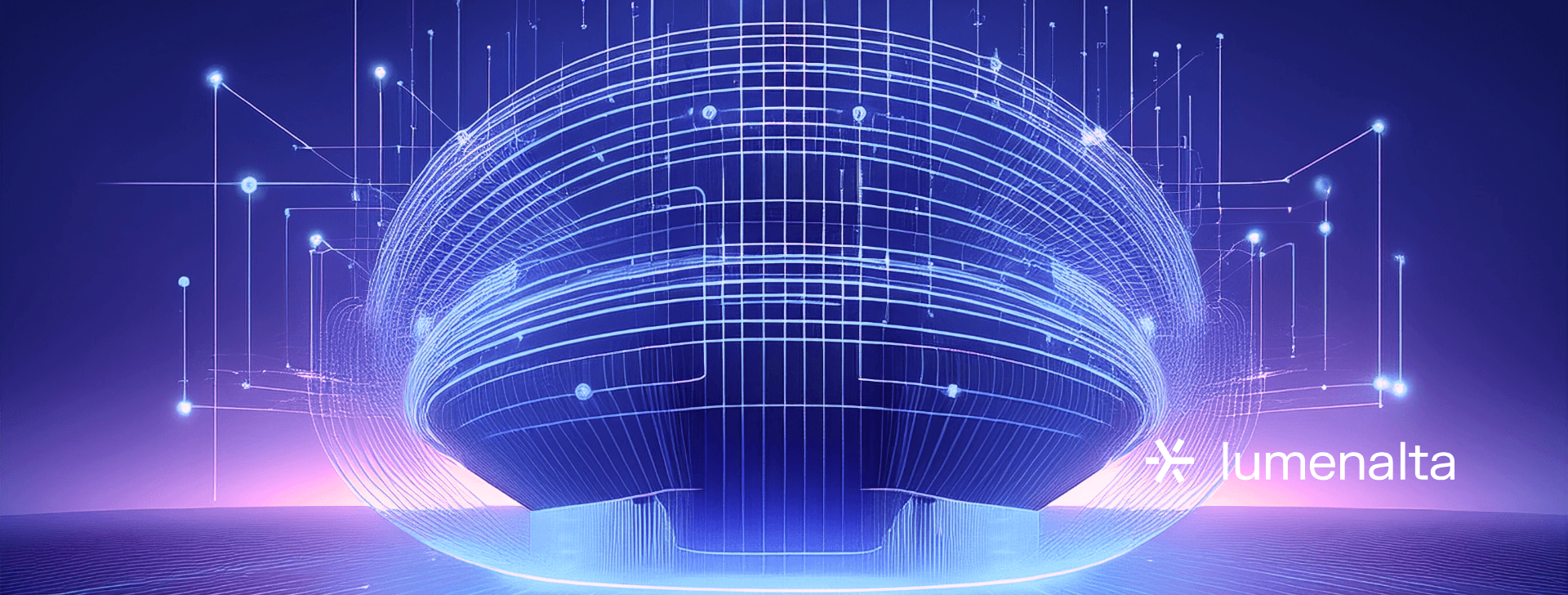
A guide to AI in telecom in 2025
APR. 3, 2025
5 Min Read
AI in telecom ensures that communication services run smoothly, efficiently, and with minimal downtime.
Many organizations recognize that advanced analytics and automated workflows are no longer optional for handling large-scale data and subscriber demands. Providers must balance cost management and innovation while catering to an ever-growing user base. Systems powered by artificial intelligence streamline everything from network operations to customer interactions, paving the way for higher returns and better experiences. Innovation in telecom relies on extracting value from data at every step of the process. Strategies built on transparency and collaboration allow stakeholders to overcome hurdles such as integration costs, skill shortages, and security complexities. Machine learning, generative models, and conversational AI solutions each contribute distinct capabilities to accelerate business objectives.
key-takeaways
- 1. AI in telecom aligns large-scale data processing with actionable insights to optimize services.
- 2. Collaborative strategies and vendor partnerships increase the success rate of AI deployments.
- 3. Conversational AI in telecom elevates customer experience through automated support and personalized interactions.
- 4. Generative models expand service possibilities with creative output, providing dynamic caller experiences and multilingual assistance.
- 5. ROI hinges on factors such as operational savings, subscriber retention, and measurable improvements to resource allocation.
What is AI in telecom?
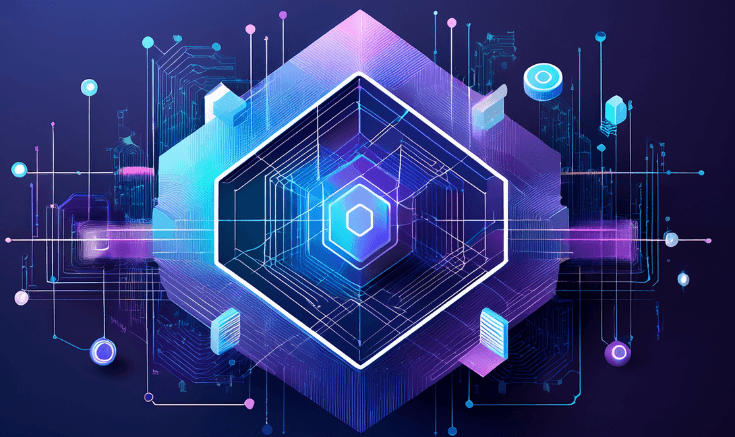
AI in telecom refers to advanced algorithms and data-backed techniques that empower communication service providers to analyze, optimize, and predict operational outcomes. This technology supports a wide range of solutions, from personalized user experiences to automated network management. Many organizations view these systems as a way to streamline processes and scale services more efficiently. Implementation often involves data gathering, real-time analytics, and ongoing improvements guided by historical trends.
These solutions have become a vital resource for shaping how voice, data, and multimedia services are delivered. Providers seek to incorporate AI in telecom to detect anomalies, refine resource usage, and expand connectivity options. Transparent governance and robust infrastructure remain key to unlocking the full potential of artificial intelligence in telecom. Strategic planning and alignment with business goals help ensure consistent outcomes for the entire value chain.
“These solutions have become a vital resource for shaping how voice, data, and multimedia services are delivered.”
Key applications of AI in telecom
Many providers rely on AI for predictive maintenance, customer service enhancements, and real-time analytics. Data is sourced from network activity, user interactions, and enterprise-level trends, allowing providers to spot inefficiencies and act promptly. This capability reduces downtime, optimizes resource allocation, and promotes stable service performance.
Stakeholders often look for streamlined ways to implement AI in telecom industry operations, from fraud detection to automated billing. Machine learning helps identify patterns in voice data, SMS usage, and large-scale bandwidth consumption. Teams can then adapt strategies to reduce operational costs and prevent revenue loss. This process ultimately makes the sector more resilient and cost-effective.
Predictive network management
Forecasting equipment failures before they occur is an important step toward operational resilience. Predictive systems track the performance of hardware components, comparing historical data to real-time metrics for early warning signals. This approach decreases expenses on emergency repairs, ensuring more stable service for end users. Teams also gain a proactive edge in resource planning, preventing unnecessary outages and improving user satisfaction.
Virtual assistants for customer service
Conversational AI in telecom has made it easier to address customer inquiries without lengthy wait times. Virtual assistants handle tasks like account balances, troubleshooting, and service upgrades, freeing support staff for more complex inquiries. Greater scalability becomes possible because these solutions can process large volumes of requests in parallel. Constant analysis of interactions also uncovers new opportunities to refine user experiences and guide operational decisions.
Automated security monitoring
Attack detection benefits significantly from artificial intelligence in telecom sector solutions that scan for suspicious activity. Pattern recognition algorithms distinguish genuine data traffic from potential threats, enabling immediate isolation of compromised endpoints. This reduction in manual oversight allows teams to focus on strategic responses. Threat intelligence is then updated as new attack vectors appear, reinforcing network defenses across multiple layers.
Benefits of implementing AI in telecom
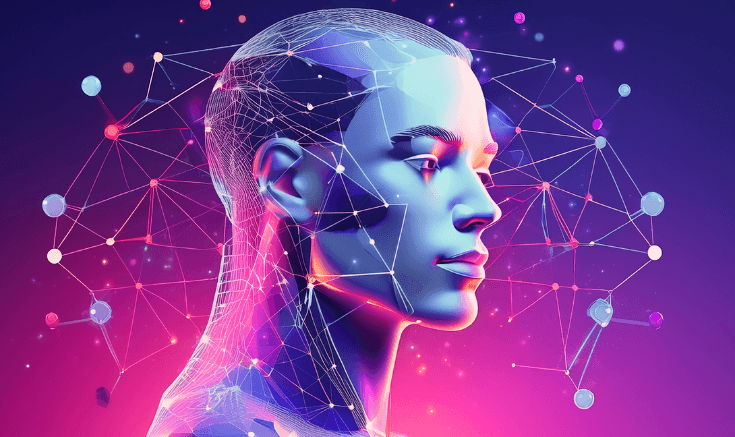
Organizations exploring AI in telecom often prioritize measurable improvements in areas like cost efficiency, service reliability, and subscriber satisfaction. Many solutions offer proactive monitoring, automated workflows, and data analytics that guide better resource allocation. Decision-makers appreciate how these benefits translate into higher profitability and faster time to market. Results also resonate with leadership teams seeking ways to expand service portfolios without significant overhead.
- Enhanced service quality: These tools anticipate demand surges, ensuring that resources are allocated efficiently and downtime is minimized.
- Reduced operational costs: Automated workflows lower manual tasks, freeing skilled staff to focus on strategic planning and innovative revenue streams.
- Targeted marketing campaigns: Intelligent segmentation reveals subscriber preferences, enabling personalized offers and stronger customer loyalty.
- Accelerated fault resolution: AI-backed diagnostics provide immediate fault identification, cutting down on frustrating delays for both providers and users.
- Data-backed strategic insights: Advanced analytics reveal long-term usage trends, guiding leadership teams to make informed expansions or improvements.
- Scalable revenue generation: AI-based platforms accommodate market growth with minimal infrastructure additions, promoting cost-effective scalability.
These benefits highlight how artificial intelligence in the telecom industry workflows can align with key performance goals. Stakeholders often see results both in the short term, through reduced costs, and in long-term strategies for expansion. Identifying such advantages makes it easier to justify investments in AI and ML in telecom. Teams can then proceed with greater clarity and set realistic benchmarks for success.
AI vs ML in telecom
The main difference between AI and ML is that AI covers a broader set of technologies aimed at simulating human-like intelligence, whereas ML focuses specifically on learning patterns from data. AI can incorporate vision recognition, language processing, and advanced reasoning, while machine learning remains the engine that drives predictive analytics. Both approaches underpin key innovations in telecom, including automated billing and user experience improvements. Teams often utilize both, but each discipline has distinct value propositions.
Machine learning relies on defined algorithms and historical data sets to refine outputs over time, but AI in telecom sector solutions might also handle more comprehensive tasks. New developments in hardware acceleration and cloud platforms have further expanded these possibilities, making it simpler to scale. The key is determining the right level of automation for any given function, ensuring reliability and cost-efficiency. This balance protects organizational priorities while embracing new capabilities.
Conversational AI's role in enhancing customer interactions
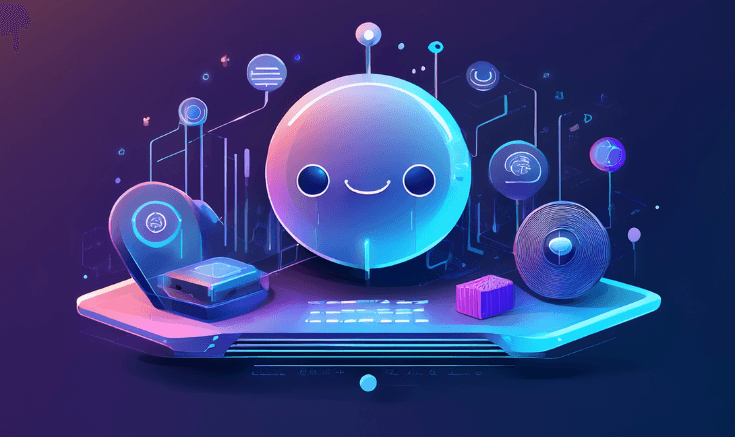
Conversational AI in telecom has significantly improved how providers connect with users through chatbots and voice assistants. Automated platforms engage individuals in real time, collecting queries and offering solutions without the need for human intervention. Companies notice greater subscriber retention because routine issues are handled swiftly. Direct feedback from these interactions informs product design and helps refine long-term service plans.
Some solutions integrate sentiment analysis to assess user satisfaction beyond simple problem resolution. This real-time data collection also supports rapid adaptation when seasonal promotions or feature rollouts are in effect. The combination of convenience and personalization forms a more meaningful customer experience. Loyalty programs become more relevant, guided by insights drawn from daily conversations.
Challenges in adopting AI within the telecom sector
Adoption of artificial intelligence in telecom sector settings does not come without obstacles. Many organizations struggle with data quality issues, skill shortages, or limited technology budgets. Resistance to operational change also emerges when teams are used to manual tasks. Achieving a viable roadmap requires addressing these concerns systematically and demonstrating tangible benefits early on.
- Skill gaps: Team members may need extensive training in data science, requiring targeted learning pathways to build confidence.
- Data governance: Poorly structured or incomplete data sets create inaccuracies and limit the effectiveness of AI-based insights.
- Integration complexity: Merging legacy systems with new AI frameworks can involve significant technical effort and project oversight.
- Security worries: Heightened data privacy regulations demand robust compliance measures and sophisticated cyber defense practices.
- Financial constraints: Investments in specialized hardware, software, and talent must align with measurable returns to secure stakeholder buy-in.
These hurdles become more approachable when leaders set clear expectations and standardize processes. Real-time tracking of progress helps illustrate the positive effects of AI implementations across different departments. Strong partnerships with vendors and consultants also mitigate risk. Proactive communication fosters greater alignment and trust in emerging tools.
The impact of generative AI on telecom services
Gen AI in telecom offers new ways to customize subscriber content, develop targeted marketing initiatives, and craft interactive features. Some organizations use generative solutions to create dynamic caller experiences or to manage large-scale multilingual support. These capabilities often depend on advanced models that can produce human-like text, audio, or images based on learned patterns. Creative elements such as chat-based promotional campaigns can stand out in a crowded marketplace.
Significant attention goes toward safeguarding this technology from misuse, including unauthorized content generation or malicious impersonation. Teams integrate ethical guidelines and monitoring protocols to preserve trust among subscribers and partners. Greater synergy with existing AI in telecom solutions becomes possible by pairing generative outputs with predictive analytics, allowing more relevant service rollouts. Each initiative must align with budget considerations and internal skill sets for the best outcomes.
Strategies for successfully integrating AI solutions in telecom
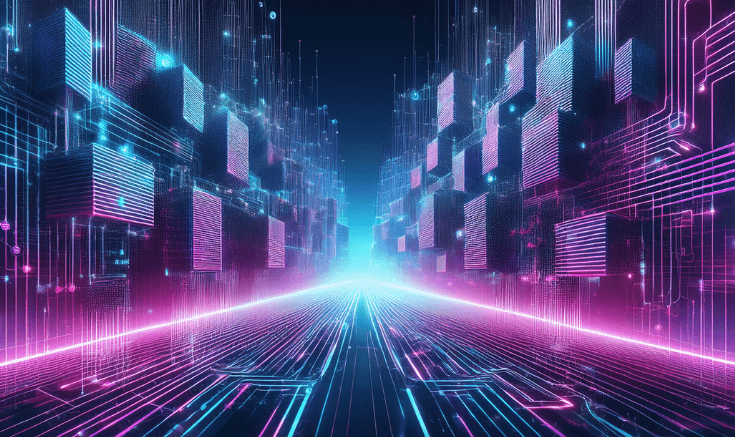
Organizations often seek a roadmap that aligns technical possibilities with clear business objectives. AI in telecom requires careful orchestration of data pipelines, workforce training, and vendor relationships. Balancing these elements ensures that outcomes serve both operational teams and executive stakeholders. Precise planning reduces friction as new processes become part of daily routines.
Leaders typically focus on incremental rollouts, starting with pilot projects that validate feasibility and ROI. This approach paves the way for broader deployments while maintaining manageable costs. Structured governance processes guide each phase, from data collection to final system integration. Clear success metrics keep teams on track and generate buy-in from key decision-makers.
Assessing infrastructure readiness
Technical evaluations set the stage for effective AI in telecom industry deployments by examining existing servers, cloud resources, and network capabilities. Sufficient processing power and robust security protocols must be available before launching any advanced analytics project. Periodic reviews of capacity allow teams to scale resources as business requirements expand. Consistent documentation ensures that every hardware and software component remains up to date.
Building internal expertise
Workforce development is a critical factor in achieving full value from AI solutions. Training programs covering data analysis, modeling techniques, and operations best practices prepare employees to handle complex workflows. Partnerships with educational institutions or specialized training providers can fill knowledge gaps and create a strong talent pipeline. Motivated teams adapt more quickly, accelerating results for stakeholders.
Managing vendor partnerships
Collaboration with external experts may be essential for specialized projects or when in-house capacity is limited. Vendors can assist with platform setup, data integration, and ongoing maintenance, reducing the burden on internal teams. Contract terms should clearly define scope, deliverables, and support options to avoid misaligned expectations. Good communication practices help maintain transparency and guide continuous improvement efforts.
“Structured governance processes guide each phase, from data collection to final system integration.”
Measuring ROI of AI in telecom
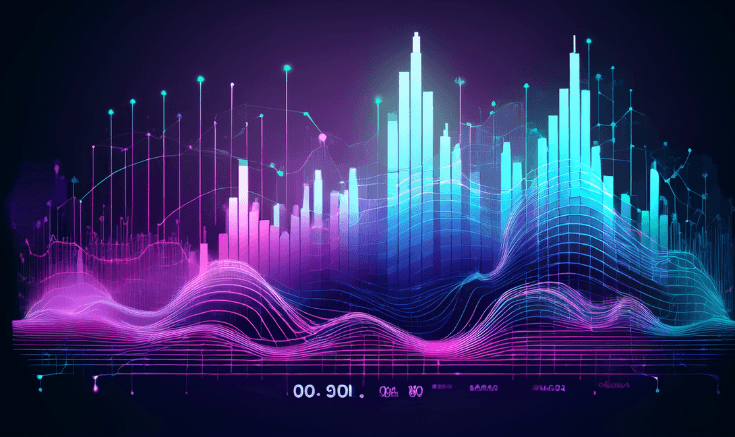
Return on investment (ROI) evaluations are shaped by multiple factors, including operational efficiency, revenue growth, and customer satisfaction metrics. Some organizations rely on key performance indicators such as average handling time in call centers, volume of resolved tickets, or upsell conversion rates. Tracking these metrics over defined periods reveals how well an AI initiative meets financial and strategic targets. Consistent measurement also permits teams to refine algorithms and improve resource allocation.
Methods for quantifying ROI vary based on organizational maturity and available data. Some rely on cost-benefit analyses that compare capital expenses with the net gains from AI-powered optimizations. Others track intangible values like employee morale and brand perception, translating these into more tangible outcomes over time. An agile approach ensures that adjustments can be made promptly if certain metrics do not meet expectations.
AI in telecom is not just a technology upgrade—it’s a path to more efficient and sustainable operations. Offering new ways to personalize and scale services, these systems free organizations to focus on delivering tangible business outcomes. At Lumenalta, we specialize in designing tailored AI solutions that align with your business goals, ensuring you are positioned to lead. Let’s chart a brighter path together.
table-of-contents
- What is AI in telecom?
- Key applications of AI in telecom
- Benefits of implementing AI in telecom
- AI vs ML in telecom
- Conversational AI's role in enhancing customer interactions
- Challenges in adopting AI within the telecom sector
- The impact of generative AI on telecom services
- Strategies for successfully integrating AI solutions in telecom
- Measuring ROI of AI in telecom
- Common questions about AI in telecom
Common questions about AI in telecom
How does AI improve real-time network performance?
What is the difference between AI and ML in the telecom industry?
Why is conversational AI in telecom a strategic priority?
How can gen AI in telecom impact marketing?
What role does security play in artificial intelligence in telecom?
Want to learn how artificial intelligence can bring more transparency and trust to your operations?