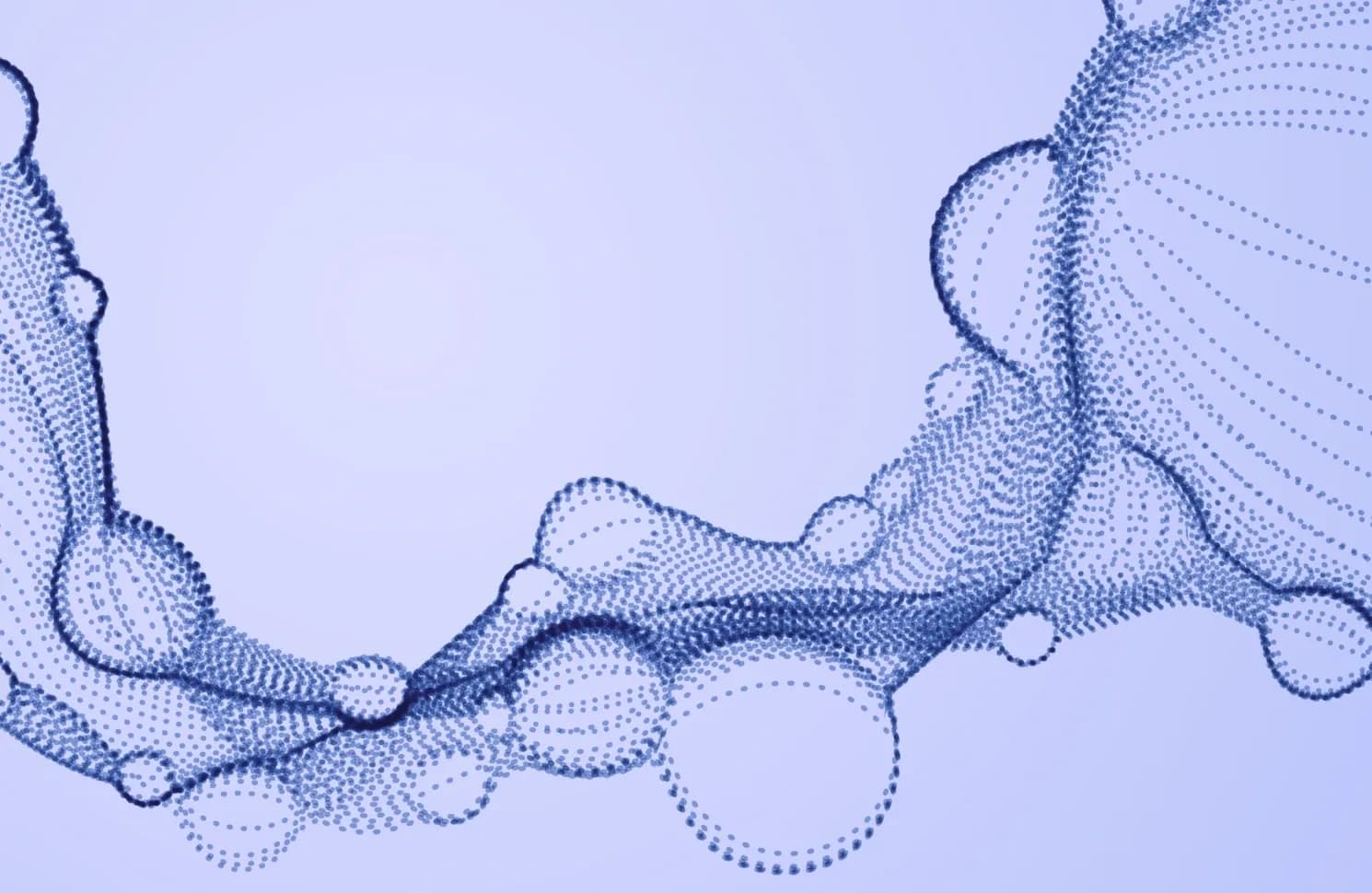
Generative AI architecture and knowledge work in financial services
DEC. 30, 2024
2 Min Read
Learn how advanced GenAI architectures help financial firms transform knowledge work and serve clients better.
Financial services institutions rely on knowledge work. However, knowledge work’s reliance on increasingly unstructured data and human expertise means that traditional data analytics aren’t as effective as they once were.
While traditional large-language models (LLM) and generative AI technologies have started to impact how financial service offerings are delivered, they aren’t quite equipped to address the pressing challenges of knowledge work. To remain competitive, financial services leaders are instead turning to advanced GenAI architectures.
By infusing important knowledge work with advanced generative AI architectures, such as retrieval-augmented generation (RAG), fine-tuning, model routing, and ontologies, institutions are reshaping how they operate, make decisions, and serve their clients.
A closer look at four advanced GenAI architectures
The financial sector is no stranger to technological innovation—and the advent of sophisticated generative AI architectures is ushering in a new era of possibilities. These technologies augment the power of LLMs by expanding their access to information and allowing businesses to be more incisive and impactful in their knowledge work.
These architectures include:
Retrieval-augmented generation (RAG)
RAG combines the power of large language models (LLMs) with the precision of information retrieval systems, producing contextually relevant outputs. Without RAG, LLMs can’t access data other than the general knowledge on which they were trained. This limits their ability to answer questions accurately and provide useful advice in the case of financial services. RAG helps overcome this limitation, multiplying the effectiveness of the GenAI technology.
In knowledge work, RAG models can enhance research capabilities, support content creation, and provide nuanced decision support by drawing from vast repositories of data or domain-specific knowledge such as financial information and market insights.
Fine-tuning and adaptive models
Out-of-the-box LLMs are generalists that can support you in a variety of basic tasks, but financial services require specialized information. Fine-tuning lets you train LLMs on specific tasks, domain-specific data, or company information so they can better understand and generate industry-specific content that supports both employees and customers. Think of it like this, an out-of-the-box LLM is just learning English, but a fine-tuned LLM is learning finance.
Routing to various models based on use cases
This technique involves directing queries to the most appropriate AI model based on the specific use case. In financial services, this could mean routing different types of financial analysis or customer inquiries to specialized models, ensuring optimal performance and accuracy while also reducing the burden of knowledge work.
Ontologies and knowledge graphs
Ontologies and knowledge graphs enhance generative AI by providing structured representations of domain-specific knowledge. While ontologies focus on defining logical relationships and rules, knowledge graphs store and query large amounts of interconnected data.
In financial services, ontologies can offer a formal framework for defining industry concepts and relationships and applying your specific institutional knowledge, while knowledge graphs can store vast amounts of interconnected financial data. By integrating these structures, financial institutions can improve the accuracy and relevance of AI-generated outputs. This approach significantly enhances AI systems’ ability to handle complex financial queries and perform knowledge-intensive tasks with greater precision and context awareness.
The problems these architectures solve
These advanced generative AI architectures address several critical challenges for financial institutions:
- Data scarcity and fragmentation: In an industry where data drives decisions, fragmented data and a lack of accurate information can get in the way of progress. Generalist LLMs aren’t equipped with enough relevant data to support financial services teams in optimizing their services. By implementing RAG, they can better synthesize insights from disparate relevant sources, providing a more comprehensive and incisive view of market trends, client needs, and more.
- Unstructured data and human expertise: Much of the valuable information in financial services exists in unstructured formats or as the tacit knowledge of experienced professionals. By fine-tuning LLM models and training them to specific domains within financial services, teams can transform unstructured data into actionable insights, complementing human intuition with accurate data-driven analysis that doesn’t require hours of research.
- Scalability and personalization: Financial services is a varied field—so a one-size-fits-all model may not cut it. Tailoring LLMs to specific domains and leveraging the most appropriate models for a given use case can make financial services teams more impactful as they deliver personalized services to their customers at scale.
Use cases for advanced GenAI architecture in financial services
Financial services institutions embrace generative AI architecture to multiply the power of LLMs and increase the effectiveness of their knowledge work across many domains and use cases. Here are some examples:
Investment research
Investment optimization: Quants can analyze market trends, trade patterns and economic indicators—at macro and micro levels—to generate insights and build models for more effective trading strategies, portfolio analysis, and manage risk. Analysis of time-series data enriched with additional events and signals can enable more advanced predictive models.
Wealth management
Personalized advisory: Wealth management teams can leverage RAG and knowledge graphs to provide tailored investment advice based on client profiles and market conditions, augmenting the capabilities of human advisors.
Client reporting: GenAI models can help automate the generation of personalized client reports with AI-driven insights and forecasts, improving client communication and satisfaction.
Commercial banking
Credit risk assessments: Teams use advanced generative AI architectures to analyze diverse financial data in order to evaluate creditworthiness more accurately and efficiently than traditional methods.
Fraud detection: Advanced AI systems can identify suspicious patterns and transactions in real time, bolstering security measures.
Consumer banking
Enhanced customer support: Chatbots and virtual assistants powered by fine-tuned LLMs trained on specific company and industry data can help handle complex customer inquiries, improving service quality, reducing response times, and freeing up time for customer support teams.
Financial planning: Generative AI tools can help customers manage their finances and plan for future goals, fostering financial literacy and customer loyalty.
Insurance
Streamlined claims processing: RAG models equipped to retrieve policy information, historical data, and incident reports can automate the evaluation of claims, making the process faster and more effective.
Intelligent underwriting: AI models can assess risk factors more comprehensively and provide policy adjustments and approvals.
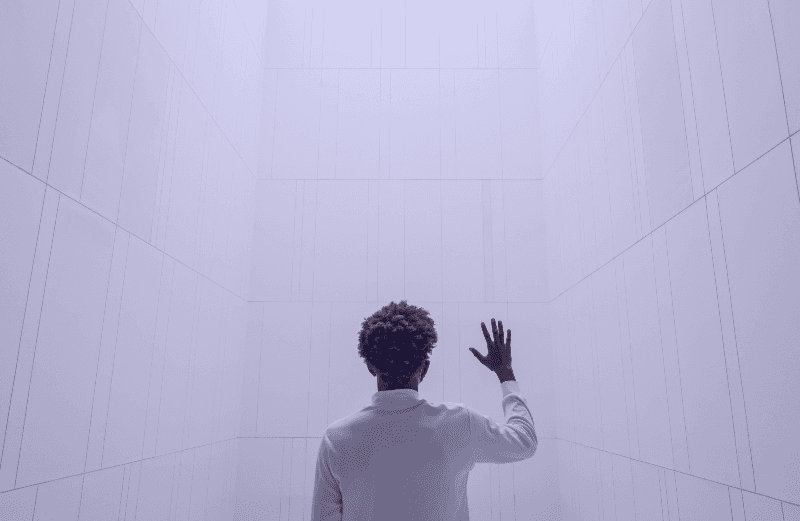
The benefits of generative AI in financial services
Beyond solving specific problems and expanding the potential of different domains, advanced GenAI models can offer several benefits and positive outcomes for knowledge work in financial services. These include:
- Enhanced decision making: RAG and fine-tuned models empower knowledge workers by rapidly providing more accurate and context-aware insights based on external sources and/or domain-specific information.
- Operational efficiency and cost savings: Each of the four advanced generative AI architectures can support teams in introducing more context-aware automation that streamlines processes. Plus, by providing more in-depth insights and rapid access to structured data and supporting employees with multiple models depending on the use case, they reduce the time spent on costly manual knowledge work.
- Empowering human workers: Getting rapid access to real-time, accurate, and actionable information from RAG and fine-tuned LLMs means that human professionals are freed up to provide their knowledge and intuition without the manual work previously required. Plus, teams can task GenAI models with routine and data-intensive processes so that their employees are freed up to focus on higher-order tasks.
The backbone of advanced AI systems: DataOps, LLMOps, and SRE
According to Gartner, “capturing business value from AI projects requires much more than having a high-performing AI model.” To fully leverage the power of advanced generative AI architectures in knowledge work, financial services teams should consider employing capabilities and methodologies that promote operational excellence, scalability, and more.
DataOps for knowledge work
Data quality, accessibility, and privacy are core considerations in financial services. When managing diverse and unstructured data sources, robust DataOps practices are crucial for ensuring quality, prioritizing data privacy, and promoting data governance and compliance—all while driving continuous improvement in productivity, throughput, and stability.
LLMOps and continuous improvement cycles
LLMOps plays a vital role in the efficient deployment, monitoring, and refinement of large language models. This empowers AI-driven systems and functions to operate more efficiently.
Meanwhile, implementing continuous improvement cycles allows institutions to incorporate real-time feedback, keeping AI models relevant and accurate in a fast-paced financial environment.
Site reliability engineering (SRE) and observability
Applying SRE principles—such as eliminating toil and embracing risk—supports the robustness and scalability of AI systems. In addition, enhanced observability allows for monitoring model performance and understanding model behavior in dynamic knowledge work environments, which is critical for maintaining trust in AI-driven financial services.
Human-in-the-loop: A crucial element in financial services knowledge work
While generative AI architectures offer immense potential, human oversight is still critical when dealing with unstructured data and complex financial decision-making. At the end of the day, human professionals have a deep understanding of the context behind every decision. Their critical thinking is needed for financial service offerings to be both accurate and impactful.
To successfully implement generative AI architectures in financial services, we need to:
Integrate human knowledge into AI systems
Develop strategies for capturing and embedding human expertise into AI workflows, ensuring that the collective wisdom of experienced professionals enhances AI outputs while also providing appropriate oversight in a highly regulated industry.
A human-in-the-loop approach supplements any initial analyses and recommendations provided by AI with final decisions that are reviewed and approved by human experts. Beyond reviewing decisions, strategies that integrate human knowledge into generative AI architecture could include further training the AI with customer support logs and content from experts or providing annotations and high-quality labels on any work produced.
Understand AI as an augmentation, not a replacement
There have been many concerns about AI taking away human jobs—but that’s far from the truth. It’s important to reframe the narrative around AI in finance, positioning it as a tool to augment human capabilities rather than replace knowledge workers. This approach fosters a symbiotic relationship between human intuition and AI-driven insights, leading to better outcomes for clients and institutions alike.
It’s time to start exploring generative AI architectures
As generative AI architectures continue to evolve, they promise to revolutionize knowledge work in the financial sector. By addressing challenges like data fragmentation, harnessing unstructured data, and enabling personalized services at scale, these technologies are set to empower financial institutions to make more informed decisions, mitigate risks more effectively, and provide stellar services to their clients.
For CIOs, CDOs, and data leaders in the financial services industry, the time to explore and invest in these advanced GenAI architectures is now. Not only will they be able to better support knowledge workers, but they’ll also drive innovation, maintain a competitive edge in an increasingly data-driven financial landscape, and unlock new possibilities that shape the future of the industry.
Ready to scale your financial services with AI?