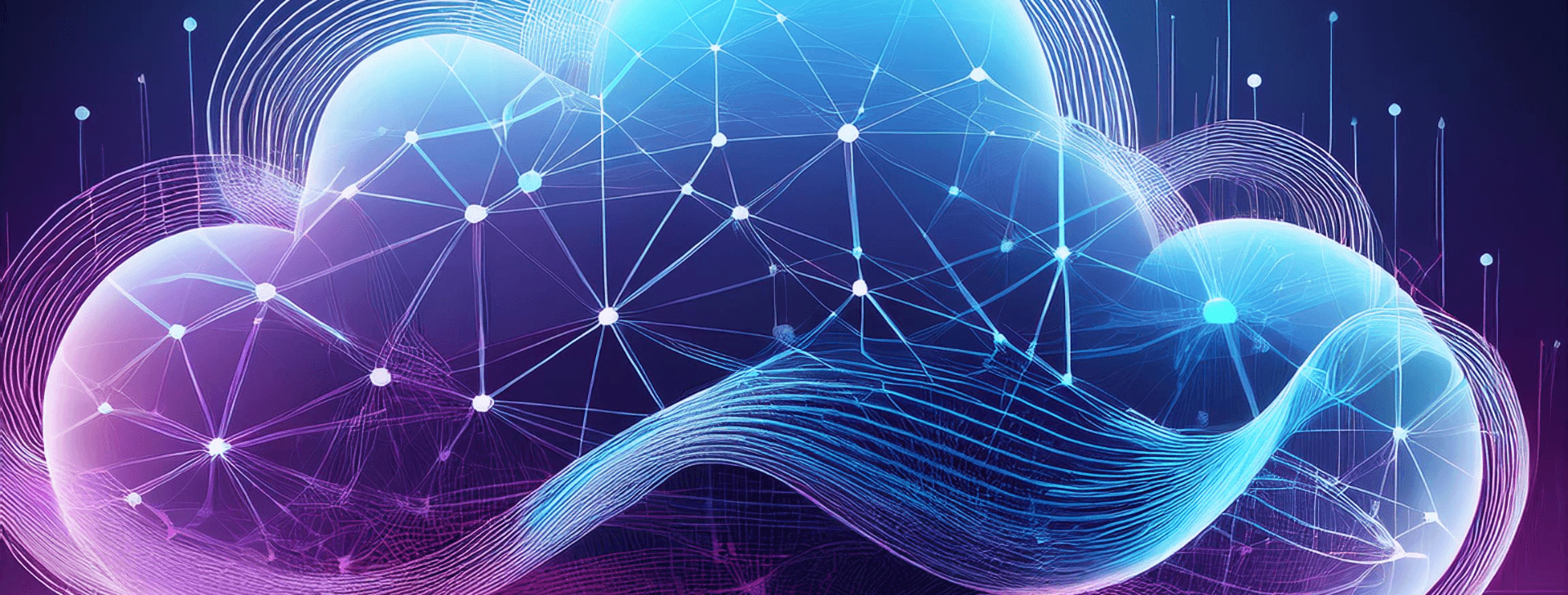
Data quality checklist (updated 2025)
DEC. 24, 2024
6 Min Read
Data quality is a critical driver of operational efficiency and business success.
Accurate, consistent, and reliable data ensures that every decision made is grounded in fact, every process runs smoothly, and every compliance standard is met. Addressing data quality proactively allows organizations to unlock untapped potential, reduce risks, and achieve measurable business outcomes.
Key takeaways
- 1. Accurate and reliable data is essential for driving operational efficiency and business success.
- 2. Establishing measurable KPIs ensures data quality efforts are aligned with organizational goals.
- 3. Automated monitoring tools and regular audits streamline data quality processes and reduce risks.
- 4. Consistently maintaining data standards builds trust and ensures compliance with regulations.
- 5. Proactive data quality management supports better decision-making and long-term scalability.
What is a data quality checklist?
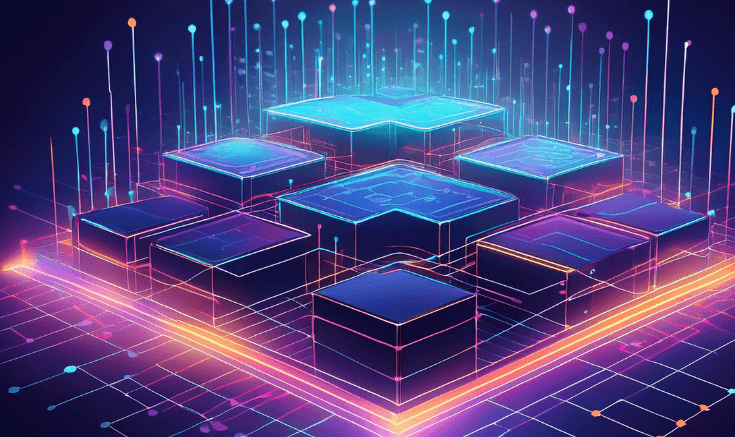
A data quality checklist is a structured framework used to evaluate, manage, and maintain the accuracy, consistency, and reliability of organizational data. It helps identify gaps, inconsistencies, and inaccuracies in datasets while ensuring alignment with business objectives. This checklist serves as a practical guide for organizations aiming to build a robust data foundation for analytics, operations, and decision-making.
High-quality data is essential for achieving operational efficiency, meeting compliance requirements, and supporting strategic goals. A well-designed data quality checklist makes sure that every dataset meets predefined standards, enabling organizations to trust their data for critical processes and insights.
"Data quality is essential for ensuring decisions, operations, and compliance efforts are based on accurate information.”
Why data quality matters for business success
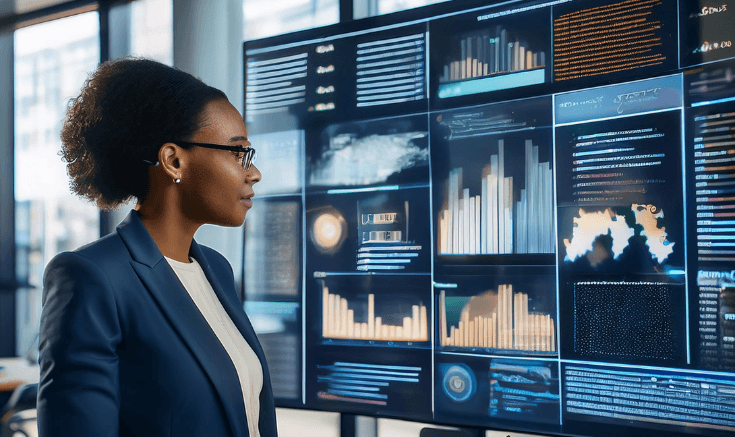
Data quality directly impacts an organization’s ability to achieve its goals, make informed decisions, and deliver value to customers. Poor-quality data can lead to inefficiencies, compliance risks, and lost opportunities, ultimately hindering business performance. High-quality data ensures that operations run smoothly, analytics yield actionable insights, and decisions are based on reliable information.
Accurate and consistent data is essential for maintaining customer trust, meeting regulatory requirements, and optimizing resources. Organizations that prioritize data quality can unlock untapped potential, improve scalability, and drive measurable business outcomes. Ensuring data integrity not only supports current operations but also prepares the business for future challenges and growth.
Key components of a comprehensive data quality checklist
A robust data quality checklist ensures datasets meet established standards for accuracy, consistency, and usability. It provides a structured approach for organizations to assess, improve, and maintain their data assets. Several essential components comprise an effective checklist, designed to address common challenges and enhance data reliability.
- Accuracy: Check that all data is correct and free from errors. Regular audits and cross-referencing against reliable sources help maintain accuracy and minimize discrepancies.
- Consistency: Verify that data is uniform across different systems and departments. Standardizing formats, naming conventions, and units of measurement reduces conflicts and improves compatibility.
- Completeness: Identify and fill gaps in datasets to make sure no critical information is missing. Establish criteria for mandatory fields and validate against them regularly.
- Timeliness: Confirm that data is up-to-date and reflective of current circumstances. Implement real-time updates or scheduled refreshes to maintain relevance.
- Validity: Validate datasets against established rules, formats, or business requirements. Use automated tools or scripts to identify and correct deviations from predefined standards.
- Uniqueness: Remove duplicate records to maintain data integrity and avoid redundancy. Deduplication ensures the accuracy of analyses and decision-making.
A comprehensive data quality checklist addresses these critical areas, helping organizations maintain reliable datasets. This structured approach reduces risks, supports better decision-making, and establishes the scalability and usability of data across all operations.
8-step data quality checklist (updated 2025)
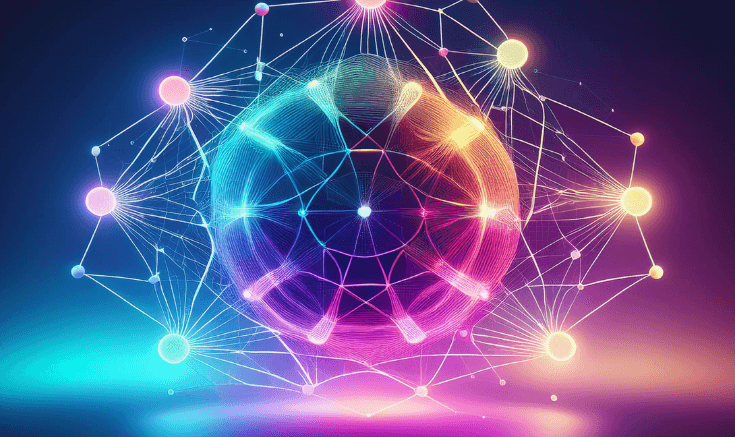
A robust data quality checklist ensures data accuracy, consistency, and reliability, serving as the backbone for sound decision-making and efficient operations. This structured guide addresses critical data quality dimensions, providing actionable steps for maintaining high standards and aligning data with business objectives.
- 1. Validate data accuracy: Cross-check datasets, use validation tools, conduct routine audits, set error thresholds
- 2. Ensure data completeness: Define mandatory fields, check for missing values, flag incomplete entries, review system integrations
- 3. Standardize data formats: Establish formatting rules, automate standardization, audit adherence, distribute guidelines
- 4. Remove duplicate entries: Use deduplication tools, define rules for duplicates, train teams, review and clean datasets
- 5. Monitor data timeliness: Schedule updates, enable real-time syncing, set expiration rules, track latency
- 6. Verify data relevance: Archive legacy data, define relevance criteria, engage stakeholders, prioritize relevant data
- 7. Implement security measures: Encrypt data, apply access controls, conduct audits, ensure compliance
- 8. Establish governance protocols: Define roles, establish escalation paths, set up committees, monitor quality metrics
Step 1: Validate data accuracy
Data accuracy is essential to build trust in the information you rely on for decision-making. Verifying that all data points are correct and free of errors minimizes operational risks and establish that outcomes are based on reliable insights.
- Cross-check datasets against authoritative sources to verify accuracy.
- Use automated validation tools to identify and correct errors in data entry.
- Conduct routine audits to ensure ongoing accuracy across all datasets.
- Set thresholds for acceptable error rates and address discrepancies immediately.
- KPI: Maintain a 95% or higher accuracy rate across critical datasets.
Accurate data supports informed decisions and reduces costly errors that could disrupt business operations or damage credibility. Trustworthy data is a critical foundation for sustainable success.
Step 2: Ensure data completeness
Completeness makes sure that no critical information is missing, which is essential for reliable reporting, compliance, and operational efficiency. Data gaps can hinder processes and obscure valuable insights.
- Define mandatory fields for each dataset and confirm that they are consistently filled.
- Regularly check for missing values in critical fields like customer details or financial records.
- Implement processes to flag incomplete entries and request updates promptly.
- Review system integrations to protect no data is lost during transfers.
- KPI: Achieve 100% completion of mandatory fields in all critical datasets.
Complete datasets allow for comprehensive analysis and prevent disruptions caused by missing information. Having all the necessary details ensures decisions and operations are fully informed and effective.
Step 3: Standardize data formats
Standardized formats create uniformity, improving data usability and reducing errors during integrations and analysis. Inconsistent formats can lead to inefficiencies and misinterpretations across systems.
- Create and enforce standards for data entry, including formats for dates, currencies, and names.
- Use automation tools to identify and resolve inconsistencies in data formats.
- Document and distribute formatting rules to all teams for consistent application.
- Regularly audit datasets to adhere to standardization guidelines.
- KPI: 100% adherence to formatting standards across all datasets within six months.
Standardizing formats enhances the compatibility of data across systems, ensuring smooth operations and eliminating barriers to effective analysis.
Step 4: Remove duplicate entries
Duplicate data inflates costs, skews analytics, and creates inefficiencies in processes. Ensuring uniqueness in datasets maintains their integrity and optimizes their value.
- Use deduplication tools to identify and merge or eliminate duplicate entries.
- Establish clear rules for determining duplicates, such as identical email addresses or phone numbers.
- Regularly review and clean datasets to prevent duplication over time.
- Train teams on avoiding duplicate entries during manual data entry processes.
- KPI: Reduce duplicate records to less than 1% of the total dataset volume.
Eliminating duplicates enhances the accuracy of reports, minimizes storage costs, and reduces operational redundancies. Clean and unique datasets maximize the reliability of business insights.
Step 5: Monitor data timeliness
Timeliness establishes that all data reflects current conditions, enabling organizations to act based on the latest information. Outdated data can lead to missed opportunities or misaligned strategies.
- Schedule regular data updates to ensure all datasets reflect the latest information.
- Implement real-time syncing for dynamic data sources, such as CRM systems.
- Set expiration rules for time-sensitive data and remove outdated records.
- Track data latency and check that it meets business requirements.
- KPI: Ensure that 90% of dynamic datasets are updated within 24 hours.
Timely data supports proactive decision-making and operational responsiveness, keeping your organization aligned with real-time needs and opportunities.
Step 6: Verify data relevance
Relevance checks that datasets are aligned with current business needs and objectives. Irrelevant or outdated data adds noise, complicates processes, and increases storage costs without contributing value.
- Identify and archive legacy data that no longer serves business purposes.
- Define relevance criteria based on business objectives and update them regularly.
- Engage stakeholders to confirm the utility of datasets for specific functions.
- Use filtering tools to isolate and prioritize relevant information during analysis.
- KPI: Archive or remove 100% of irrelevant data from active datasets within one year.
Relevant data focuses resources on actionable insights, streamlining operations and enhancing decision-making. This process reduces clutter and ensures data supports measurable outcomes.
Step 7: Implement security measures
Data security protects sensitive information and establishes compliance with regulatory standards. Safeguarding data from breaches builds trust with stakeholders and maintains the integrity of your systems.
- Encrypt data both in transit and at rest to prevent unauthorized access.
- Implement access controls to restrict data handling to authorized personnel only.
- Conduct regular security audits to identify vulnerabilities and address them promptly.
- Ensure compliance with relevant regulations such as GDPR, HIPAA, or industry-specific standards.
- KPI: Achieve zero security breaches and maintain 100% regulatory compliance.
Strong security measures protect against costly breaches and reputational risks while fostering confidence in data handling processes.
Step 8: Establish governance protocols
Governance establishes accountability and oversight, enabling consistent adherence to data quality standards. Without governance, efforts to maintain quality can become fragmented and ineffective.
- Define governance roles, such as data stewards and quality managers, with clear responsibilities.
- Establish escalation paths for addressing data quality issues promptly.
- Set up governance committees to review metrics and enforce accountability.
- Use dashboards to monitor quality metrics and compliance across teams.
- KPI: Conduct governance reviews quarterly, with 100% resolution of identified issues.
Effective governance creates a culture of accountability, ensuring ongoing data quality improvements and alignment with organizational goals.
This comprehensive data quality checklist addresses all critical dimensions of maintaining high-quality datasets. Ensuring accuracy, completeness, timeliness, and security fosters trust and operational efficiency. Regular reviews and updates keep the checklist aligned with business needs, ensuring data continues to support measurable outcomes and organizational success. Implementing this framework creates a scalable, reliable foundation for future growth and innovation.
“Automated monitoring tools and regular audits streamline data quality processes and reduce risks.”
Creating and implementing a data quality checklist
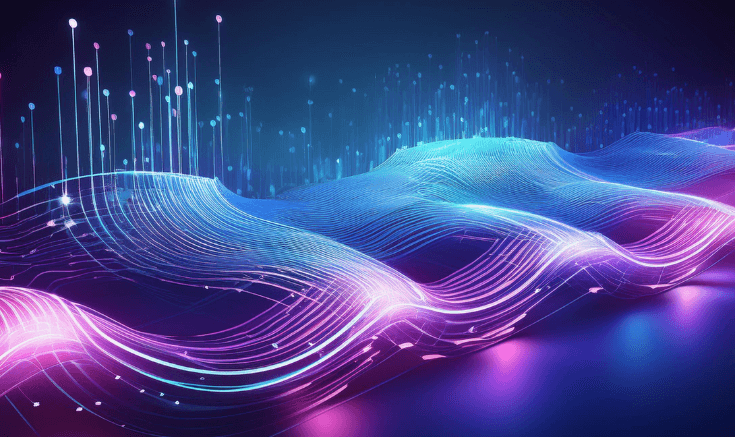
Creating and implementing a data quality checklist is a strategic process that ensures organizations maintain reliable, accurate, and actionable data. This structured approach addresses key dimensions of data quality, aligning them with organizational goals and operational priorities. By systematically evaluating and enhancing data quality, businesses can improve decision-making, drive operational efficiency, and build trust in their data assets.
Define data quality objectives
Defining clear objectives establishes the purpose and focus of a data quality initiative. These objectives align efforts with organizational priorities and provide meaningful outcomes. Business objectives impacted by data quality, such as compliance, customer experience, or operational efficiency, should be identified early. Setting measurable benchmarks, such as improving data completeness to 95% or reducing duplicate entries by 80%, helps guide the process. Objectives should also be documented and approved by stakeholders to ensure alignment.
This step provides a framework for evaluating progress and establishes accountability throughout the initiative. A focused approach minimizes wasted effort and delivers measurable results that align with business goals.
Assess existing data quality
A comprehensive assessment identifies gaps, inconsistencies, and strengths in the current state of your data. Audits are essential for evaluating accuracy, consistency, and completeness across datasets. Missing or redundant fields, error sources, and integration mismatches should be identified. Datasets should be categorized by priority based on their role in decision-making and operations.
Documenting current quality levels and challenges helps establish a baseline for targeted improvements. This baseline enables organizations to focus efforts on areas with the most significant impact, setting the stage for long-term success.
Develop data quality rules
Establishing clear data quality rules creates standards for consistency and reliability across all datasets. These rules guide validation and provide uniformity, reducing errors and improving data usability. Examples include defining validation rules for critical fields, standardizing naming conventions, and establishing criteria for mandatory fields.
Documenting and distributing these rules to stakeholders establishes transparency and adherence. Consistent enforcement of these standards reduces discrepancies and supports accurate analytics across the organization.
Implement quality control processes
Quality control processes ensure data adheres to established rules and standards, preventing errors from propagating. Integrating validation tools into ETL workflows, setting up error detection systems, and scheduling regular audits are effective strategies.
Access controls and remediation plans address unauthorized changes and quickly resolve issues. Automation minimizes manual intervention, making quality control processes more efficient and reliable.
Train teams and assign responsibilities
Training and role assignments corroborate effective implementation by fostering team alignment and accountability. Teams should be educated on data quality rules, tools, and processes through workshops, webinars, or training materials. Assigning data stewardship roles to oversee compliance and quality control ensures sustained efforts.
Establishing feedback loops allows teams to report challenges and suggest improvements, ensuring the framework remains effective and adaptive. Empowered teams build a strong foundation for long-term data quality success.
Monitor and refine the checklist
Continuous monitoring ensures that the data quality checklist changes with organizational needs. Real-time dashboards tracking data quality metrics like error rates or completeness scores enable regular reviews. User feedback and quarterly updates to the checklist address gaps, inefficiencies, or emerging challenges.
This living document ensures the framework adapts to meet new demands, driving ongoing improvements in data quality and operational outcomes. Regular refinement supports scalability and alignment with evolving business objectives.
A well-implemented data quality checklist provides a reliable framework for sustained business success. It builds trust in data, enhances decision-making, and ensures compliance with organizational goals. Continuous refinement fosters accountability and operational excellence, enabling organizations to adapt to challenges and achieve long-term scalability.
How to measure and monitor data quality effectively
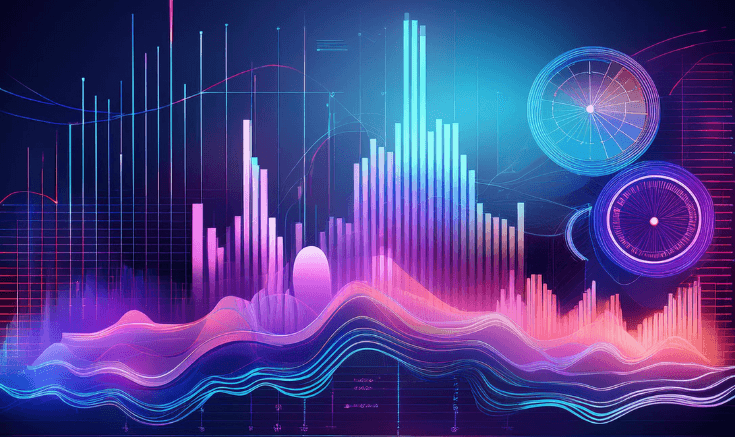
Measuring and monitoring data quality affirms that organizations maintain high standards and continuously improve their data assets. Establishing clear metrics and consistent monitoring processes allows businesses to track progress, address issues proactively, and align data quality with operational and strategic goals.
- Establish key performance indicators (KPIs): Define metrics that quantify the success of data quality initiatives, such as data accuracy rates, completeness percentages, error rates, and timeliness benchmarks. Tailor these indicators to align with specific business objectives and review them regularly to ensure relevance.
- Implement automated monitoring tools: Use tools to track data quality metrics, detect anomalies, and flag inconsistencies in real time. Automation reduces manual effort, checking data remains accurate and up to date, and facilitates timely resolution of issues.
- Conduct regular audits: Schedule periodic audits to gain a comprehensive understanding of your data quality. Align these audits with your data governance framework, focusing on critical datasets to identify recurring errors, patterns, or systemic issues.
- Incorporate stakeholder feedback: Engage with stakeholders to corroborate the data quality framework addresses operational needs and user challenges. Collect feedback from teams and end-users to highlight practical issues and identify opportunities for improvement.
Effective data quality monitoring ensures that your organization can trust its data to meet operational and strategic goals. Regular audits, automated tools, and stakeholder engagement help to maintain high standards, reduce inefficiencies, and address issues proactively. These practices not only build confidence in your data but also enable sustainable growth and measurable business outcomes over time.
Data quality is the backbone of modern business operations, ensuring that every decision and process is supported by accurate, actionable insights. At Lumenalta, we provide tailored solutions to help organizations maintain the highest standards of data quality, enabling seamless operations and strategic growth.
Partner with us to build a future-proof data framework that supports your goals today and tomorrow.
Table of contents
- What is a data quality checklist?
- Why data quality matters for business success
- Key components of a comprehensive data quality checklist
- 8-step data quality checklist (updated 2025)
- Step 1: Validate data accuracy
- Step 2: Ensure data completeness
- Step 3: Standardize data formats
- Step 4: Remove duplicate entries
- Step 5: Monitor data timeliness
- Step 6: Verify data relevance
- Step 7: Implement security measures
- Step 8: Establish governance protocols
- Creating and implementing a data quality checklist
- Define data quality objectives
- Assess existing data quality
- Develop data quality rules
- Implement quality control processes
- Train teams and assign responsibilities
- Monitor and refine the checklist
- How to measure and monitor data quality effectively
- Common questions about data quality checklist
Common questions about data quality
What is a data quality checklist?
Why is data quality important for businesses?
How can I measure the success of data quality initiatives?
What tools can help improve data quality?
How often should data quality checks be conducted?
Want to learn how data quality can bring more transparency and trust to your operations?