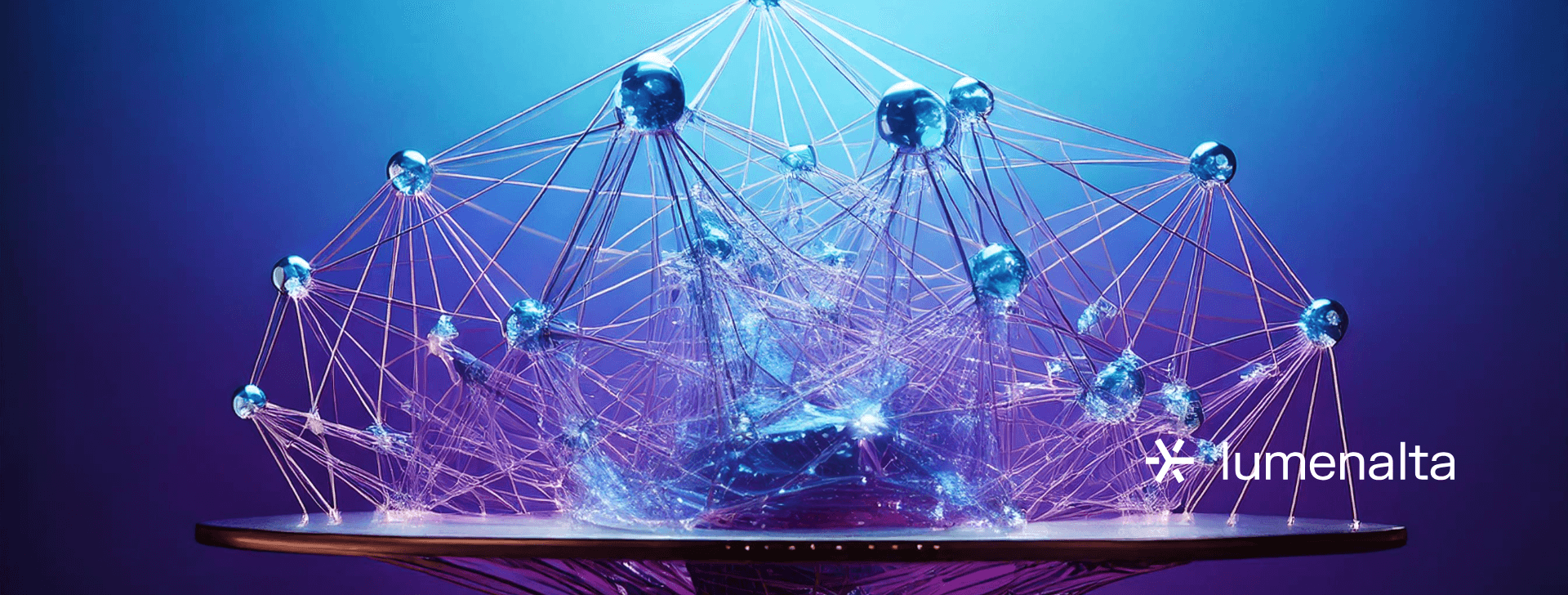
Fairness in generative AI
DEC. 29, 2024
6 Min Read
Fairness in generative AI is more than a technical objective—it is a critical foundation for building systems that align with ethical values and societal needs.
Without addressing fairness, generative AI risks perpetuating biases, creating inequities, and eroding trust. As these systems continue to shape industries and influence everyday decisions, implementing fairness principles ensures that generative AI not only innovates but also uplifts various communities and stakeholders.
Key takeaways
- 1. Fairness in generative AI is essential to creating systems that produce unbiased and inclusive outputs across different contexts.
- 2. Developing equitable AI systems requires varying datasets, fairness-focused algorithms, and collaborative, multidisciplinary approaches.
- 3. Transparency and explainability empower users to trust and evaluate AI systems while addressing biases in outputs.
- 4. Continuous monitoring and synthetic data enhance fairness by improving representativeness and adapting to societal changes.
- 5. Trends in fairness focus on early integration, participatory design, and regulatory clarity, shaping future developments in generative AI.
Understanding fairness in generative AI
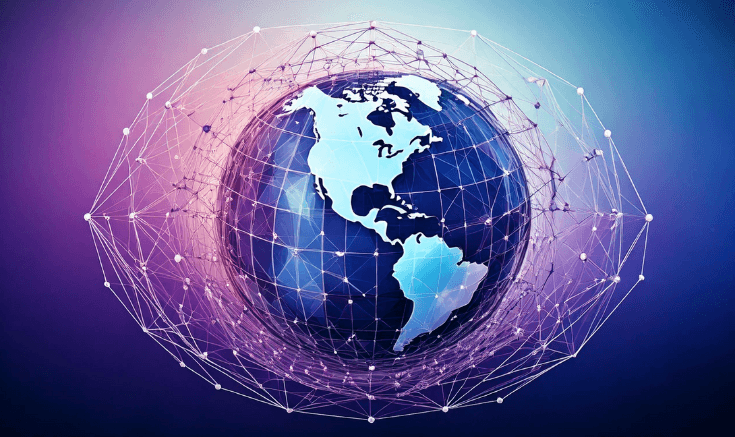
Fairness in generative AI refers to the development and deployment of artificial intelligence systems that produce outputs without bias, ensuring equitable treatment across different demographic groups and contexts. This principle has grown significantly as generative AI applications expand into critical areas like hiring, healthcare, and financial services. When AI-generated decisions or outputs perpetuate existing inequities or introduce new biases, they can amplify societal disparities and damage trust in these systems.
The principle of fairness in generative AI entails designing systems that respect inclusivity while minimizing bias in data, algorithms, and outputs. Achieving this requires a multi-faceted approach that addresses the inherent challenges in AI, such as biased training data or algorithmic predispositions. Developers and organizations must ensure these systems are transparent, justifiable, and aligned with ethical guidelines to create equitable outcomes.
Principles of fairness in generative AI
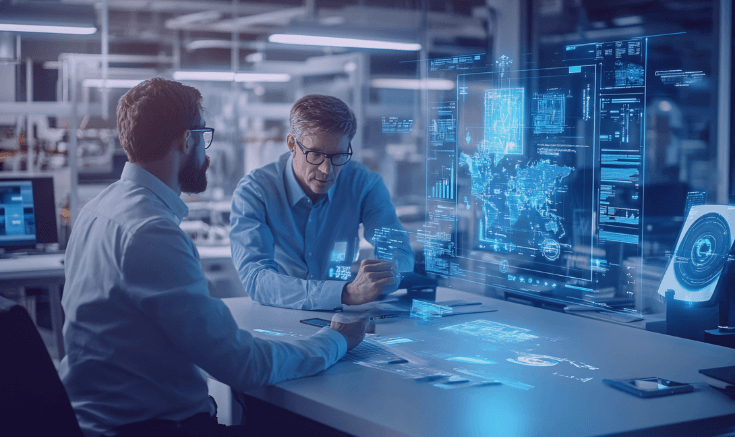
Fairness in generative AI reflects the ethical commitment to produce unbiased, equitable, and responsible outcomes. Generative AI has transformative potential, but without fair design and implementation, it risks amplifying existing societal disparities or introducing new forms of discrimination. Addressing fairness in these systems enhances their utility, broadens trust, and aligns their capabilities with ethical expectations. The principles below serve as a critical foundation for organizations striving to develop and deploy AI that meets these standards.
Inclusivity and representation
Effective fairness begins with representation. Generative AI systems require datasets that reflect the identities, experiences, and contexts of the users they serve. When training data captures a broad spectrum of variables, such as age, gender, ethnicity, geography, and socio-economic conditions, outputs are less likely to perpetuate existing inequalities or fail to meet user needs.
Inclusive design also considers the accessibility of AI systems for individuals with varying abilities. AI models that generate outputs usable across populations create broader opportunities for equitable outcomes. Collaboration with interdisciplinary teams and stakeholders during development introduces additional safeguards against blind spots, enriching the overall inclusiveness of the system.
Transparency and explainability
A transparent AI system reveals how it processes information and produces results. This principle ensures that stakeholders have a clear understanding of the data sources, algorithms, and workflows driving the system. Transparency allows for scrutiny, making it easier to identify potential sources of bias or errors.
Explainability builds on this by providing clear, accessible insights into why specific outputs were generated. Systems that deliver understandable explanations empower users to challenge outputs when necessary and contribute to addressing unintentional unfairness. Documenting methodologies and sharing these insights with relevant audiences can establish accountability and maintain confidence in the system.
Accountability in design and deployment
Accountability emphasizes the responsibility of organizations and developers in creating and maintaining fair AI systems. From the initial stages of data selection and model training to ongoing monitoring post-deployment, every step must be guided by ethical frameworks. Establishing governance processes allows organizations to take ownership of outcomes and correct any unintended consequences.
This principle also promotes the integration of proactive feedback mechanisms. Continuous monitoring and iterative improvements allow developers to address bias or fairness gaps dynamically. Accountability further requires independent audits, fostering trust and ensuring organizations remain aligned with fairness goals over time.
Fairness in outcomes
Fairness in outcomes centers on minimizing disparities in the effects or benefits of AI-generated outputs. Regular evaluations using fairness metrics help identify patterns where specific groups may be disadvantaged or unfairly favored. Outputs should undergo rigorous testing to assess alignment with the intended use cases and ethical guidelines.
In contexts such as hiring or healthcare, unfair outcomes could lead to serious social and economic consequences. Proactive calibration of models to reduce group-based inequities strengthens the reliability of generative AI systems. Fair outcomes serve as a tangible indicator of ethical success, ensuring that AI contributes positively across different applications.
These principles offer a structured approach to fairness in generative AI, aligning technology with ethical and societal needs. When organizations adopt these practices, they advance AI systems capable of fostering trust, reducing bias, and delivering equitable impact at scale.
“Fairness requires that generative AI systems are transparent in how they function and explainable in their outputs.”
Challenges in ensuring fairness in generative AI
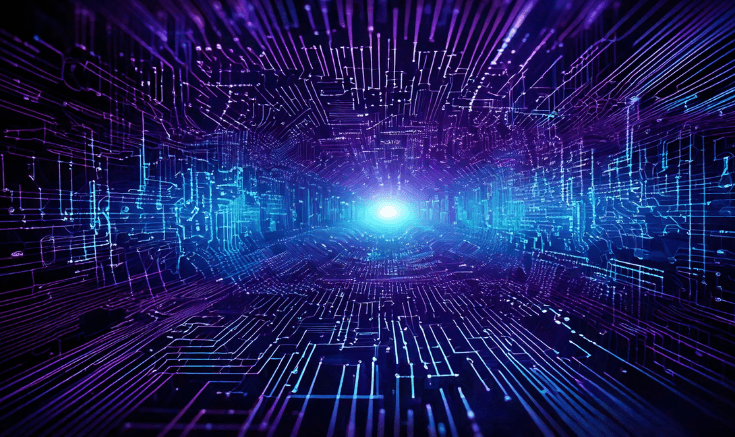
The path to achieving fairness in generative AI is filled with significant obstacles, ranging from technical complexities to ethical considerations. Generative AI systems, while powerful, operate within the constraints of their design and the data used to train them. These limitations introduce risks of bias, lack of accountability, and unintended outcomes, which can undermine trust and fairness in their applications. Understanding these challenges is critical for creating systems that uphold ethical standards while producing equitable outputs.
Each challenge reflects a nuanced issue in the lifecycle of generative AI systems. From the data used to the algorithmic choices made by developers, every stage presents unique risks that can inadvertently affect fairness. Without structured approaches to addressing these challenges, AI systems may perpetuate inequalities or fail to meet the ethical and societal standards expected of them.
- Bias in training data: The reliance of generative AI on vast datasets often results in outputs that reflect and reinforce historical and societal biases. Training data collected from historical sources can encode discriminatory patterns, which translate into biased outputs when left unchecked.
- Lack of diversity in development teams: When teams creating AI systems lack differing perspectives, the risk of overlooking critical fairness issues increases. Homogeneous teams may not identify biases in design, testing, or deployment, leading to unintended outcomes that disproportionately affect certain groups.
- Difficulty in measuring fairness: Fairness lacks a universal definition, and its measurement depends heavily on context. Establishing appropriate metrics and benchmarks for different applications is challenging, especially in areas requiring high ethical sensitivity.
- Algorithmic opacity: Many generative AI systems function as black boxes, with limited visibility into how they generate outputs. This opacity makes it difficult to evaluate or explain the fairness of the processes behind their results, complicating efforts to address potential issues.
- Trade-offs with performance metrics: Improving fairness can require balancing it against other performance measures, such as accuracy or speed. Achieving this balance without sacrificing critical functionality needs continuous iteration and prioritization.
- Dynamic societal norms: Fairness develops alongside societal values, ethics, and cultural standards. Keeping generative AI aligned with shifting norms requires flexibility in design and consistent monitoring throughout its use.
- Regulatory and ethical uncertainties: With laws and ethical standards around AI still developing, organizations face ambiguity about compliance requirements and best practices. This lack of clear guidance adds complexity to aligning AI systems with fairness expectations.
Overcoming these challenges is essential for the responsible use of generative AI across industries. Addressing bias, enhancing transparency, and fostering accountability are fundamental steps toward building systems that reflect societal values and create equitable opportunities. These challenges are not insurmountable, but they require a concerted effort from developers, regulators, and users to produce meaningful progress toward fairness in generative AI.
Best practices for implementing fairness in AI systems
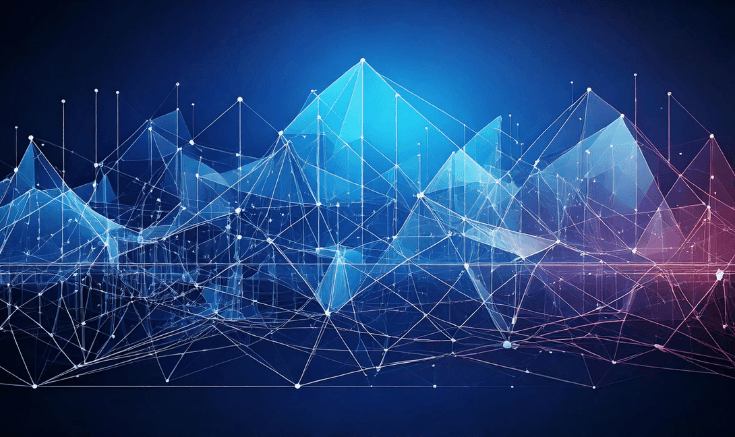
Embedding fairness into generative AI systems requires more than theoretical principles; it needs actionable strategies throughout development, deployment, and beyond. Organizations must address biases, promote inclusivity, and foster transparency to create systems that meet ethical and societal expectations. Fairness is not achieved through a single step but through consistent application of best practices that reduce bias, improve accountability, and promote equitable outcomes. These practices provide a critical framework for organizations to approach fairness methodically while maximizing the usability and trustworthiness of their AI systems.
Use diverse and representative datasets
Generative AI systems rely heavily on the quality of their training data. Diverse and representative datasets reduce the risk of outputs reflecting narrow, biased perspectives. When data includes varied demographic groups, socio-economic conditions, and cultural contexts, systems are better equipped to generate outputs that resonate equitably across different user bases.
Using data audits to identify and address representation gaps is an effective strategy for improving dataset quality. Additionally, practices such as oversampling underrepresented groups and removing irrelevant correlations in the data create opportunities for fairer outputs. Investing in dataset refinement ensures that the generative AI system operates in alignment with ethical goals and societal expectations.
Apply fairness-focused algorithms and techniques
Algorithms designed with fairness as a priority are powerful tools for addressing systemic biases in generative AI. Techniques such as re-weighting datasets during training, adversarial debiasing, or adding fairness constraints allow models to correct disparities and produce equitable results. These algorithmic adjustments create systems that balance fairness with performance, even when working with imperfect data.
Regular testing of these techniques during the development and deployment phases ensures they remain effective. Establishing fairness benchmarks tied to specific metrics or use cases further supports systems in meeting the fairness expectations set by developers and stakeholders.
Embed transparency and explainability
Transparency enhances trust in generative AI systems by clarifying how they operate. Explaining processes such as data collection, algorithm design, and output generation provides stakeholders with actionable insights into potential biases or flaws. Transparency also reduces the likelihood of misuse or misunderstanding, making it easier for users to assess and engage with the system.
Explainability tools like interpretable models and feature attribution techniques can further illuminate how AI systems function. These tools break down complex processes into understandable elements, making fairness assessments more accessible and actionable for technical and non-technical stakeholders alike.
Involve multidisciplinary teams
Fairness in generative AI benefits from the involvement of multidisciplinary teams. Collaboration between developers, ethicists, domain experts, and community representatives introduces multiple perspectives that highlight potential biases and fairness risks. Each stakeholder adds value by addressing unique challenges and creating solutions tailored to varied user contexts.
Multidisciplinary input also enhances the development process by addressing blind spots and balancing technical capabilities with ethical considerations. Bringing varied voices into conversations about fairness strengthens the credibility and impact of generative AI systems in real applications.
Monitor and audit systems continuously
Ongoing monitoring is essential for maintaining fairness in generative AI systems over time. These systems often progress as they interact with new data, contexts, or use cases, making regular evaluations critical. Continuous monitoring involves testing outputs against established fairness benchmarks and analyzing performance metrics for signs of bias or disparities.
Audits conducted at regular intervals provide an additional layer of accountability. Evaluations that include fairness testing, algorithm reviews, and user feedback create opportunities for developers to identify emerging issues and recalibrate systems as needed. Continuous improvement processes reinforce fairness as an ongoing commitment rather than a one-time goal.
Best practices for fairness in generative AI form the foundation for systems that align with ethical values and user expectations. Organizations that prioritize representative data, fairness-focused algorithms, and multidisciplinary collaboration develop systems capable of achieving equitable outcomes. Transparency and continuous monitoring further solidify this framework, creating an enduring standard for fairness in generative AI. These practices are not just tools for compliance; they are essential for building systems that users trust and value across a wide range of applications.
“Generative AI systems require datasets that represent the full spectrum of human diversity to reduce bias and promote fairness.”
7 examples of fairness in generative AI
Generative AI systems that prioritize fairness demonstrate how technology can address societal inequities and deliver inclusive solutions. These examples reflect thoughtful implementation, where fairness principles are embedded throughout the system design. Whether used for language translation, hiring platforms, or medical diagnostics, these systems highlight the transformative potential of generative AI when developed with equitable practices. Each case underscores the importance of reducing bias, increasing inclusivity, and meeting the needs of varied communities through AI innovations.
1. Equitable language translation systems
Language translation models have grown to incorporate regional dialects, gender neutrality, and cultural nuances, creating more inclusive and unbiased outputs. These systems rely on extensive datasets representing a variety of languages and dialects, reducing favoritism toward dominant languages. For instance, translation systems that handle gender-specific languages carefully avoid reinforcing stereotypes, delivering more balanced and appropriate results for users around the globe.
2. Bias-free hiring platforms
Generative AI has been applied to hiring processes to eliminate biases often present in traditional methods. Resume-screening algorithms trained with differing datasets reduce discrimination by anonymizing candidate profiles and focusing on skills rather than demographic indicators. These platforms increase opportunities for underrepresented groups and help organizations build more varied workforces without compromising hiring quality.
3. Inclusive content generation tools
Content generation tools designed with fairness principles help avoid stereotypes in text and media outputs. AI writing assistants trained on balanced datasets suggest language that is inclusive and free from harmful bias. These tools permit marketing, education, and communication professionals to engage mixed audiences effectively while maintaining ethical standards.
4. Accessible educational resources
Generative AI systems have been deployed to create educational materials for students with disabilities, offering outputs like text-to-speech content, Braille translations, and video captions. These systems expand access to education by addressing the learning needs of diversified students, fostering equity in academic opportunities. Inclusive educational tools demonstrate how fairness-focused generative AI can break barriers for marginalized groups.
5. Healthcare diagnostics with minimized bias
In healthcare, AI-based diagnostic systems are designed to minimize disparities across different demographic groups. These tools analyze medical data with consideration for gender, racial, and socio-economic factors to reduce inaccuracies in diagnoses. For example, models trained on varied medical datasets avoid biases that could disproportionately affect specific populations, leading to more equitable healthcare outcomes.
6. Fair credit scoring systems
AI systems for credit scoring have embraced fairness principles by using algorithms that exclude discriminatory variables such as race or gender. These models rely on alternative credit data and assess risk equitably, broadening access to loans and financial services for underserved communities. Fair credit scoring systems play a vital role in reducing socioeconomic disparities and expanding opportunities for financial independence.
7. Ethical artistic creation tools
Artistic generative AI systems promote inclusivity by incorporating different cultural datasets into their creative processes. Music, literature, and visual art generated by AI reflect a wide range of global traditions, respecting cultural diversity. These systems prioritize fairness by avoiding the overrepresentation of dominant cultural norms, fostering creativity that resonates with audiences worldwide.
These examples illustrate the meaningful impact of fairness in generative AI across industries and applications. Systems designed to reduce bias and foster inclusivity offer ethical and practical benefits, making technology more accessible and equitable. Generative AI can reshape industries while reflecting societal values, creating tools that empower various communities and promote positive change. Each successful application of fairness reinforces the importance of adopting ethical practices in AI design and development, setting the stage for future advancements that prioritize equity and inclusion.
Future trends for fairness in generative AI
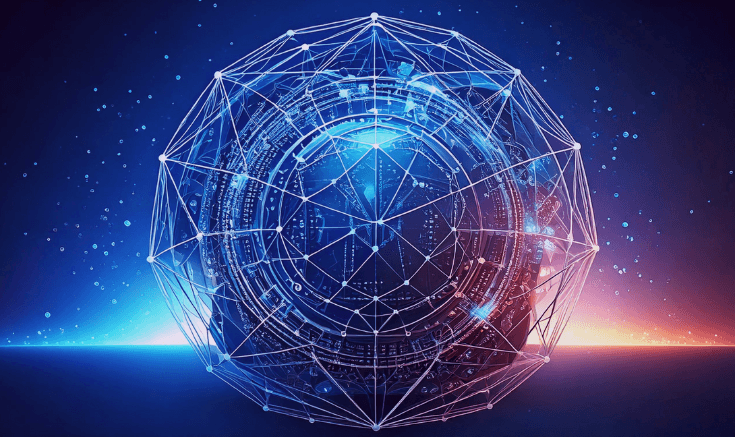
Fairness in generative AI is developing through innovations that address biases and align systems with societal needs. As AI becomes integral to industries like healthcare and education, creating equitable and inclusive systems is a priority. Emerging trends reflect a focus on early integration of fairness principles, advanced evaluation tools, and collaborative development practices.
Developers are incorporating fairness principles during the initial stages of AI design, reducing the likelihood of biased outputs. Improved fairness metrics and evaluation methods are helping to assess systems against benchmarks tailored to specific industries, allowing better identification of biases and solutions. Also, synthetic data is becoming an effective way to address representation gaps in training datasets. This approach allows developers to create balanced datasets without relying on historically skewed sources, enhancing inclusivity and equitable outcomes.
Partnerships between technologists, ethicists, and regulators are creating stronger guidelines and frameworks to align fairness practices globally. These efforts promote accountability and ensure that AI systems are transparent and aligned with ethical standards. The growing emphasis on community participation is ensuring fairness reflects the varied needs of users. Engaging with affected populations during the design and testing phases ensures outputs are relevant and equitable for a wide range of users.
Generative AI has the power to redefine industries and expand opportunities, but only when fairness is embedded into its design. At Lumenalta, we specialize in creating ethical, innovative solutions that meet the highest standards of inclusivity and equity. Partner with us to lead with AI systems that are designed to serve all.
Let’s build a brighter, fairer future together.
table-of-contents
Common questions about generative AI
What is one challenge in ensuring fairness in generative AI?
What does the principle of fairness in generative AI entail?
How can generative AI models be improved for fairness?
Why is transparency important in generative AI systems?
What industries benefit the most from fair generative AI systems?
Want to learn how generative AI can bring more transparency and trust to your operations?