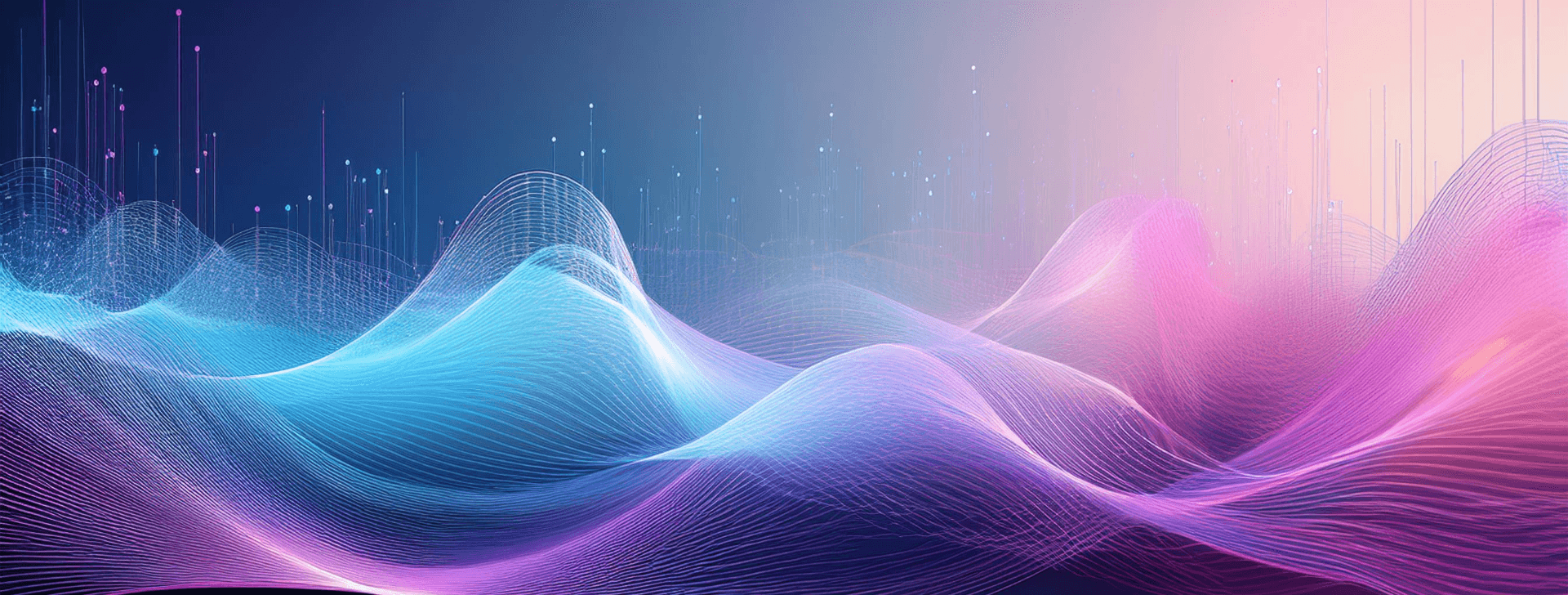
What is data and analytics?
NOV. 19, 2024
8 Min Read
Unlock the potential of your data to drive smarter, faster, and more impactful decisions.
Data and analytics are the strategic engines powering today’s businesses, enabling them to uncover hidden patterns, predict outcomes, and make smarter decisions. By transforming raw data into actionable insights, organizations can anticipate market shifts, optimize operations, and stay ahead of the competition.
Key takeaways
- 1. Data and analytics are essential for data-driven decision-making, helping businesses understand trends, optimize operations, and enhance customer relationships.
- 2. The four main types of analytics—descriptive, diagnostic, predictive, and prescriptive—each play unique roles in guiding strategic and proactive business actions.
- 3. Big data analytics enables the processing of massive, unstructured data in real time, allowing companies to respond swiftly to market changes and customer needs.
- 4. A structured data analytics process ensures quality insights, involving data collection, storage, processing, cleansing, and analysis for comprehensive results.
- 5. The future of data and analytics lies in AI and emerging trends like edge computing and real-time analytics, which will drive faster and more accurate insights.
Understanding data and analytics
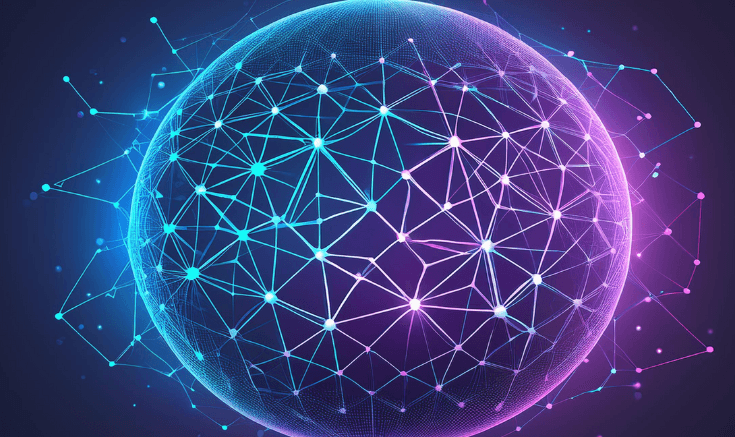
Data analytics involves the systematic examination of raw data to extract patterns, correlations, and meaningful insights that drive better decision-making. This process utilizes a combination of computational techniques, statistical methods, and machine learning to convert unstructured data into structured, valuable information. The goal is to provide businesses with a deeper understanding of their operations, customer behaviors, and market dynamics, ultimately enhancing their ability to react strategically.
Key components of data analytics include:
- Data mining: Extracting useful patterns and trends from large datasets.
- Statistical analysis: Employing mathematical techniques to interpret data and validate insights.
- Predictive modeling: Using historical data to predict future outcomes, allowing companies to prepare for potential scenarios.
- Visualization: Representing data insights visually to improve interpretation and communication of findings across teams.
Data analytics spans across both structured and unstructured data formats. Structured data typically resides in relational databases and includes highly organized information such as customer profiles, transaction histories, and operational metrics. In contrast, unstructured data includes social media posts, emails, and other informal data sources, which require specialized techniques to analyze effectively. By making sense of this diverse data landscape, analytics enables organizations to navigate complex business environments with clarity and confidence.
"Data analytics involves the systematic examination of raw data to extract patterns, correlations, and meaningful insights that drive better decision-making."
Importance of data and analytics
For companies striving to stay relevant and competitive, data and analytics are invaluable. By transforming raw data into actionable insights, analytics enables organizations to respond to market changes swiftly, enhance their operational efficiency, and strengthen customer relationships. A data-driven approach to business decision-making not only improves immediate outcomes but also lays the groundwork for long-term growth and innovation.
The importance of data and analytics can be summarized in the following ways:
- Identifying and acting on emerging trends in real time: Analytics empowers businesses to keep a pulse on current trends, helping them adapt quickly to shifts in market demand, customer preferences, or competitor activities. Retailers, for instance, can adjust inventory and pricing dynamically based on real-time sales data to optimize stock levels and maximize sales.
- Driving personalized customer experiences through tailored solutions: Data analytics allows businesses to build detailed customer profiles and understand individual preferences, enabling them to offer personalized products, services, and marketing messages. This approach fosters customer loyalty and improves satisfaction by making interactions more relevant and valuable.
- Optimizing operations by uncovering inefficiencies and streamlining processes: Analytics can reveal areas where resources are underutilized, processes are bottlenecked, or operations are overly costly. For example, in manufacturing, analytics might identify delays in the supply chain, allowing businesses to adjust processes, reduce waste, and improve delivery times.
- Enhancing risk management by predicting potential issues: Predictive analytics is crucial for identifying potential risks before they escalate. For financial institutions, this might mean detecting unusual transaction patterns to prevent fraud, while for healthcare providers, it might involve predicting patient health risks to improve care outcomes. By proactively managing risks, organizations can protect themselves from costly disruptions and maintain stability in uncertain conditions.
In sum, data and analytics are not just tools for understanding the past; they are vital assets for anticipating the future, ensuring businesses can act with confidence and foresight.
Types of data analytics
Different types of data analytics enable businesses to gain insights at various stages, from understanding historical trends to predicting future outcomes and recommending the best actions. Each type has a specific focus and application, and together, they provide a holistic view that empowers organizations to make well-informed, proactive decisions.
Descriptive analytics
Descriptive analytics is the foundational layer of data analysis, centered on summarizing historical data to reveal what has already happened. This type of analytics involves the use of basic statistical methods such as averages, percentages, and frequencies to interpret past performance. By highlighting key trends, patterns, and metrics, descriptive analytics helps companies understand how they performed in different areas and establish a benchmark for evaluating progress over time.
Applications of descriptive analytics include:
- Financial reports: Summarizing revenue, profit, expenses, and other key financial metrics over time to evaluate business performance.
- Customer analytics: Identifying common customer demographics and behaviors based on past sales data.
- Operational metrics: Analyzing production rates, inventory levels, or service response times to monitor efficiency and productivity.
Descriptive analytics is essential for establishing a clear baseline, allowing companies to use data as a factual reference for strategic planning and performance assessment.
Diagnostic analytics
Diagnostic analytics builds upon descriptive analytics by exploring why certain events or trends occurred. It goes beyond identifying “what happened” to investigate the factors and dependencies that influenced these outcomes. This is achieved by applying techniques such as drill-down, data discovery, and correlation analysis to uncover root causes and explain underlying drivers.
For example:
- Marketing attribution: Determining which channels contributed most effectively to conversions, helping marketers allocate resources more effectively.
- Churn analysis: Understanding why customers have discontinued their subscriptions or purchases by examining interactions, complaints, and behavior patterns.
- Product performance: Diagnosing why certain products perform better in specific markets, regions, or demographics.
Diagnostic analytics provides context, enabling companies to refine their strategies based on a deeper understanding of causative factors. This level of insight is invaluable for making strategic adjustments and preventing the recurrence of negative outcomes.
Predictive analytics
Predictive analytics uses historical data, statistical algorithms, and machine learning models to forecast future outcomes. By identifying patterns and trends in past data, predictive analytics can project likely future trends, allowing organizations to take proactive steps and prepare for potential challenges or opportunities.
Common applications include:
- Demand forecasting: Retailers can predict future demand for products, enabling better inventory management and reducing stockouts or excess stock.
- Risk assessment: Banks and insurance companies assess the likelihood of defaults or claims by analyzing customer credit histories and behavior.
- Predictive maintenance: Manufacturing firms use predictive analytics to anticipate equipment failures before they happen, minimizing downtime and reducing repair costs.
Predictive analytics offers companies a forward-looking perspective, helping them anticipate changes, optimize resources, and act decisively in dynamic environments.
Prescriptive analytics
Prescriptive analytics takes the insights gained from predictive models a step further, providing specific recommendations on the actions an organization should take to achieve desired outcomes. This type of analytics integrates predictive insights with optimization algorithms and simulations to evaluate potential strategies, assess risks, and suggest optimal courses of action.
Examples of prescriptive analytics include:
- Dynamic pricing: E-commerce platforms use prescriptive analytics to adjust prices in real time based on supply, demand, competitor pricing, and consumer behavior.
- Supply chain optimization: Logistics companies identify the most efficient routes, inventory levels, and transportation methods to minimize costs while meeting demand.
- Healthcare treatment plans: Prescriptive analytics can suggest personalized treatment paths based on patient health data, optimizing care and reducing treatment costs.
Prescriptive analytics is particularly valuable in complex decision-making scenarios where multiple factors must be weighed to find the best possible solution. It helps organizations make calculated decisions by balancing potential risks with rewards, ultimately supporting more effective and impactful strategies.
Together, these types of data analytics allow organizations to interpret past data, understand root causes, forecast future trends, and make strategic, data-backed decisions. By leveraging all four types, businesses can transform raw data into comprehensive insights that guide them through both immediate and long-term planning.
Big data analytics
What is big data?
Big data refers to massive, complex data sets generated at high velocity, which are challenging to process using traditional methods. It includes data from social media, IoT devices, and other digital interactions, providing a rich source of insights for organizations.
Difference between traditional analytics and big data analytics
Unlike traditional analytics, which works with structured and often smaller datasets, big data analytics can handle unstructured data and leverage high-performance computing to process vast amounts of information in real time. This capability enables companies to react swiftly to changing conditions.
Big data analytics methods and applications
Big data analytics employs methods such as data mining, machine learning, and predictive analytics. Applications span multiple sectors:
- Retail: Personalized marketing based on consumer behavior.
- Healthcare: Predicting patient outcomes through electronic health records.
- Finance: Fraud detection by analyzing transaction patterns.
"Predictive analytics offers companies a forward-looking perspective, helping them anticipate changes, optimize resources, and act decisively in dynamic environments."
Real-world examples of responsible AI
Several organizations have implemented responsible AI solutions to address business challenges while maintaining fairness and transparency:
- Microsoft: The company developed AI tools that help public sector organizations ensure compliance with ethical standards, providing transparency in government operations.
- Google Health: Using responsible AI frameworks, Google has built predictive healthcare models to assist clinicians in providing more accurate patient diagnoses without introducing biases.
- Amazon: The retail giant utilizes AI-driven recommendation systems that adhere to strict privacy standards, ensuring customer data remains secure while improving the shopping experience.
These examples illustrate how responsible AI enables companies to unlock innovation without compromising trust. As industries adopt AI solutions, embedding ethical practices within these systems will become essential to delivering sustainable value and maintaining competitive advantage.
Data analytics process
The data analytics process is a structured, multi-step approach designed to convert raw data into meaningful insights that can drive business decisions. Each step in this process is essential to ensure data accuracy, quality, and relevance, leading to high-value outcomes. Here’s a closer look at each stage:
Data collection
Data collection is the foundational step in the analytics process, involving the gathering of data from a range of sources. These sources can include transactional systems, social media platforms, IoT sensors, website interactions, and customer feedback channels. This step is critical as it forms the basis of analysis—poor data collection can lead to skewed or incomplete insights.
Data sources for collection often include:
- Internal systems: Data from CRM systems, ERP platforms, and other internal databases.
- External data: Public datasets, social media feeds, and third-party information relevant to market trends or consumer behaviors.
- IoT devices: Sensors and smart devices that provide real-time data on asset conditions, environmental changes, or consumer interactions.
Properly capturing and organizing this data ensures a holistic view of the factors influencing business performance.
Data storage
After data collection, the next step is data storage. Given the vast volumes of data organizations work with today, secure and scalable storage solutions are critical. Data storage enables easy access, efficient retrieval, and reliable backup of data, creating a solid foundation for the processing and analysis stages.
Common storage solutions include:
- Cloud storage: Services like AWS, Google Cloud, and Azure offer scalable storage with added security, making it ideal for growing datasets.
- Data warehouses: Data warehouses, like Snowflake or Google BigQuery, consolidate large volumes of structured and semi-structured data, allowing for high-speed querying and analysis.
- Data lakes: For handling both structured and unstructured data, data lakes provide a flexible storage environment, useful for big data projects and advanced analytics.
Storing data in structured, accessible environments ensures that analysts and tools can easily retrieve and work with the data when needed.
Data processing
Data processing involves transforming raw data into a structured format suitable for analysis. This step includes organizing, categorizing, and formatting data, ensuring consistency across different sources. Processing is often necessary because raw data arrives in various forms and structures, which can complicate analysis.
Key activities in data processing include:
- Data transformation: Converting data into a consistent format, such as standardizing dates, numbers, and other entries.
- Data integration: Combining data from multiple sources, ensuring a unified dataset that represents the full scope of collected information.
- Data enrichment: Adding relevant external data or additional variables to enrich the existing data set, enhancing its value for analysis.
Data processing ensures that data is reliable, making it easier to uncover patterns and extract insights in the later stages.
Data cleansing
Data cleansing is the process of refining data by removing errors, inconsistencies, or inaccuracies. This step is crucial to maintaining data quality, as faulty or duplicate entries can lead to misleading analysis results. High-quality data is foundational to accurate and actionable insights, so data cleansing is a necessary quality control measure.
Key aspects of data cleansing include:
- Error correction: Identifying and correcting any inaccurate entries, such as typos or incorrect values.
- Duplicate removal: Eliminating redundant entries that could skew results.
- Handling missing values: Deciding on appropriate methods for addressing gaps in data, such as filling them with averages or removing incomplete records.
Data cleansing ensures the dataset is reliable and consistent, enabling more accurate and meaningful analysis.
Data analysis
Data analysis is the stage where meaningful insights are extracted from the prepared dataset. Various analytical techniques are applied to interpret the data, identifying trends, patterns, and relationships. This is where data is transformed into actionable insights, guiding business decisions and strategy.
Typical methods used in data analysis include:
- Descriptive analysis: Summarizing historical data to understand past performance.
- Predictive modeling: Forecasting future trends based on historical patterns.
- Diagnostic analysis: Investigating the underlying causes of observed patterns.
By applying the right methods, organizations can leverage data analysis to make informed decisions, optimize operations, and anticipate market shifts.
Data analytics techniques and tools
Data analytics relies on various techniques and tools to handle data effectively and generate insights. Each tool and technique is suited to specific data types and objectives, from summarizing trends to predictive modeling.
Statistical analysis
Statistical analysis is foundational to data analytics, using mathematical methods to summarize and interpret data. By calculating metrics like mean, median, standard deviation, and correlation, statistical analysis helps identify patterns and relationships in the data.
Examples of statistical techniques include:
- Descriptive statistics: Summarizes data, providing insight into central tendencies and variability.
- Inferential statistics: Draws conclusions about a larger population based on a sample, helping to generalize findings.
- Regression analysis: Examines relationships between variables, enabling prediction and trend identification.
Statistical analysis is essential for transforming raw data into meaningful summaries, which support deeper analytical exploration.
Machine learning
Machine learning (ML) is a branch of artificial intelligence that enables systems to learn from data and improve predictions or actions over time. By training algorithms on large datasets, ML models can identify complex patterns and make accurate predictions.
Key machine learning methods include:
- Supervised learning: Models are trained with labeled data to make predictions, such as classifying customer segments or forecasting sales.
- Unsupervised learning: Algorithms identify patterns in unlabeled data, often used for clustering and anomaly detection.
- Reinforcement learning: Models learn by receiving feedback on actions, useful for optimizing complex processes like inventory management.
Machine learning is a powerful tool in data analytics, especially in predictive and prescriptive analytics, enabling organizations to automate and enhance decision-making processes.
Data visualization
Data visualization transforms data into graphical representations, making it easier to interpret and communicate complex information. By using charts, graphs, heatmaps, and dashboards, data visualization tools provide a visual summary of insights, helping stakeholders understand trends at a glance.
Popular data visualization tools include:
- Tableau: Offers interactive dashboards and a wide range of chart options, making it popular for exploratory data analysis.
- Power BI: Microsoft’s tool integrates well with other Microsoft products, ideal for enterprise reporting.
- D3.js: A JavaScript library that enables custom, web-based visualizations for unique, dynamic graphics.
Visualizations make data more accessible, aiding in quicker decision-making and helping non-technical stakeholders engage with the data’s story.
Natural language processing (NLP)
Natural language processing (NLP) focuses on analyzing and understanding human language, enabling computers to interpret and respond to text or voice data. NLP is valuable in applications where language-based data is abundant, such as customer feedback, social media, and customer service interactions.
Key NLP techniques include:
- Sentiment analysis: Determines whether language expresses positive, negative, or neutral emotions, useful in customer feedback analysis.
- Entity recognition: Identifies specific elements within text, such as names, dates, and locations.
- Topic modeling: Categorizes large sets of text data based on themes or topics, allowing businesses to understand common subjects of interest.
NLP helps businesses gain insights from unstructured text data, improving customer service, monitoring brand sentiment, and identifying emerging topics.
Text mining
Text mining is extracting valuable information from unstructured text data, often used alongside NLP. It involves parsing large volumes of text to find trends, keywords, or correlations that reveal actionable insights. This technique is especially useful for analyzing customer reviews, feedback forms, emails, and other written sources.
Text mining methods include:
- Keyword extraction: Identifies the most common or relevant words in a dataset.
- Clustering: Groups text data based on similarities, helping to categorize feedback or comments.
- Document summarization: Condenses large documents into concise summaries, useful for quickly understanding long reports or articles.
Text mining allows organizations to transform textual information into quantitative data, enabling insights that support decision-making in areas like customer experience, marketing, and product development.
These techniques and tools collectively empower organizations to harness data analytics effectively, turning raw data into actionable insights that drive competitive advantage and strategic growth. By choosing the right mix of methods, businesses can align their analytics processes with their unique objectives, ensuring maximum value from their data investments.
Common applications of data and analytics
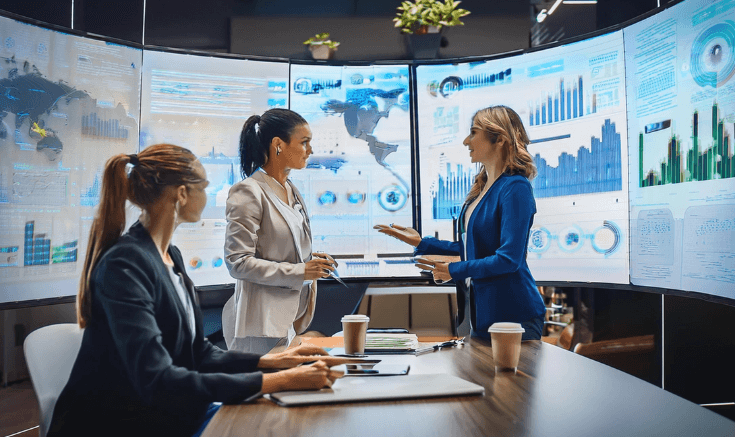
Data analytics has broad applications across industries, including:
- Business intelligence (BI): Organizations use BI tools to gather and analyze business data, facilitating strategic decision-making.
- Healthcare analytics: Hospitals and clinics leverage analytics for patient care improvements, predicting disease outbreaks, and optimizing resource allocation.
- HR analytics: Analyzing workforce data helps HR departments manage employee performance, predict turnover, and enhance recruitment strategies.
- Supply chain and logistics analytics: Companies use analytics to optimize routes, reduce delivery times, and manage inventory effectively.
- Financial analytics: Banks apply analytics for fraud detection, risk assessment, and personalized financial advice.
- Oil and gas industry analytics: By analyzing geological data and sensor information, firms can enhance exploration success and streamline drilling operations.
"Emerging trends such as edge computing, real-time analytics, and augmented analytics are revolutionizing the data landscape by allowing faster, more localized processing and integrating more dynamic, interactive analytics capabilities."
Implementing a data and analytics strategy
A well-defined data and analytics strategy is essential for any organization seeking to harness the power of data effectively. This strategy ensures that data collection, processing, analysis, and insights are aligned with business objectives, supporting decision-making at every level. Implementing a robust data and analytics strategy requires careful planning, clear objectives, and investment in both technology and talent. Let’s look at the key aspects involved in building a successful data and analytics strategy.
Building data and analytics capabilities
To establish a successful data analytics function, organizations must first build a strong foundation, which involves investing in the right infrastructure, technologies, and talent. This stage is crucial to ensure data quality, security, and accessibility across the organization, creating the framework necessary for meaningful analysis.
- Talent acquisition: Hiring skilled data professionals is foundational. Building an effective data team typically includes data scientists, data engineers, analysts, and business intelligence (BI) specialists, each contributing unique skills to gather, process, and interpret data. As data roles become increasingly specialized, it’s essential to assess the organization’s specific needs and hire accordingly.
- Technological infrastructure: Investing in scalable and secure data infrastructure is critical to support analytics. This includes data storage solutions such as cloud platforms, data lakes, and data warehouses that can handle the organization’s data volume and complexity. Many organizations are adopting cloud solutions due to their flexibility, scalability, and lower upfront costs.
- Data governance and security: A structured data governance framework ensures data quality, compliance, and security. Implementing governance policies, such as access controls, data classification, and auditing practices, protects sensitive information and ensures compliance with regulations like GDPR. Governance also involves data stewardship, where designated team members oversee data quality and integrity.
- Training and skill development: Data and analytics are rapidly evolving fields, and upskilling employees is essential. Organizations should prioritize continuous training, allowing employees to stay updated with the latest analytical techniques, software, and tools. Offering certifications, workshops, and seminars helps build internal expertise and encourages knowledge sharing across teams.
Building data and analytics capabilities involves a balance of technology and talent, ensuring the organization is well-prepared to derive meaningful insights from its data investments.
Best practices in data analytics
Implementing best practices ensures that data analytics functions efficiently, producing high-quality insights that drive better decision-making. Here are some best practices that organizations should follow:
Data warehousing
Data warehousing is a best practice that allows organizations to consolidate data from various sources into a single repository, making it easier to access and analyze information. By centralizing data, data warehouses improve the efficiency and speed of querying large datasets, supporting complex analyses across departments.
Advantages of data warehousing include:
- Centralized access: With all data in one place, analysts can access information quickly, reducing the time needed to gather and prepare data for analysis.
- Improved data quality: Data warehouses often incorporate data cleansing processes, ensuring that information is accurate, consistent, and reliable.
- Scalability: Modern data warehouses, particularly cloud-based ones, are highly scalable, allowing organizations to expand storage and processing capabilities as data volumes grow.
Data warehousing enhances analytics by creating a streamlined environment where data is readily available, reducing silos, and fostering data-driven decision-making across the organization.
Collaboration
Effective data analytics requires collaboration across departments to ensure data initiatives align with broader business goals. Collaborative efforts among IT, operations, marketing, finance, and other departments allow for a shared understanding of the organization’s data needs and challenges. This alignment promotes a unified approach to data management and helps departments benefit from insights relevant to their specific goals.
Key aspects of collaborative data analytics include:
- Cross-functional teams: Establishing teams with members from different departments helps incorporate diverse perspectives into data projects, enhancing the relevance and applicability of insights.
- Shared goals and metrics: Defining shared KPIs and metrics aligns analytics with the company’s broader objectives, ensuring that insights are actionable and beneficial across functions.
- Transparent communication: Encouraging open communication about data initiatives and findings helps departments understand and act on insights, fostering a culture of data-driven decision-making.
By fostering collaboration, organizations create a data culture that supports mutual goals, enhancing the overall impact of analytics.
Continual improvement
The data analytics field is dynamic, with new tools, techniques, and methodologies constantly emerging. Adopting a mindset of continual improvement ensures that an organization’s data practices evolve and remain competitive. Regularly revisiting and refining analytics practices helps organizations adapt to changing market conditions, customer behaviors, and technological advancements.
Practices to support continual improvement include:
- Regular reviews and audits: Conducting periodic assessments of data processes, tools, and techniques helps identify areas for improvement. Audits can reveal gaps in data quality, security, or process efficiency, prompting timely updates.
- Experimentation and innovation: Encouraging experimentation with new tools, data sources, and analytical techniques promotes a culture of innovation. Testing new methods can yield fresh insights and reveal more efficient ways to analyze data.
- Feedback loops: Gathering feedback from users of analytics outputs, such as department heads or executives, provides valuable insights into how well data analytics is meeting business needs and where adjustments are required.
Continual improvement not only enhances data quality and relevance but also enables organizations to stay agile, ensuring they can respond quickly to emerging trends and shifts in the market.
Implementing a robust data and analytics strategy demands a combination of technical investments, strong governance, and collaboration across the organization. By focusing on building data capabilities and following best practices in warehousing, collaboration, and continuous improvement, organizations can create an analytics framework that consistently delivers value, insight, and competitive advantage.
Future considerations for data and analytics
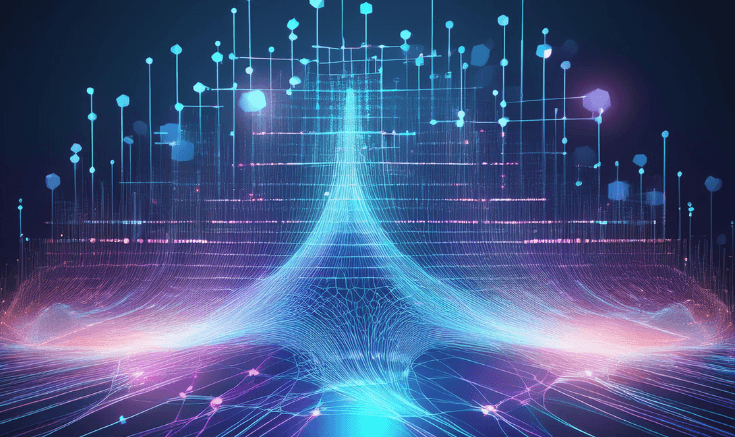
The future of data and analytics is being shaped by rapid advancements in artificial intelligence (AI) and other emerging technologies that are transforming how organizations derive insights and make decisions. AI is enhancing data analytics with advanced tools like deep learning, which enables more accurate predictions, pattern recognition, and even autonomous decision-making in complex environments.
Additionally, emerging trends such as edge computing, real-time analytics, and augmented analytics are revolutionizing the data landscape by allowing faster, more localized processing and integrating more dynamic, interactive analytics capabilities. These innovations are not only increasing the speed and precision of insights but also empowering businesses to act on data in real time, creating new opportunities to remain agile and responsive in an increasingly data-driven world.
Data and analytics represent an unparalleled opportunity for businesses to gain a competitive edge, drive innovation, and make informed decisions based on actionable insights. By understanding the different types of analytics, the process involved, and the tools and techniques available, organizations can implement a successful data-driven strategy. For businesses seeking to leverage data and analytics in a way that aligns with their unique goals, Lumenalta offers tailored solutions designed to empower transformation through expert-driven analytics solutions.
Table of contents
- Understanding data and analytics
- Importance of data and analytics
- Types of data analytics
- Big data analytics
- Data analytics process
- Data analytics techniques and tools
- Common applications of data and analytics
- Implementing a data and analytics strategy
- Future considerations for data and analytics
- Common questions about data and analytics
Common questions about data and analytics
What are data and analytics, and why are they important?
What is the difference between traditional analytics and big data analytics?
What are the main types of data analytics?
How does machine learning enhance data analytics?
What are the emerging trends in data and analytics?
Want to learn how data & analytics can bring more transparency and trust to your operations?