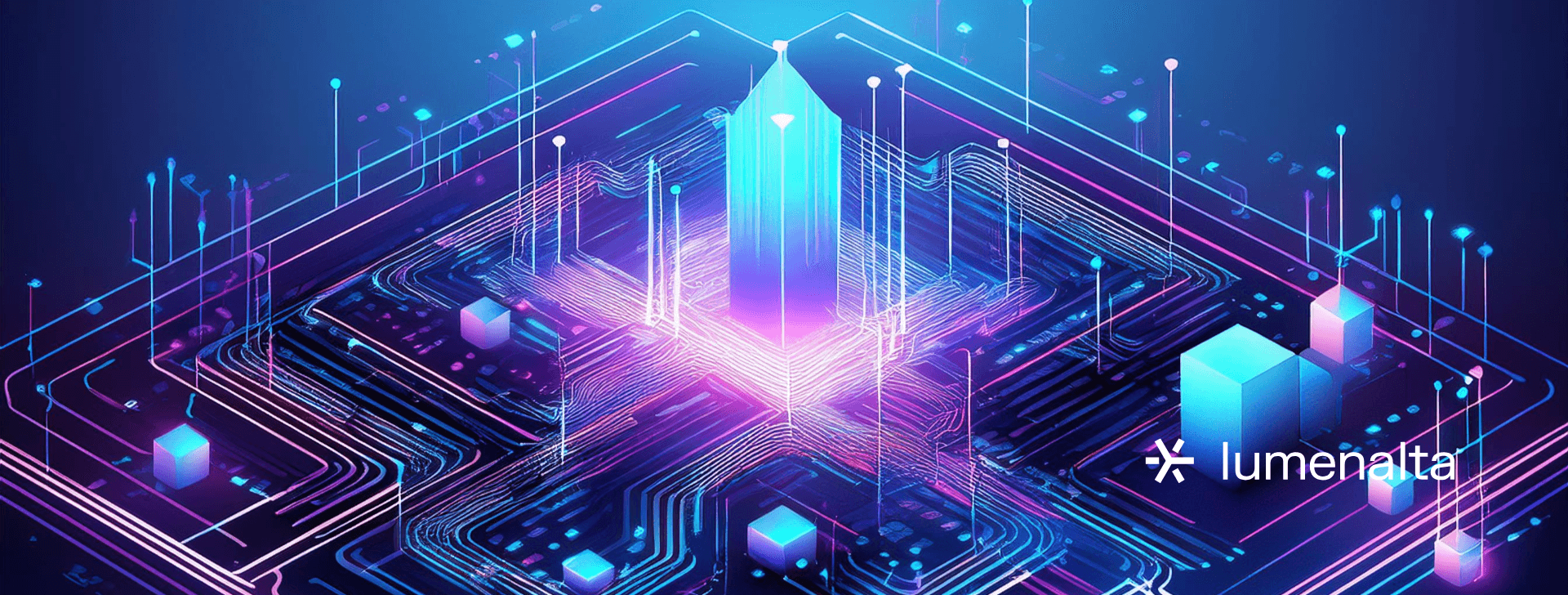
AI readiness checklist (updated 2025)
FEB. 18, 2025
5 Min Read
AI readiness can dramatically boost your organization’s growth potential.
AI has emerged as a powerful accelerator of business efficiency and growth. Organizations that integrate AI-based technologies stand to reduce costs, increase resilience, and unlock new opportunities. Teams still face challenges around data, governance, and talent, especially when aiming to deliver quick wins and tangible ROI. A practical AI readiness checklist bridges these gaps and sets a clear roadmap for success.
Decision channels, workforce skills, and security all come into play when evaluating AI projects. Leaders often ask how to gauge the right moment to invest more deeply and how to manage associated risks. Clear benchmarks, such as pilot results and robust data management, remove guesswork from the process. An effective AI readiness checklist helps create alignment across departments, ensuring every AI initiative targets the outcomes that matter most.
Understanding AI readiness
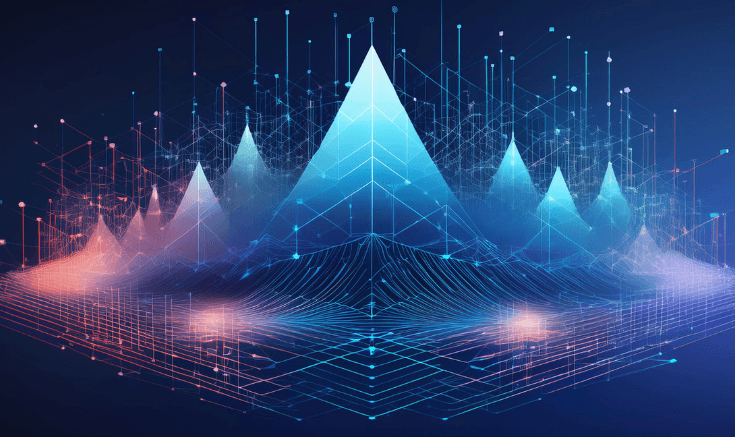
Modern organizations often look to artificial intelligence (AI) to gain efficiency and reveal new possibilities for cost savings. AI readiness refers to a company’s capacity to deploy AI-based processes, machine learning (ML) solutions, and data analytics in a purposeful manner. This concept focuses on strategic alignment, infrastructure, and workforce capabilities. Technical leaders benefit when they understand the existing challenges that might limit effective AI adoption.
Many key leaders evaluate AI readiness as a strategic advantage. Cross-functional teams need consistent processes, data governance, and flexible technology stacks to accommodate ongoing improvements. Trust also plays a central role because stakeholders want assurance that advanced automation will enhance existing services and workflows. A strong AI readiness foundation helps teams expedite time to market and secure meaningful ROI.
Importance of AI readiness in 2025
The year 2025 signals a pivotal moment for organizations seeking data-focused insights and predictive modeling. AI adoption is expanding across finance, healthcare, logistics, and other sectors, leading to fresh business models and improved user experiences. Being prepared involves more than technology alone; it calls for alignment between leadership, operations, and innovation. This combination shapes how quickly solutions are deployed and scaled for long-term success.
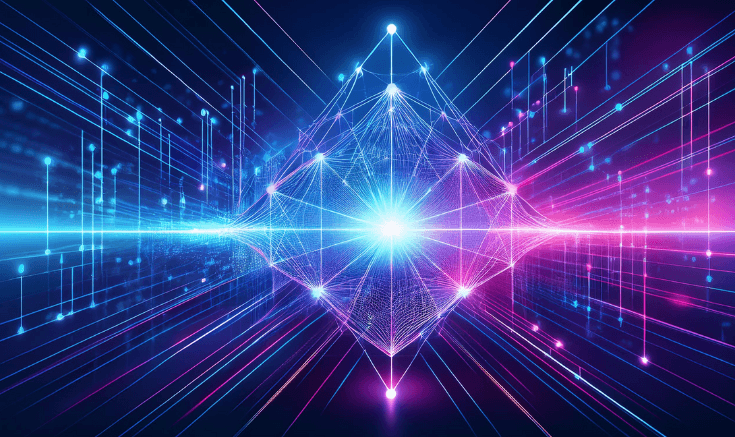
Stakeholders view AI readiness as a catalyst for agile projects, cost optimization, and faster returns for investors. Companies that prioritize readiness often see more efficient resource allocation and stronger momentum around projects. Delays in AI planning can lead to missed revenue opportunities, especially when others in the market are moving toward advanced automation. A forward-looking stance in 2025 positions teams to deliver tangible value through well-orchestrated AI investments.
Key components of AI readiness
Key components of AI readiness guide organizations toward practical strategies and outcomes. Each piece builds on the next to form a cohesive plan that ties together people, systems, and goals. A structured approach reduces guesswork and ensures alignment with larger objectives. Leaders often rely on these components to assess current capabilities, plan capacity, and identify improvement areas.
- Technology infrastructure: Reliable data storage, robust security protocols, and scalable computing resources form the basis of an AI-capable organization. Teams that focus on building an adaptable infrastructure often reduce operational costs and maintain consistent performance. Strong architecture also simplifies expansions into new lines of business. This approach provides the agility needed for timely rollouts.
- Data management and quality: AI systems rely on structured, cleaned, and regularly updated data sets. Effective governance protocols ensure data accuracy and consistency across all departments. Quality data supports advanced modeling and analysis without misinterpretations. This aspect boosts trust among technical and non-technical teams when adopting new solutions.
- Skilled workforce: An organization’s workforce should include roles such as data scientists, AI engineers, and analysts with relevant experience. This talent pool becomes more valuable when continuous learning paths are available, fostering professional growth. Upskilling existing team members can fill critical gaps and help with solution development. Focused mentorship and training budgets can accelerate readiness.
- Governance and ethics: Clear policies around AI usage, data handling, and accountability protect both consumers and the organization. Well-defined guidelines also address bias detection and fair model outcomes. Stakeholders often need transparent communication to feel confident about AI-based decisions. This safeguards trust while reducing legal or reputational risks.
- Cross-functional collaboration: AI projects succeed when technical, operational, and financial departments unite under common goals. Bringing varied perspectives into planning avoids missed requirements and fosters collective ownership of solutions. Communication channels that encourage real-time feedback produce better synergy. This unity accelerates project completion and highlights new opportunities for automation.
- Budget and ROI evaluation: Financial planning is essential for resource allocation, pilot testing, and scaling AI initiatives. Clear ROI metrics give stakeholders a basis for measuring progress and celebrating quick wins. Early results often reveal potential expansions or refinements that can increase profit margins. Systematic evaluation helps keep AI programs aligned with wider organizational aims.
- Change management: New AI-focused initiatives require thoughtful planning around workforce transitions and user adoption. Communication campaigns, training sessions, and pilot programs can minimize uncertainty. Incremental rollouts keep project risks manageable and help gather real-time feedback. This approach eases tension and ensures that each step forward is validated by practical results.
These seven components serve as a reference point for any organization preparing to integrate AI. Each element aims to reduce barriers, support alignment, and maintain momentum from initial concept to operational maturity. Leaders who prioritize these areas often gain a clearer sense of how AI can fit into broader objectives. The upcoming AI readiness checklist provides an actionable roadmap for addressing these components in more detail.
AI readiness checklist
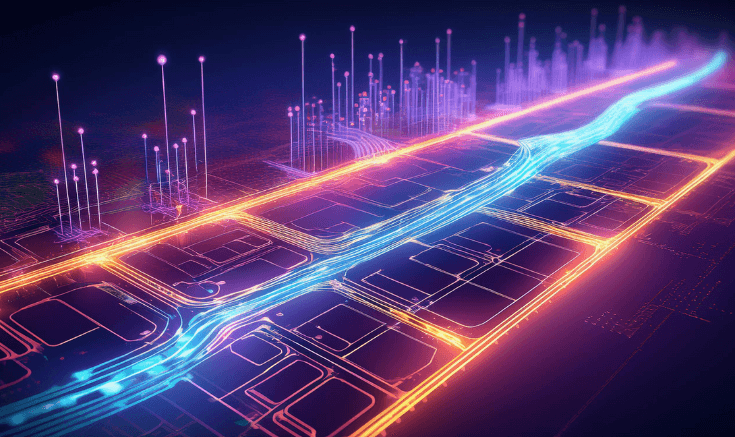
This AI readiness checklist highlights practical steps that guide teams from initial exploration to full-scale adoption. Each checkpoint is tailored to improve cost-effectiveness, shorten project timelines, and reveal fresh business potential. Organizational leaders often seek direct, actionable guidance that tackles daily challenges. An 11-step approach simplifies the process and turns ambitious ideas into tangible outcomes.
Managers, analysts, and executive sponsors can use these steps to gauge progress and set milestones for success. This AI readiness checklist also supports resource planning and risk mitigation, ensuring a better return on investments. Aligning technical teams and business units around these points helps unify efforts toward a shared outcome. When each step is addressed thoroughly, organizations see faster results, enhanced workforce adoption, and stronger ROI.
1. Establish clear objectives
Defining specific goals for your AI initiative ensures that stakeholders remain focused on the most important outcomes. A well-articulated objective streamlines approval channels, budgets, and operational timelines. Specificity also reduces scope creep by highlighting areas with the highest impact. Documented objectives provide a benchmark for measuring progress at each phase.
- Identify core business challenges or user needs your AI project should address
- Outline measurable KPIs (e.g., cost savings, process speed) for each objective
- Secure leadership consensus on how AI outcomes fit overall strategy
Clear objectives promote alignment across finance, operations, and technology teams. This unified vision often results in more direct paths to ROI.
2. Evaluate data readiness
Reviewing your organization’s data landscape uncovers potential gaps and inconsistencies. Complete, well-governed data sets reduce misinterpretation and support advanced modeling. Reliable data checks also encourage trust in analytical outputs, preventing resource waste on flawed inputs. Early detection of data issues avoids bottlenecks later in the project.
- Audit existing data sources for accuracy, freshness, and format uniformity
- Clean and standardize overlapping or inconsistent data sets
- Confirm data accessibility for cross-functional teams and plan governance
A thorough data evaluation helps teams reach production faster and lowers project costs by sidestepping avoidable corrections.
3. Secure executive sponsorship
Leadership approval is a strong catalyst for AI projects. Sponsors expedite resource allocation, remove policy barriers, and advocate for department-wide participation. Their involvement signals that AI is a strategic endeavor, which boosts internal momentum. This backing also provides a sense of confidence for teams hesitant about new processes.
- Identify a high-ranking executive or stakeholder willing to champion the AI effort
- Collaborate with sponsors on budget approvals and resource planning
- Agree on periodic checkpoints for progress reviews
Support from top-level management streamlines timelines, ensuring the project remains a priority across all departments.
4. Build a cross-functional task force
A task force that includes data engineers, operational leaders, finance experts, and end users fosters balanced solutions. This group collaborates on requirements, identifies conflicts, and manages phased rollouts. Open communication reveals overlooked details and fresh ways to apply AI insights. Shared decision-making creates broader ownership of outcomes.
- Invite representatives from each department impacted by AI
- Assign clear roles, responsibilities, and deliverables to every member
- Schedule regular syncs for updates and real-time feedback
A united task force tackles project barriers more effectively, accelerating solution delivery and ensuring all viewpoints are heard.
5. Develop a pilot project
A smaller-scale pilot showcases AI feasibility, clarifies capabilities, and reveals early data or process misalignments. This approach demonstrates return on investment potential before committing substantial resources. Pilot outcomes also generate organizational enthusiasm, paving the way for wider AI adoption.
- Pick a target use case with measurable impact and manageable scope
- Validate data pipelines and performance metrics throughout the pilot
- Document lessons learned to guide future expansions
Successful pilot projects bring evidence-based proof to stakeholders, which often leads to additional funding for larger deployments.
6. Identify required skill sets
Knowing which roles and expertise are critical helps avoid bottlenecks. AI initiatives typically need data science, ML development, and analytics talent. Upskilling or hiring external consultants are ways to fill gaps. Focused career development for existing employees also strengthens internal buy-in.
- Pinpoint current employees with relevant data or modeling experience
- Evaluate whether additional hires or contractors are needed
- Budget for ongoing training or certification programs
Appropriate skill sets reduce risk, speed up project timelines, and improve the quality of AI outputs.
7. Outline data governance standards
Formal governance processes protect data integrity, ensure privacy, and minimize compliance risks. This step clarifies ownership, access levels, and update intervals. Well-defined rules improve model accuracy and maintain trust. Teams benefit from a clear chain of responsibility for data-related decisions.
- Document who can access specific data sets
- Define refresh schedules and establish auditing procedures
- Include policies for bias detection and ethical usage
Robust governance strengthens brand credibility and simplifies future scaling or integration efforts.
8. Adopt robust security measures
Breaches can be costly and harm an organization’s reputation. Encryption, regular audits, and secure access controls limit vulnerabilities. A proactive security posture safeguards sensitive information and instills user confidence. Defensive layers also minimize cleanup expenses after potential incidents.
- Review existing security frameworks and address any immediate gaps
- Implement multi-factor authentication for critical systems
- Conduct scheduled penetration tests or vulnerability scans
Strong security gives leaders confidence to explore advanced AI features without risking data exposure.
9. Plan for scaling strategies
Well-defined expansion milestones keep AI readiness aligned with growth targets. Forward-looking plans cover infrastructure needs, cost projections, and workforce capacity. This approach limits disruptions when new use cases emerge or pilot programs expand. Scalable AI ensures that successful solutions can be replicated elsewhere.
- Map out phases for incremental growth (e.g., more data sources, new user groups)
- Set budget thresholds and resource allocations for each scaling step
- Outline backup plans to handle unexpected demand spikes
Proactive scaling adds agility to the organization, letting teams pivot without halting progress or draining budgets.
10. Integrate monitoring and analytics
Real-time insights help teams track AI performance and catch anomalies early. Monitoring prevents issues like data drift or degraded model accuracy. Consistent reviews of metrics keep AI solutions beneficial and aligned with evolving needs. Quick adjustments also maintain user satisfaction.
- Deploy a centralized dashboard for performance metrics
- Implement alerts for unusual activity or significant accuracy drops
- Create a feedback loop with end users for ongoing improvements
Ongoing analytics reinforce transparency and validate that resources are used efficiently.
11. Establish continuous improvement processes
AI can stall without regular updates and refinements. Structured improvement cycles respond to fresh data, performance metrics, and user feedback. Frequent sprints keep teams accountable and maintain project relevance. A deliberate review method also builds confidence in AI outcomes.
- Set periodic check-ins for feature enhancements
- Gather user feedback to guide iterative adjustments
- Reassess goals and metrics when business conditions shift
This final step confirms that readiness is not just a one-time event but a sustained effort that drives innovation.
Completing each of these 11 steps can transform an abstract idea into a solid AI program. Each action reduces confusion, fosters stakeholder alignment, and accelerates results. Efforts like data governance, pilot testing, and security audits gain structure when viewed through this AI readiness checklist. A long-term perspective turns readiness into an ongoing practice rather than a one-off task.
Crafting an AI implementation strategy
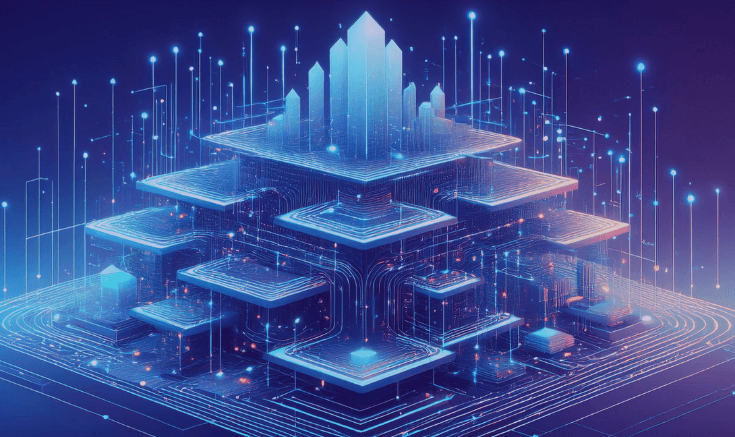
An effective AI implementation strategy aligns every initiative with organizational priorities and stakeholder needs. Setting realistic milestones is vital, especially when balancing resources across multiple teams. Data usage, privacy considerations, and training programs must be clarified early to avoid confusion. The following subsections describe two strategic approaches that guide successful AI adoption.
Top-down alignment
Leaders who champion AI assign clear objectives, approve key investments, and encourage cultural acceptance from the executive level. This approach fosters a shared commitment among department heads, which speeds up resource allocation. Periodic checkpoints validate that each project remains on course and meets expected metrics. A top-down framework works best in organizations where leadership strongly supports long-term AI initiatives.
Collaborative approach
Some enterprises opt for a more inclusive method, inviting cross-functional inputs from the outset. This strategy collects insights from various roles, resulting in robust solutions that address different operational needs. Transparent discussions around data handling and ethical usage foster trust among technical teams and front-line employees. A collaborative model ensures that each participant feels a sense of ownership, which can improve the longevity of AI programs.
Measuring AI readiness success
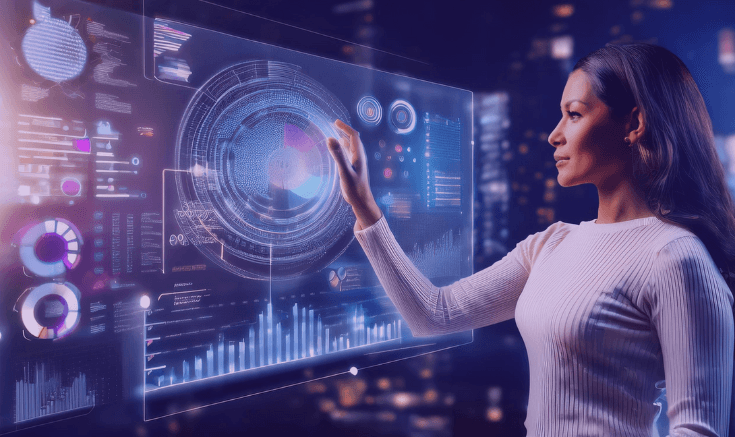
Measuring AI readiness success relies on consistent benchmarks tied to organizational goals. Common metrics include cost savings, productivity boosts, and error rate reductions in day-to-day operations. Clear data points guide resource allocations and highlight priorities that matter most to executive sponsors. Many teams incorporate ongoing evaluation cycles, refining AI solutions based on new metrics or feedback.
Success also involves cultural adoption, reflected in how openly employees embrace AI tools and processes. Readiness can stall when communication gaps persist or when training programs lack depth. Leaders often track user engagement metrics and gather feedback to ensure buy-in at all levels. Over time, a well-measured approach reveals the true ROI of AI initiatives and positions the organization for consistent improvements.
AI readiness is more than a simple technology upgrade—it is a direct route to faster, more cost-effective, and scalable operations. Each data-driven decision becomes an opportunity to optimize resources, identify untapped potential, and focus on what truly matters: producing measurable outcomes. At Lumenalta, we specialize in crafting tailored AI solutions that align with your core business objectives, ensuring you gain practical results from every initiative. Let’s chart a brighter path together.
Common questions about AI readiness
How does an AI readiness checklist help with cost optimization?
Who should be involved in completing the AI readiness checklist?
Is security addressed in the AI readiness checklist?
When is the right time to expand AI projects beyond a pilot?
How can a team measure success after following the AI readiness checklist?
Want to learn how AI can bring more transparency and trust to your operations?