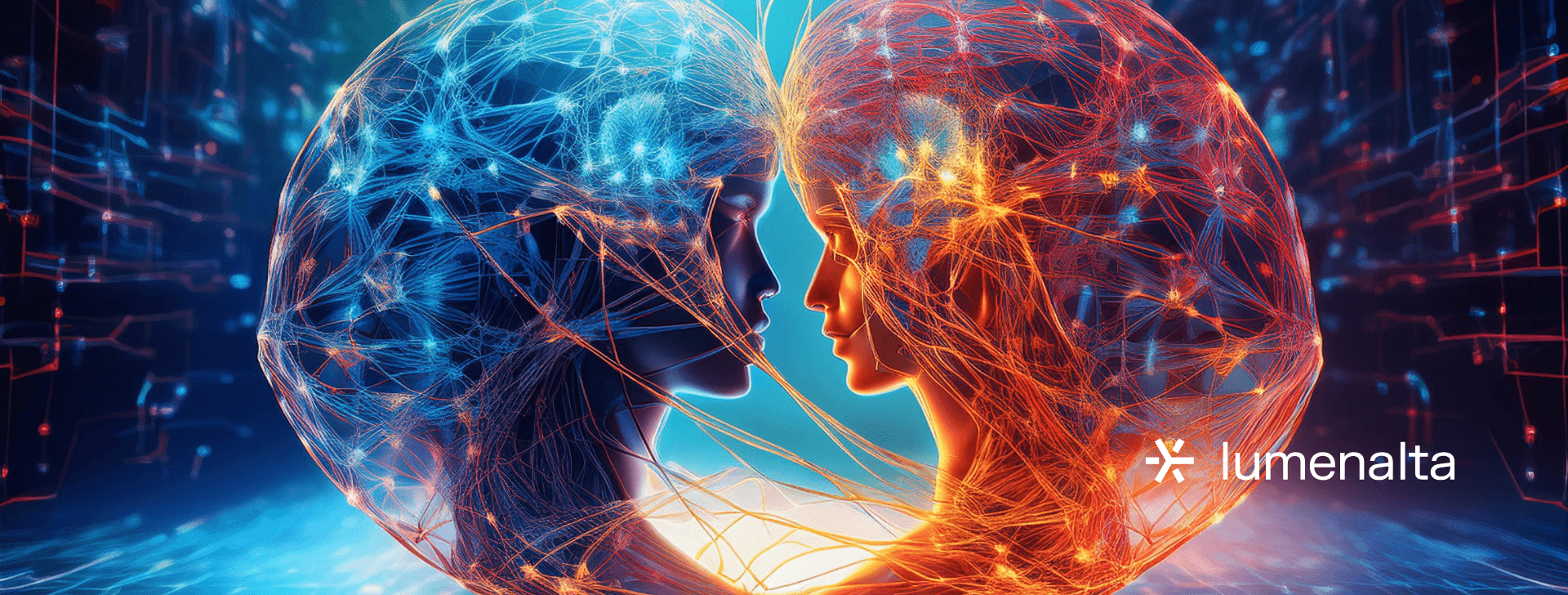
Natural language processing vs generative AI
JAN. 15, 2025
6 Min Read
Natural language processing (NLP) and generative AI (genAI) represent two of the most impactful advancements in artificial intelligence.
Both technologies have modified businesses' operations, offering solutions that enhance efficiency, scalability, and innovation. While NLP specializes in understanding and processing human language, generative AI focuses on creating new, human-like content. They provide businesses with tools to address challenges, reveal new opportunities, and achieve measurable results.
Key takeaways
- 1. NLP interprets and processes human language, while generative AI creates new content by learning from patterns in data.
- 2. Businesses can use NLP to analyze customer feedback, automate workflows, and extract insights from unstructured text.
- 3. Generative AI lets organizations produce scalable, human-like content, saving time and improving resource efficiency.
- 4. Combining NLP and generative AI supports applications like intelligent chatbots, personalized marketing, and automated reporting.
- 5. Selecting the right solution depends on your business goals, with both technologies offering significant opportunities for measurable impact.
What is natural language processing NLP?
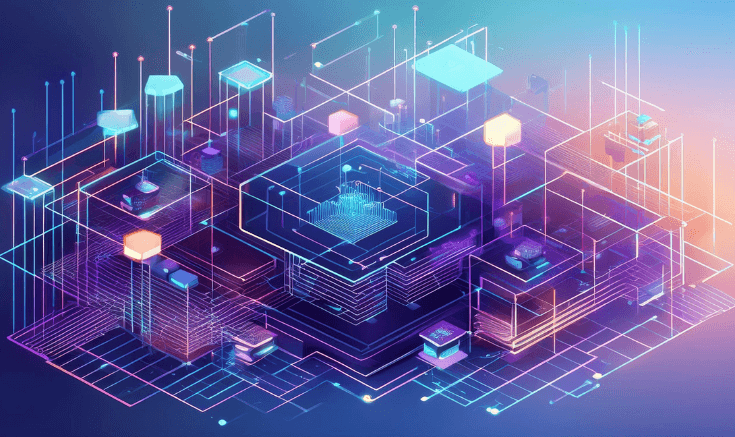
Natural language processing is an advanced field of artificial intelligence that authorizes computers to interpret and interact with human language. It involves using algorithms and computational models to analyze, process, and generate text or speech in meaningful ways. Breaking down the complexities of human communication allows NLP systems to understand the nuances of language, including syntax, semantics, and context.
NLP functions include language translation, text classification, sentiment analysis, and named entity recognition. These tasks power applications widely used in industries seeking efficiency and value creation, such as chatbots, email filters, and automated transcription services. NLP also plays a key role in extracting actionable insights from large volumes of unstructured text, such as customer feedback or legal documents, helping businesses discover untapped opportunities and optimize strategies.
Organizations integrate NLP solutions to reduce the time spent on manual processes, improve operational accuracy, and achieve better outcomes for internal stakeholders and end-users. These applications are scalable, making them suitable for businesses of all sizes, from startups streamlining processes to enterprises managing data more effectively. Adoption of NLP continues to show measurable impact in areas like cost reduction, customer satisfaction, and process optimization.
"Natural language processing allows machines to understand, interpret, and generate text or speech in ways that mimic human communication."
What is generative AI?
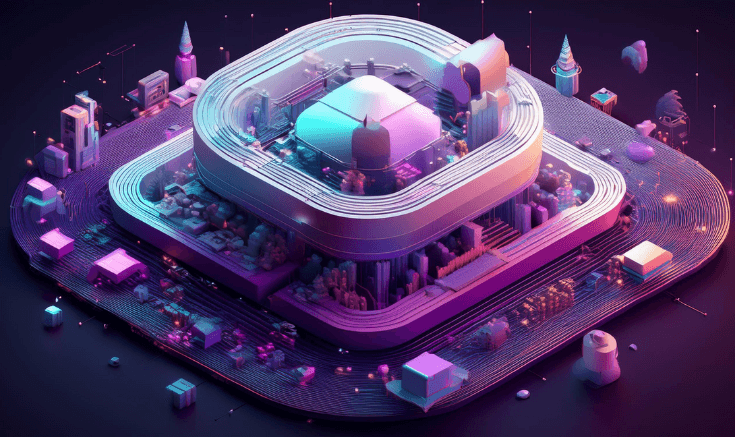
Generative artificial intelligence (AI) refers to machine learning models designed to create new, original content. Unlike traditional AI systems that classify or predict based on existing data, generative AI synthesizes outputs like text, images, videos, or audio by learning patterns from vast datasets. These systems use advanced architectures, such as transformers and generative adversarial networks (GANs), to generate content that mimics human creativity and intelligence.
Applications of generative AI include creating realistic images, drafting human-like text, producing code, and even composing music. This technology is reshaping how businesses approach creativity and automation, providing tools to accelerate workflows, personalize customer interactions, and scale content production. Generative AI also supports cost-effective innovation by enabling teams to experiment with prototypes and simulate scenarios in less time.
Organizations investing in generative AI can realize significant time-to-value improvements and explore untapped potential for scaling operations. As the technology progresses, it remains a critical tool for driving efficiency and maximizing business returns across industries.
Key differences between NLP and generative AI
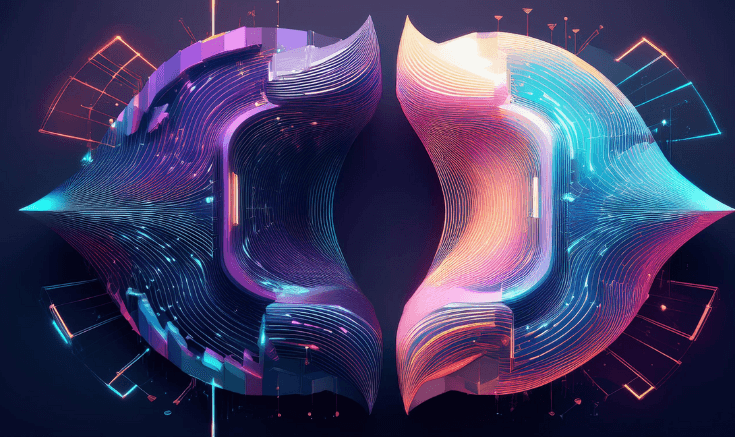
The main difference between natural language processing (NLP) and generative AI is their objectives and capabilities. NLP focuses on processing and understanding human language, making it essential for interpreting existing data and improving communication workflows. Generative AI, on the other hand, emphasizes the creation of new content, such as text, images, or audio, by analyzing patterns in data. Businesses often use these technologies to streamline operations, increase productivity, and unveil new growth opportunities. Understanding their differences is critical to selecting the right solution for specific needs.
Aspect | Natural language processing (NLP) | Generative AI (genAI) |
---|---|---|
Objective | Understand, analyze, and process human language. | Generate new content, such as text, images, or code. |
Functionality | Performs tasks like text classification, translation, and sentiment analysis. | Creates human-like outputs by synthesizing learned patterns. |
Core technologies | Relies on parsing, tokenization, semantic analysis, and machine learning models. | Utilizes advanced architectures, like transformers and GANs. |
Application scope | Optimizes workflows involving language understanding, such as chatbots or summarization. | Automates content creation and supports innovation, such as generating creative assets or simulations. |
Output | Processes and refines existing data. | Produces original, human-like outputs. |
Although NLP and generative AI serve different purposes, they complement each other effectively in many scenarios. Businesses aiming to scale operations, enhance customer engagement, or reduce time-to-market can benefit from combining these technologies. NLP interprets and organizes data, while generative AI builds on this foundation to deliver creative and scalable solutions. Integrating both can result in measurable improvements across cost efficiency, speed, and strategic outcomes.
How NLP and generative AI work together
When natural language processing and generative AI are combined, they create robust solutions that enhance productivity, creativity, and efficiency across industries. These technologies complement one another by using their strengths—NLP’s ability to understand and analyze language and generative AI’s capacity to produce original content. They provide organizations with tools to solve complex challenges and unlock new business opportunities.
- Enhanced customer support: NLP processes customer inquiries to identify intent, while generative AI crafts personalized, human-like responses. This combination improves response quality and reduces wait times.
- Content generation and refinement: NLP extracts key insights from data, and generative AI creates summaries, articles, or reports tailored to specific needs. This approach increases scalability for content production.
- Intelligent chatbots and assistants: NLP interprets user queries, while generative AI formulates conversational and contextually accurate replies. This makes virtual assistants more engaging and effective in delivering information.
- Language translation and localization: NLP identifies nuances in language, and generative AI produces more accurate and culturally relevant translations. This enables businesses to reach global audiences with minimal effort.
- Automated document processing: NLP extracts critical data from unstructured documents, and generative AI converts the extracted data into usable formats, such as summaries, templates, or structured datasets.
Integrating these technologies streamlines workflows and reduces reliance on manual processes, allowing teams to focus on higher-value tasks. The synergy between NLP and generative AI empowers businesses to scale operations, accelerate time to value, and create future-proof strategies that adapt to updated customer expectations.
Industry-specific applications: NLP vs generative AI
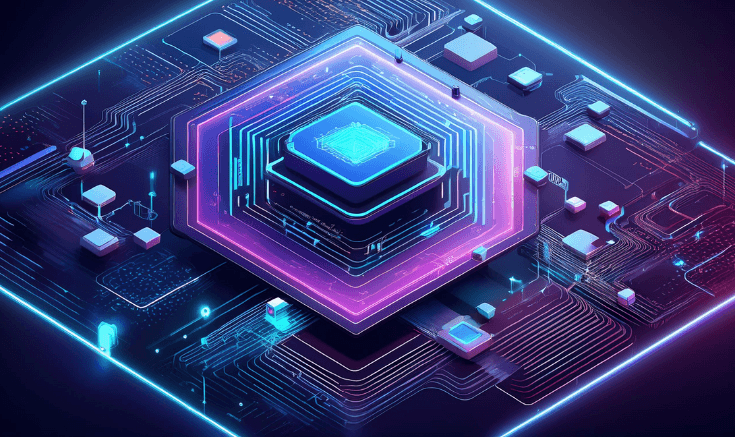
Natural language processing and generative AI have found diverse applications across industries, each delivering tailored solutions to improve efficiency and drive measurable outcomes. While NLP focuses on processing and interpreting language, generative AI excels at creating new content. They address unique challenges and unlock opportunities for growth in various sectors. Below are key industry-specific use cases:
Retail
Retailers use NLP to analyze customer feedback, process reviews, and enhance product recommendations. Sentiment analysis helps businesses understand customer preferences and trends, allowing more targeted marketing campaigns. Generative AI complements this by creating personalized content, such as promotional emails or product descriptions, tailored to individual shoppers. Together, these technologies improve customer engagement and streamline operational efficiency.
Financial services
In financial services, NLP processes large volumes of unstructured data, such as legal documents, customer queries, and transaction records, to identify patterns and extract actionable insights. Fraud detection systems rely on NLP to flag unusual behavior. Generative AI adds value by automating report generation, simulating market scenarios, and personalizing client communications, helping firms save time and resources while maintaining regulatory compliance.
Healthcare
Healthcare providers use NLP to extract relevant information from patient records, medical literature, and diagnostic reports. This reduces manual data entry and helps identify critical trends in patient care. Generative AI aids in creating patient summaries, drafting discharge notes, and simulating treatment outcomes. These technologies enhance clinical workflows and improve patient outcomes by providing timely, actionable information.
Logistics
Logistics companies leverage NLP for route optimization, shipment tracking, and analyzing supply chain data. Chatbots powered by NLP assist customers with shipping inquiries and delivery updates. Generative AI contributes by forecasting demand, generating dynamic pricing strategies, and creating reports for supply chain planning. This combination reduces delays and improves operational transparency across logistics networks.
Media and entertainment
In media and entertainment, NLP analyzes audience feedback, categorizes content, and allows voice recognition systems. Generative AI enhances this by creating personalized recommendations, automating scriptwriting, or generating immersive visuals for gaming and films. These technologies permit companies to deliver tailored content faster, improving audience retention and driving creativity at scale.
Businesses across these industries are discovering how NLP and generative AI can complement each other to address sector-specific challenges. Their combined capabilities help organizations improve operational efficiency, enhance customer satisfaction, and develop strategies that scale with future needs.
"NLP processes and refines existing data, while generative AI produces original, human-like outputs."
Choosing between NLP vs generative AI
Determining whether to prioritize natural language processing (NLP) or generative AI depends on the specific business objectives and challenges. Each technology serves unique purposes, and selecting the right approach requires understanding their strengths and aligning them with measurable outcomes.
NLP is ideal for businesses that analyze existing data, improve communication processes, or extract insights from unstructured text. For instance, companies handling large volumes of customer feedback, legal documentation, or email communications often benefit from NLP tools. These solutions can quickly process and organize language-based data, improving accuracy and saving time.
Generative AI is better suited for tasks involving the creation of new content. Businesses that need to produce personalized marketing materials, draft reports, or simulate creative outputs will find generative AI valuable. This technology allows organizations to scale content production without increasing resource costs, making it particularly useful for marketing, design, or R&D functions.
In many cases, the most effective strategy is a combination of both. NLP can process and analyze data to identify patterns or gaps, while generative AI builds on this foundation to produce content that adds value. For example, combining these technologies can create advanced chatbots that interpret user input and generate highly accurate responses or produce automated reports based on structured data analysis.
Evaluating business goals, resource availability, and desired outcomes will guide the decision on implementing NLP, generative AI, or both. Choosing the right approach can reduce operational costs, improve efficiency, and unlock growth opportunities that align with your organization’s long-term strategy.
Successful strategies for implementing NLP and generative AI
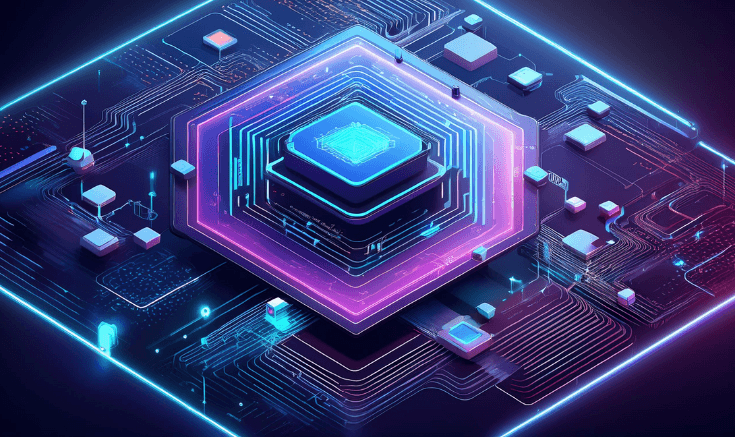
- Start with clear objectives: Identify specific goals, such as reducing manual processes, improving customer engagement, or accelerating content creation. Defining measurable outcomes ensures the implementation aligns with business priorities and delivers value quickly.
- Evaluate use cases: Assess where NLP, generative AI, or combining both can create the most significant impact. Use cases like customer service automation, document processing, or personalized marketing provide high-value opportunities for adoption.
- Invest in high-quality data: Both technologies rely on clean, well-structured datasets for training and operation. Ensure your organization collects and curates reliable data to improve the accuracy and performance of these solutions.
- Prioritize stakeholder alignment: Gain buy-in from leadership, IT teams, and end-users by communicating the benefits of NLP and generative AI. Educating teams about the value of these tools can reduce resistance and foster collaboration.
- Adopt modular solutions: Opt for tools and platforms that integrate with existing systems without disrupting workflows. Modular and scalable solutions allow for phased implementation, reducing upfront costs and risks.
- Monitor and optimize performance: Regularly measure the outcomes of your NLP and generative AI applications to track their impact. Key performance indicators like processing speed, cost reduction, or customer satisfaction can help refine strategies and guide further improvements.
- Plan for governance and ethics: Establish guidelines for ethical AI usage to mitigate risks such as data privacy breaches or algorithm biases. Transparent policies build trust and support long-term adoption.
With these strategies, organizations implementing NLP and generative AI can streamline operations, improve time to value, and maximize returns on investment. Combining strong governance, measurable goals, and continuous optimization ensures these technologies become integral tools for sustained business growth.
Natural language processing and generative AI offer more than just technical solutions—they are catalysts for operational efficiency, innovation, and measurable business value. By integrating these advanced technologies into your workflows, your organization can unlock untapped potential and drive strategic success. At Lumenalta, we specialize in crafting tailored AI solutions that align with your goals, ensuring your business is equipped to succeed. Let’s chart a brighter path forward together.
Table of contents
- What is natural language processing (NLP)?
- What is generative AI?
- Key differences between NLP and generative AI
- How NLP and generative AI work together
- Industry-specific applications: NLP vs generative AI
- Choosing between NLP vs generative AI
- Successful strategies for implementing NLP and generative AI
- Common questions about NLP vs generative AI
Common questions about NLP vs generative AI
What is the main difference between NLP and generative AI?
How do businesses use NLP in day-to-day operations?
What are some practical uses for generative AI?
Can NLP and generative AI work together?
Is it expensive to implement NLP or generative AI?
Want to learn how NLP can bring more transparency and trust to your operations?