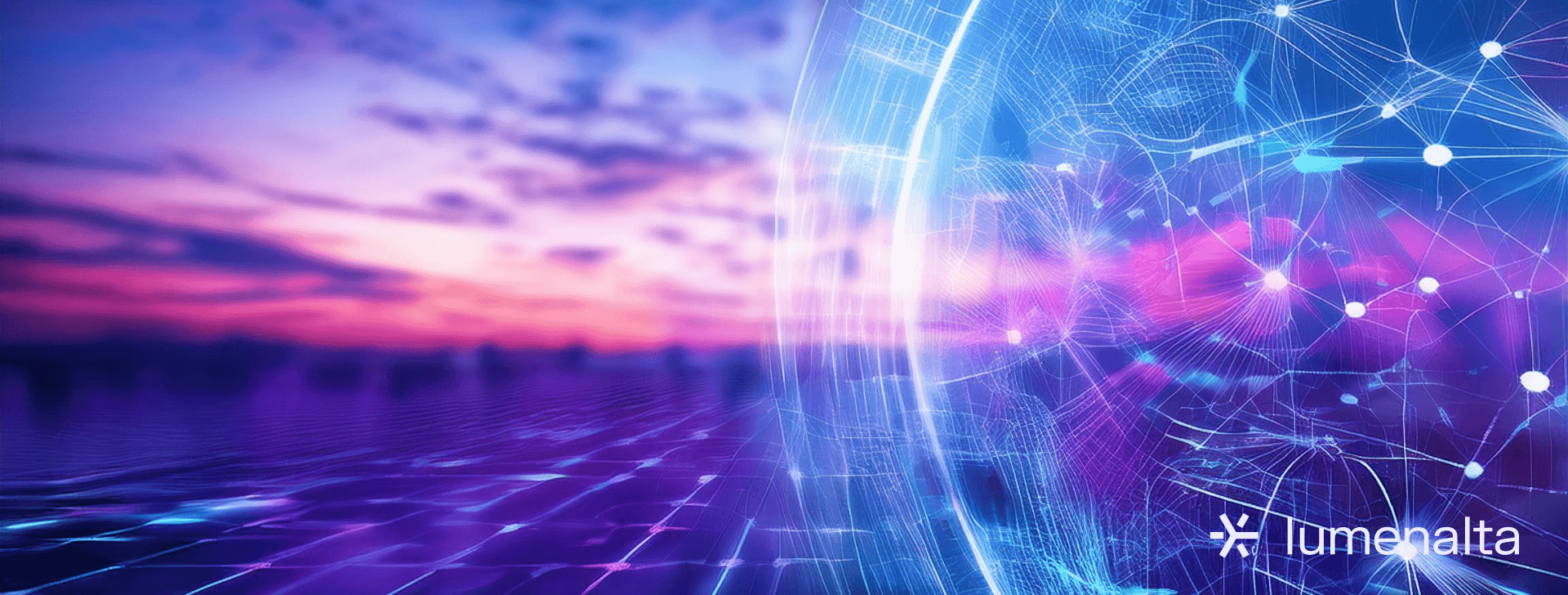
Generative, predictive, or conversational AI? Understanding the differences
OCT. 2, 2024
5 Min Read
AI is reshaping the future of business, transforming how organizations engage with customers, create content, and predict outcomes.
From chatbots delivering real-time assistance to algorithms forecasting demand shifts, technologies like generative AI, predictive AI, and conversational AI are becoming essential tools for innovation and efficiency. Each AI model offers distinct capabilities: generative AI thrives in content creation by producing original text, images, or code; predictive AI leverages historical data to provide actionable insights through forecasts; and conversational AI drives dynamic, human-like engagement across multiple touchpoints, ensuring seamless interactions.
The key to unlocking AI's potential lies in aligning these technologies with business goals. Generative AI supports automation and creative scalability, predictive AI enables proactive decision-making, and conversational AI enhances customer experience through personalized, real-time interactions. Businesses that combine these technologies—such as using predictive analytics to enhance chatbot performance—can unlock deeper customer insights and operational efficiencies. Strategic integration of these AI models ensures not only immediate value but also long-term impact in an increasingly competitive landscape.
Key takeaways
- 1. Different AI models serve distinct functions: Generative AI creates content, predictive AI forecasts trends and outcomes, and conversational AI enables dynamic, real-time interactions.
- 2. Strategic integration of AI models unlocks synergies: Predictive AI can enhance chatbots by offering data-informed recommendations, while generative AI can power dynamic responses, delivering engaging customer experiences.
- 3. Conversational AI excels in personalized engagement: By maintaining fluid, real-time dialogue, conversational AI improves customer satisfaction, reduces response times, and offers seamless escalation to human agents.
- 4. Generative AI democratizes creativity and supports scalability: It enables businesses to automate creative processes like content generation and coding, making innovation accessible across teams.
- 5. Predictive AI empowers data-driven decisions: Through advanced forecasting, predictive AI provides insights that help businesses optimize supply chains, finances, and customer experiences.
- 6. Choosing the right AI tool ensures impact: Aligning AI models with specific business objectives guarantees greater efficiency, productivity, and operational success. Integrating multiple AI types can amplify outcomes across industries, from retail to healthcare.
Why differentiating AI types is crucial for businesses
To stay competitive in today’s fast-evolving landscape, businesses need to integrate AI technologies strategically into their operations. However, not all AI models serve the same purpose, and choosing the wrong type can lead to inefficiencies, wasted resources, and missed opportunities. Generative AI focuses on producing new content, such as text or images, predictive AI leverages historical data to forecast trends, and conversational AI enables real-time interactions with customers. Each category addresses a distinct set of challenges, making it essential for organizations to understand their unique capabilities and use them effectively.
Proper differentiation allows companies to deploy AI solutions tailored to their specific needs, whether for automating content creation, enhancing customer engagement, or improving decision-making with data-driven insights. For instance, generative AI may be ideal for marketing teams looking to create personalized email campaigns at scale, while predictive AI can empower logistics teams to forecast demand and optimize inventory management. Conversely, conversational AI is particularly valuable for industries requiring continuous customer interaction, such as retail and finance, where virtual assistants and chatbots can streamline user experiences. Aligning the right AI type with operational goals ensures businesses achieve greater efficiency, productivity, and competitive advantage.
What is conversational AI?
Conversational AI engages users through dynamic, human-like conversations across text and voice channels. Unlike traditional chatbots that rely on predefined scripts, conversational AI uses natural language processing (NLP) to understand user input and machine learning (ML) to improve over time. This makes the system adaptable, capable of understanding context, and responsive to different tones and phrases, delivering a fluid conversational experience.
A core strength of conversational AI is real-time interaction. Systems like virtual assistants and chatbots respond immediately to user queries, helping with everything from customer service to scheduling appointments. With dialogue management capabilities, these tools can maintain the flow of multi-turn conversations, ensuring responses remain relevant even as topics shift. Additionally, conversational AI integrates with sentiment analysis tools, detecting frustration or satisfaction in user input, which helps provide more appropriate and empathetic responses.
The key differentiator of conversational AI is its ability to engage users throughout the interaction lifecycle, adapting to real-time dialogue and escalating complex inquiries to human agents when necessary. For example, customer support chatbots may resolve routine inquiries but seamlessly hand off complicated issues to human representatives with the conversation history intact. This ensures both efficiency and a personalized customer experience, making conversational AI particularly valuable for industries like e-commerce, finance, and healthcare.
"AI technologies serve diverse roles across industries, each addressing distinct operational needs—from automating creative production to enabling real-time customer interaction and forecasting future trends."
What is generative AI?
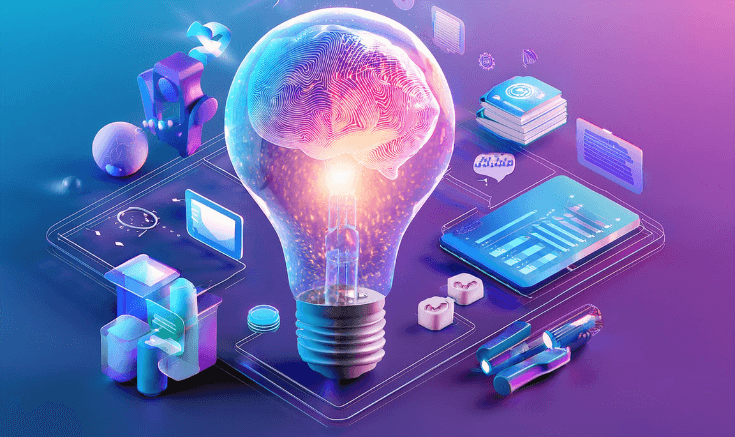
Generative AI tools are widely used in marketing to create personalized email campaigns, social media posts, and product descriptions. In the creative industries, artists and designers use AI to generate visuals or explore new design possibilities. Software developers leverage generative AI to write code snippets or prototype applications. Furthermore, tools like ChatGPT and DALL·E demonstrate how generative AI can assist users by drafting emails, generating visual art, or summarizing large texts.
One of the most significant aspects of generative AI is that it democratizes creativity by making tools accessible to non-specialists, enabling companies to scale content production efficiently. While the technology holds great promise, challenges around bias, intellectual property, and data privacy remain, as generative models rely heavily on vast datasets for training.
What is predictive AI?
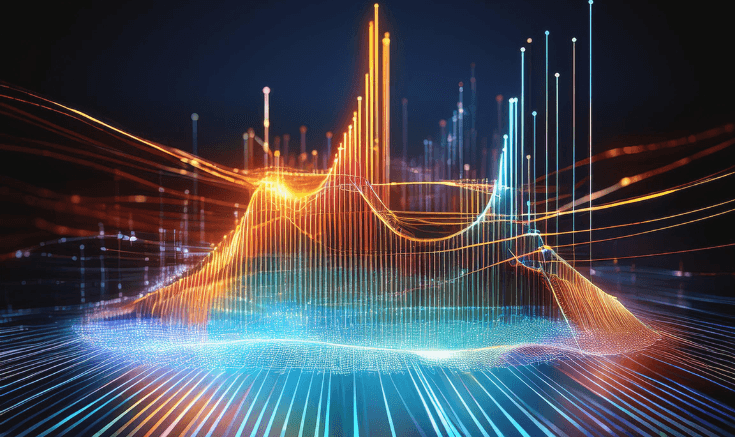
This type of AI is used extensively in finance to predict stock movements, credit risks, or loan defaults. In retail, predictive AI helps companies forecast product demand and optimize inventory management, reducing the risk of overstocking or stockouts. Healthcare organizations rely on predictive AI for patient monitoring, predicting disease outbreaks, and personalizing treatment plans based on historical medical data.
The predictive power of AI is not limited to historical trends; advanced predictive models incorporate real-time data inputs, allowing businesses to adjust strategies dynamically. This makes predictive AI invaluable for supply chain management—where disruptions can be anticipated early—or for marketing campaigns, where personalized recommendations based on predictive analytics can improve customer engagement.
While predictive AI provides powerful insights, it is essential to manage the risks associated with biased data and model accuracy. Businesses must continuously update and refine their predictive models to ensure reliable forecasts.
Generative AI vs. predictive AI vs. conversational AI
AI technologies serve diverse roles across industries, each addressing distinct operational needs—from automating creative production to enabling real-time customer interaction and forecasting future trends. This expanded comparison offers a deeper look at the core differences, use cases, and potential integrations of generative AI, predictive AI, and conversational AI, helping businesses make informed choices about the right AI models to deploy for their specific goals.
Feature | Generative AI | Predictive AI | Conversational AI |
---|---|---|---|
Primary focus | Content creation | Forecasting outcomes | Real-time interaction |
Key technology | Transformers, generative adversarial networks (GANs), variational autoencoders (VAEs) | Statistical algorithms, time series analysis, regression models | NLP models, ML models, dialogue management systems |
Use cases | Marketing (ad copy, product descriptions), creative industries, software development | Finance (stock market predictions), logistics (inventory forecasting), healthcare (patient risk prediction) | Customer service, virtual assistants, e-commerce chatbots |
Output | New content (e.g., text, images, videos, code) | Forecasts, trends, predictions | Interactive dialogue across channels |
Strength | Mimics human creativity, democratizes content production | Provides actionable insights and proactive decision-making | Engages users in real time and adapts to emotions |
Challenges | Managing bias, intellectual property, and data privacy concerns | Requires frequent model updates to maintain accuracy, susceptible to data bias | Ensuring conversational continuity, emotional recognition, and smooth human-agent handoff |
Industry examples | Retail & marketing: Generates personalized product descriptions | Finance: Predicts loan defaults and credit risks | Banking & healthcare: Virtual assistants handle customer queries and patient support |
Integration potential | Can integrate with predictive AI for personalized marketing content | Enhances conversational AI by offering data-driven recommendations | Combines with generative AI to deliver creative and engaging chat responses. |
Selecting the right AI tool involves evaluating your business’s immediate and long-term goals, the type of data available, and the operational challenges you aim to address. Organizations can also benefit from combining multiple AI models—such as integrating predictive AI with conversational systems for more targeted customer interactions—to unlock even greater value and efficiency.
Key differences between AI models
Core focus
- Generative AI: Creates new content (text, images, videos) based on patterns in large datasets.
- Predictive AI: Uses historical data to forecast outcomes, such as demand trends or customer behavior.
- Conversational AI: Delivers real-time, context-aware interactions via chatbots and virtual assistants.
Key technologies
- Generative AI: Transformers, GANs, variational autoencoders (VAEs).
- Predictive AI: Statistical models, regression, time series analysis.
- Conversational AI: NLP models, ML algorithms, dialogue management systems.
Business applications
- Generative AI: Marketing automation, personalized product descriptions, content creation.
- Predictive AI: Financial forecasting, supply chain optimization, healthcare risk prediction.
- Conversational AI: Virtual assistants in banking, customer service bots, educational chat support.
Integration potential
- Predictive AI enhances conversational AI by offering data-informed recommendations.
- Generative AI can be embedded within conversational systems to generate dynamic, engaging responses.
Successfully deploying AI requires businesses to balance the strengths and limitations of each model. Generative AI drives creative automation, predictive AI delivers actionable insights, and conversational AI engages users through real-time interactions. Addressing the challenges specific to each model ensures sustainable, effective AI solutions. The real value, however, comes from integrating these technologies strategically to unlock their full potential—providing not only innovation but also tangible business outcomes. With thoughtful planning, businesses can deploy AI solutions that enhance productivity, foster meaningful customer engagement, and maintain operational excellence.
"Organizations can also benefit from combining multiple AI models—such as integrating predictive AI with conversational systems for more targeted customer interactions—to unlock even greater value and efficiency."
Combining AI models for greater impact
The future of business lies not just in adopting individual AI technologies, but in strategically integrating different AI models to unlock synergies that maximize operational impact. By combining generative, predictive, and conversational AI, businesses can achieve higher efficiency, deeper insights, and more engaging customer experiences.
Examples of combined AI models:
Predictive + conversational AI
- Integrating predictive AI with chatbots or virtual assistants helps anticipate user needs in real time. Predictive models can analyze behavior patterns to recommend products or proactively address common issues during chats.
- In retail, a chatbot integrated with predictive AI might suggest product alternatives based on trending demand or inventory changes, driving customer satisfaction and sales.
Generative + conversational AI
- Embedding generative AI into conversational systems enhances engagement by producing dynamic, personalized responses. For example, a travel assistant powered by generative AI can generate unique itineraries based on user preferences.
- This combination creates engaging dialogues that feel human, adding value through storytelling or tailored recommendations.
Predictive + generative AI
- Predictive AI models forecast customer behavior, while generative AI can produce targeted marketing content such as personalized emails or product descriptions based on those insights.
- This collaboration allows companies to automate campaigns while maintaining a high degree of relevance.
All three models working together
- A healthcare chatbot might combine all three models to provide comprehensive patient support: predictive AI for risk assessments, generative AI for personalized care plans, and conversational AI for real-time interaction.
The integration of multiple AI models ensures businesses offer personalized, efficient, and meaningful interactions while optimizing operations at scale. Combining the models helps organizations meet customer needs proactively and deliver innovative experiences seamlessly.
Challenges and limitations of each AI model
While AI technologies provide significant benefits, businesses must address specific challenges associated with each model to ensure sustainable and effective deployment.
Generative AI
- Bias in content: Models can replicate biases present in the datasets they were trained on.
- Intellectual property risks: Generated content might resemble copyrighted materials, leading to legal concerns.
- Data privacy issues: Training requires extensive datasets, raising compliance challenges with privacy regulations.
Predictive AI
- Model decay: Predictive models must be updated frequently to maintain accuracy, especially in fast-changing environments.
- Data bias: Incomplete or biased datasets can distort forecasts, leading to flawed decision-making.
- Interpretability: Predictive outputs often require expert interpretation to ensure appropriate actions are taken.
Conversational AI
- Maintaining natural conversations: Long-term, fluid interaction remains challenging, particularly in complex dialogues.
- Sentiment analysis: Detecting subtle emotional shifts accurately is difficult, impacting the quality of engagement.
- Seamless human-agent transitions: Smooth handoffs during escalated queries are essential but often tricky to execute effectively.
The convergence of these three AI types opens new possibilities for business optimization and customer engagement. While generative AI excels at creating personalized content at scale, predictive AI empowers organizations to forecast trends and mitigate risks with data-driven insights. Meanwhile, conversational AI bridges the gap between human and digital interactions, offering real-time support that feels personalized and context-aware. Businesses that combine these technologies can amplify their operational capabilities—for example, by embedding predictive AI insights within conversational chatbots to anticipate user needs or using generative AI to provide personalized responses in real time. Ultimately, selecting and integrating the right AI solutions ensures greater efficiency, enhanced customer experiences, and competitive advantage in an increasingly AI-driven landscape.
Common applications of conversational AI across industries
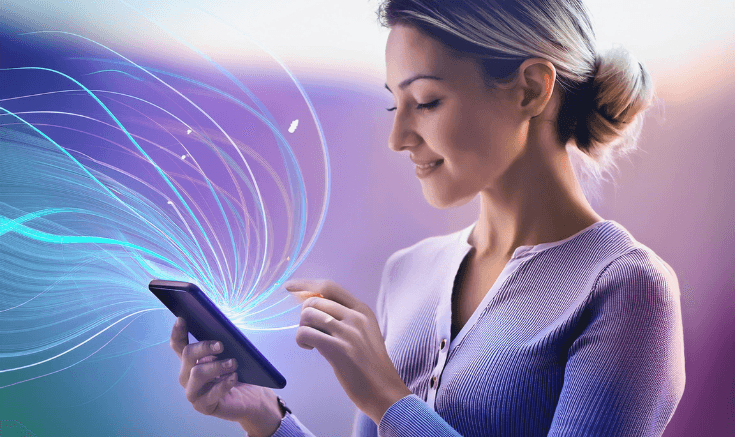
- Retail and e-commerce: Chatbots assist with product recommendations, order tracking, and returns processing.
- Healthcare: Virtual assistants help with appointment scheduling and symptom checking, improving patient care.
- Banking: AI agents manage account inquiries, fraud detection, and loan applications, enhancing customer service.
- Travel and hospitality: Virtual agents streamline booking processes and provide personalized travel recommendations.
- Education: Chatbots offer academic support, handle administrative tasks, and facilitate online learning.
These real-world applications demonstrate how conversational AI empowers businesses to operate more efficiently by automating repetitive tasks and enhancing service delivery. By integrating AI systems into various processes, organizations can deliver consistent, personalized support across channels, improve customer satisfaction, and boost productivity. As industries continue to evolve, the demand for intelligent, real-time interactions will only grow, making conversational AI an indispensable part of future business strategies.
Key differentiator of conversational AI
The defining strength of conversational AI lies in its ability to facilitate real-time, context-aware interactions that feel natural and seamless. Unlike generative and predictive AI, which focus on content creation and data-driven forecasts, conversational AI prioritizes dynamic user engagement.
Here’s how conversational AI differentiates itself:
- Instant response times: Ensures users receive answers immediately, improving customer experience and reducing waiting times.
- Context-aware conversations: Adapts to the flow of dialogue, maintaining relevance as topics evolve or shift mid-conversation.
- Sentiment analysis integration: Recognizes user emotions such as frustration or satisfaction and adjusts responses to offer a more empathetic interaction.
- Multi-turn dialogue management: Ensures continuity across multiple exchanges, making conversations feel cohesive and human-like.
- Seamless human-agent transition: Transfers complex queries to human agents when needed, with full conversation history for a smooth handoff.
- Omnichannel presence: Operates across chat, voice, and other messaging platforms to provide consistent engagement across channels.
These strengths make conversational AI ideal for industries such as customer service, finance, and healthcare, where personalized and immediate interactions drive customer satisfaction. The ability to engage users effectively and respond in real time not only enhances user experience but also builds long-term relationships and trust.
Future trends shaping generative, predictive, and conversational AI
AI technologies are advancing rapidly, evolving to meet the increasing demands for smarter, more adaptive systems. As businesses seek deeper insights and more personalized interactions, AI models are beginning to integrate and complement one another, giving rise to new possibilities. The following trends outline the key directions shaping the future of generative, predictive, and conversational AI, highlighting how these models are likely to evolve in tandem.
- Multimodal AI: Future systems will integrate text, voice, and image inputs, offering more immersive user experiences.
- Proactive AI: Predictive models will power conversational AI to anticipate user needs and deliver personalized interactions.
- Emotional AI: Advancements in sentiment analysis will enable AI systems to detect emotions and respond empathetically.
- AI fusion: Generative, predictive, and conversational AI technologies will increasingly complement each other, delivering enhanced business outcomes.
These trends highlight the evolving capabilities of AI, empowering businesses to create smarter, more responsive systems that meet user demands.
Lumenalta’s approach aligns with these trends by empowering businesses to integrate the right AI tools to meet their operational goals. With a focus on sustainable growth and innovation, we help ensure that our clients maintain ownership of their AI solutions, delivering long-term impact and competitive advantage.
table-of-contents
- Why differentiating AI types is crucial for businesses
- What is conversational AI?
- What is generative AI?
- What is predictive AI?
- Generative AI vs. predictive AI vs. conversational AI
- Common applications of conversational AI across industries
- Key differentiator of conversational AI
- Choosing the right AI for your business needs
- Future trends shaping generative, predictive, and conversational AI
- Common questions about generative AI vs. predictive AI vs. conversational AI
Common questions about generative AI vs. predictive AI vs. conversational AI
What are the main differences between generative AI and predictive AI?
What is the key differentiator of conversational AI compared to other AI models?
When should a business choose conversational AI over predictive AI?
How can companies combine multiple AI models effectively?
What are the challenges of adopting generative, predictive, and conversational AI?
Want to learn how artificial intelligence can bring more transparency and trust to your operations?