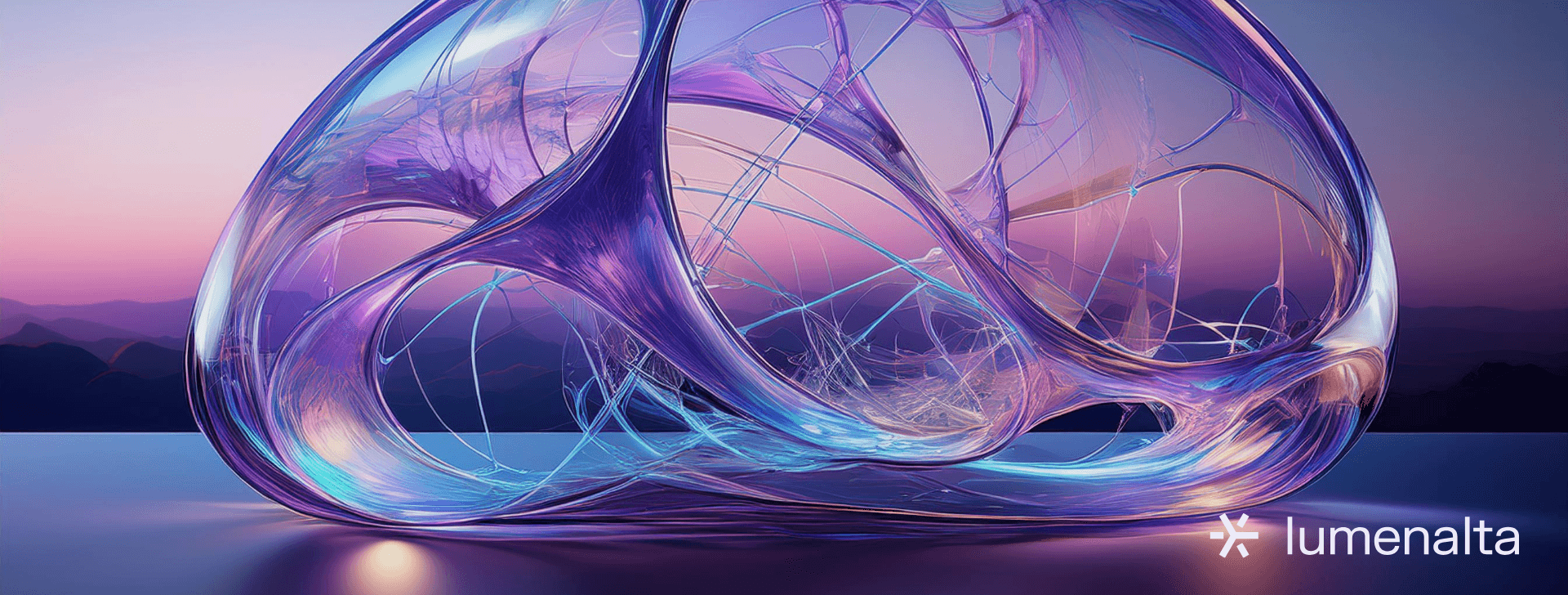
What is conversational AI?
OCT. 31, 2024
6 Min Read
Conversational AI has become a cornerstone of digital transformation, redefining how businesses interact with customers, partners, and employees.
At its core, conversational AI leverages advanced technologies such as natural language processing (NLP) and machine learning (ML) to simulate human-like conversations through text or voice. Whether managing customer inquiries via chatbots, automating appointment scheduling with virtual assistants, or delivering personalized product recommendations, conversational AI offers a way for businesses to interact seamlessly with users at scale.
Today, businesses across industries are implementing conversational AI to enhance customer experience, increase operational efficiency, and reduce costs. As users demand quicker, more convenient service, AI-driven chatbots and voice assistants fill the gap by providing real-time, personalized responses. This blog will explore how conversational AI works, its components, use cases, benefits, challenges, and the steps required to build one. With conversational AI at the forefront of innovation, organizations are reaping measurable rewards by integrating these systems into their business strategies.
Key takeaways
- 1. Conversational AI seamlessly combines NLP, machine learning, and voice recognition to deliver real-time, human-like interactions.
- 2. AI-powered solutions enhance customer experiences, automate routine inquiries, and provide businesses with operational efficiencies.
- 3. Conversational AI systems must continuously learn from interactions to improve their accuracy, contextual understanding, and relevance.
- 4. Challenges such as data privacy compliance, integration difficulties, and training requirements must be addressed for successful implementation.
- 5. AI technology will continue to evolve, driving personalized user experiences, emotional intelligence, and integration with immersive digital environments.
How does conversational AI work?
Conversational AI integrates a sophisticated combination of technologies, all working harmoniously to simulate seamless interactions between humans and machines. These technologies ensure that every user interaction—whether through voice, text, or chat—is processed, understood, and responded to in a way that feels both natural and relevant. The goal of conversational AI is not just to recognize inputs but to offer meaningful exchanges, maintaining context throughout the interaction and providing timely, personalized responses. From understanding user intent to generating human-like replies, each stage plays a crucial role in delivering efficient communication at scale.
A well-functioning conversational AI system must account for several layers of complexity, such as understanding accents, deciphering ambiguous queries, and maintaining conversation flow across multiple exchanges. This entire process requires synergy between various technical components to ensure that responses align with the user’s expectations. Let’s explore the essential stages involved in this process:
Automatic speech recognition (ASR)
ASR is the technology responsible for converting spoken language into text. It forms the first step for voice-based systems like virtual assistants (e.g., Siri or Google Assistant). This step relies on advanced algorithms and acoustic models to identify phonemes and words, even accounting for background noise or accents. An effective ASR system ensures that the user’s spoken input is transcribed accurately, setting the foundation for further processing.
Natural language processing (NLP)
NLP breaks down the user’s input into structured data. By parsing the syntax and analyzing grammatical patterns, the system identifies key phrases, entities, and parts of speech. NLP is essential in determining the context of user queries, ensuring that even ambiguous inputs are correctly understood. It also helps the system detect sentiment and tone, adding nuance to the interaction.
Natural language understanding (NLU)
While NLP focuses on processing the structure of language, NLU dives into the meaning behind the words. This component extracts the user’s intent and key information, such as dates, locations, or product names. For example, when a user says, "When will my order arrive?", the NLU identifies the intent (checking order status) and extracts relevant details. NLU also manages ambiguous terms and conversational slang to maintain accuracy.
Dialogue management system
The dialog manager ensures the flow of conversation is logical and consistent. It determines what the AI should say next by analyzing the context of the conversation. Whether asking clarifying questions, transitioning topics, or confirming details, this component ensures a smooth conversational experience. It also handles multi-turn interactions, maintaining context across several exchanges.
Natural language generation (NLG)
NLG transforms structured data into human-like responses. Instead of delivering robotic or template-based answers, NLG creates personalized and contextually appropriate replies. For example, an AI might say, "Your order will arrive tomorrow by 5 PM" instead of a generic “Your order is on the way.” This capability ensures conversations feel natural and engaging.
Machine learning (ML)
Machine learning continuously refines the AI system's ability to interpret and respond effectively. The system learns from every user interaction, improving its understanding of language, user intent, and optimal responses over time. ML also enables the AI to adapt to changes in user behavior, new products, or evolving trends.
Text-to-speech (TTS) conversion
TTS plays a crucial role in voice-based interactions by converting AI-generated text responses into spoken language. Advanced TTS systems ensure that the responses maintain natural cadence, tone, and inflection, providing a human-like voice experience. This component is critical for virtual assistants, customer service bots, or interactive voice response (IVR) systems.
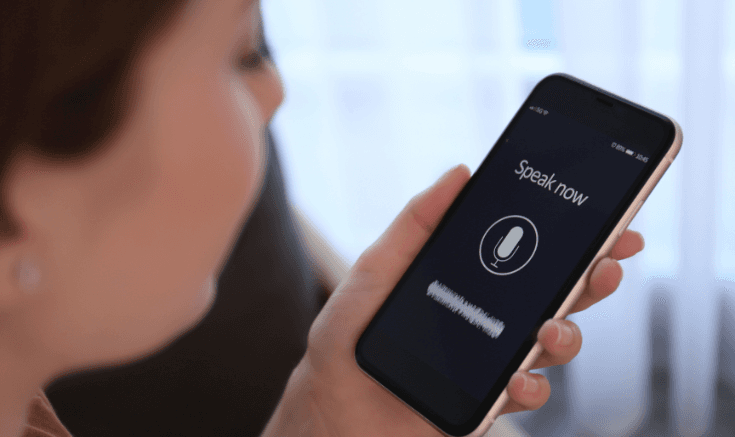
Other Components
Dialog manager
A conversation is rarely limited to a single exchange. The dialog manager ensures the AI follows the correct path, maintaining coherence throughout the interaction. It tracks the conversation context, remembers previous inputs, and ensures that follow-up questions align with the user’s goals. It also gracefully handles interruptions or unexpected queries without breaking the flow.
APIs for integration
Seamless integration is essential for delivering context-aware responses. APIs allow conversational AI to connect with external databases, CRMs, and other enterprise systems. For example, an AI bot assisting with order inquiries might use APIs to pull real-time information from the company’s inventory system, ensuring users receive accurate and timely responses.
Why seamless interactions matter
The effectiveness of conversational AI lies in its ability to deliver frictionless interactions across various channels. By handling routine inquiries, managing complex processes, and personalizing responses, these systems meet user expectations at scale, improving satisfaction and engagement. Moreover, the integration of ML ensures that conversational AI evolves continuously, becoming smarter and more intuitive over time.
For businesses, this translates into more efficient operations, lower support costs, and enhanced customer experiences. Whether deployed as a chatbot, voice assistant, or interactive customer service agent, conversational AI ensures that users receive timely and accurate support, strengthening relationships and driving long-term value.
"Training your AI system with relevant data is essential to ensure it delivers accurate and meaningful responses."
8 use cases of conversational AI
Conversational AI offers immense versatility, making it a valuable asset across industries by automating both customer-facing and operational tasks. Its ability to understand and respond naturally to human inputs enables businesses to deliver consistent, personalized interactions that meet customer expectations while streamlining internal processes. Whether in customer service, healthcare, retail, or financial services, conversational AI plays a key role in improving operational efficiency, reducing costs, and enhancing user experiences. Below is a deeper dive into the diverse ways businesses are leveraging conversational AI across various industries.
1. Customer support and service
AI-powered chatbots and virtual agents are transforming customer service by offering instant responses to common inquiries. Instead of waiting on hold, customers can engage with a chatbot to troubleshoot issues, access product details, or resolve complaints. Virtual assistants also handle repetitive queries, freeing up human agents to focus on more complex cases. Many companies use conversational AI to provide 24/7 support, ensuring customers receive help outside of traditional working hours. Additionally, these systems can seamlessly escalate cases to human agents when needed, ensuring no disruption in service quality.
2. E-commerce and retail
Conversational AI is revolutionizing how retailers engage with customers. Online shopping assistants use AI to help customers find products, track orders, and receive personalized recommendations. Through dynamic interactions, chatbots provide real-time product suggestions based on browsing history or user preferences, enhancing the shopping experience. Some retailers also use virtual agents for post-purchase support, such as handling returns or processing refunds. AI chat interfaces reduce cart abandonment by guiding customers through the checkout process and answering last-minute queries in real time, ultimately increasing conversion rates.
3. Healthcare and telemedicine
In healthcare, conversational AI streamlines patient management by automating appointment scheduling, sending reminders, and offering symptom checks. Virtual health assistants act as a first line of support, guiding patients to the appropriate care services based on their inputs. AI bots also improve the efficiency of telemedicine by gathering preliminary patient information before consultations, allowing healthcare providers to focus on diagnosis and treatment. Some AI systems offer mental health support through anonymous chats, helping users manage stress or anxiety between therapy sessions.
4. Banking and financial services
Financial institutions use conversational AI to enhance customer experiences and automate routine banking tasks. AI-powered assistants help customers manage accounts, process payments, and receive alerts about suspicious activity. Many banks offer chatbots within mobile apps to guide users through services such as loan applications, investment advice, or fraud reporting. Conversational AI also enables secure transactions through voice authentication, providing customers with fast, frictionless access to their financial data. This reduces the need for customers to visit physical branches, improving convenience and service accessibility.
5. SaaS business support
SaaS companies integrate conversational AI to enhance customer onboarding, offer in-app assistance, and provide personalized support. AI-powered bots walk new users through product features, ensuring smooth adoption and reducing churn. These systems also provide troubleshooting assistance, resolving technical issues without requiring human intervention. SaaS providers benefit from conversational AI by gathering user feedback during interactions, which can be used to refine product offerings and optimize the customer journey. AI bots can also proactively notify users of new features or updates, maintaining engagement and driving retention.
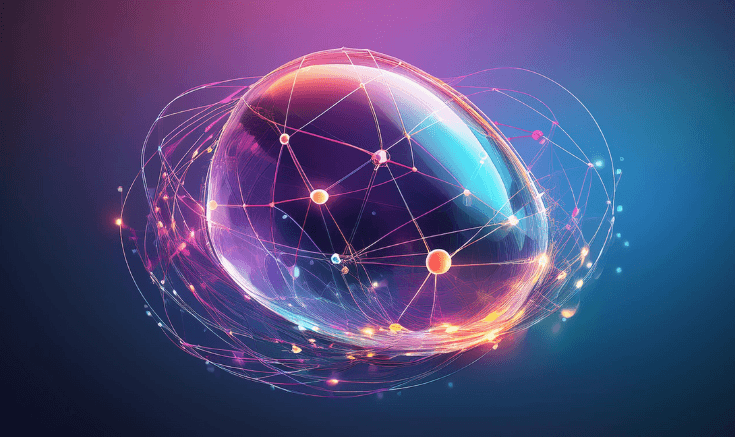
6. Human resources and recruitment
HR departments use conversational AI to automate parts of the recruitment process, such as screening applicants, scheduling interviews, and answering frequently asked questions about job openings. AI bots can conduct preliminary candidate assessments, ensuring only qualified applicants proceed to the next stage. During onboarding, AI-driven assistants provide new employees with essential information, helping them acclimate to the company culture. These bots are also used internally to handle common employee inquiries related to payroll, benefits, or leave policies, reducing HR workload.
7. Travel and hospitality
Travel agencies and hospitality providers use conversational AI to improve guest experiences and streamline operations. AI-powered virtual assistants help users book flights, hotels, and vacation packages while offering personalized recommendations based on travel preferences. Hotels deploy chatbots to handle guest requests, such as room service or concierge services, improving response times. Additionally, travel companies use AI to provide real-time updates on bookings, weather forecasts, and itinerary changes, ensuring travelers have all the information they need at their fingertips.
8. Education and e-learning
Educational institutions and e-learning platforms leverage conversational AI to provide virtual tutoring, administrative support, and personalized learning experiences. AI-driven assistants help students with course selections, assignment deadlines, and access to learning resources. Instructors also benefit from AI bots that handle repetitive tasks, such as answering student inquiries or grading quizzes. Additionally, conversational AI supports personalized learning by analyzing student performance and offering targeted recommendations to improve outcomes.
In each of these scenarios, conversational AI enhances both operational efficiency and customer satisfaction by automating routine tasks and delivering personalized interactions. Businesses that deploy conversational AI not only improve service quality but also reduce costs, increase productivity, and drive higher customer engagement. As AI technology continues to evolve, these systems will become even more integral to creating seamless, responsive experiences across industries.
Benefits and challenges of conversational AI
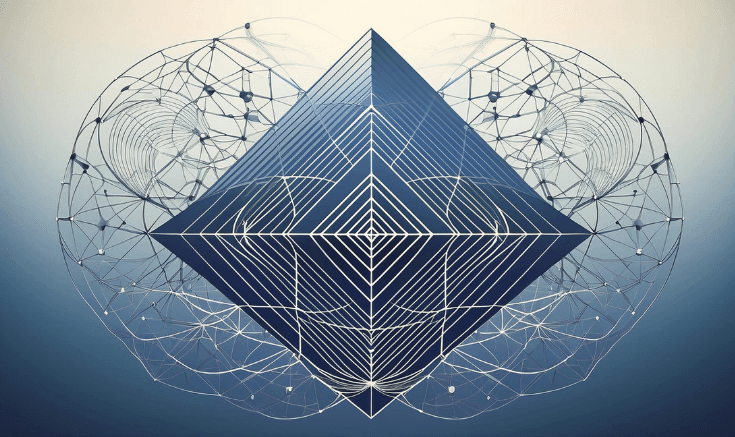
Implementing conversational AI brings substantial advantages, helping businesses enhance customer experiences, streamline operations, and reduce costs. However, integrating such technology comes with its share of challenges, requiring careful planning and continuous optimization. Understanding both the benefits and obstacles allows businesses to maximize the value of conversational AI while navigating potential pitfalls.
Benefits | Challenges |
---|---|
Enhanced customer experience: AI offers quick, personalized responses, improving customer satisfaction and fostering brand loyalty. | Data privacy and security: Managing sensitive customer data requires strict compliance with regulations such as GDPR and CCPA, along with robust encryption measures. |
Increased operational efficiency: Automation of routine inquiries frees human agents to focus on complex tasks, driving productivity and lowering costs. | Integration complexity: Seamless integration with existing systems, such as CRMs and databases, can be time-consuming and resource-intensive. |
Scalability and 24/7 availability: AI can handle unlimited inquiries simultaneously, ensuring businesses are accessible around the clock without increasing staffing needs. | Contextual understanding limitations: AI systems often struggle with maintaining conversational context over multi-turn interactions, reducing their effectiveness in complex scenarios. |
Data-driven insights: Every interaction generates valuable data, enabling businesses to analyze trends, preferences, and areas for improvement. | Language and cultural nuances: AI must account for dialects, colloquialisms, and regional expressions, which complicate development and deployment. |
Cost savings: Automating customer support and operational tasks reduces the need for extensive staffing, driving significant savings without compromising service quality. | Training and maintenance: Continuous updates and training are necessary to keep AI models relevant, requiring an ongoing commitment to monitoring and optimization. |
A balanced approach to maximizing benefits and addressing challenges
Conversational AI offers undeniable value to organizations across industries by enhancing engagement, increasing efficiency, and reducing costs. The scalability and availability of these systems ensure that businesses can meet user expectations around the clock, offering consistent service even outside regular business hours. Additionally, the ability to generate actionable insights from every interaction empowers companies to make data-driven decisions, refine their strategies, and better serve their customers.
However, implementing conversational AI is not without its hurdles. Integrating AI with existing systems demands careful planning, and maintaining the accuracy and contextual understanding of conversations requires continuous refinement. Compliance with data privacy laws is paramount, especially in industries handling sensitive information, such as finance and healthcare. Furthermore, AI systems must accommodate regional and linguistic variations, ensuring interactions are inclusive and culturally appropriate.
To succeed, businesses need a thoughtful, strategic approach that balances the benefits with the challenges. By investing in robust security protocols, seamless integrations, and ongoing model training, organizations can unlock the full potential of conversational AI while mitigating risks. With the right framework in place, conversational AI becomes a powerful tool for driving long-term business impact, improving customer experiences, and staying ahead in an increasingly competitive market.
"With the right framework in place, conversational AI becomes a powerful tool for driving long-term business impact, improving customer experiences, and staying ahead in an increasingly competitive market."
How to build a conversational AI: A step-by-step guide
Developing a conversational AI system requires a structured, iterative approach to ensure the solution meets user needs and aligns with business objectives. Building one successfully involves defining clear goals, choosing the right technologies, and continuously refining the system based on real-world interactions. Below is a detailed, step-by-step guide to building a robust conversational AI solution.
1. Define your objectives and use cases
The foundation of any successful conversational AI project lies in clearly identifying its purpose. Start by determining the primary goals your AI system will achieve—whether it’s streamlining customer support, automating routine tasks, or enhancing the user experience. Define specific use cases that align with your business needs. For example, will the AI handle order tracking inquiries, troubleshoot technical issues, or assist with onboarding new users? This step helps ensure that the AI solution delivers measurable value and meets user expectations. Having clearly defined use cases also allows you to create performance metrics to monitor the system’s effectiveness over time.
Example use cases: Reducing customer service response time, guiding e-commerce customers through checkout, or providing employees with automated HR support.
2. Select the right platforms and tools
Choosing the right platform is critical to the success of your conversational AI system. Consider whether your use cases require text-based, voice-based, or multi-channel interactions. Tools like Google Dialogflow, Amazon Lex, or Microsoft Bot Framework offer different capabilities. Evaluate these platforms for their NLP (natural language processing) strength, integration ease, and scalability. Your choice of tools should also align with your business’s existing tech stack to facilitate seamless integration.
Key factors to evaluate: Can the platform handle complex conversations? Does it support multiple languages? Does it offer pre-built connectors for integration with your CRM or customer database?
3. Design the conversation flow
Creating a clear and logical conversation flow ensures the AI interacts with users smoothly across various scenarios. Begin by mapping out conversation paths, including greetings, questions, and follow-up responses. Structure fallback responses for when the AI cannot understand a query, ensuring the system gracefully handles errors or ambiguous input. A well-designed dialog flow keeps the interaction engaging and context-aware, helping users feel that they are interacting with a helpful assistant rather than an automated system.
Tip: Use flowcharts or decision trees to visualize the conversation paths and ensure all potential user inputs are accounted for.
4. Develop and train your AI models
Training your AI system with relevant data is essential to ensure it delivers accurate and meaningful responses. Use a combination of real-world scenarios and sample interactions to train the NLP and NLU components. This step involves creating datasets with example queries and expected outputs, allowing the AI to learn user intent effectively. If the AI will interact with specific industry jargon, ensure that these terms are included in the training data. Continuous training and refinement are required to improve the AI’s understanding and adapt it to evolving user behaviors.
Example: An AI assistant in retail might be trained to understand phrases like “track my package” or “return policy details.”
5. Integrate with business systems and APIs
For conversational AI to provide meaningful responses, it must connect seamlessly with existing business systems. APIs allow the AI to access data in real time from your CRM, inventory system, or customer database. For example, an AI chatbot handling order inquiries must integrate with your order management system to pull up accurate delivery information. Ensure the system’s APIs are secure and scalable to handle increasing volumes of interactions as your business grows.
Best practices: Use RESTful APIs or pre-built integrations to minimize development time and ensure smooth data flow between the AI and external systems.
6. Test and iterate
Testing is a crucial phase in building conversational AI. Conduct multiple rounds of testing to identify gaps in the conversation flow, uncover bugs, and measure the AI’s accuracy. Use both scripted tests (predefined scenarios) and unscripted tests (real user inputs) to evaluate the AI’s performance. Pay close attention to how well the AI maintains context across multi-turn interactions. Gather user feedback and analyze conversation logs to understand where the AI falls short. Continuous iteration ensures that the system evolves based on real-world interactions.
Key metrics to monitor: Response accuracy, resolution rate, user satisfaction, and fallback frequency.
7. Deploy and monitor
Once the conversational AI is ready, it’s time to deploy it across the appropriate channels—whether that’s a website chatbot, mobile app, voice interface, or messaging platform like WhatsApp. Ensure a smooth launch by conducting a soft rollout with a limited audience to monitor performance. Implement monitoring tools to track key metrics such as response time, accuracy, and user satisfaction. Use analytics to identify trends and areas for improvement, ensuring the AI continues to meet user needs over time.
Proactive monitoring: Set up alerts for when the AI encounters high fallback rates or unresolved queries, allowing your team to intervene quickly.
Continuous improvement: The key to long-term success
Building a conversational AI system is not a one-and-done process. It requires continuous monitoring and iterative improvements to stay relevant and effective. User behaviors and business needs change over time, and your AI solution must adapt accordingly. Regular updates to training data, improvements to the conversation flow, and integrations with new systems ensure that the AI remains a valuable asset. Businesses must also stay ahead of technological advancements, such as new NLP models or emerging communication platforms, to maintain a competitive edge.
By following this step-by-step guide, businesses can build conversational AI systems that deliver meaningful, real-time interactions. With the right approach, AI solutions not only enhance user experience but also improve operational efficiency, helping organizations achieve their strategic goals.
Future trends in conversational AI
Conversational AI is evolving rapidly, unlocking new possibilities for how businesses interact with users. As technology advances, AI systems will become increasingly sophisticated, offering more natural, intuitive interactions. Multimodal communication will integrate text, voice, and visual inputs, creating seamless experiences across devices and platforms.
Emotionally intelligent AI will play a significant role in shaping future interactions. Systems capable of recognizing and responding to emotions will deliver more empathetic experiences, fostering stronger customer connections. Additionally, hyper-personalization will drive deeper engagement, as AI learns from individual user behavior and tailors responses to meet specific needs.
In the near future, conversational AI will also become a core component of the metaverse, providing virtual assistance within immersive digital environments. Businesses investing in these emerging technologies today will be well-positioned to lead the way as conversational AI redefines user experiences across industries.
The path forward with conversational AI
Conversational AI is reshaping the way businesses interact, providing scalable solutions that enhance customer experiences and streamline operations. Its ability to deliver real-time, personalized interactions allows organizations to meet growing user expectations while optimizing their internal processes. Companies that integrate conversational AI today are better positioned to handle the complexities of modern business operations, offering 24/7 support, cost savings, and improved efficiency.
Lumenalta empowers businesses to harness the full potential of AI-driven solutions through our tailored approach. With a focus on solving for outcomes, not just delivering scope, we design intelligent systems that align with your business needs and future goals. Our AI solutions, built with industry expertise and radical engagement, ensure you achieve measurable impact quickly.
Common questions about conversational AI
What is conversational AI and how does it work?
What are some real-world examples of conversational AI?
Why is conversational AI important for businesses today?
How can SaaS businesses benefit from conversational AI?
What are the key challenges of implementing conversational AI?
Want to learn how conversational AI can bring more transparency and trust to your operations?