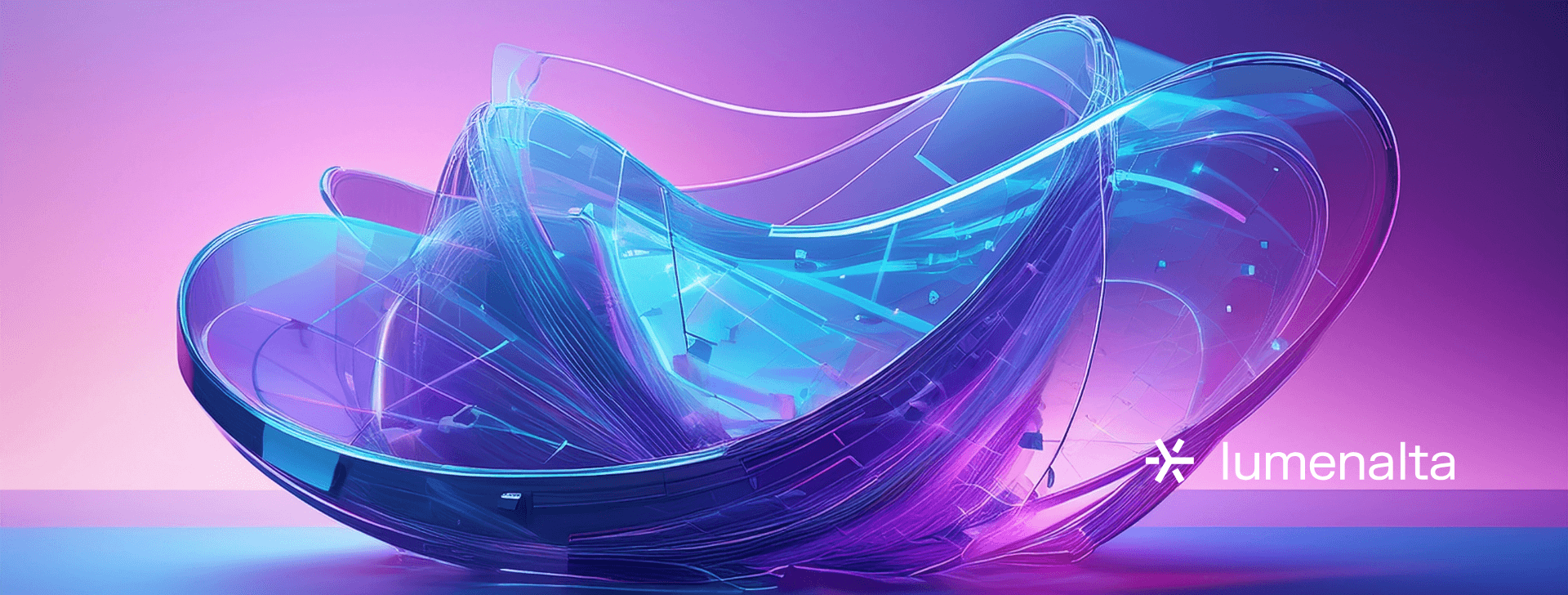
A guide to AI product development in 2025
MAR. 8, 2025
8 Min Read
AI product development is the key to boosting profits, streamlining operations, and staying relevant.
Many organizations see it as a path toward personalized user experiences, improved resource allocation, and stronger risk controls. Practical results stem from consistent planning, data-centric frameworks, and thoughtful investment in the right talent. Adopting these models can be the turning point for organizations that want to innovate efficiently.
key-takeaways
- 1. AI product development relies on well-defined objectives, data handling, and ongoing validation for consistent results.
- 2. Quantifiable metrics, such as conversion rates or user retention, keep teams accountable and focused on impact.
- 3. Generative AI extends beyond text creation to include visual prototyping and rapid concept testing.
- 4. Infrastructure readiness and data accuracy often determine how smoothly new AI initiatives can launch.
- 5. Scalability and iterative refinement require close collaboration between technical staff and key stakeholders.
What is AI product development?
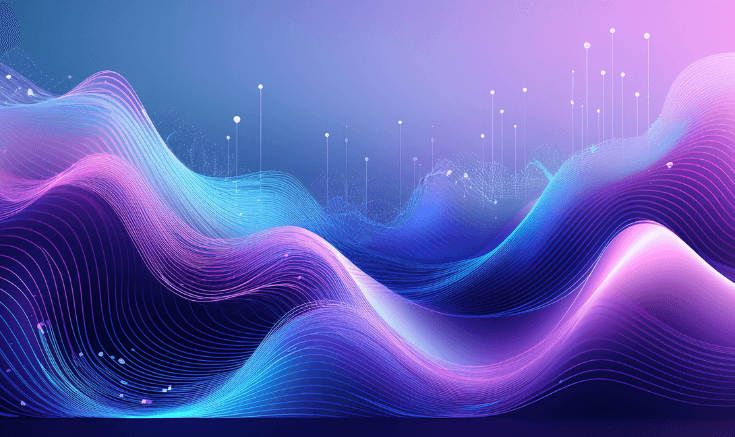
AI product development is the specialized approach of designing, building, and maintaining digital solutions powered by predictive and cognitive algorithms. This strategy involves incorporating data analysis, machine learning (ML), and language models to address real business challenges. Teams often focus on maximizing efficiency, cutting costs, and refining the user experience. Solutions range from advanced recommendation engines in online retail to dynamic forecasting platforms in logistics.
AI-backed product development can provide structured frameworks for iterating on innovative features while reducing overall time to market. Teams often integrate specialized data pipelines and automated workflows, ensuring consistent quality and scalability. This approach can empower leaders in industries such as healthcare and financial services to identify new revenue streams and improve ROI. AI for product development also supports better alignment between cross-functional teams, ensuring that every stakeholder is working toward a shared goal.
"AI product development is the specialized approach of designing, building, and maintaining digital solutions powered by predictive and cognitive algorithms."
Benefits of AI product development
Organizations adopting AI product development often see direct benefits tied to scalability and precision. Leaders are also looking for ways to streamline daily tasks, which this method addresses by using machine-backed processes for faster outcomes. Implementation can bolster decision clarity, allowing you to adjust market strategies based on reliable insights. Developers and stakeholders alike discover that automated pipelines mean fewer bottlenecks and better alignment with business goals.
- Enhanced forecasting: Data-powered models provide accurate predictions of trends and resource demands, paving the way for minimized risks and higher returns.
- Operational efficiency: Automated workloads free up human talent to tackle strategic priorities, improving output without compromising on quality.
- Personalized solutions: Intelligent algorithms adapt to user behaviors, leading to more engaging interactions that build customer loyalty.
- Cost optimization: Streamlined workflows help you reduce expenses, directing budgets toward innovation and growth instead of reactive maintenance.
- Rapid prototyping: Fast iteration cycles accelerate proof-of-concept validation and shorten the AI product development process, saving time and funds.
Each benefit brings tangible improvements to business models. Practical steps like operational automation or tailored recommendations can yield better outcomes across multiple sectors. Forward-thinking leaders often emphasize these advantages when shaping their technology roadmaps. This type of planning creates space for new opportunities and ensures that progress stays aligned with strategic objectives.
Key steps in the AI product development process
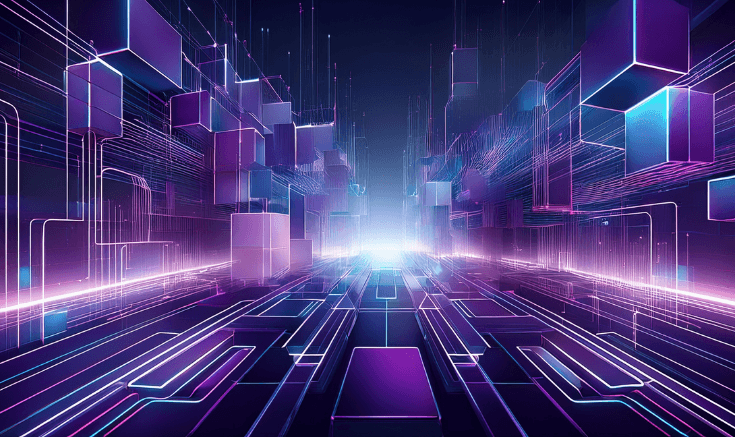
Effective AI product development process planning depends on detailed requirements gathering and a proactive mindset. This approach considers data sources, stakeholder expectations, and timelines from the outset. Comprehensive analysis allows you to pinpoint clear goals that align with broader business metrics. Clarity around objectives serves as a strong foundation before launching any new initiative.
Breakdowns in planning often stem from insufficient communication between departments and a lack of structured procedures. Early identification of potential pitfalls helps you reduce delays later. Careful orchestration across data scientists, engineers, and product managers fosters a cycle of continuous improvement. Each phase can be managed through consistent feedback loops that reveal where to refine resource allocation.
Set clear metrics and objectives
Teams at the start of AI for product development often define what success looks like through quantifiable metrics, such as reduced churn or higher conversion rates. This process involves mapping each metric to the business priority it represents, ensuring practical alignment. Leaders usually consult cross-functional colleagues to confirm that targets are realistic and reflective of user needs. Early collaboration lowers the risk of misaligned goals that might stall long-term progress.
Collect relevant data
Gathering data from both internal and external sources creates a robust foundation for any model. Structured archives such as CRM systems, as well as unstructured data from social media or call logs, often serve as the core of many advanced solutions. Data engineering teams transform raw inputs into standardized formats to ensure consistency. Ongoing data validation is essential for preventing inaccuracies that may skew downstream results.
Build and validate models
Development teams experiment with various approaches, including neural networks, decision trees, or specialized LLM product development frameworks. Each algorithm is tested against known datasets to check for accuracy, precision, and recall. Smaller pilot runs help refine parameters before a broader rollout. This stage often involves multiple iterations that foster improved outcomes and resource efficiency.
Integrate with existing infrastructure
Aligning new models with current platforms and data pipelines can be complex, but systematic planning streamlines the process. Some organizations rely on containerization or microservices to keep deployments flexible and easily scalable. Clear governance policies prevent confusion over version control and resource allocation. Thorough documentation also supports future enhancements, making it simpler to iterate on features over time.
Iterate and monitor performance
Analytics dashboards track metrics such as user adoption and cost savings, highlighting real-time insights for product owners. Continual monitoring reveals subtle shifts in data distributions that might undermine system performance. Routine evaluations guide updates to underlying models or processes, keeping solutions aligned with business goals. Long-term success often hinges on refining these systems based on periodic reviews and direct feedback from stakeholders.
Challenges with AI and product development
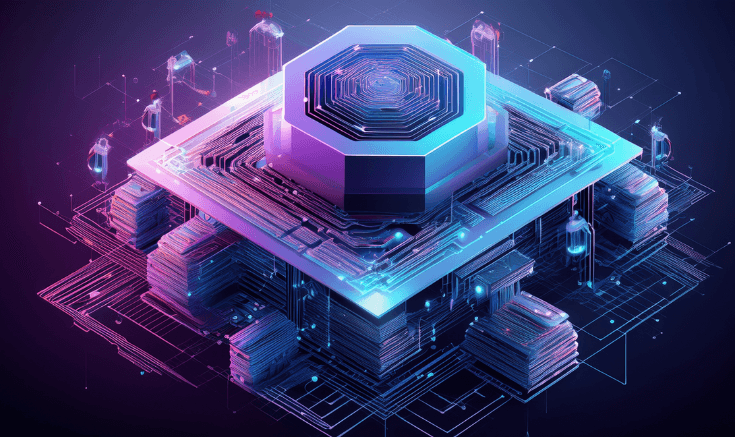
Adopting AI and product development can deliver remarkable returns, but there are obstacles that might limit outcomes. Some companies face skill gaps, while others struggle with large-scale data management or regulatory constraints. Shifting priorities within management teams can also interrupt resource allocation, leading to delayed features and budget overruns. Proactive planning and an understanding of potential risks can soften many of these challenges.
- Regulatory complexity: Different regions enforce varying privacy and security requirements, adding layers of compliance steps.
- Data quality issues: Inaccurate or incomplete data can undermine model reliability and skew any resulting predictions.
- Infrastructure readiness: Legacy systems often need modernization before advanced solutions can be deployed effectively.
- Talent shortage: Experts with specialized AI knowledge are often scarce, raising costs and limiting hiring options.
- Resistance to change: Stakeholders may hesitate to replace traditional approaches, slowing overall momentum.
Addressing each challenge head-on can save valuable resources and ensure consistent progress in product development AI initiatives. Thoughtful project management often includes ongoing training, robust documentation, and incremental feature rollouts. Teams that prioritize internal education and clarity around objectives tend to avoid many pitfalls. This type of preparation sets a strong foundation for building advanced capabilities.
Tools for AI product development
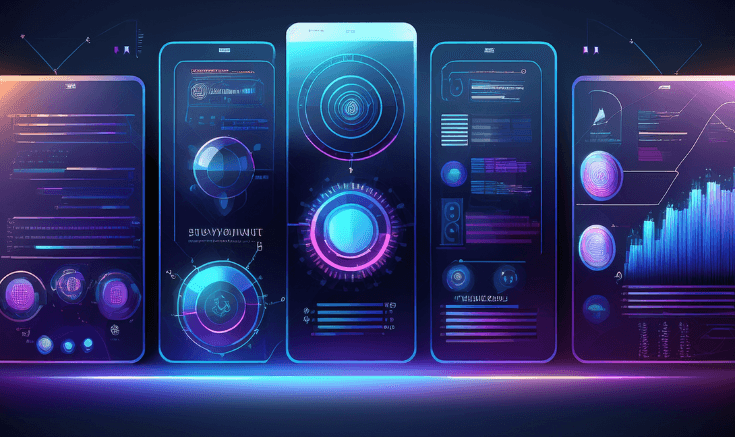
A variety of AI tools for product development empower teams to streamline workflows and maintain quality. Platforms designed for data engineering, such as Apache Spark or similar open-source frameworks, handle large volumes of information with minimal latency. DevOps pipelines customized for ML allow continuous integration and deployment, helping teams release new features more quickly. GUI-based interfaces also let non-technical stakeholders run tests or review analytics without writing code, lowering collaboration barriers.
Model optimization frameworks often come with prebuilt modules for tasks like hyperparameter tuning, making it simpler to iterate on performance. Product development AI tools also include specialized solutions for real-time inference, letting businesses integrate predictive capabilities into customer-facing applications. Collaboration features within these platforms allow multiple departments to provide input, ensuring a balanced approach to risk and reward. Many organizations adopt both open-source and commercial solutions, aiming to maximize flexibility while containing operational costs.
Generative AI in product development
Generative AI in product development focuses on models that create text, images, or other content based on patterns learned from varied datasets. This discipline can reshape how products are designed and tested, offering concepts that might not be immediately apparent through manual methods. Teams often use these advanced techniques to refine user interfaces, branding assets, or personalized recommendations. Generative AI product development demands careful curation of source data to maintain originality and relevance.
Content generation for user engagement
Natural language systems can produce marketing copy, chat responses, or even draft emails that resonate with target audiences. This approach saves time and resources, particularly for businesses handling a high volume of customer queries. Tailoring text outputs to specific industries ensures that messaging remains accurate and brand-consistent. Analysts commonly track click-through rates or user satisfaction to measure how well these generative models perform.
Design prototyping and creative assets
Visual-based generative models advance the design phase by swiftly producing mock-ups for websites, apps, or product packaging. Designers can then select from machine-created samples and refine them to align with brand guidelines. This method offers a fresh perspective that sometimes sparks new ideas, reducing the time spent in trial-and-error. Many creative teams find that generative capabilities open pathways to aesthetic innovation without overshooting budgets.
Iterative concept testing
Enterprises often run parallel tests of generative outputs, selecting the variations that resonate best with specific user segments. Granular feedback loops indicate which design elements or text patterns yield higher conversions or brand loyalty. This ongoing refinement process reduces guesswork, especially when tackling new markets or rapidly shifting consumer tastes. Creative teams integrate these insights into future releases, supporting a continuous cycle of innovation.
“Generative AI product development demands careful curation of source data to maintain originality and relevance.”
Strategies for adopting AI product development
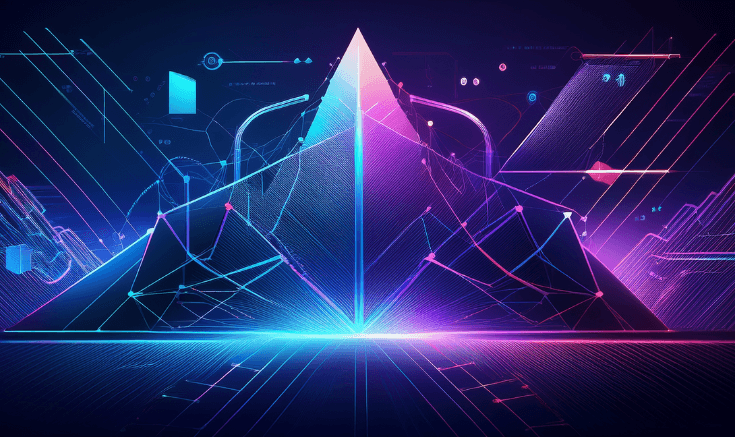
Execution often starts with a clear roadmap that identifies specific goals, budgets, and timelines. Many leaders pilot smaller initiatives first, demonstrating tangible impact to upper management before larger investments. Cross-functional workshops help clarify scope and keep each department aligned around desired outcomes. A measured pace also allows for mid-project assessments that catch inefficiencies early and support quick course corrections.
Implementation should be accompanied by robust change management, ensuring that teams feel comfortable with newly introduced processes. Clear guidelines around privacy, compliance, and data handling encourage trust among stakeholders. Ongoing education can drive continuous improvement, especially as staff members refine their skills in specialized areas like data science or advanced analytics. Each of these actions leads to better risk mitigation and measurable business gains.
AI product development can accelerate revenue growth, optimize resources, and foster greater adaptability across business functions. This approach supports better alignment between technical capabilities and strategic targets, creating a foundation for ongoing success. At Lumenalta, we focus on building tailored AI solutions that address real challenges and deliver measurable returns. Let’s chart a brighter path.
table-of-contents
- What is AI product development?
- Benefits of AI product development
- Key steps in the AI product development process
- Challenges with AI and product development
- Tools for AI product development
- Generative AI in product development
- Strategies for adopting AI product development
- Common questions about AI product development
Common questions about AI product development
How do businesses start with AI product development?
What role do large language models play in product development AI?
Which industries benefit most from AI-based products?
How can AI product development help with cost control?
What is the most common challenge when scaling product development AI solutions?
Want to learn how AI can bring more transparency and trust to your operations?