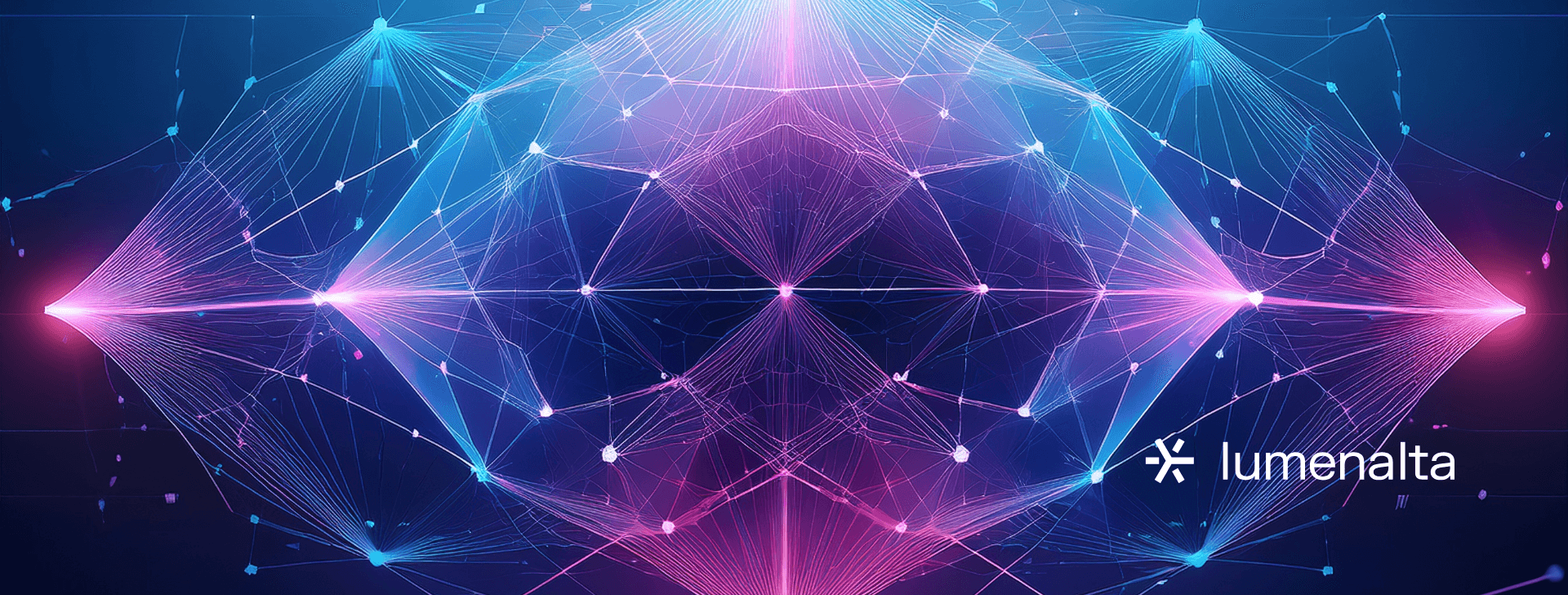
How advanced is AI today (updated 2025)
MAR. 31, 2025
8 Min Read
Advanced AI is now recognized as a vital resource for organizations seeking dependable insights, lower costs, and faster routes to growth.
This focus on automated learning and accurate forecasting has evolved from initial experimental stages into strategic applications that drive tangible returns. Leaders across multiple sectors utilize these techniques to reshape operations, from financial security to predictive product recommendations. Forward-thinking planning, robust infrastructure, and strong data principles serve as cornerstones for AI-powered success.
key-takeaways
- 1. Neural network technologies evolve through continuous data, leading to more flexible and efficient performance.
- 2. Multiple industries benefit from real-time analysis, streamlined workflows, and cost reduction.
- 3. Adopting advanced AI requires careful attention to data quality, infrastructure, and ethical oversight.
- 4. Earlier methods rely on static programming, while modern systems adapt based on shifting data inputs.
- 5. Future progress revolves around automation, personalization, and explainable models for ethical assurance.
What is advanced AI?
Advanced AI refers to modern neural network technologies that handle tasks once restricted to complex human thought. This innovation covers deep learning, ML, and natural language processing at performance levels not previously achieved. Many leaders rely on these algorithms to accelerate data evaluation and enhance resource planning. The question many professionals pose is how advanced AI is, and the answer points to sophisticated capabilities that unlock measurable results.
Researchers classify advanced AI as systems that learn, predict, and refine outputs through continuous data feedback. While classic software followed predefined rules, advanced methods shifted toward algorithms capable of layering deeper data connections. Many enterprises see the potential for cost savings when these approaches are applied at scale. Rapid development in this field indicates that advanced intelligence is no longer a theoretical concept but a proven strategy for growth.
“The question many professionals pose is how advanced is AI, and the answer points to sophisticated capabilities that unlock measurable results.”
Benefits of advanced AI capabilities
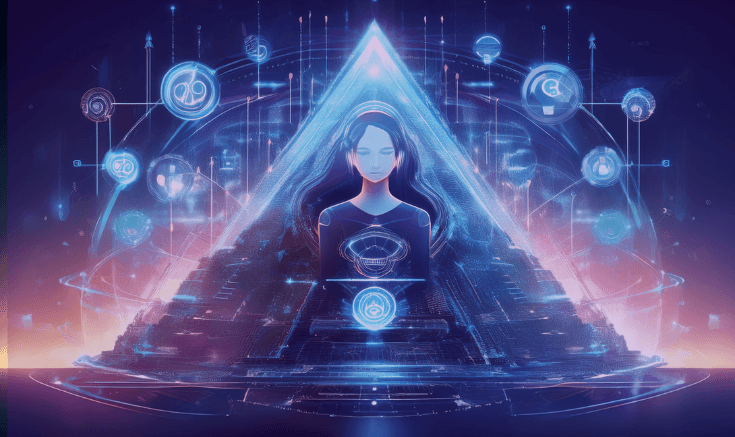
Organizations apply advanced AI capabilities to secure stronger insights, automate tasks, and cut overhead. This approach is known for superior speed to market, leading many teams to adopt data-centric techniques for immediate results. The benefits extend beyond simple time savings, especially when leaders focus on aligning technical investments with measurable financial outcomes. Many still wonder how advanced AI is in these scenarios, and the following list shows how it can optimize varied operations.
- Faster data processing: Advanced platforms evaluate high-volume data sets in real time, helping teams adjust strategies more quickly. Stakeholders see immediate returns through accurate forecasting and proactive improvements.
- Improved customer interactions: Automated tools review user behavior across multiple channels, allowing for more personalized engagements. The outcome is better client retention and increased cross-selling prospects.
- Cost reduction: Deploying advanced AI solutions can streamline workflows, eliminate manual tasks, and reduce rework. Companies channel the resulting savings toward research, strategic upgrades, or staff training.
- Enhanced risk analysis: Models detect anomalies and assess potential threats with greater accuracy, reducing guesswork. Security teams identify untapped vulnerabilities faster, mitigating overall exposure to data breaches.
- Scalability: Flexible AI infrastructures grow in line with shifting user needs, preventing resource overextension. Managers handle expansions efficiently and maintain consistent performance, even during peak data loads.
These points confirm the immediate relevance of advanced AI capabilities for cost savings and streamlined processes. Teams across the globe apply these methods to discover fresh opportunities and optimize ROI. The technology empowers leaders to minimize inefficiencies and adapt more swiftly in high-pressure situations. Overall, the resourcefulness of these approaches sets a strong foundation for the next wave of AI-based innovation.
Applications of advanced AI
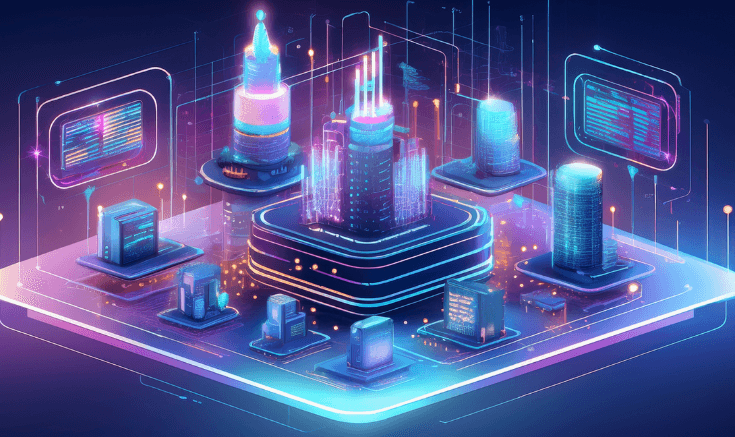
Healthcare solutions
Healthcare systems use advanced AI to improve patient data management, diagnostics, and treatment planning. These solutions analyze complex health records, detect early symptoms, and suggest personalized treatments that may lower complications. This approach supports doctors in handling large patient volumes without compromising quality. Teams that handle critical tasks report better resource allocation and faster recovery rates.
Financial services
Financial institutions rely on predictive analytics and pattern recognition to spot fraud, manage risks, and boost operational accuracy. AI tools review enormous transaction volumes at high speed, helping compliance teams maintain regulatory standards. This tactic strengthens client trust by identifying suspicious behavior well before it escalates. As a result, losses from fraudulent activities are held in check, and customer confidence grows.
Retail optimization
Retail organizations integrate AI personalization for product suggestions and dynamic pricing. Algorithms look at purchase behavior, browsing patterns, and seasonal trends to guide pricing decisions that drive revenue. This strategy creates targeted marketing campaigns, ensuring customers receive offers that suit real-time preferences. Many businesses observe improved margins and greater brand loyalty by implementing advanced data insights.
Challenges of advanced AI
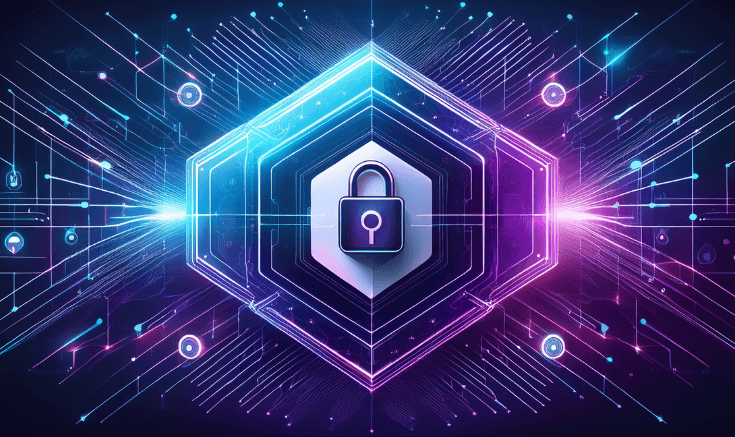
Advanced AI brings exceptional capabilities, but certain hurdles remain that could delay adoption. Some organizations hesitate due to ethical considerations, data security, and regulatory uncertainties. Careful planning is essential, especially when large-scale implementation impacts employees and resource allocation. This list sheds light on five core difficulties faced when building advanced systems.
- Data quality concerns: Effective AI requires accurate, up-to-date information. Incomplete or biased sets can undermine model validity and lead to misguided conclusions.
- Infrastructure complexity: AI frameworks often require specialized hardware, cloud services, and network setups. High-performance clusters can be expensive and may need ongoing maintenance.
- Skill gaps: Many teams lack deep data science expertise, making optimizing AI models difficult. Upskilling staff or hiring external specialists can increase costs and prolong timelines.
- Ethical considerations: Unclear guidelines around privacy and accountability can heighten organizational risk. Stakeholders might struggle to define guardrails that protect both user interests and operational goals.
Resistance to shifts: Integrating AI-based processes may spark cultural pushback. Clear communication and leadership support are necessary to secure buy-in across all levels.
These hurdles highlight the importance of strategic planning before launching large-scale initiatives. Early assessments of data accuracy, operational requirements, and stakeholder readiness often reduce project delays. Many organizations conduct pilot programs to identify blind spots and measure success metrics before wider rollouts. A structured roadmap can resolve these obstacles and lead to more confident use of advanced AI.
How advanced AI solutions compare to earlier methods
The main difference between earlier methods and advanced AI solutions is the capacity to learn and adapt over time without rigid programming. Traditional software typically follows fixed instructions, while new techniques update models through ongoing data analysis. This capability assists teams in refining outcomes and scaling projects in a more cost-effective manner. The comparison below offers a concise view of how these approaches differ in practical deployments.
Factor | Earlier methods | Advanced AI solutions |
---|---|---|
Features | Relies on static code and predefined logic | Uses algorithms that learn from data inputs |
Adaptability | Requires manual updates for new behaviors | Modifies outputs in near real time based on shifting data patterns |
Resource usage | Often limited to single-server setups | Uses distributed computing for higher scalability |
Earlier systems still serve essential functions, but modern techniques handle far more complexity and volume. Teams that rely on advanced AI solutions frequently detect emerging trends sooner and adjust resource allocation accordingly. This agility supports faster project lifecycles and fosters a culture of data-centric reasoning. Combined with robust automation features, these solutions help organizations shorten time to value in critical tasks.
“Earlier systems still serve essential functions, but modern techniques handle far more complexity and volume.”
Examples of advanced AI
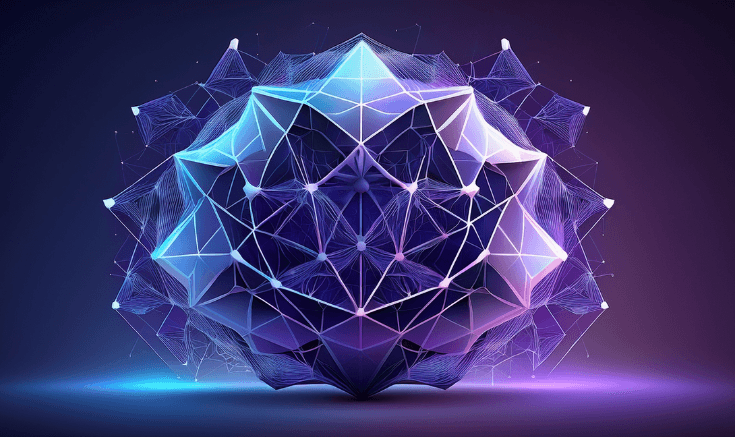
Practical applications of advanced AI appear across varied sectors, each showcasing a unique advantage. Some focus on automating customer support, while others target operational streamlining in supply chains. These examples highlight how advanced AI is when it intersects with everyday tasks. The following points present some of the more visible implementations.
- Virtual assistants: Conversational interfaces handle queries on everything from product recommendations to scheduling. Organizations integrate these AI-based helpers with back-end databases to produce quick, accurate responses.
- Predictive maintenance: Sensors and algorithms anticipate equipment malfunctions before breakdowns occur. This approach helps industrial teams save on repairs and reduce downtime.
- Fraud detection: Financial platforms spot unusual activity and flag suspicious transactions in real time. This layer of protection bolsters client trust and mitigates potential losses.
- Personalized marketing: Recommender systems generate tailored offers based on user profiles and past behaviors. Merchants improve customer satisfaction and boost sales through targeted promotions.
- Autonomous vehicles: Self-driving technologies process sensor data to make safe and efficient route decisions. Manufacturers continue to refine these capabilities as safety regulations advance.
Such wide-ranging use cases confirm that advanced AI is no longer confined to research labs. Many solutions now integrate seamlessly into public and private sectors, producing tangible outcomes. Stakeholders value the speed to market and adaptability that these tools bring. Opportunities continue to grow as more organizations invest in data-focused initiatives and refine their AI implementations.
Predictions for advanced AI progress in 2025
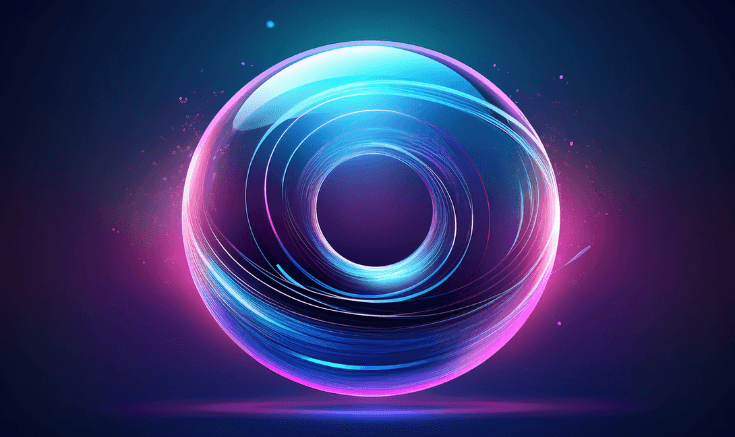
Increased automation for industrial processes
Factories are poised to incorporate AI for assembly, inspection, and resource allocation with minimal human supervision. Executives want systems that can cut production times and reallocate labor to high-value tasks. This focus on operational refinement often includes advanced sensor networks and machine vision. Stakeholders foresee significant cost savings through reduced waste and predictable maintenance cycles.
Expansion of personalized consumer offerings
AI personalization is expected to move beyond simple product suggestions, shaping every digital interaction. Retailers plan to combine real-time data with advanced analytics to predict user preferences across various channels. This method could extend into areas like custom finance packages and tailored healthcare coverage. Specialists anticipate a stronger need for data governance, ensuring privacy while delivering relevant results.
Growth in explainable AI and ethical oversight
Explainable models provide transparent reasons behind each result, reassuring stakeholders about fairness and accountability. Many regulators call for standardized guidelines that allow deeper scrutiny of complex algorithms. Researchers are responding with methods that highlight the weighting of factors, clarifying how outputs emerge. This development opens doors for safer large-scale deployment, where all parties understand the technology’s purpose and limitations.
Advanced AI offers more than just automated processes—it delivers a precise framework for sustainable growth and tangible returns. This technology offers capabilities that streamline data workloads, identify new revenue channels, and optimize resource allocation. At Lumenalta, we specialize in creating tailored solutions that align with specific business goals, ensuring you’re prepared for the next wave of innovation. Let’s chart a brighter path together.
Common questions about advanced AI
How advanced is AI for small businesses?
Are there risks with advanced AI solutions?
Do organizations need specialized staff to use advanced AI?
What is the primary benefit of advanced AI in manufacturing?
Will advanced AI replace human roles?
Want to learn how artificial intelligence can bring more transparency and trust to your operations?