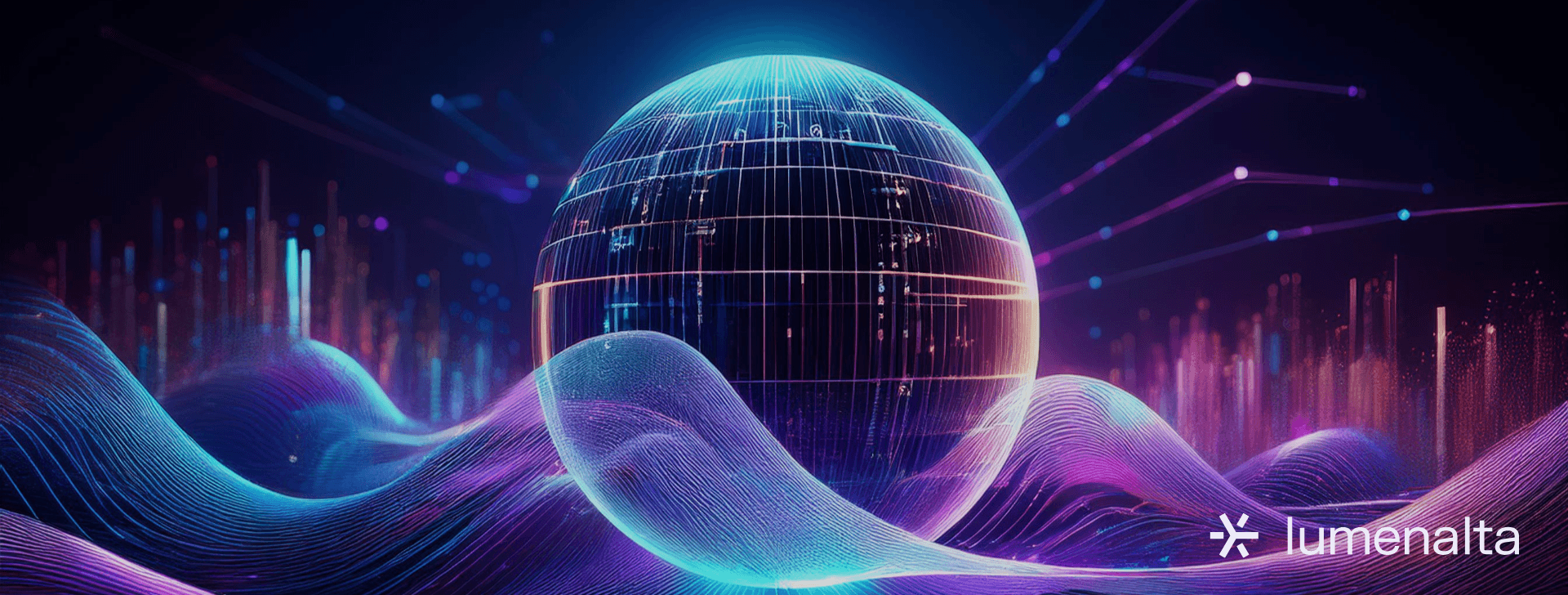
6 generative AI use cases in banking and financial services
OCT. 17, 2024
6 Min Read
The financial services industry is entering a new era of efficiency and personalization, driven by advancements in generative artificial intelligence (AI).
Unlike traditional AI models that rely on analyzing existing data, generative AI systems create new outputs, such as financial reports, risk assessments, and customer interactions. This technology holds the potential to revolutionize operations by automating complex processes, enhancing customer experiences, and supporting smarter decision-making across the financial ecosystem.
Generative AI allows banks and financial institutions to reduce manual workloads, improve accuracy, and deliver hyper-personalized services. From providing real-time investment advice to identifying fraudulent transactions, this technology addresses critical industry challenges with precision and speed. As financial institutions strive to stay competitive in a fast-evolving market, embracing generative AI will be key to driving sustainable growth, mitigating risks, and meeting the rising expectations of today’s customers.
Key takeaways
- 1. Generative AI delivers hyper-personalized financial services by analyzing real-time customer data, offering tailored product recommendations, investment advice, and savings plans.
- 2. AI-powered fraud detection improves security by identifying suspicious transactions in real time, reducing false positives, and preventing financial losses.
- 3. Virtual assistants and chatbots streamline customer service by automating routine inquiries, enabling 24/7 support, and reducing operational costs.
- 4. Generative AI enhances credit risk assessment by leveraging diverse datasets to create dynamic risk profiles, promoting financial inclusion and speeding up loan approvals.
- 5. AI-driven automation improves document processing and compliance by extracting, validating, and reporting data in real time, reducing manual errors and ensuring regulatory adherence.
What is generative AI and why it matters in financial services
Generative AI refers to artificial intelligence systems capable of producing new and meaningful content, such as text, images, audio, or even sophisticated models and financial predictions. Unlike traditional AI, which typically analyzes existing data to make decisions, generative AI creates new outputs by learning patterns from large datasets. Its ability to generate original content and simulate decision-making processes makes it particularly valuable in the highly dynamic financial services sector.
In financial services, generative AI holds transformative potential by addressing key operational challenges and enhancing customer interactions. Below are several ways it brings value to the industry:
- Automate routine tasks and processes: Generative AI can streamline back-office operations, such as document processing, data entry, and reconciliation, which traditionally require hours of manual effort. For instance, AI models can generate regulatory compliance reports, auto-fill customer forms, or even process transactions with minimal human intervention, freeing up employees to focus on high-value activities.
- Provide personalized financial advice and recommendations: Generative AI algorithms can analyze a customer’s financial behavior, spending patterns, and preferences to generate tailored financial advice. Robo-advisors equipped with these models help customers make informed decisions about investments, savings plans, and insurance products, enhancing personalization and improving customer satisfaction.
- Enhance risk assessment and fraud detection capabilities: AI-generated models are revolutionizing how financial institutions assess creditworthiness, predict market risks, and detect fraudulent activities. By generating risk models in real time, financial institutions can identify patterns that indicate suspicious transactions or emerging risks, improving accuracy in areas such as underwriting or anti-money laundering (AML) processes.
- Improve customer service through advanced chatbots and virtual assistants: Chatbots and AI-powered virtual assistants are becoming essential tools for banks and financial institutions. These conversational agents can generate human-like responses to customer inquiries, handle account management tasks, and resolve common service issues around the clock, significantly enhancing the customer experience.
- Generate detailed financial reports and analyses: Financial institutions generate vast amounts of data daily. Generative AI models are adept at processing this data and producing comprehensive financial reports, forecasts, and performance analyses. AI-generated insights not only save time but also ensure accuracy, providing decision-makers with the critical information they need to drive business strategies.
Generative AI matters in financial services because it enables firms to operate more efficiently, stay agile in the face of change, and deliver more value to customers. It empowers institutions to meet rising customer expectations, comply with evolving regulations, and manage risks more effectively. As a result, financial institutions that leverage generative AI are better positioned to maintain a competitive edge, improve operational efficiency, and unlock new opportunities for growth.
“Generative AI can streamline back-office operations, such as document processing, data entry, and reconciliation, which traditionally require hours of manual effort.”
6 generative AI use cases in banking and financial services
Generative AI is revolutionizing the financial sector by improving efficiency, enhancing decision-making, and enabling hyper-personalized customer experiences. Unlike traditional AI, which analyzes existing data, generative AI creates new outputs—such as insights, predictions, and automated responses—that directly impact how financial services operate. From streamlining back-office operations to providing personalized financial advice and real-time fraud detection, generative AI addresses the growing need for speed, accuracy, and tailored solutions in banking.
As competition intensifies and customer expectations evolve, financial institutions must adopt innovative technologies to remain agile and responsive. Generative AI offers a unique advantage by automating routine processes, mitigating risks through predictive analytics, and enhancing compliance with real-time reporting.
1. Personalized financial advice and product recommendations
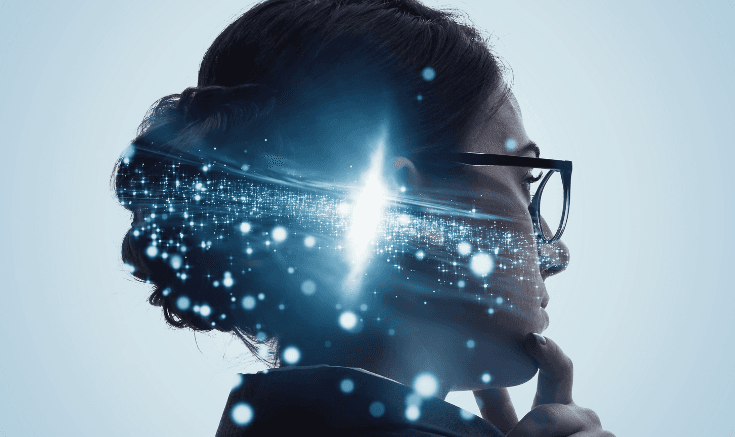
Generative AI allows banks and financial institutions to deliver hyper-personalized services by analyzing customer-specific data, such as transaction histories, income patterns, spending habits, and long-term financial goals. With these insights, AI-driven robo-advisors can provide tailored recommendations for investments, savings plans, loans, and insurance products. This goes beyond generic advice, enabling institutions to dynamically adjust recommendations based on real-time events, such as market fluctuations or changes in the customer’s financial behavior. Personalized advice not only enhances customer satisfaction but also deepens relationships by fostering trust and creating opportunities for cross-selling products at the right moments.
- Real-world example: Wells Fargo’s predictive banking feature provides personalized prompts about future financial activities, improving user engagement.
- Why this matters: Personalized services are increasingly expected by customers and are key to fostering long-term loyalty and trust. This also allows financial institutions to cross-sell relevant products more effectively, driving revenue.
As customer expectations for tailored services grow, generative AI offers financial institutions a way to deliver hyper-personalized experiences that build trust and deepen relationships. The ability to analyze real-time customer behavior and provide actionable insights empowers institutions to proactively meet customer needs. This personalization not only enhances satisfaction and loyalty but also unlocks new revenue streams through cross-selling. Institutions that successfully integrate AI-driven personalization into their operations will position themselves as leaders in customer-centric banking.
2. Fraud detection and prevention in real time
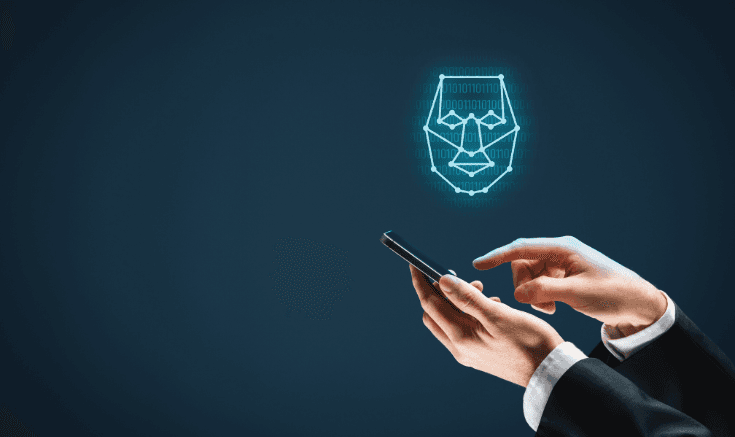
Fraud detection has become a top priority in the financial services sector as digital transactions increase and cybercriminals become more sophisticated. Generative AI plays a critical role in proactively identifying fraud by analyzing large volumes of transactional data to detect anomalies in real time. Unlike traditional rule-based systems, AI-powered models can learn from evolving patterns, flagging unusual behavior—such as unauthorized account access or abnormal spending patterns—before it results in significant financial loss. Additionally, generative AI reduces false positives, which can frustrate customers, while simultaneously improving security, building trust, and protecting the institution’s reputation.
- Real-world example: PayPal utilizes AI-powered systems to detect fraud by comparing transactions with historical patterns, reducing financial losses.
- Why this matters: Financial institutions face constant security threats. Real-time fraud detection ensures customer data protection and maintains the integrity of the institution’s services.
In an era of increasing cybersecurity threats, real-time fraud detection powered by generative AI becomes essential for maintaining trust and protecting both financial institutions and their customers. AI’s ability to identify patterns and predict fraudulent behavior before it occurs strengthens the institution’s defenses while minimizing the inconvenience of false alarms. By ensuring security without sacrificing the customer experience, institutions can build long-term trust and safeguard their reputation in the competitive financial market.
3. Automated customer service and virtual financial assistants
AI-powered chatbots and virtual assistants are reshaping customer service by providing always-on, instant support across multiple channels. These virtual agents can handle common inquiries—such as account balances and transaction history—or perform more complex tasks like loan applications and password resets. Generative AI models use natural language processing (NLP) to deliver human-like conversations, offering proactive advice and personalized interactions. With automation handling routine tasks, human agents can focus on higher-value customer interactions, improving efficiency and satisfaction. Financial institutions benefit from reduced operational costs and faster response times, while customers enjoy seamless, round-the-clock service.
- Real-world example: Bank of America’s Erica, a virtual assistant, assists customers with account information, bill payments, and personalized financial advice through chat or voice.
- Why this matters: Automation reduces operational costs and enhances customer satisfaction by enabling seamless, always-on support without increasing staffing needs.
Automated customer service powered by generative AI provides financial institutions with a competitive advantage by enabling 24/7 support and reducing operational costs. As chatbots and virtual assistants become more sophisticated, they will play a crucial role in delivering fast, accurate, and personalized interactions across multiple channels. This not only frees up human agents to handle more complex inquiries but also improves customer satisfaction by providing seamless, real-time service. Institutions that adopt these AI tools will benefit from increased efficiency and stronger customer relationships.
4. Credit risk assessment and loan underwriting
Generative AI enhances the accuracy and speed of credit risk assessments by analyzing vast datasets, including transaction records, employment data, and behavioral trends. Traditional credit scoring models often rely on static indicators, leaving out nuanced insights about a borrower’s current financial state. AI-powered models, however, can provide real-time risk profiles that reflect up-to-date borrower behavior, allowing for faster and more reliable loan approvals. Additionally, these models enable financial institutions to extend credit to customers with non-traditional profiles, such as gig economy workers, promoting financial inclusion. This dynamic approach helps reduce default rates and enhances loan portfolio performance.
- Real-world example: Upstart, an AI-based lending platform, uses non-traditional data to assess creditworthiness, approving loans quickly for customers who might otherwise be denied by conventional models.
- Why this matters: Accurate risk assessments expand financial access and reduce default rates, helping institutions serve a broader range of customers while managing risks.
Generative AI transforms credit risk assessment by enabling real-time decision-making and expanding financial access to non-traditional borrowers. By leveraging AI to generate dynamic risk profiles, institutions can offer faster loan approvals while maintaining strong risk management. This approach promotes financial inclusion, strengthens portfolio performance, and ensures institutions can meet the evolving needs of their customers. As the lending landscape becomes more competitive, institutions that implement AI-enhanced underwriting processes will gain an edge in delivering both efficiency and responsible lending.
5. Market analysis and forecasting for investment strategies
Investment strategies are becoming increasingly data-driven, with AI-powered models helping institutions anticipate market trends and optimize portfolios. Generative AI analyzes vast amounts of financial data, including market sentiment, economic indicators, and corporate reports, to generate predictive insights. These models can simulate various market scenarios, allowing financial institutions to conduct stress tests and evaluate the potential impact of market shifts on their portfolios. With accurate forecasting and scenario analysis, firms can make more informed investment decisions, manage risks proactively, and maximize returns for clients, gaining a competitive edge in volatile markets.
- Real-world example: Goldman Sachs uses generative AI to optimize trading strategies and forecast market trends, gaining a competitive edge in dynamic markets.
- Why this matters: Predictive insights allow financial firms to stay ahead of market trends and make data-driven investment decisions, maximizing returns while mitigating risks.
In volatile financial markets, the ability to anticipate trends and proactively manage portfolios is a crucial differentiator. Generative AI equips financial institutions with predictive insights and scenario simulations that optimize investment strategies and mitigate risks. Firms that leverage AI to enhance their market analysis will be better positioned to make data-driven decisions, seize emerging opportunities, and deliver superior returns to their clients. As market dynamics evolve, institutions that integrate AI into their investment strategies will stand out by maintaining agility and foresight.
6. Document processing and compliance automation
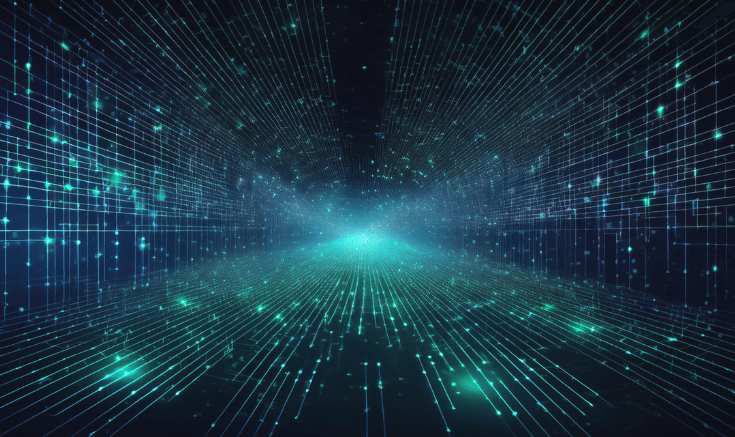
Financial institutions generate and manage massive volumes of documents, from loan applications and invoices to regulatory filings. Generative AI automates document processing by extracting key data from unstructured sources, minimizing the need for manual entry and reducing human errors. This automation not only speeds up processing but also ensures regulatory compliance by generating real-time reports and alerts for any discrepancies. As regulatory requirements become more complex, financial institutions benefit from reduced compliance risks and operational costs. By streamlining reporting and ensuring accuracy, AI helps institutions stay ahead of evolving regulations and avoids penalties for non-compliance.
- Real-world example: HSBC utilizes AI to process compliance documents efficiently, ensuring adherence to evolving regulations and minimizing manual errors.
- Why this matters: Regulatory compliance is critical in the financial sector, and automation reduces the burden on compliance teams, saving time and ensuring timely reporting.
Managing large volumes of documents and staying compliant with complex regulations can be resource-intensive for financial institutions. Generative AI offers a solution by automating these processes, improving accuracy, and ensuring real-time compliance. Institutions that adopt AI-driven document processing will reduce operational costs, avoid regulatory penalties, and improve efficiency. By streamlining compliance efforts and maintaining data integrity, financial institutions can focus on growth and innovation while confidently navigating an increasingly complex regulatory environment.
Generative AI is revolutionizing financial services, enabling hyper-personalized interactions, real-time fraud detection, faster loan approvals, and optimized investment strategies. By leveraging these technologies, financial institutions can enhance efficiency, improve customer satisfaction, and gain a competitive advantage in a rapidly evolving market. Institutions that adopt these AI-driven solutions will position themselves for long-term success, meeting the demands of modern customers while driving sustainable growth.
“Generative AI models are adept at processing data and producing comprehensive financial reports, forecasts, and performance analyses.”
Benefits of generative AI for financial institutions
The adoption of generative AI in financial services offers numerous advantages that go beyond simple automation, helping institutions gain a competitive edge. By strategically integrating AI technologies, financial institutions unlock operational efficiencies, deliver superior customer experiences, and drive innovation at scale.
Benefit | Description |
---|---|
Increased operational efficiency | AI automates time-consuming tasks such as document processing, compliance checks, and transaction reconciliation, reducing dependency on manual labor. |
Enhanced customer experience | AI-powered virtual assistants enable personalized, 24/7 support, providing proactive financial advice, instant query resolution, and tailored recommendations. |
Improved decision-making | AI-generated insights allow for more accurate and timely decision-making by analyzing large datasets in real time to identify risks and seize market opportunities. |
Faster time-to-market | AI accelerates product development cycles by automating repetitive processes and generating insights, helping institutions adapt to market and regulatory changes. |
Better risk management | AI models provide precise credit assessments, fraud detection, and predictive analytics, enabling proactive risk management with real-time scenario generation. |
Regulatory compliance and reporting | Generative AI automates compliance checks and ensures adherence to regulatory requirements, minimizing the risk of non-compliance with real-time reporting. |
Cost savings | Automation reduces overhead costs by minimizing manual labor, leading to faster turnaround times and helping achieve sustainable cost reductions. |
Scalability for growing demands | AI-powered systems handle larger volumes of transactions and inquiries without requiring additional staffing, ensuring operational resilience during growth phases. |
Challenges when adopting generative AI in finance
While the benefits of generative AI are compelling, financial institutions must navigate several challenges to realize its full potential.
Challenge | Description |
---|---|
Data quality and privacy management | Ensuring accurate and secure data is essential for building reliable AI models; data governance frameworks are required for privacy compliance. |
Ethical considerations and fairness | Financial institutions must address biases in AI algorithms to avoid unintended harm and build trust with customers and regulators. |
Navigating regulatory compliance | AI adoption requires alignment with evolving regulations, necessitating compliance tools to ensure model transparency and proper decision-making. |
Integration with legacy systems | Deploying new AI technologies alongside outdated infrastructure often requires hybrid approaches to ensure smooth integration. |
Talent acquisition and workforce development | There is a shortage of skilled AI professionals, making talent acquisition, training, and partnerships essential for managing AI solutions. |
Explainability and transparency | The 'black box' nature of AI makes it difficult to explain decisions; developing explainable AI models ensures regulatory compliance. |
Mitigating model bias and ensuring fairness | Regular audits and fairness checks are essential to avoid perpetuating existing inequalities through biased AI-driven decisions. |
Addressing cybersecurity risks | Financial institutions need robust security measures to protect AI models and data from cyber threats, ensuring operational continuity. |
Implementing generative AI for financial services
Successfully integrating generative AI into financial services requires more than just technological expertise—it demands strategic leadership, cross-functional collaboration, and a focus on business outcomes. Below are key best practices for banks and CEOs to follow when implementing generative AI effectively.
Step 1: Define clear objectives and align AI with business goals
AI initiatives must be purpose-driven, with clearly defined objectives that align with the broader business strategy. Whether the goal is to improve operational efficiency, enhance customer service, or mitigate risks, banks need to ensure that AI investments directly contribute to measurable outcomes.
Best practices:
- Identify high-impact use cases with clear KPIs, such as cost savings, increased revenue, or customer satisfaction
- Align AI initiatives with the institution's overall digital transformation roadmap
- Involve business units early in the planning process to ensure AI solutions address real business challenges
Step 2: Build a cross-functional implementation team
AI adoption is not solely an IT responsibility—it requires input from across the organization. Building a cross-functional team ensures that AI models are developed with the right expertise and meet the needs of both technical and business stakeholders.
Best practices:
- Assemble a team that includes data scientists, IT professionals, compliance officers, and business unit leaders
- Foster collaboration between technical and non-technical teams to align AI projects with operational realities
- Create agile working groups to manage implementation, ensuring flexibility for iteration and refinement
Step 3: Start small with pilot programs before scaling
Implementing generative AI at scale can be complex and resource-intensive. Leaders should begin with pilot programs to validate AI models, gather feedback, and measure initial outcomes. Once successful, these pilots can be scaled across the organization.
Best practices:
- Focus on specific, manageable use cases—such as automating customer inquiries or improving credit risk assessments
- Monitor pilot performance closely to identify areas for improvement before broader deployment
- Use insights from pilot projects to develop best practices and scalability frameworks
Step 4: Prioritize data quality and governance
The success of generative AI depends on access to high-quality, well-managed data. Leaders must ensure robust data governance policies are in place to maintain data accuracy, security, and compliance with regulations.
Best practices:
- Establish strong data governance frameworks to manage data quality and ensure regulatory compliance
- Implement processes for data cleaning, normalization, and integration from multiple sources
- Adopt privacy-by-design principles to protect customer data and comply with evolving data privacy laws
Step 5: Invest in talent development and strategic partnerships
AI adoption requires skilled talent that can build, deploy, and maintain complex AI systems. CEOs should focus on talent acquisition and foster partnerships with external vendors to access specialized expertise when needed.
Best practices:
- Invest in hiring and training data scientists, engineers, and AI specialists
- Partner with technology providers, cloud platforms, and research institutions to accelerate innovation
- Develop internal training programs to upskill employees and ensure continuous learning
Step 6: Ensure explainability, transparency, and trust
Financial institutions must ensure that AI models are transparent and explainable to regulators, customers, and internal stakeholders. Building trust in AI solutions is critical, especially for decisions related to lending, investments, and risk management.
Best practices:
- Use explainable AI (XAI) techniques to make model outputs and decision-making processes transparent
- Develop documentation and reporting tools to communicate AI decisions clearly to customers and regulators
- Regularly audit AI models to identify and mitigate potential biases, ensuring fair and ethical decision-making
Step 7: Monitor performance and iterate continuously
AI implementation is an ongoing process that requires regular monitoring and optimization. Financial institutions should continuously evaluate AI models to ensure they remain effective in dynamic market conditions.
Best practices:
- Set up real-time monitoring systems to track AI model performance and detect anomalies
- Develop feedback loops to gather insights from end-users and stakeholders
- Regularly retrain AI models with updated data to maintain relevance and accuracy
Step 8: Foster a culture of innovation and adaptability
AI adoption requires a cultural shift that embraces innovation and agility. Leaders must create an environment that encourages experimentation, continuous learning, and rapid adaptation to change.
Best practices:
- Promote a mindset of experimentation, where teams are encouraged to test and refine AI solutions
- Recognize and reward innovation across the organization to drive adoption and engagement
- Stay informed about emerging AI trends and technologies to remain competitive
Implementing generative AI is a complex journey that requires strategic planning, cross-functional collaboration, and ongoing evaluation. By following these best practices, banks and CEOs can maximize the benefits of generative AI, ensuring it aligns with business objectives while driving meaningful outcomes. Institutions that get it right will gain a competitive advantage, improve operational efficiency, and deliver superior customer experiences, positioning themselves for long-term success in an AI-powered financial landscape.
“As AI continues to advance, financial services will move towards greater autonomy, personalization, and sustainability, paving the way for a more efficient and inclusive financial future.”
Responsible governance and ethics: Key priorities for financial leaders
Implementing generative AI in financial services requires financial leaders to prioritize transparency, fairness, and accountability. AI systems must be designed to ensure clear decision-making processes that can be explained to customers, regulators, and stakeholders. This transparency builds trust, particularly in sensitive areas like lending and investments, where "black box" models can undermine confidence and complicate compliance efforts.
Addressing bias is essential to ensure AI systems do not perpetuate inequalities in lending, underwriting, or hiring. Financial leaders must regularly audit models and use diverse datasets to promote fairness. Governance frameworks should also align AI initiatives with the institution’s ethical values, ensuring that AI enhances customer outcomes without reinforcing social disparities.
Data privacy and security are equally critical. As AI relies heavily on customer data, institutions must adhere to privacy regulations and implement privacy-by-design principles. Clear communication about how personal data is used further builds trust. In parallel, strong cybersecurity measures must protect AI systems from external threats, ensuring that only authorized personnel manage critical operations and data.
Governance frameworks should incorporate continuous monitoring, transparent reporting, and regular audits to maintain alignment with ethical standards and business goals. Financial institutions must remain agile, anticipating new regulations and engaging with industry bodies to shape future compliance standards. A proactive approach to governance not only mitigates risks but also establishes the institution as a leader in ethical innovation, strengthening relationships with customers and regulators alike.
By balancing innovation with responsibility, financial leaders can position AI as a driver of sustainable growth while maintaining the highest standards of governance and ethics. This approach ensures generative AI serves both business and societal interests, fostering trust and long-term success.
How generative AI is reshaping finance
The ongoing evolution of generative AI is set to transform financial services in profound ways, driving innovation and redefining operational practices. These emerging trends will shape the future of finance, offering both opportunities and challenges for institutions striving to remain competitive in a dynamic market.
Hyper-personalization
Hyper-personalization is becoming a standard expectation, with generative AI enabling financial institutions to deliver products and services tailored to individual customers. AI systems can analyze real-time data on spending behavior, preferences, and financial goals, allowing banks to offer dynamic recommendations for loans, investments, or savings plans. Hyper-personalization strengthens customer loyalty by delivering meaningful, context-aware interactions at every touchpoint.
Autonomous finance
Autonomous finance is poised to take financial automation to the next level. AI systems will handle routine financial tasks—such as bill payments, savings transfers, and portfolio rebalancing—without human intervention. With these tasks managed autonomously, individuals and businesses can shift focus to more complex financial planning and long-term strategies, confident that routine transactions are optimized in the background.
Predictive analytics
Predictive analytics will see significant advancements as AI models become better at forecasting market trends and financial outcomes. With access to more data and improved algorithms, predictive models will provide financial institutions with deeper insights into future market behavior, helping them mitigate risks and identify profitable opportunities. This capability will empower both customers and institutions to make better financial decisions with increased accuracy.
Conversational AI
Conversational AI will enhance customer engagement by enabling seamless, human-like interactions with virtual assistants and chatbots. Advances in natural language processing (NLP) will allow AI-powered systems to handle more sophisticated customer queries, providing support across multiple channels, including voice interfaces. Financial assistants will not only respond to inquiries but also proactively recommend financial strategies, strengthening customer relationships.
Blockchain and AI integration
Blockchain and AI integration will redefine how financial transactions are managed, enhancing security, transparency, and efficiency. Blockchain technology ensures trust and accountability, while AI improves transaction validation and fraud detection. Together, these technologies will streamline cross-border payments, smart contracts, and digital identities, creating a more secure and efficient financial ecosystem.
Augmented decision-making
Augmented decision-making will empower financial professionals by combining human expertise with AI-generated insights. In complex scenarios such as investment management, AI will provide real-time recommendations based on market conditions and historical data. This augmented approach allows decision-makers to make faster, more informed choices, improving performance across trading desks, risk management, and wealth advisory services.
Regulatory technology (RegTech)
Regulatory technology (RegTech) will evolve with AI, automating and enhancing compliance processes. As regulatory requirements become more complex, AI systems will monitor transactions, generate compliance reports, and detect non-compliant activities in real time. This reduces the burden on compliance teams while ensuring financial institutions remain aligned with evolving regulatory frameworks.
Sustainable finance
Sustainable finance will increasingly rely on AI for assessing and promoting environmentally and socially responsible investments. AI will analyze ESG (Environmental, Social, and Governance) metrics and forecast sustainability trends, helping institutions make data-driven decisions that align with societal values. This shift will encourage greater investment in sustainable initiatives, promoting long-term economic and environmental resilience.
These trends underscore how generative AI is reshaping finance by enabling smarter operations, deeper customer engagement, and more responsible investment strategies. Institutions that adopt these innovations will gain a significant competitive edge, becoming more agile, secure, and customer-focused. As AI continues to advance, financial services will move towards greater autonomy, personalization, and sustainability, paving the way for a more efficient and inclusive financial future.
Generative AI is poised to transform every aspect of the financial services industry, from customer interactions to back-office operations. By embracing this technology responsibly and strategically, financial institutions can unlock new levels of efficiency, innovation, and customer satisfaction. As the landscape continues to evolve, staying informed about the latest developments and best practices in generative AI will be crucial for maintaining a competitive edge in the rapidly changing world of finance.
table-of-contents
- What is generative AI and why it matters in financial services
- 6 generative AI use cases in banking and financial services
- Benefits of generative AI for financial institutions
- Challenges when adopting generative AI in finance
- Implementing generative AI for financial services
- Responsible governance and ethics: Key priorities for financial leaders
- How generative AI is reshaping finance
- Common questions about generative AI use cases in banking and financial services
Common questions about generative AI use cases in banking and financial services
What is the difference between generative AI and traditional AI?
How does generative AI improve fraud detection?
Can generative AI help financial institutions become more efficient?
How does AI support financial inclusion?
What challenges should financial institutions expect when adopting generative AI?
Want to learn how data services can bring more transparency and trust to your operations?