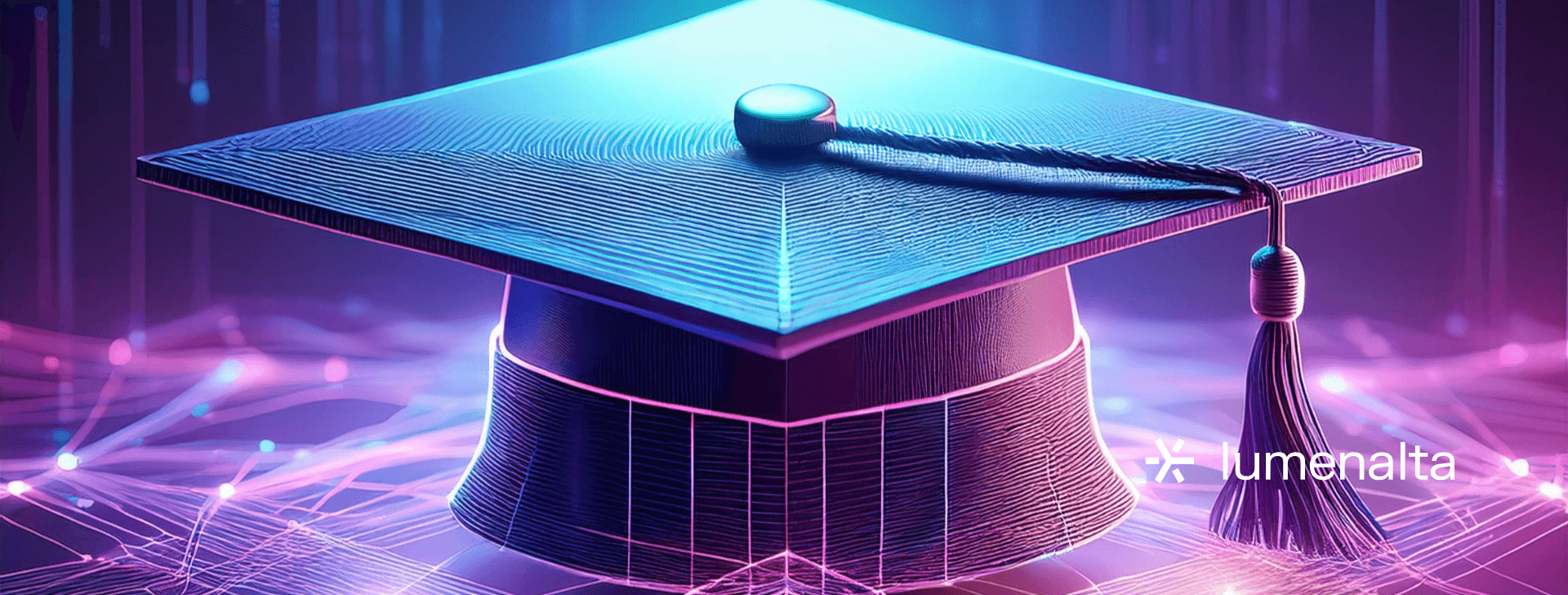
11 benefits of machine learning in education
DEC. 28, 2024
9 Min Read
Machine learning in education is reshaping how institutions deliver knowledge, manage resources, and support students.
This technology analyzes vast data to personalize learning, streamline operations, and optimize outcomes. Adopting machine learning allows educators and administrators to address complex challenges with greater efficiency and precision, ensuring every learner has the opportunity to succeed.
Key takeaways
- 1. Machine learning personalizes learning experiences by analyzing student data to tailor content and teaching strategies.
- 2. Automated administrative processes reduce workload and enhance efficiency for educators and administrators.
- 3. Real-time insights and data analysis help institutions make informed decisions and improve learning outcomes.
- 4. Equitable and unbiased grading systems promote trust and fairness in student evaluations.
- 5. Advanced digitization of materials and predictive analytics prepare institutions for future educational challenges.
What is machine learning in education?
Machine learning is a transformative technology that equips educational institutions with tools to automate processes, personalize student experiences, and optimize operations. Leveraging algorithms and data insights enable machine learning to adapt to individual learning needs and institutional challenges, revolutionizing traditional education.
At its core, machine learning uses data to identify patterns and improve decision processes. This means dynamically assessing student performance, predicting academic outcomes, and tailoring educational materials to enhance engagement in education. Beyond students, machine learning enhances teacher efficiency, facilitates administrative tasks, and even improves long-term strategic planning for institutions. As adoption grows, its potential to drive equity and innovation in education becomes evident, ensuring more tailored and impactful learning experiences.
"Machine learning enables the development of tailored learning paths for individual students by analyzing their strengths, weaknesses, and preferences."
11 benefits of machine learning in education
Machine learning creates significant opportunities to reshape the educational experience for students, educators, and administrators. Its application spans personalized instruction, operational improvements, and greater resource access. Understanding how this technology supports teaching and learning can help institutions unlock its full potential. From streamlining processes to improving equity, the possibilities are as varied as they are impactful.
1. Personalized learning experience
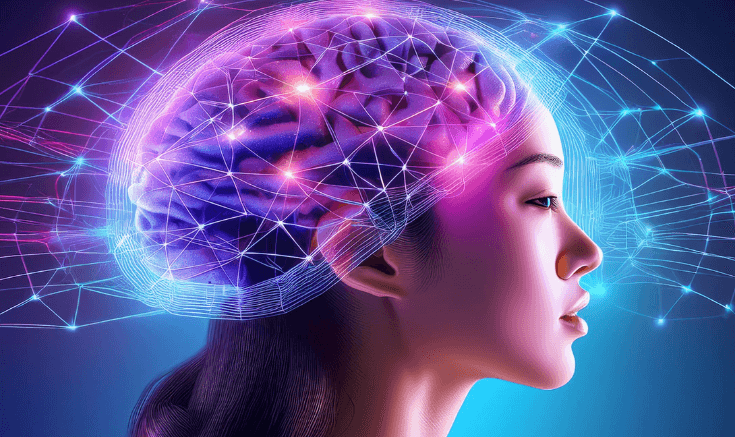
Machine learning enables the development of tailored learning paths for individual students by analyzing their strengths, weaknesses, and preferences. Algorithms assess how students interact with the material, identifying areas where they excel and topics that need reinforcement. Adaptive learning platforms use this data to suggest resources, pace lessons, or even reframe concepts in ways students better understand.
Personalization benefits students by making learning more engaging and efficient. Educators also gain a clearer picture of where interventions are necessary. This targeted approach replaces one-size-fits-all instruction with a system designed for success, ensuring that every student receives the support needed to flourish academically.
2. Improved learning outcomes
Machine learning improves educational outcomes by offering insights into student performance. Predictive analytics assess past performance and engagement to identify factors contributing to success or struggles. For example, if students consistently underperform on quizzes, machine learning tools can suggest specific content reviews or alternative teaching strategies.
This evidence-based approach helps educators refine their methods, making teaching more effective. Students benefit from enhanced support and guidance, which can significantly boost understanding, retention, and long-term academic achievement.
3. Enhanced operational efficiency
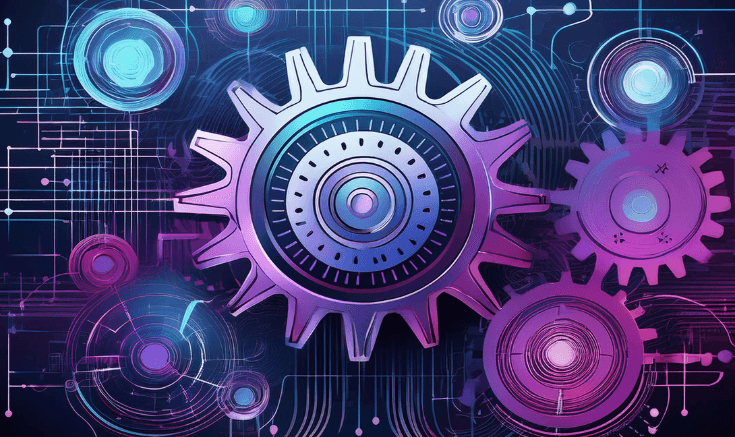
Educational institutions often face challenges in managing resources effectively. Machine learning optimizes administrative processes such as course scheduling, resource allocation, and even maintenance of campus facilities. Algorithms streamline workflows, ensuring resources are used where they have the greatest impact.
These efficiencies free educators and administrators to focus more on core responsibilities. Students indirectly benefit, as well, by accessing a more responsive and organized learning background.
4. Reduced administrative workload
Machine learning automates time-intensive administrative tasks like attendance tracking, assignment grading, and data entry. Educators reclaim valuable time to concentrate on teaching and mentoring by delegating repetitive processes to intelligent systems.
For example, automated systems can analyze submitted essays for grammatical errors, plagiarism, or alignment with assignment objectives. This level of automation speeds up routine tasks and maintains high levels of accuracy and consistency.
5. More accurate assessment and grading
Subjective grading and human error are ongoing challenges in education. Machine learning minimizes these issues by applying consistent criteria to assess student work. It objectively evaluates written assignments, quizzes, and even complex projects, ensuring fairer outcomes.
This level of accuracy helps students trust the evaluation process while allowing educators to focus on qualitative aspects of teaching. Over time, such fairness in grading can encourage greater effort and engagement from students.
6. Greater access to online learning
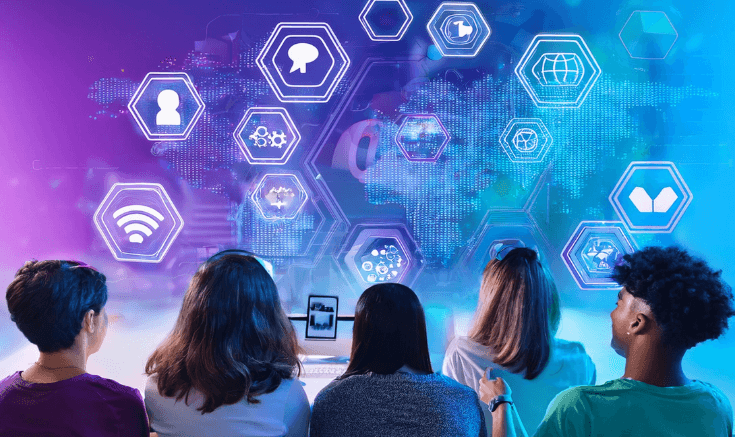
Machine learning supports the creation of more accessible and inclusive online learning platforms. Algorithms analyze various learning needs and adjust course delivery methods to meet them. This adaptability ensures learners of all abilities, languages, or geographical locations can participate in high-quality education.
The rise of intelligent chatbots, AI-backed tutors, and real-time feedback systems makes virtual learning settings more supportive and effective. These tools extend opportunities for lifelong learning beyond traditional classrooms, fostering equitable education worldwide.
7. Faster research and data analysis
Academic research demands extensive data collection and analysis, tasks where machine learning excels. Algorithms can process large datasets instantly, identifying trends, correlations, or anomalies. This capability accelerates research timelines, enabling educators and students to derive insights faster.
In fields like scientific studies or social research, machine learning simplifies complex data modeling. The technology supports evidence-based innovations, allowing researchers to dedicate more effort to interpretation and implementation.
8. Improved enrollment processes
Admissions offices often deal with large volumes of applications. Machine learning simplifies this process by analyzing applicant data to identify qualified candidates. Algorithms consider grades, extracurricular activities, and personal essays, offering insights to admissions teams.
By streamlining the enrollment process, institutions reduce manual effort while ensuring decisions are aligned with their strategic goals. Students benefit from faster communication and clarity during the application process, improving their overall experience.
9. Predictions of future career paths
Machine learning helps students make informed decisions about their futures by analyzing data on academic performance, skills, and market trends. Career guidance platforms use this technology to suggest fields of study or professions that align with students’ aptitudes and interests.
This forward-looking approach enables students to plan strategically for long-term success. Institutions that integrate these tools can better prepare graduates for job markets, reinforcing their commitment to student outcomes.
10. Unbiased grading and feedback
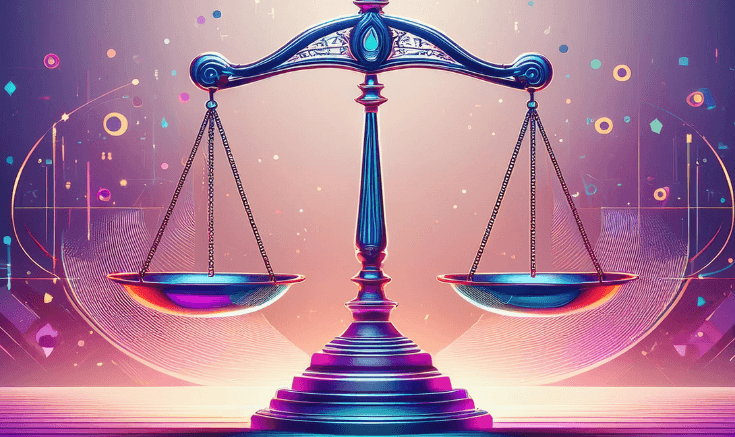
Bias in grading can negatively impact student morale and progress. Machine learning eliminates subjective biases by applying uniform criteria across assessments. Algorithms focus purely on content, ensuring that evaluations are impartial and merit-based.
This objectivity builds trust in the grading system while providing students with constructive, data-backed feedback. Educators also gain an additional tool for reflecting on and improving their teaching methods.
11. Digitization of learning materials
Machine learning automates the digitization and organization of educational content, making it easily accessible and searchable. Algorithms categorize resources by subject, difficulty level, and learning objectives, streamlining access for educators and students.
This digital approach promotes flexibility in learning, as students can access resources on demand. Institutions also reduce reliance on physical materials, supporting environmentally sustainable practices and modernizing educational delivery.
Integrating machine learning into education represents a fundamental shift in how institutions, educators, and students approach learning and operational efficiency. Each benefit—from personalized learning experiences to unbiased grading—contributes to a more inclusive, equitable, and impactful educational conditions. These advancements enhance individual learning outcomes and streamline institutional processes, ensuring resources are allocated efficiently and educators can focus on meaningful teaching. Machine learning's capacity to analyze data at scale, provide actionable insights, and adapt to diverse learner needs makes it a critical tool for modern education.
Institutions adopting machine learning can unlock unprecedented opportunities to prepare students for future challenges and career paths. Beyond the classroom, this technology supports educational equity, broadens access to learning resources, and fosters innovation in teaching methods. Its role in digitizing materials and delivering predictive insights positions education systems to stay relevant and responsive. Through its ability to optimize both operational and learning outcomes, machine learning is not just improving education but empowering it to reach new heights, setting the foundation for a brighter, more accessible future for all learners.
"Machine learning automates time-intensive administrative tasks like attendance tracking, assignment grading, and data entry, allowing educators to reclaim valuable time for teaching and mentoring."
Strategies for implementing machine learning in education
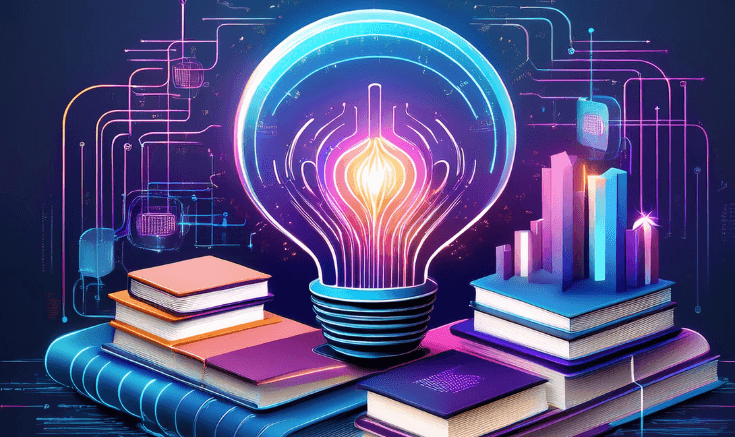
Implementing machine learning in education requires a strategic approach to ensure its full potential is realized. Institutions must focus on aligning the technology with their unique goals and challenges. This includes identifying areas where machine learning can deliver measurable value, such as personalized learning, administrative automation, or data-led decisions. Careful planning can help educators and administrators adopt this technology effectively while minimizing risks and costs.
The first step involves conducting a thorough needs assessment to pinpoint opportunities where machine learning can make a difference. This ensures that investments in technology address specific gaps or inefficiencies. Training educators and staff to understand and leverage these tools is equally important. Their ability to interpret insights and use machine learning systems will directly impact success. Institutions should also prioritize scalability by choosing solutions that grow with their needs.
Collaboration with technology partners and data experts can accelerate implementation. These partnerships provide access to specialized expertise, ensuring systems are integrated seamlessly with existing platforms. Regular evaluation and iteration are also essential. Institutions should monitor the outcomes of machine learning initiatives and refine their approaches to maximize effectiveness. With a thoughtful and deliberate implementation plan, education systems can use the transformative power of machine learning to drive meaningful improvements.
Measuring business impact of machine learning in education
Understanding the business impact of machine learning in education helps institutions evaluate the return on investment and determine the value delivered through technological advancements. Measurable outcomes ensure decision-makers can justify expenditures while fine-tuning their strategies for ongoing success. With the right metrics in place, educational institutions can link machine learning initiatives directly to improvements in efficiency, student engagement, and institutional growth.
Assessing operational efficiency
Operational efficiency is one of the most immediate ways machine learning demonstrates value in education. Algorithms automate attendance tracking, resource scheduling, and performance reporting tasks. Metrics like time saved on administrative duties and resource optimization highlight the effectiveness of machine learning tools.
Schools can measure efficiency gains by comparing pre- and post-implementation performance data. Reduced administrative overhead means more time for educators to focus on students and strategic planning. These improvements ripple across the institution, creating a smoother operational workflow.
Evaluating student outcomes
Student success is a key indicator of the business impact of machine learning in education. Metrics such as test scores, retention rates, and course completion rates offer concrete evidence of the technology's effectiveness. Personalized learning systems, for example, adapt to individual needs, improving comprehension and engagement.
Tracking student performance data over time reveals how machine learning influences outcomes. Institutions can identify patterns that show where interventions are most needed, ensuring continuous improvement. Enhanced academic results boost the institution's reputation, attracting more students and funding opportunities.
Measuring cost-effectiveness
Cost-effectiveness remains a critical consideration for educational institutions adopting new technologies. Machine learning systems reduce manual workloads and streamline operations, saving money over time. Evaluating this benefit involves calculating the return on investment by comparing implementation costs with savings from automated processes.
In addition to reducing expenditures, these tools help institutions allocate funds more strategically. Predictive analytics guide resource distribution, ensuring budgets align with priority areas like student support and curriculum development. Cost-effectiveness improves financial sustainability, positioning institutions for long-term growth.
Analyzing scalability and future readiness
The scalability of machine learning tools is another important metric for assessing their business impact. Systems that adapt to growing student populations or expanded curricula demonstrate long-term value. Evaluating how well these solutions integrate with existing platforms highlights their potential to evolve with institutional needs.
Future readiness ensures machine learning systems keep pace with technological advancements and educational trends. Institutions that prioritize adaptability stay competitive and prepared for challenges. Regularly assessing scalability and readiness provides a roadmap for maintaining relevance.
Examining these dimensions—operational efficiency, student outcomes, cost-effectiveness, and scalability—enables institutions to comprehensively view how machine learning impacts their educational mission. These measurable benefits ensure that machine learning continues to serve as a valuable asset in driving institutional success and fostering academic excellence.
Machine learning transforms education into a personalized experience that fosters growth and efficiency for students and institutions. Lumenalta specializes in creating custom machine learning solutions tailored to educational needs, empowering organizations to enhance learning outcomes, streamline operations, and prepare for the future.
Let’s build a brighter path to education together.
table-of-contents
- What is machine learning in education?
- 11 benefits of machine learning in education
- 1. Personalized learning experience
- 2. Improved learning outcomes
- 3. Enhanced operational efficiency
- 4. Reduced administrative workload
- 5. More accurate assessment and grading
- 6. Greater access to online learning
- 7. Faster research and data analysis
- 8. Improved enrollment processes
- 9. Predictions of future career paths
- 10. Unbiased grading and feedback
- 11. Digitization of learning materials
- Strategies for implementing machine learning in education
- Measuring business impact of machine learning in education
- Common questions about machine learning in education
Common questions about machine learning in education
How does machine learning enhance education?
What are the operational benefits of machine learning in education?
Can machine learning improve online learning platforms?
What is the role of machine learning in future career preparation?
Are machine learning systems in education scalable?
Want to learn how machine learning can bring more transparency and trust to your operations?