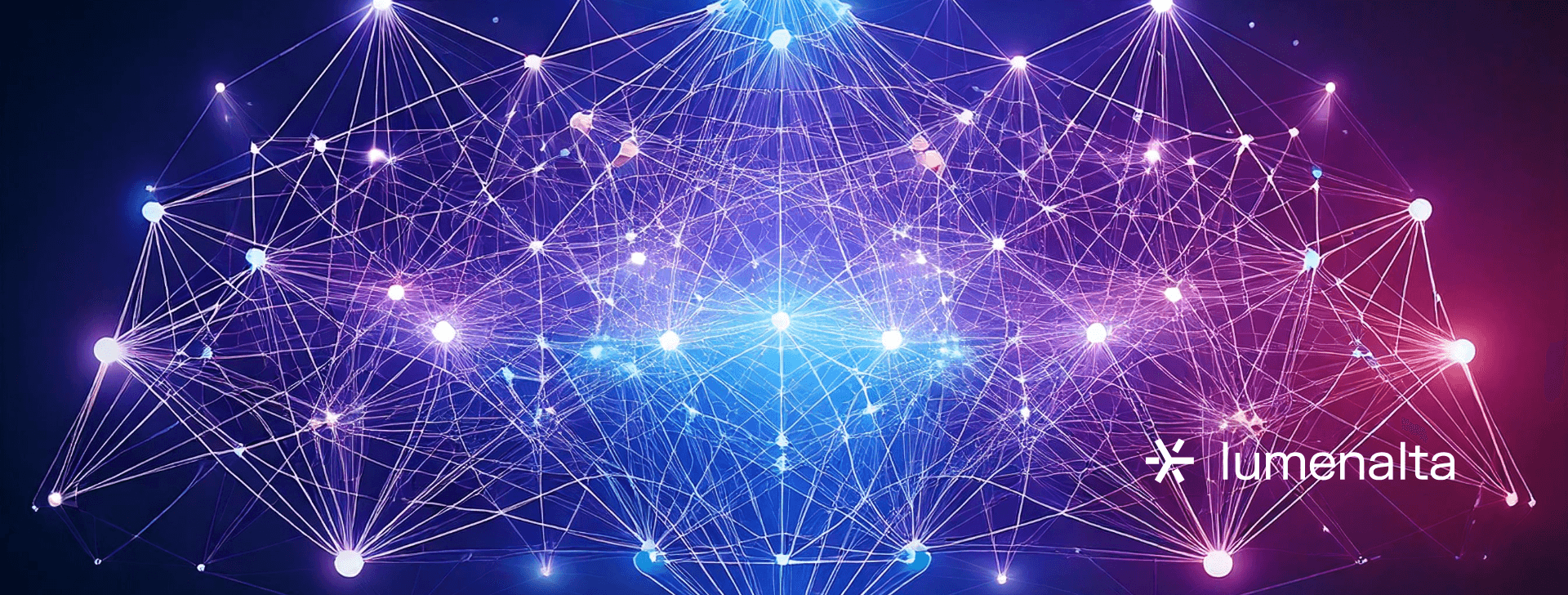
What is machine learning (ML)?
DEC. 6, 2024
6 Min Read
Machine learning revolutionizes how businesses approach challenges, reveal opportunities, and drive measurable success.
Its ability to process vast datasets, adapt to fluid needs, and deliver precise insights positions it as a cornerstone of modern innovation. As organizations turn to data-centric strategies, understanding machine learning’s capabilities is critical for unlocking scalable, impactful, and future-proof solutions.
Key takeaways
- 1. Machine learning processes large datasets to deliver precise insights, automating workflows and improving decision-making across industries.
- 2. Distinct categories of machine learning—supervised, unsupervised, and reinforcement learning—offer solutions tailored to specific challenges and goals.
- 3. Deep learning, a subset of machine learning, leverages neural networks for handling unstructured data, driving advancements in fields like AI and robotics.
- 4. Successful implementation depends on clear objectives, robust data infrastructure, and seamless integration with existing systems.
- 5. Measuring machine learning’s impact through KPIs ensures businesses achieve scalable, cost-effective, and future-proof outcomes.
What is machine learning?
Machine learning is a powerful subset of artificial intelligence focused on enabling systems to analyze data, identify patterns, and make decisions with minimal human intervention. Instead of being explicitly programmed with predefined rules, these systems leverage algorithms that adapt and improve over time. This approach helps businesses and industries tackle challenges in real time and at scale.
The meaning of machine learning lies in its ability to transform raw data into actionable insights, revealing untapped potential across various sectors. It automates decision processes, offering precise and reliable results that maximize efficiency while minimizing resource usage. From enhancing operational workflows to enabling smarter forecasting, machine learning ensures faster paths to value.
The definition of machine learning revolves around its adaptability. Algorithms are trained on historical data and adjusted as new information becomes available, making the process both dynamic and future-proof. This capability reduces the time and effort needed to develop scalable solutions, aligning with the growing demand for cost-effective and impactful technologies.
Machine learning operations prioritize measurable business outcomes. Either improving supply chain efficiency, reducing customer churn, or enabling personalized recommendations, machine learning consistently delivers value. Integrating seamlessly into existing systems helps machine learning support change management, foster stakeholder alignment, and ensure long-term success.
“Machine learning operates on a foundational principle: Enabling systems to learn from data and refine their performance over time without explicit programming.”
How machine learning works
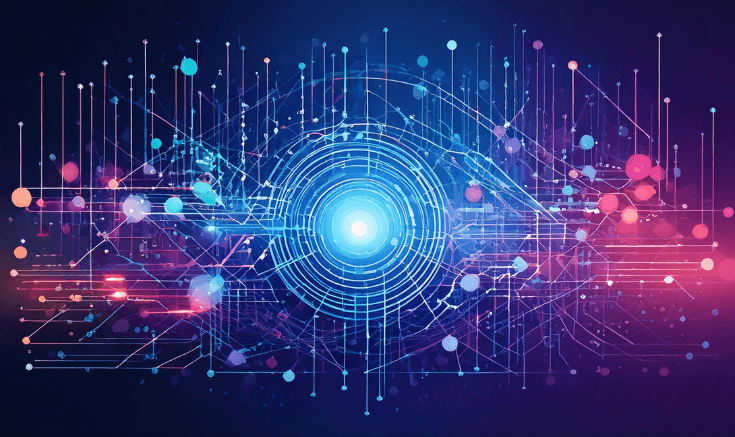
Machine learning operates on a foundational principle: Enabling systems to learn from data and refine their performance over time without explicit programming. This process begins with feeding algorithms structured or unstructured datasets, which the system analyzes to display patterns and relationships. Once patterns are detected, the system applies its findings to make predictions or automate decisions, continuously adjusting its performance as new data becomes available.
The effectiveness of machine learning lies in its structured workflow. It starts with data collection and preparation, a stage focused on aggregating high-quality data for training the algorithms. Algorithms then process this data through training models, where patterns are learned. Testing and validation ensure these models can generalize to new data, minimizing errors and biases.
Machine learning frameworks are designed to adapt dynamically, supporting scalability and real-time responsiveness. This adaptability reduces the time required for implementation, delivering faster paths to measurable results. Seamless integration into existing infrastructure ensures alignment with business strategies, improving overall efficiency and unlocking untapped potential.
Tailored for practical applications, this technology supports cost-effective scaling and delivers actionable insights. Machine learning accelerates innovation while maximizing returns on investment.
3 types of machine learning
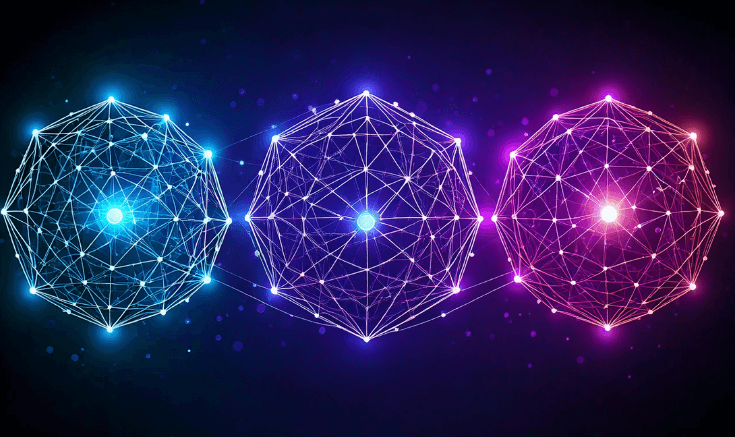
Machine learning operates through different learning techniques, each addressing distinct challenges and opportunities. These methods—supervised, unsupervised, and reinforcement learning—form the foundation of its capabilities. Either used to analyze historical data, identify trends, or improve decisions, these techniques help businesses achieve results that are both cost-effective and scalable. Choosing the right type of machine learning ensures solutions are tailored to meet industry-specific needs, offering speed to value and measurable outcomes.
Each machine learning type is designed to solve specific types of problems and delivers unique benefits:
1. Supervised learning
Models learn from labeled data, where inputs are paired with known outputs. This approach excels in scenarios where precision is crucial, such as fraud detection, predictive maintenance, and customer churn prediction. Supervised learning ensures reliability and consistency, particularly in applications requiring clearly defined outcomes.
2. Unsupervised learning
Models identify patterns or clusters in unlabeled data. Unsupervised learning is ideal for exploring unknown relationships in data, such as identifying customer segments or detecting anomalies in financial transactions. This method enables organizations to expose untapped potential, fostering innovation and competitive differentiation.
3. Reinforcement learning
Models improve through a feedback system of rewards and penalties, simulating trial-and-error learning. Commonly applied in robotics, supply chain optimization, and autonomous systems, reinforcement learning performs well in settings requiring dynamic adaptability.
Understanding these methods allows businesses to deploy machine learning strategically, unlocking value across diverse domains. Supervised learning drives operational accuracy, unsupervised learning reveals hidden opportunities, and reinforcement learning enhances continuous improvement. Together, these techniques empower organizations to utilize data, optimize performance, and achieve sustainable growth while aligning seamlessly with broader business strategies.
Machine learning applications and use cases
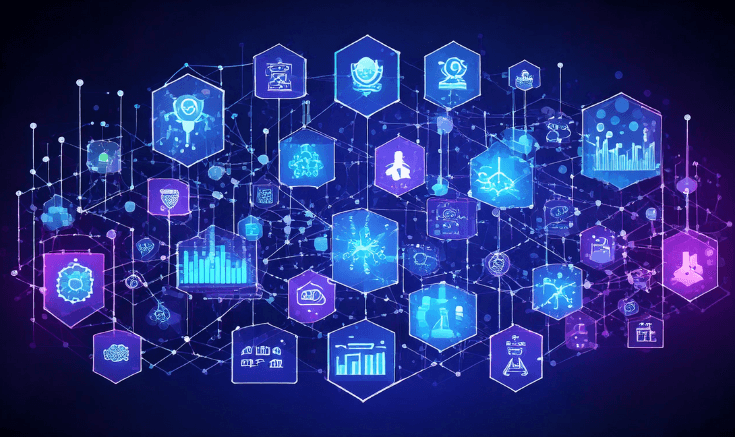
Machine learning continues to transform industries by addressing complex challenges with precision and adaptability. Its applications span varied sectors, driving innovation and improving efficiency across critical operations. Businesses leveraging machine learning benefit from faster decisions, streamlined processes, and enhanced customer experiences. Below are some of the most impactful use cases, illustrating the breadth of its capabilities.
Fraud detection and security
Machine learning excels in identifying suspicious patterns and anomalies in financial transactions. Algorithms analyze vast datasets in real time, flagging irregularities that indicate fraudulent behavior. Financial institutions use machine learning to reduce losses, protect assets, and maintain customer trust. This application strengthens operational security while optimizing response times and minimizing risks.
Personalized customer experiences
Retailers and online platforms rely on machine learning to deliver tailored recommendations and improve customer engagement. Algorithms analyze purchase histories, browsing patterns, and preferences to create personalized shopping experiences. This approach boosts customer retention, increases revenue, and exposes new growth opportunities through deeper audience insights.
Predictive maintenance in industrial operations
Manufacturing and logistics industries deploy machine learning to anticipate equipment failures and reduce downtime. Predictive models analyze operational data to identify signs of wear or inefficiency, enabling proactive maintenance. This reduces repair costs, extends equipment lifespan, and improves overall operational efficiency.
Supply chain optimization
Supply chain operations benefit significantly from machine learning’s ability to manage inventory, forecast demand, and streamline distribution. Algorithms analyze variables like consumer demand, production schedules, and delivery timelines, ensuring smooth operations and cost savings. Machine learning optimizes resource allocation, improving speed and accuracy across the supply chain.
Healthcare diagnostics and treatment planning
Machine learning supports healthcare professionals with precise diagnostic tools and personalized treatment recommendations. Models process patient data to identify potential conditions or suggest therapies based on historical outcomes. This enhances the quality of care, reduces diagnostic errors, and accelerates recovery times.
Autonomous vehicles and transportation
Self-driving vehicles depend on machine learning for object detection and navigation. Algorithms process real-time data from sensors and cameras, ensuring safety and efficiency on the road. This application reduces human error, lowers transportation costs, and promotes sustainable mobility solutions.
Machine learning adapts to various business conditions, offering solutions that are scalable and impactful. From securing financial systems to enhancing customer experiences, its applications provide measurable benefits that align with organizational goals. Whether optimizing operations or driving innovation, machine learning is reshaping industries with efficiency, precision, and forward-thinking strategies.
“Machine learning offers a scalable entry point for organizations focusing on operational efficiency and faster time to value, while deep learning drives advanced innovation.”
7 benefits of machine learning
Machine learning offers transformative benefits that drive measurable impact across industries. Its ability to process large datasets, generate actionable insights, and adapt to evolving needs positions it as a cornerstone of modern business strategies. Organizations adopting machine learning solutions achieve faster results, reduce costs, and unlock new growth opportunities. Below are seven critical benefits, each highlighting how machine learning addresses key business priorities.
- Improved decisions: Machine learning models analyze data patterns to deliver precise, data-backed insights. This reduces reliance on intuition, leading to better strategic choices. Businesses leverage this advantage to minimize risks and enhance operational accuracy, ensuring that decisions align with measurable goals and long-term growth.
- Cost efficiency through automation: Automating repetitive tasks with machine learning reduces operational overhead while increasing efficiency. Processes such as data entry, customer support, and quality assurance benefit from faster execution and fewer errors. This scalability creates opportunities for cost-effective growth and resource optimization.
- Enhanced customer experiences: Personalization powered by machine learning delivers tailored recommendations and improved engagement. From retail to entertainment, businesses use these insights to deepen customer loyalty and drive revenue. Customized solutions resonate more effectively with audiences, ensuring a sustainable market presence.
- Predictive analytics for operational planning: Forecasting tools driven by machine learning provide actionable insights into future trends and demands. Industries like logistics, healthcare, and finance use these predictions to allocate resources effectively, ensuring smooth operations and improved outcomes.
- Scalability and adaptability: Machine learning solutions adapt seamlessly to growing data volumes and business conditions. Models trained on initial datasets can handle increasing complexity, future-proofing operations while minimizing disruptions. This flexibility supports long-term growth without frequent system overhauls.
- Enhanced security and fraud detection: Real-time monitoring powered by machine learning identifies threats and irregularities faster than traditional methods. Financial institutions and e-commerce platforms rely on these capabilities to safeguard assets, prevent fraud, and maintain trust. This proactive approach reduces risks and protects sensitive information.
- Accelerated research and innovation: Machine learning unlocks new possibilities in research and development. Its capacity to process complex datasets enables breakthroughs in medicine, technology, and environmental science. Faster iterations and discoveries position organizations as leaders in their fields, maximizing returns on innovation investments.
Machine learning enables businesses to address challenges with precision and efficiency. Each benefit reinforces its value as a strategic investment, offering outcomes that are scalable, future-proof, and aligned with measurable goals. By integrating these solutions into existing workflows, organizations can unlock their full potential, fostering sustained success and market leadership.
Machine learning vs deep learning
The main differences between machine learning vs deep learning lie in their approach, complexity, and data requirements. Machine learning uses algorithms to analyze data and improve performance based on defined rules and patterns, while deep learning employs artificial neural networks that simulate human brain functionality. Machine learning works effectively with structured data and smaller datasets, providing faster results with less computational effort. Deep learning, on the other hand, requires large datasets and significant processing power to handle unstructured data like images, speech, and videos.
Machine learning excels in scenarios that demand quick, actionable insights and operational efficiency. It is commonly used in applications such as fraud detection, predictive analytics, and quality assurance. Deep learning addresses more intricate challenges, such as natural language processing and autonomous vehicle systems, where precise and complex computations are essential.
Understanding the distinctions helps businesses determine which approach best aligns with their goals. Machine learning supports faster implementation and scalability, making it ideal for organizations looking to maximize returns within constrained budgets. Deep learning drives breakthrough innovations, catering to industries requiring advanced problem-solving capabilities. Both methods ensure measurable impact, enabling businesses to unlock new potential.
Machine learning vs artificial intelligence
The main differences between machine learning and artificial intelligence lie in their scope and application. Artificial intelligence refers to the overarching goal of creating systems that simulate human intelligence, enabling tasks such as reasoning, problem-solving, and perception. Machine learning, as a subset of artificial intelligence, focuses on the development of algorithms that allow systems to learn from data and improve performance over time.
Artificial intelligence encompasses a broader range of capabilities, including rule-based systems, robotics, and advanced decisions. Machine learning provides a specific pathway for achieving these goals by processing data to display patterns, predictions, and actionable insights. For example, while artificial intelligence might aim to create a conversational chatbot, machine learning would handle the data processing required to make the chatbot's responses more relevant and personalized.
These differences inform the strategic use of each technology. Machine learning supports immediate, measurable goals, offering faster time to value for businesses optimizing processes like fraud detection or customer segmentation. Artificial intelligence projects often involve broader innovation, focusing on transformative capabilities like autonomous systems or advanced problem-solving.
Organizations benefit most when these technologies are deployed together, balancing short-term gains with long-term vision. Machine learning ensures scalable and efficient operations, while artificial intelligence drives future-proof strategies and groundbreaking advancements. This alignment helps maximize returns and improve adaptability.
Implementing machine learning strategies
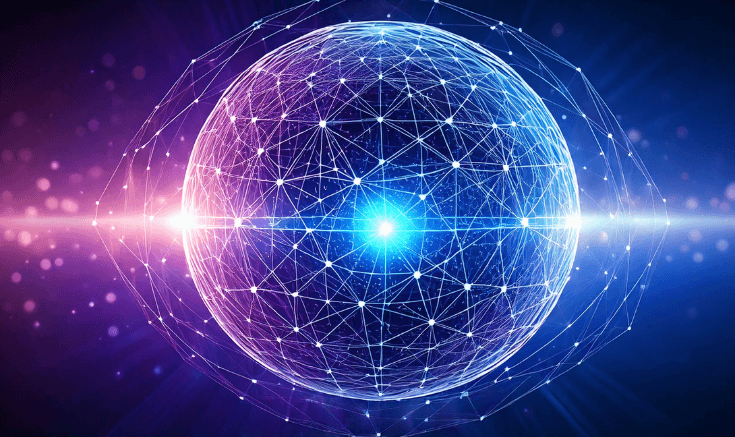
Successfully implementing machine learning strategies requires a structured approach that aligns technical capabilities with business objectives. Organizations must prioritize clear goals, robust data management, and seamless integration to maximize machine learning's potential. A well-executed strategy accelerates time to value, supports scalability, and ensures measurable outcomes across various operational areas.
Identifying use cases and setting goals
The first step in implementing machine learning involves identifying specific business challenges or opportunities that machine learning can address. These may include improving demand forecasting, streamlining customer support, or optimizing supply chain logistics. Defining measurable goals, such as cost reduction or increased revenue, ensures alignment with broader business priorities. Focusing on use cases with high ROI potential enables organizations to deliver impact efficiently while addressing stakeholders’ needs.
Building and managing data infrastructure
High-quality data forms the foundation of effective machine learning strategies. Establishing robust data collection, storage, and processing systems ensures that models are trained with accurate and relevant information. Data infrastructure should be scalable, secure, and aligned with governance standards to support seamless integration into existing workflows. Effective data management enhances decision capabilities and reduces time spent on manual adjustments.
Choosing the right algorithms and tools
Selecting appropriate machine learning models and tools depends on the complexity of the problem and the type of data available. Supervised learning models may suit tasks requiring clear outputs, while unsupervised learning is better for exploratory data analysis. Leveraging cloud-based platforms or custom-built solutions can further accelerate deployment and ensure cost efficiency. Tailoring algorithms to fit business needs fosters scalable, future-proof operations.
Driving change management and stakeholder alignment
Implementing machine learning often requires a shift in organizational processes and culture. Ensuring buy-in from stakeholders across departments is critical to success. Transparent communication about machine learning’s benefits, risks, and expected outcomes fosters trust and collaboration. Training programs and regular updates can help teams adapt to new workflows, ensuring smooth adoption and sustained value creation.
A thoughtful implementation strategy transforms machine learning from a technical concept into a practical tool for business growth. By focusing on use case alignment, strong data infrastructure, and collaborative change management, organizations can unlock measurable benefits. These strategies help drive innovation and set the stage for scalable, impactful machine learning solutions.
Measuring business impact of machine learning
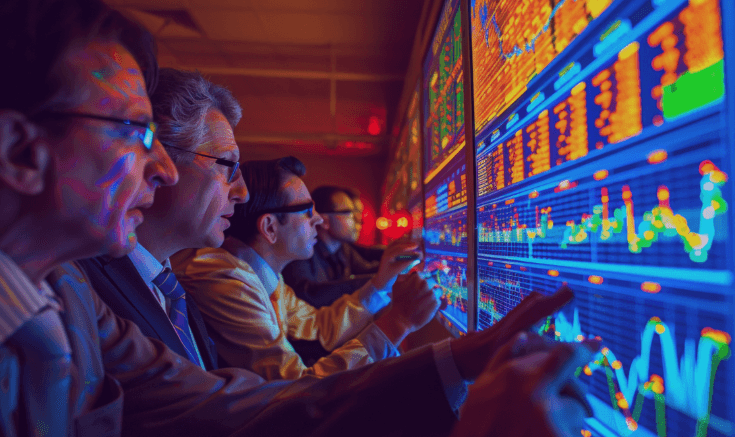
The true value of machine learning lies in its ability to deliver measurable results that align with organizational goals. Businesses need to evaluate these impacts systematically, ensuring machine learning implementations drive efficiency, improve scalability, and generate tangible returns. A clear measurement framework helps decision-makers understand the outcomes of their investments and optimize strategies for long-term success.
- Operational efficiency: Measuring reductions in processing time, error rates, or resource usage highlights the practical benefits machine learning delivers. Improved efficiency translates into cost savings and faster time to value.
- Revenue growth: Tracking revenue streams influenced by machine learning, such as upselling through personalized recommendations or increasing conversions from predictive analytics, showcases its direct financial impact.
- Customer satisfaction: Metrics like Net Promoter Score (NPS), customer retention rates, or average resolution times reflect how machine learning enhances user experiences and strengthens brand loyalty.
- Cost reduction: Machine learning’s role in automating repetitive tasks or optimizing resource allocation can be quantified in reduced labor or operational expenses.
- Scalability: Analyzing the system’s ability to handle growing data volumes or use cases demonstrates its adaptability and future-proof potential.
- Accuracy improvements: Comparing the accuracy of machine learning models to traditional approaches ensures decision is data-driven and reliable.
- Time to value: Measuring the speed at which machine learning implementations deliver actionable insights or operational improvements provides clarity on ROI.
Machine learning’s success is not limited to isolated achievements; its value compounds through continuous refinement and integration. Businesses gain an edge when they consistently evaluate and adapt their strategies, ensuring alignment with shifting market demands and operational goals. A robust measurement framework guarantees that machine learning investments are impactful, scalable, and geared toward maximizing both immediate and long-term returns.
Machine learning isn’t just a technological advancement—it’s a powerful tool for transforming data into impactful decisions and strategies. From automating workflows to exposing actionable insights, it empowers organizations to scale, innovate, and lead with confidence. At Lumenalta, we specialize in creating tailored machine learning solutions that align with your goals, delivering real value and measurable outcomes.
Let’s shape the future of your business together.
table-of-contents
- What is machine learning?
- How machine learning works
- 3 types of machine learning
- Machine learning applications and use cases
- 7 benefits of machine learning
- Machine learning vs deep learning
- Machine learning vs artificial intelligence
- Implementing machine learning strategies
- Measuring business impact of machine learning
- Common questions about machine learning
Common questions about machine learning
What is the basic meaning of machine learning?
How is machine learning applied in businesses?
What are the key differences between machine learning and deep learning?
Why is machine learning important for scalability?
How do businesses measure the success of machine learning projects?
Want to learn how machine learning can bring more transparency and trust to your operations?