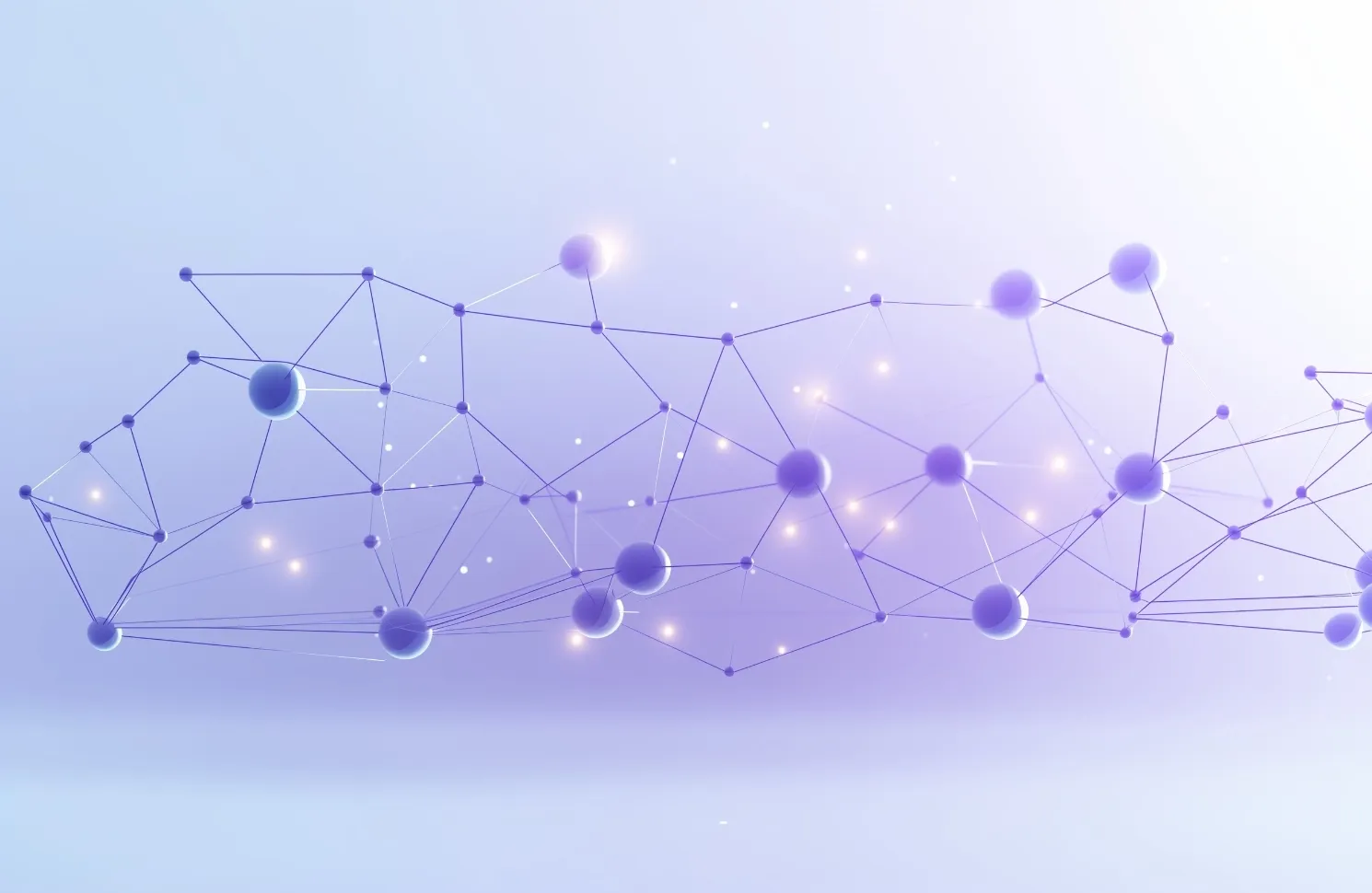
The paradox of data quality
JUL. 24, 2024
2 Min Read
Every organization has limited resources, and dedicating too much of these to pursuing perfect data takes away from competing priorities.
It makes intuitive sense: If data is the lifeblood of modern organizations, then maximizing data quality should be a top priority.
Turns out that thinking is a bit too cut-and-dried. There’s a paradox at work — strong data quality is important, but an obsession with achieving near-perfect data can be counterproductive.
Every organization has limited resources, and dedicating too much of these to pursuing perfect data takes away from competing priorities. Instead, a balanced approach focused on preventing quality issues at the source is often the best course of action.
The myth of perfect data quality
Pepar Hugo, Sr. Data Engineer at Lumenalta, says that “chasing perfect data resembles a siren’s song — alluring yet ultimately treacherous.” Organizations often find themselves sinking valuable time, money, and resources into an endless quest for an unattainable goal.
The reality of limited resources
Organizations are drowning in data. 463 zettabytes of data will be created on a daily basis by 2025, and managing it requires hefty investments in tech, infrastructure, and skilled professionals.
It’s not just the sheer volume that’s a challenge. Raw data is rarely usable in its original form. It needs to be cleaned, transformed, and meticulously validated before offering any meaningful insights. Each of these is often a time-consuming and complex process.
And even after all that effort, data quality assurance is an ongoing battle. New data is constantly coming in, requiring continuous monitoring and maintenance. Even for organizations with deep pockets, keeping up with robust data quality tools can be a financial burden.
The hidden costs of perfection
What’s more, the pursuit of perfection eventually leads to diminishing returns. As data quality improves, the payoff from each incremental improvement shrinks, and you eventually hit a point where the resources required to achieve marginal improvements outweigh the benefits gained.
There are also opportunity costs to consider. The time, money, and manpower poured into achieving data perfection must come from somewhere. Unless your organization has a lot of slack built in, other critical business priorities will suffer.
Upstream versus downstream data quality management
Many companies find themselves trapped in a cycle of reactivity. Rather than preventing quality issues at the source, they play a never-ending game of whack-a-mole as problems pop up.
Breaking free of this cycle requires a mindset shift: instead of dealing with issues as they arise downstream, it’s better to focus on the root of the problem.
Pepar uses a manufacturing analogy to illustrate the point. “Imagine your data pipeline as a factory assembly line. If a faulty product rolls off the conveyor belt, you could try to fix each individual defect, but that’s a time-consuming and inefficient process. The smarter approach is to fix the mold itself.”
By focusing on data quality upstream — at the point of entry — you’re essentially fixing the mold, ensuring that the data flowing through your pipeline is accurate, consistent, and reliable from the beginning.
Think of investing in data quality like building a house. A sturdy foundation might seem like an extra expense at first, but it’s far more cost-effective than constantly dealing with cracks, leaks, and structural problems down the line.
Moderation as the key to data quality management
In addition to proactivity, most organizations’ data quality management goal should be finding the sweet spot between perfection and pragmatism.
“An excessive focus on perfection can quickly backfire,” Pepar highlights. “It usually leads to “analysis paralysis,” where organizations get so caught up in chasing flawless data that they neglect to act on the valuable insights they already have. This can stifle innovation and impede decision-making.”
Initially, some leaders may balk at the idea of “compromising” on data quality. It can feel like cutting corners. But this approach isn’t about lowering standards; it's about using your resources wisely.
Instead of chasing the mirage of flawless data, adopt a risk-based approach by defining what “good enough” looks like for different types of information. This involves setting thresholds for accuracy, completeness, and consistency that align with how the data is used and its impact on your business.
Examples of risk categories in data quality
High-impact data
This information drives your most important business decisions. Think financial reports for investors, customer data used for targeted marketing, or patient records in healthcare. For this kind of data, you should set the bar high with strict validation rules, frequent cleanups, and real-time monitoring.
Moderate-impact data
This data keeps your business running smoothly, like inventory numbers, employee records, or sales figures. It’s important but not mission-critical. You can afford to be more relaxed here, perhaps relying on regular audits and occasional cleanups to maintain quality.
Low-impact data
This is the data you use for general insights and analysis, like website traffic stats or social media metrics. Since it doesn’t directly impact major decisions, the quality standards can be more flexible. Basic checks and occasional tidying up should be enough to keep things in order.
Advantages of a risk-based approach to data quality
Maximizes ROI
Focusing on specific areas helps you avoid falling prey to diminishing returns. Rather than wasting resources on minor issues, prioritizing high-risk elements gives you the biggest bang for your buck.
Reduces risk
A risk-based approach allows organizations to efficiently identify and mitigate their biggest vulnerabilities.
Improves decision-making
High-quality data is the foundation of informed decision-making. When you trust the accuracy of your most critical data, you can confidently make mission-critical choices.
How to build a robust data foundation
So, how do you go about building a robust foundation for your data? Here’s an overview from Pepar.
“Start with a thorough assessment of each data element. Ask tough questions: What are the potential consequences if this data is inaccurate or incomplete? How likely is it that this data will be compromised? How vulnerable is it to unauthorized access or manipulation?”
Use the answers to these questions to create a risk profile for each data element and prioritize your data quality efforts accordingly. High-stakes data — the kind that could significantly impact your business — gets the most attention. Less critical data, while still important, might not need the same level of scrutiny.
This approach is all about pragmatism. It acknowledges that perfect data is a fantasy and focuses instead on achieving a level of quality that’s good enough for your specific needs.
Guiding principles for data quality management
Implementing robust data quality frameworks
Strong data quality governance should encompass the following key elements:
Clearly defined roles and responsibilities
Everyone involved in handling data needs to know who’s in charge of what. This means designating data owners (responsible for overall data quality), data stewards (ensuring data follows the rules), and data custodians (managing the technical side of things).
Data quality standards
Set clear, measurable standards for what good data looks like in your organization. These standards should cover accuracy, completeness, consistency, and timeliness, among other factors. Ensure these standards align with your business goals and are regularly reviewed to stay relevant.
Issue resolution
Have a plan for dealing with data quality issues when they arise. There should be a process in place to figure out the root cause of the problem and take steps to prevent it from happening again.
Leveraging automation for efficiency
Manual data management is a relic of the past. Modern automation tools can take on a wide range of tasks, including:
Data cleansing
Think of this as spring cleaning for your data. Automated tools can quickly spot and fix errors, inconsistencies, and duplicates. They can also standardize formats and remove outliers.
Data validation
Automated validation rules can check for valid data types, ranges, and formats, as well as enforce specific business rules.
Data lineage tracking
Automated tools can track your data’s journey, which is incredibly useful for understanding and fixing quality problems.
Importance of a proactive, risk-based approach
The pursuit of perfect data is a noble goal, but it’s not always the most practical one. In the real world, organizations face constraints on their time, resources, and budget.
A proactive, risk-based approach to data quality management is the answer for most businesses. Prioritizing efforts upstream — fixing the mold rather than individual defects — lets you ensure that data is accurate and reliable from the very beginning. In doing so, CIOs can ensure their data works for them, not the other way around.