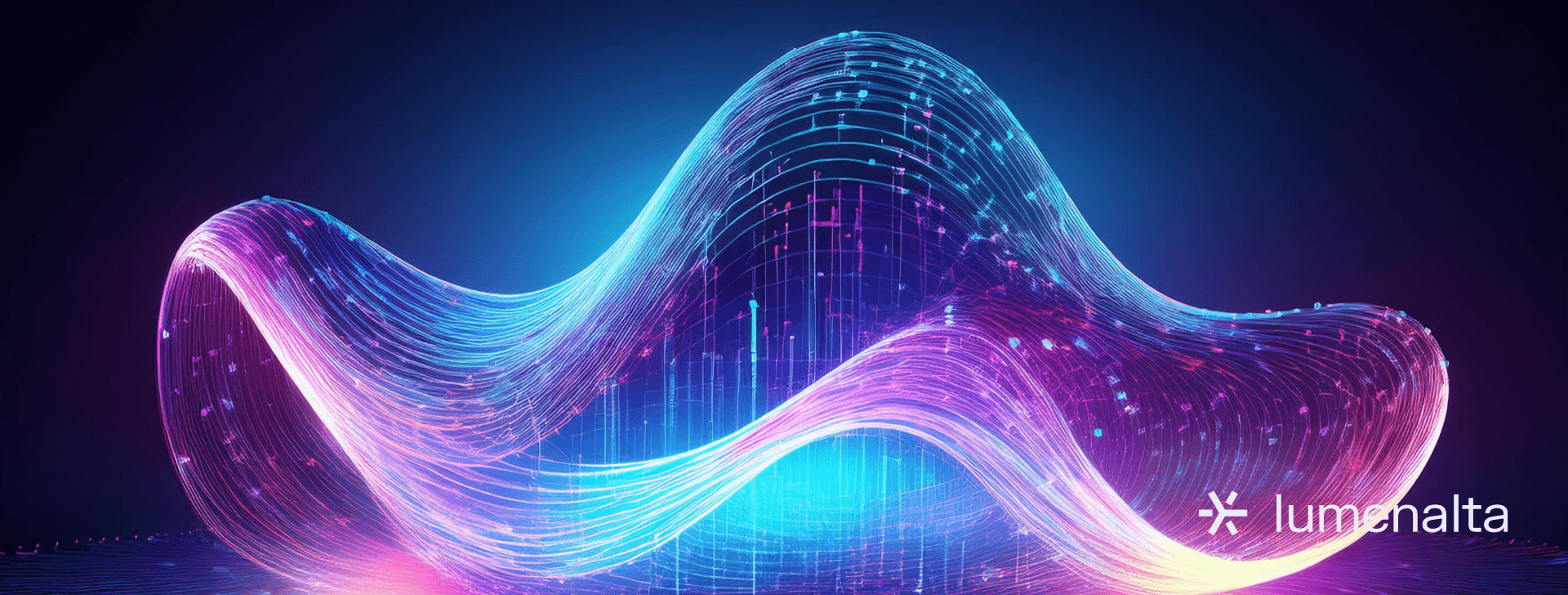
What is data intelligence?
NOV. 15, 2024
6 Min Read
Data intelligence is reimagining how organizations value their data, integrating analytics, governance, and operational strategies into one cohesive framework.
Turning raw data into actionable insights unlocks data intelligence, permitting more intelligent evaluation and greater efficiency. It combines advanced tools like machine learning, real-time feedback systems, and predictive analytics to find patterns and opportunities that were previously inaccessible.
Key components of data intelligence include data quality management, governance, and visualization. These elements ensure that insights are accurate, reliable, and accessible to decision-makers across the organization. By embedding strong governance practices, businesses can maintain data integrity and comply with regulatory standards while maximizing the strategic value of their data.
The benefits of data intelligence extend beyond operational efficiency to include innovative advantage. Using real-time analytics and advanced tools lets companies adapt quickly to market changes, optimize workflows, and unlock new growth opportunities. This holistic approach to managing and utilizing data is driving transformative success in every industry.
Key takeaways
- 1. Effective data management ensures data is accurate, accessible, and secure, forming a foundation for operational excellence and strategic growth.
- 2. Key components of a data management strategy include governance, architecture, quality, security, and analytics for actionable insights.
- 3. Varied platforms like DBMS, data lakes, and cloud systems cater to specific organizational needs, allowing scalable and efficient data handling.
- 4. Overcoming challenges such as data silos, security risks, and compliance requires targeted solutions and proactive management practices.
- 5. Future trends highlight the growing importance of AI, real-time processing, and sustainable practices in driving innovation and data-backed decisions.
Understanding data intelligence
Data intelligence refers to the practice of converting raw data into meaningful insights to guide management, improve efficiency, and drive innovation. It combines advanced analytics, artificial intelligence, and machine learning with data management to discover patterns and trends that traditional methods might miss. It integrates various data sources and applies real-time analytics, allowing organizations to understand past performance and predict future outcomes.
This approach is vital in an era where businesses deal with large volumes of data from various sources like IoT devices, customer interactions, and operational systems. Without a structured process to extract actionable insights, this data remains an untapped resource. Data intelligence bridges this gap, ensuring that data is accurate, accessible, and aligned with organizational goals, empowering informed decisions that deliver measurable business outcomes.
"Data intelligence bridges the gap by ensuring that data is accurate, accessible, and aligned with organizational goals, empowering informed decisions that deliver measurable business outcomes."
Key components of data intelligence
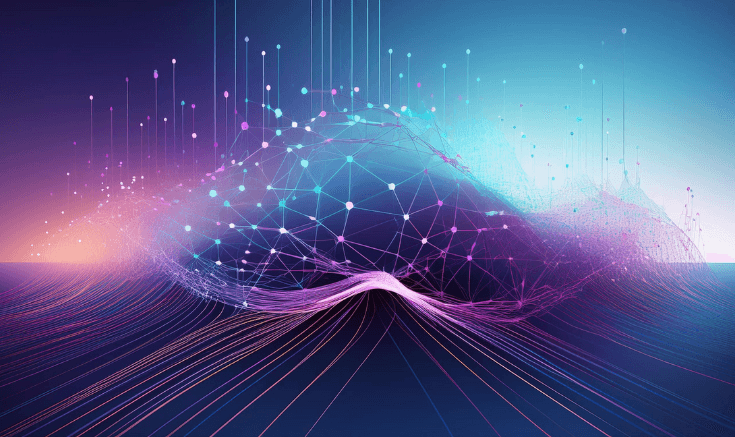
Data intelligence encompasses a series of connected components that allow organizations to modify data into strategic insights. These components go beyond simple data analysis, creating a system where information is not only processed but also refined, contextualized, and utilized effectively. Each component plays a unique role in ensuring that data serves its intended purpose, driving better decisions, enhancing operational performance, and supporting long-term goals. From capturing raw data to implementing advanced analytics, this framework ensures that every step contributes to actionable outcomes.
Data collection and integration
The foundation of data intelligence lies in gathering data from different sources and integrating it into a unified system. This step ensures all relevant data—whether structured, semi-structured, or unstructured—is available for analysis. Systems such as customer relationship management platforms, IoT sensors, and financial tools contribute streams of data that need seamless aggregation for maximum utility.
Data quality management
Maintaining high data quality is crucial for accurate insights. Errors, inconsistencies, and missing information can lead to flawed analyses and misguided decisions. This often involves cleansing processes and validation checks to eliminate redundancies or inaccuracies.
Advanced analytics
Analytics rebuilds raw data into meaningful insights through techniques like statistical analysis, predictive modeling, and machine learning. This component helps organizations locate hidden patterns, forecast trends, and identify opportunities that would otherwise remain unnoticed. Utilizing advanced analytics tools gives stakeholders an edge.
Data governance
Data governance ensures that data is used responsibly and securely across the organization. It involves setting policies, establishing roles, and maintaining compliance with regulatory requirements. Strong governance frameworks protect data integrity and build trust in how it is managed and shared.
Visualization and reporting
Insights derived from data intelligence are only impactful when effectively communicated. Visualization tools like dashboards and real-time reports translate complex datasets into understandable formats. These tools help stakeholders make informed decisions quickly, supported by clear and concise information.
Continuous monitoring and feedback
Modern business aspects require that data intelligence systems operate as iterative processes. Continuous monitoring identifies deviations or emerging trends, allowing businesses to adapt strategies promptly. Feedback loops enhance these systems, ensuring they progress to meet organizational needs.
Understanding these components provides a roadmap for organizations aiming to leverage data intelligence effectively. Each aspect contributes to a system where raw data is converted into a strategic asset, supporting objectives like operational excellence, enhanced governance, and long-term growth. This comprehensive framework ensures businesses remain agile and proactive.
Benefits of data intelligence for organizations
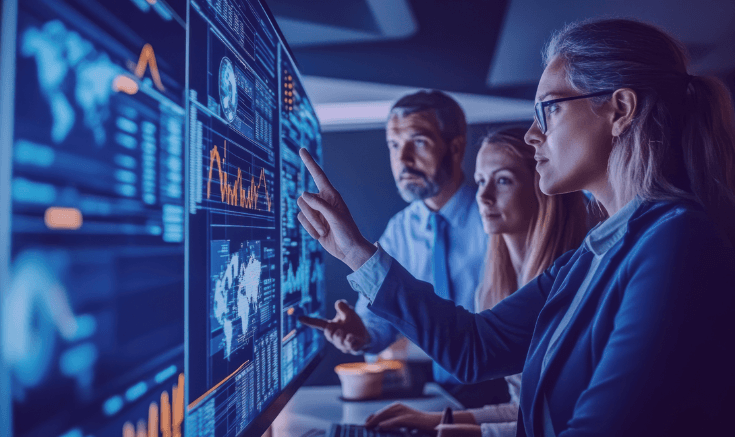
Data intelligence drives meaningful change across industries by giving organizations actionable insights that improve decision-making, operational efficiency, and data positioning. Its benefits span various domains, modifying how businesses operate and succeed.
- Improved evaluation: actionable insights fuel strategic choices. Data intelligence delivers precise, real-time insights, reducing guesswork and permitting informed decisions. Predictive analytics and trend forecasting help organizations anticipate challenges and capitalize on opportunities.
- Enhanced operational efficiency: streamlining processes saves resources. Data intelligence solves inefficiencies in workflows and processes, equipping businesses to optimize operations. Automation of repetitive tasks further reduces costs and accelerates delivery timelines.
- Increased revenue opportunities: identifying new markets and trends. By analyzing customer behavior, market dynamics, and historical data, organizations can find untapped opportunities, improve product offerings, and target high-value segments.
- Stronger customer relationships: personalized experiences build loyalty. Insights from data intelligence allow businesses to tailor their offerings, enhancing customer satisfaction and retention. Advanced customer analytics refine engagement strategies, fostering deeper connections.
- Improved risk management: mitigating threats proactively. Risk assessment becomes more effective with data intelligence, as it identifies vulnerabilities and monitors changes in risk factors. Financial forecasting, compliance tracking, and threat detection safeguard organizational stability.
- Better resource allocation: data-centric strategies boost efficiency. Organizations can prioritize investments and allocate resources effectively using intelligence-backed strategies. This ensures the highest impact from operational, marketing, and development efforts.
Data intelligence reshapes organizations’ capabilities by creating a framework for more intelligent decisions, optimized processes, and personalized interactions. With a robust approach, businesses can unlock efficiencies and innovation, establishing themselves as leaders in their respective industries.
Types of data intelligence applications
Data intelligence applications span several industries, each using insights to address unique challenges and opportunities. These applications demonstrate the versatility of data intelligence, driving value through innovation and improved regulation. Organizations use tailored approaches to utilizing data intelligence, reshaping operations, customer experiences, and market positioning.
- Customer analytics: creating tailored experiences. Organizations use data intelligence to analyze customer behavior and preferences. Insights guide personalized marketing campaigns, product recommendations, and customer service improvements, enhancing loyalty and retention.
- Operational optimization: driving efficiency across workflows. Data intelligence helps identify bottlenecks and inefficiencies in processes. Predictive maintenance in manufacturing, supply chain optimization in logistics, and automated scheduling are common examples.
- Financial insights: improving fiscal management. Applications in financial analytics include fraud detection, revenue forecasting, and expense optimization. Real-time tracking ensures better financial stability and strategic planning.
- Healthcare advancements: modifying patient outcomes. In healthcare, data intelligence supports diagnostics, treatment planning, and resource allocation. Predictive models improve patient outcomes by identifying risks early and optimizing care delivery.
- Risk management: mitigating potential disruptions. Data intelligence is pivotal in identifying and responding to risks. Insurance firms use it for accurate underwriting, while businesses leverage it for cybersecurity threat detection and regulatory compliance.
- Market intelligence: staying innovative. Analyzing competitor actions, market trends, and consumer feedback helps businesses refine strategies and innovate faster.
Each application showcases how data intelligence integrates with organizational goals to create measurable benefits. Aligning data intelligence with specific objectives enhances businesses' adaptability, efficiency, and resilience. This ensures that data intelligence is a tool for sustainable growth.
"Each of these applications showcases how data intelligence integrates with organizational goals to create measurable benefits."
Implementing data intelligence
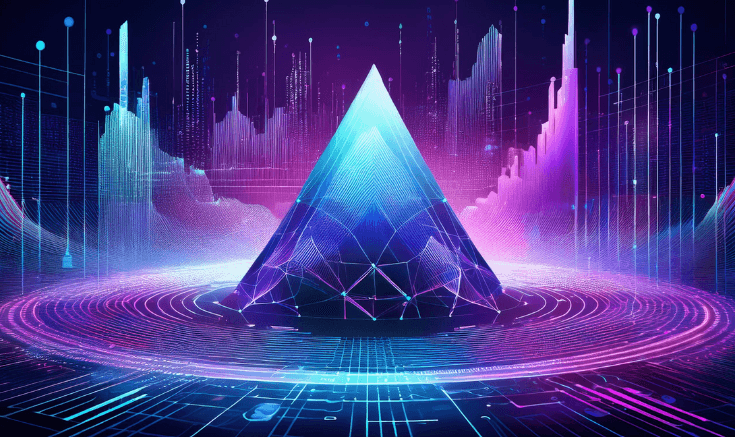
Implementing data intelligence requires a strategic approach that aligns with an organization’s goals, infrastructure, and capabilities. This process converts raw data into a valuable asset, allowing more intelligent decisions and greater efficiency. The journey involves careful planning, robust execution, and continuous refinement to ensure lasting impact.
Establishing clear objectives
A successful implementation starts with defining clear goals. These objectives guide the design and deployment of data intelligence systems, ensuring that insights directly address business priorities. Whether the focus is on improving customer engagement or optimizing operations, clarity is crucial for aligning resources and expectations.
Building a strong data foundation
A robust data infrastructure is essential for effective data intelligence. This includes integrating multiple data sources, ensuring data quality, and adopting scalable technologies. Cloud-based platforms, data warehouses, and real-time pipelines often form the pillar of modern data intelligence systems.
Utilizing the right expertise
Organizations must assemble a multidisciplinary team of data scientists, analysts, and business stakeholders. Collaboration ensures that technical solutions align with practical needs and strategic outcomes. Training and upskilling employees also play a key role in maintaining proficiency as technologies develop.
Prioritizing iterative processes
Data intelligence succeeds in adaptability. Implementing iterative development cycles, such as agile methodologies, allows teams to refine systems based on actual feedback. Regular evaluation of insights and their applications helps organizations stay aligned with business conditions.
Implementing data intelligence is not a one-size-fits-all effort. Each organization’s unique goals and challenges shape the approach, requiring a combination of technical expertise, operational flexibility, and strategic vision. This integrated strategy ensures that data intelligence becomes a driving force for sustainable growth and business advantages.
Data intelligence tools and technologies
The success of data intelligence initiatives relies heavily on selecting and deploying the right tools and technologies. These solutions facilitate every step of the process, from data collection to actionable insights, ensuring organizations achieve their strategic goals efficiently and effectively.
Organizations have a wealth of options to choose from, each offering unique capabilities tailored to specific business needs. These tools are not just technological assets; they are the foundation of modern management processes.
- Data integration platforms: simplifying data aggregation. Tools like ETL (extract, transform, load) systems unify data from various sources, implementing seamless integration and preparation for analysis. They ensure data consistency and readiness for downstream processes.
- Analytics software: Building actionable insights. Platforms such as business intelligence tools offer dashboards, predictive models, and advanced analytics capabilities. These tools help users identify trends, forecast outcomes, and make data-led decisions.
- Artificial intelligence and machine learning frameworks: driving automation and predictions. AI and machine learning technologies automate repetitive tasks and find hidden patterns in data. These frameworks accelerate innovation and improve accuracy in applications like customer segmentation and fraud detection.
- Data visualization tools: communicating insights effectively. Tools such as Tableau and Power BI translate complex datasets into visually intuitive charts and reports. They let stakeholders grasp critical insights at a glance, improving collaboration and regulation.
- Data governance solutions: ensuring compliance and security. Technologies for data governance focus on managing access, ensuring regulatory compliance, and protecting sensitive information. These tools establish trust and accountability in data use.
- Cloud-based platforms: facilitating scalability and accessibility. Cloud services offer scalable storage and computing power, making them ideal for organizations with growing data needs. They support real-time analytics and promote global collaboration.
These tools work together to form a comprehensive ecosystem that supports data intelligence initiatives. With the right combination of technologies, organizations can convert raw data into a strategic asset, driving innovation, efficiency, and growth.
"Embedding data governance into business intelligence efforts secures a dynamic edge while maintaining the integrity and security of an organization’s most valuable resource—data."
Challenges in data intelligence adoption
While data intelligence offers transformative potential, organizations often face significant hurdles during its adoption. These challenges can impede progress, increase costs, and reduce the overall effectiveness of data initiatives. Addressing these obstacles requires a proactive strategy, aligned resources, and organizational commitment.
- Data silos: hindering integration efforts. Disconnected systems and departments create data silos, making it difficult to access and unify data for analysis. Overcoming this challenge requires robust data integration strategies and cross-departmental collaboration.
- Data quality issues: reducing trust in insights. Poor-quality data with inaccuracies, inconsistencies, or missing values leads to unreliable results. Implementing rigorous data cleansing processes and ongoing quality assurance measures is essential to maintain credibility.
- Skills gap: limiting expertise in advanced analytics. Many organizations struggle with a lack of skilled personnel to implement and manage data intelligence systems. Investing in employee training, hiring specialized talent, or outsourcing expertise can bridge this gap.
- High implementation costs: straining budgets. Deploying comprehensive data intelligence solutions often requires significant upfront investment in tools, infrastructure, and talent. Opting for scalable, cloud-based platforms can help reduce initial costs.
- Privacy and compliance concerns: creating regulatory risks. Adhering to laws like GDPR or HIPAA is a critical challenge, especially when managing sensitive data. Robust data governance frameworks and compliance tools ensure adherence to legal requirements.
- Resistance to change: slowing organizational adoption. Cultural resistance and skepticism from employees can hinder adoption. Clear communication of benefits, leadership buy-in, and involving teams early in the process are key to fostering acceptance.
These challenges highlight the complexity of adopting data intelligence systems and underscore the importance of strategic planning and resource allocation. Overcoming these obstacles allows organizations to fully leverage the potential of data intelligence, reshaping challenges into opportunities for innovation and growth.
Data intelligence vs. data analytics
Data intelligence and data analytics are connected concepts often used interchangeably, but they represent distinct aspects of working with data. Understanding their differences helps organizations determine where to focus their efforts for maximum impact.
Data analytics centers on examining raw data to identify patterns, trends, and correlations. It typically involves methods like statistical analysis, predictive modeling, and machine learning. The goal is to interpret the data and generate actionable insights for specific use cases. Data analytics tools are widely used in reporting, forecasting, and problem-solving across industries.
Data intelligence, on the other hand, encompasses a broader scope. It integrates data analytics with strategic context, governance, and operational processes. While analytics focuses on generating insights, intelligence ensures these insights are actionable, aligned with organizational goals, and securely managed. It also emphasizes using data to predict, adapt, and innovate at a systemic level.
The difference lies in scope and application: data analytics provides the "what" and "why," while data intelligence focuses on the "how" and "what's next." They allow organizations to optimize their data use and drive meaningful outcomes.
Importance of data governance in business intelligence
Data governance is a critical component of successful business intelligence initiatives, ensuring that data is reliable, secure, and used responsibly. It provides the framework for managing data assets, aligning them with organizational goals, and maintaining compliance with legal and ethical standards.
Effective data governance establishes clear policies and procedures for data access, quality, and accountability. These measures build trust in the data and ensure those making decisions rely on insights with confidence. Governance frameworks also mitigate risks associated with data breaches, mismanagement, or non-compliance with regulations such as GDPR or CCPA.
Regulatory compliance and operational efficiency improve when data governance is prioritized. Reducing redundancies, ensuring data consistency, and enhancing collaboration across teams become achievable through standardized practices. These benefits create a strong foundation for business intelligence tools, letting organizations make informed decisions aligning with their strategic objectives.
Embedding data governance into business intelligence efforts secures an industry edge while maintaining the integrity and security of an organization’s most valuable resource—data. This alignment drives better evaluation and fosters innovation and long-term sustainability.
Data intelligence has become a foundation of modern business operations, offering the tools and frameworks to convert raw data into actionable insights. From its foundational components to its vast applications, data intelligence allows organizations to improve control, enhance efficiency, and achieve long-term growth. Overcoming challenges and using the right tools ensures a comprehensive approach to adopting this transformative strategy.
Data intelligence isn’t just about managing information—it’s about unlocking strategic opportunities that reimagine business outcomes. Organizations can drive better decisions and more resilient operations by integrating advanced analytics, governance, and real-time insights. At Lumenalta, we create tailored data intelligence solutions that align with your goals, helping you achieve measurable results.
Let’s shape a future powered by intelligent data.
Table of contents
- Understanding data intelligence
- Key components of data intelligence
- Benefits of data intelligence for organizations
- Types of data intelligence applications
- Implementing data intelligence
- Data intelligence tools and technologies
- Challenges in data intelligence adoption
- Data intelligence vs. data analytics
- Importance of data governance in business intelligence
- Common questions about data intelligence
Common questions about data intelligence
What is the purpose of data intelligence in business?
How is data intelligence different from data analytics?
What tools are commonly used in data intelligence?
Why is data governance essential for effective business intelligence?
How can small businesses adopt data intelligence cost-effectively?
Want to learn how data intelligence can bring more transparency and trust to your operations?