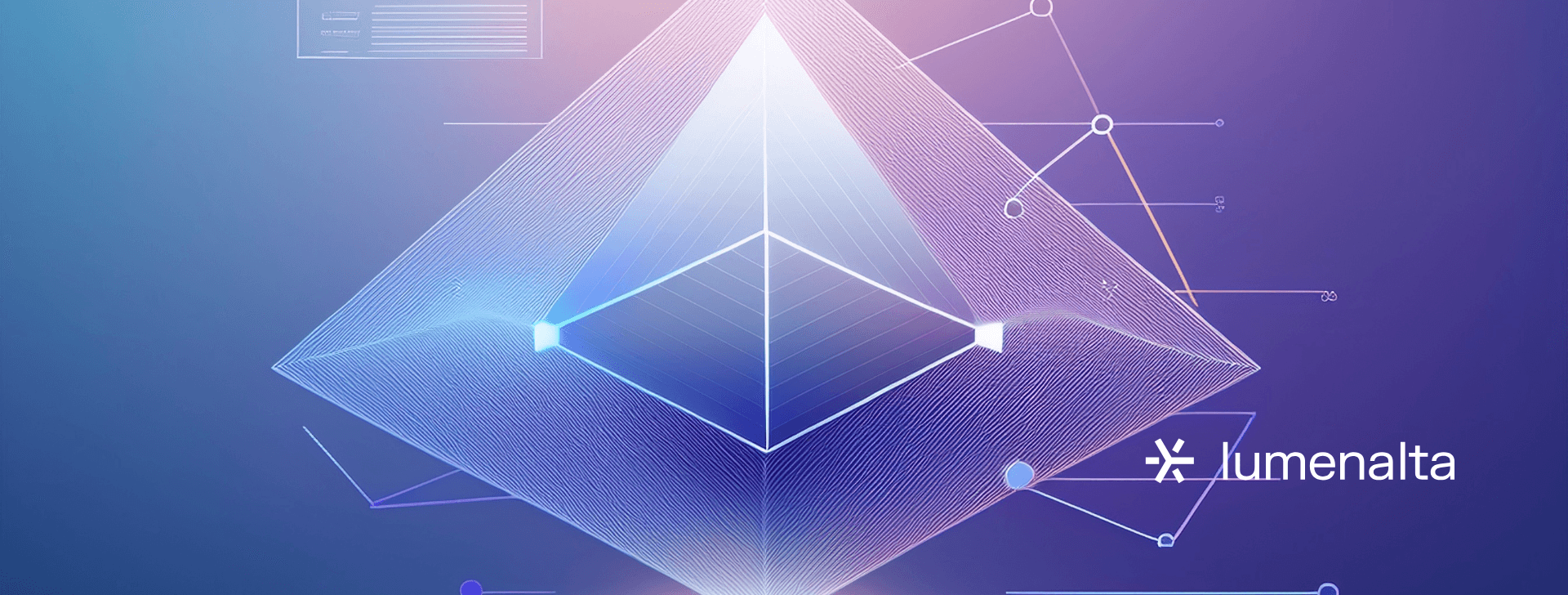
5 ways AI in product development drives efficiency
MAR. 30, 2025
5 Min Read
AI in product development delivers accurate forecasts and precise resource allocation that accelerate time to market and boost measurable efficiency.
As teams face increasing pressure to reduce costs while scaling innovation, AI offers a direct path to smarter decisions backed by real-time data. From refining early-stage concepts to streamlining final production, AI solutions eliminate guesswork and reduce rework across every phase. The result is a faster, more reliable development process aligning with business goals and user expectations.
key-takeaways
- 1. Predictive analytics reveals risks and opportunities early, driving precise decisions.
- 2. Automated testing shortens QA cycles while preserving high standards.
- 3. Data-backed insights reveal growth opportunities that propel revenue.
- 4. Intelligent resource allocation reduces spending and shortens production timelines.
- 5. AI in food product development, as well as other sectors, unlocks agile approaches that cater to market needs.
Understanding AI in product development
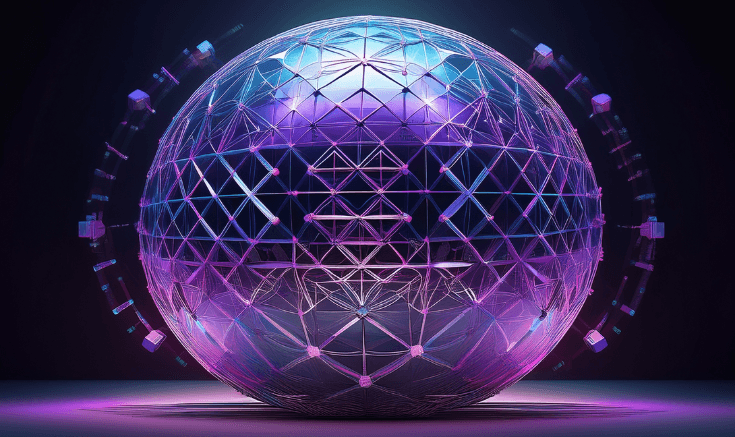
Artificial intelligence brings valuable speed and accuracy to the entire product life cycle. Machine learning applies data-backed insights that quickly identify patterns and forecast outcomes, reducing guesswork during key planning stages. Teams gain a more precise view of potential market acceptance, performance bottlenecks, and ideal resource allocation. Each of these benefits helps you shorten development timelines while improving product viability.
Organizations across industries consider this approach an important tool for scaling up ideas without overspending. Insights derived from neural networks, natural language processing, and other forms of machine learning help clarify each step, from design ideation to production optimization. Teams can focus on iterative improvements, resulting in steady progress without wasting time on avoidable errors. This structured method supports cross-functional collaboration, making AI in product development an engine for long-term gains.
Many decision-makers also value its potential for creating measurable business impact. This includes boosting returns for investors, aligning products with user needs, and improving consistency in real time. Data analytics solutions powered by machine learning reinforce trust among stakeholders, showcasing evidence-based rationale for decisions. That level of transparency often accelerates momentum, as everyone can see how each choice contributes to the final result.
"Machine learning applies data-centric insights that quickly identify patterns and forecast outcomes, reducing guesswork during key planning stages.”
5 ways AI in product development drives efficiency
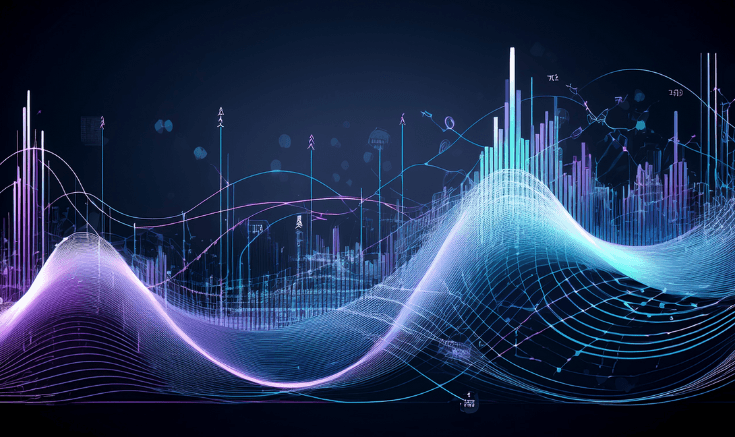
1. Predictive analytics refines product specifications
Real-time predictions help teams refine initial specs before significant resources are spent. Detailed insights about user preferences, market trends, and potential cost fluctuations inform design priorities, preventing expensive redesigns once production is already in motion. This process ensures that AI in product development focuses on relevant features, balancing quality with speed to market. Early detection of potential issues means teams maintain control over budgets and timelines.
Teams ultimately find measurable gains as they reduce rework and maintain consistency. These accurate forecasts also drive alignment among designers, engineers, and marketing professionals, leading to strategic consensus. That level of shared clarity translates into faster production cycles and balanced resource allocation. When data shape product specifications, new offerings fit customer demands more closely, boosting profitability.
2. Rapid prototyping streamlines time to market
Machine learning tools automate model creation and simulation, generating multiple prototypes in far less time than manual approaches. Engineers often rely on these results to identify flaws and potential improvements at each stage, which reduces development overhead. Quick prototype validation gets products to market sooner, minimizing the window where competitors might capture consumer attention first. This process lets leadership confirm which prototypes offer the strongest commercial potential.
Teams benefit from cost savings and improved scalability as well. Continuous testing produces actionable insights on everything from materials choices to user interactions. This iterative feedback loop means final designs reach a refined state faster than relying solely on traditional trial-and-error. Rapid prototyping facilitated by AI in product development ultimately preserves momentum and puts you in a stronger position to meet shifting consumer needs.
3. Automated testing enhances quality assurance
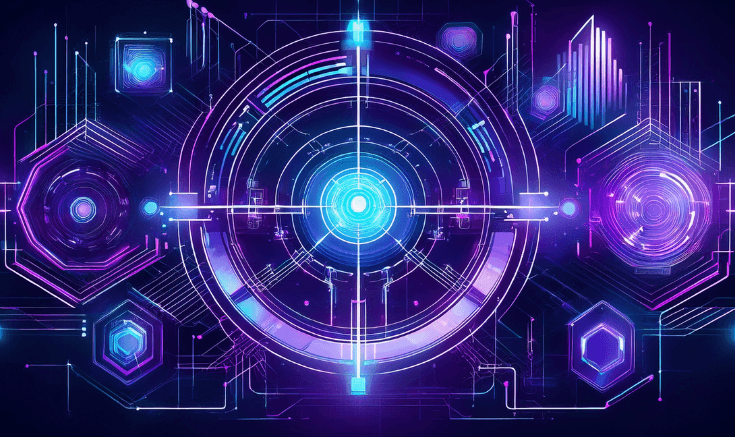
Quality assurance (QA) can demand extensive hours and resources when handled manually. AI solutions identify software and hardware inconsistencies more efficiently, checking for vulnerabilities and functional gaps. Thorough automated testing reveals critical risks that might undermine product performance, allowing teams to address them right away. This approach adds an extra layer of reliability that protects customer satisfaction.
Product teams see a noticeable boost in confidence and speed after introducing automated QA steps. That improvement often translates into fewer post-release patches, saving both time and money. Engineers can then devote energy to strategic tasks rather than hunting for elusive bugs. Constant validation ensures consistent performance under varied conditions, helping your projects maintain a solid reputation for reliability.
4. Data-based innovation promotes scalability
Precise analytics offer a deeper view into how products perform under different scenarios. This helps you determine which features are truly essential, which can be retired, and which justify additional investment. Evidence-based decisions reduce guesswork and encourage smarter project expansion, especially when exploring AI in new product development. Scaling becomes less of a risk because each decision is guided by real data, lowering the chance of expensive missteps.
Teams often realize new revenue streams by identifying areas for pivot or optimization. Automated data analysis reveals untapped opportunities, such as overlooked consumer segments or niche product improvements. These findings generate tangible value, allowing you to target evolving preferences before they become widespread. Data-based methods also foster better governance since transparent metrics for all stakeholders support decisions.
5. Intelligent resource allocation reduces costs
Resource misalignment can stall progress and inflate expenses, especially for complex initiatives. Advanced algorithms assign the right tasks to the right teams and predict which materials, timeline, and budget will best support each phase. Managers maintain a holistic view of progress and cost, ensuring alignment with strategic objectives. When resources are placed precisely where they are needed, the project avoids major delays or costly scope expansions.
Better resource usage drives measurable impact. Engineers focus on core tasks rather than administrative chores, and inventory managers only order what they truly need. This efficiency eliminates rework and frees up capital for other projects, accelerating the next wave of innovation. Stakeholders also see that cost management is a priority, which fosters trust and clarity around the product pipeline.
“Thorough automated testing reveals critical risks that might undermine product performance, allowing teams to address them right away.”
Challenges of AI in product development
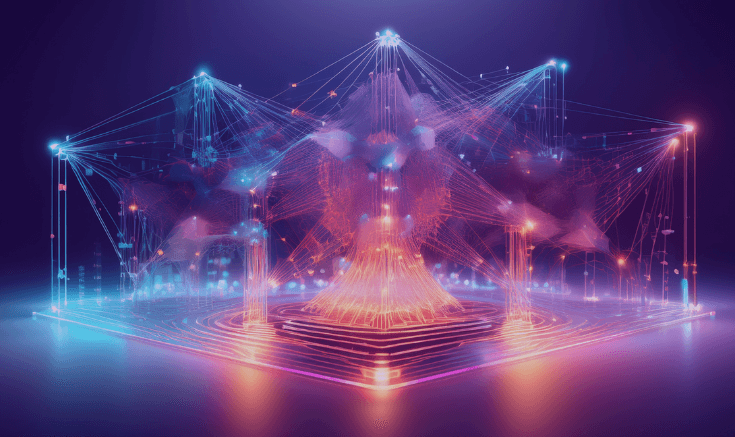
Introducing machine learning creates a variety of hurdles related to technical complexity and organizational acceptance. These difficulties can disrupt established processes or require considerable time to address. Each challenge might affect your ability to integrate AI in product development at scale, making it vital to anticipate and mitigate them. Effective planning reduces potential obstacles and helps you maintain forward momentum.
- Data quality issues: Flawed or fragmented data sources can lead to inaccurate models and flawed results.
- Organizational pushback: Teams may resist adopting new workflows due to fear of change or uncertainty about job roles.
- Skills shortage: Finding specialists who can optimize machine learning strategies and maintain infrastructures is sometimes a struggle.
- Model biases: Algorithms reflect any biases in the training data, potentially causing skewed predictions or recommendations.
- Security concerns: Sensitive corporate data might need extra safeguards, demanding resources for encryption and robust governance.
Careful orchestration across departments often mitigates these risks. Leaders monitor potential trouble spots while technical experts verify data hygiene and identify vulnerabilities. Transparent communications build trust, ensuring each team member understands why these solutions matter and how their role can adapt. That balanced approach prevents confusion and sets the foundation for beneficial outcomes.
Implementing AI in new product development
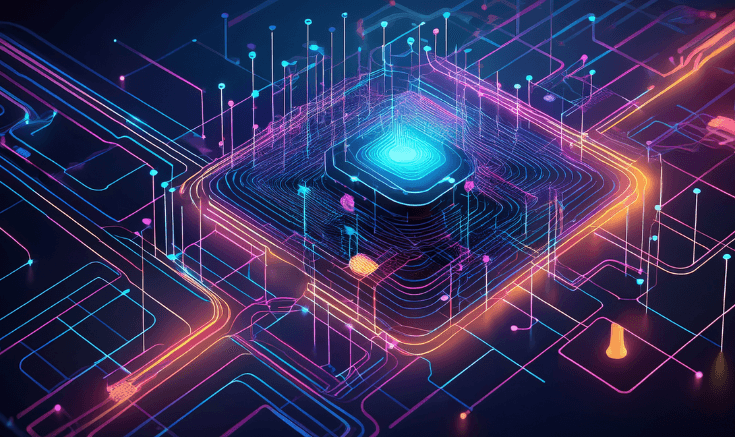
Establishing clear objectives is often the first step toward meaningful adoption. Clear goals ensure each implementation targets the right pain points, whether it involves automating design tasks, improving supply chains, or refining predictive demand analysis. Objectives often revolve around faster speed to market, cost efficiency, and opportunities to capture more revenue. These priorities shape the data collection, algorithm selection, and solution deployment.
Organizations often spot unique applications when exploring AI in new product development. Timely consumer feedback, real-time performance metrics, and internal operational insights all feed into machine learning systems that refine decisions across multiple teams. This strategy also extends to AI in food product development, where recipe creation, nutrition data analysis, and shelf-life forecasting become more systematic. That level of precision keeps production on budget, addresses health regulations, and aligns products with consumer tastes.
Clear cross-functional ownership leads to better outcomes. Engineers develop models that align with marketing requirements for user experience, and production managers customize logistics to handle variable demand. Collaborative governance structures also encourage stakeholders to keep an open line of communication, reducing misunderstanding and reinforcing shared success. This consistent alignment builds confidence among decision-makers and fosters practical momentum for continued growth.
AI in product development is a direct route to faster time to value, scalable processes, and tangible returns. Concentrating on advanced analytics, automation, and strategic insights, these solutions simplify the journey from concept to completion. At Lumenalta, our approach centers on client-based solutions that help you meet emerging demands while maintaining control over operational goals. Let’s chart a brighter path together.
table-of-contents
- Understanding AI in product development
- 5 ways AI in product development drives efficiency
- 1. Predictive analytics refines product specifications
- 2. Rapid prototyping streamlines time to market
- 3. Automated testing enhances quality assurance
- 4. Data-based innovation promotes scalability
- 5. Intelligent resource allocation reduces costs
- Challenges of AI in product development
- Implementing AI in new product development
- Common questions about AI in product development
Common questions about AI in product development
How does AI in product development influence time to market?
What is the role of AI in new product development?
Can AI improve collaboration within product teams?
What does AI in food product development help with?
How do companies overcome barriers to adopting AI in product development?
Want to learn how artificial intelligence can bring more transparency and trust to your operations?