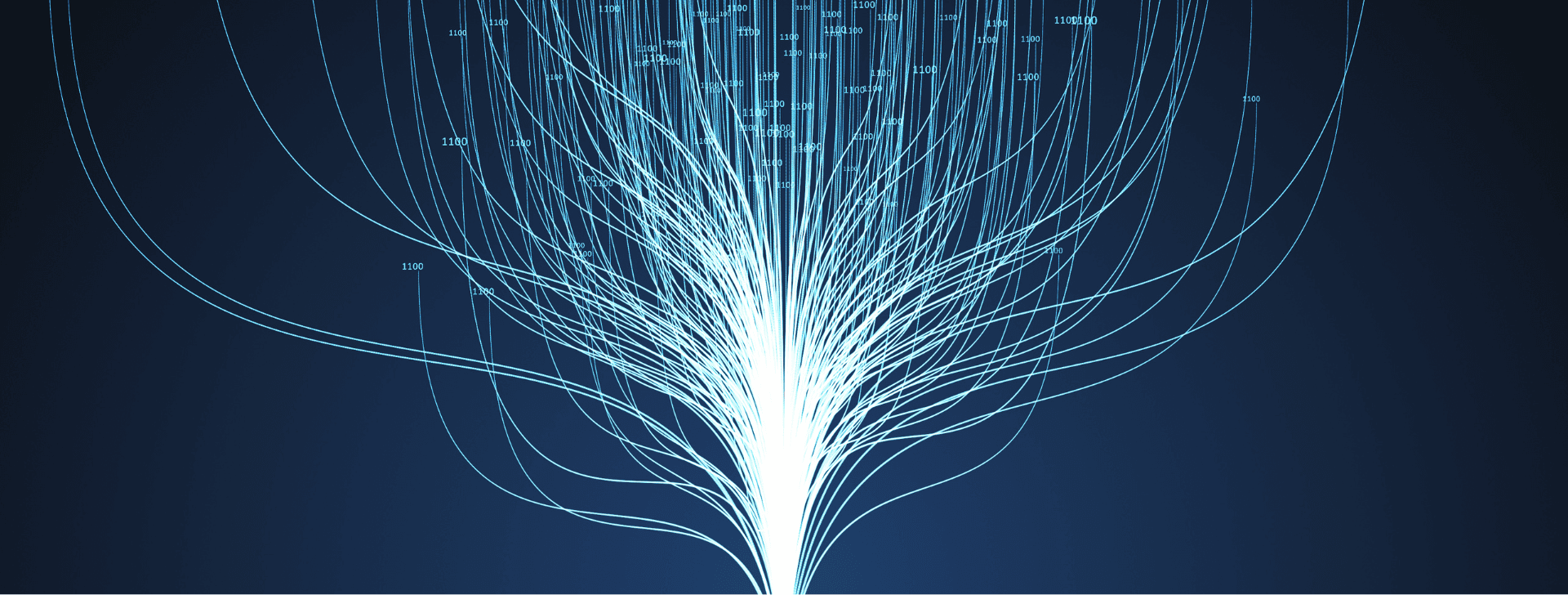
AI's impact on modern data governance strategies
OCT. 3, 2024
8 Min Read
AI-driven data governance has emerged as a crucial tool in transforming data into a strategic asset.
Leveraging AI to streamline data governance frameworks not only addresses traditional data management challenges but also creates new opportunities for scalable, compliant, and high-quality data processes. Understanding the interplay between AI and data governance is essential for enterprises aiming for sustained digital innovation.
Key takeaways
- 1. AI significantly enhances data quality and accuracy by automating data cleansing, anomaly detection, and standardization, making data more reliable for decision-making.
- 2. AI-driven data governance boosts decision-making capabilities, allowing businesses to make faster, data-driven choices through predictive analytics and real-time insights.
- 3. Scalability and flexibility are key strengths of AI, enabling organizations to manage growing data volumes seamlessly without compromising performance or efficiency.
- 4. AI helps address data security and compliance challenges by automating regulatory monitoring, risk detection, and advanced data privacy measures to meet industry standards.
- 5. Emerging trends like federated learning and synthetic data generation are transforming how organizations use AI for data governance, enhancing data privacy and model accuracy.
Why data governance needs AI today
The explosion of data and complexity
Data volumes are growing at an unprecedented rate, driven by advancements in AI and machine learning (ML). As organizations harness data for competitive advantage, managing this explosion has become increasingly challenging. Businesses need robust data governance frameworks that can handle structured, unstructured, and semi-structured data while maintaining data integrity and compliance.
AI plays a dual role in addressing these challenges—helping organizations not only manage data complexity but also extract valuable insights to drive decision-making. Leveraging AI for data governance enables businesses to transition from reactive data management to proactive data strategies.
Key components of AI-driven data governance
Understanding the key components of AI-driven data governance is crucial for organizations aiming to maximize the value derived from their data assets. AI enhances various aspects of data management by introducing automation, precision, and scalability into traditional governance practices.
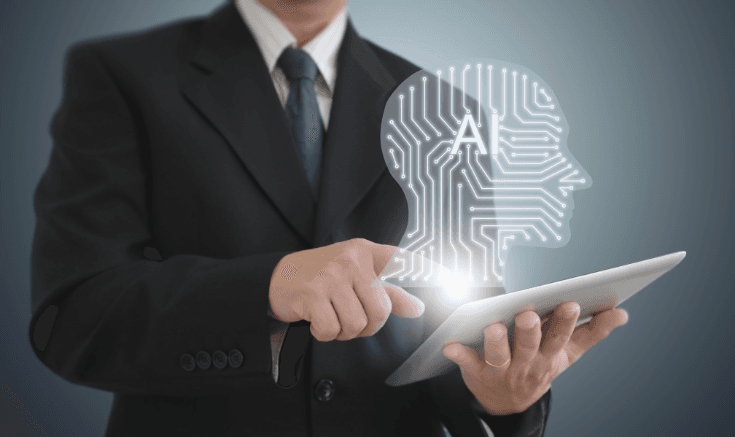
1. Automating data quality and integrity checks
AI technologies like machine learning algorithms continuously monitor data to identify inconsistencies, correct inaccuracies, and ensure data remains high-quality.
- Data cleansing: AI automatically cleans and normalizes data, reducing errors and improving accuracy.
- Anomaly detection: Machine learning models identify patterns and outliers in real time, ensuring timely intervention for data discrepancies.
2. Enhancing data security and compliance
AI tools play a pivotal role in ensuring that data is secure, compliant, and protected from unauthorized access.
- Regulatory compliance automation: AI-driven solutions automate the interpretation and enforcement of compliance requirements, minimizing risks of breaches.
- Advanced encryption: AI optimizes encryption protocols to secure sensitive data against cyber threats.
3. Intelligent data cataloging and classification
AI technologies streamline data cataloging by automatically tagging and organizing data based on its content and context.
- Metadata management: AI-driven tools create and maintain detailed metadata, improving data discoverability and usage.
- Data lineage tracking: Enhanced tracking capabilities help trace data transformations across its lifecycle, aiding in audits and compliance.
4. Proactive risk management
AI in data governance empowers organizations to anticipate potential risks and take preventive measures to mitigate them.
- Predictive analysis: AI models analyze historical data trends to predict future risks and recommend risk-mitigation strategies.
- Real-time alerts: Automated alerts notify stakeholders of potential issues, enabling quicker responses to data breaches.
5. AI-powered data integration
Seamlessly integrating data from multiple sources is essential for a unified view of an organization's data landscape.
- Data unification: AI tools enable the merging of disparate data sets into a cohesive structure, ensuring consistency.
- Scalability: AI-driven integration platforms can scale as the organization grows, handling larger data volumes efficiently.
Understanding these key components allows organizations to implement a holistic AI-driven data governance strategy that not only ensures data accuracy and security but also drives more informed business decisions and greater operational efficiency.
AI and data lineage: Tracking data across its lifecycle
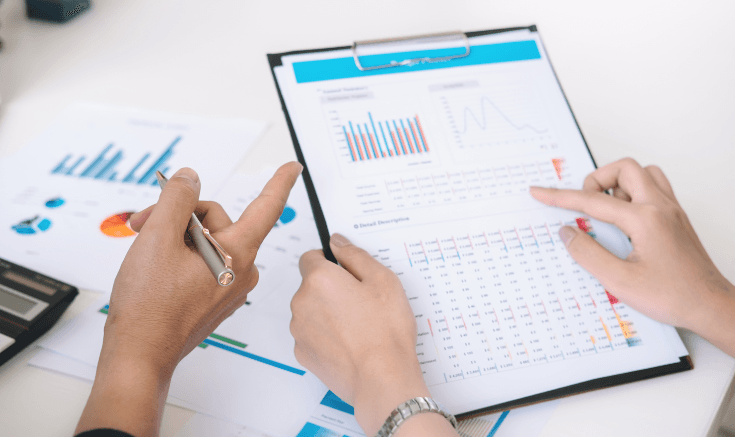
Data lineage refers to the tracking of data’s origins, its movements, and any transformations it undergoes throughout its lifecycle. AI enhances data lineage by providing automated, precise tracking that aids in compliance, auditing, and decision-making.
Importance of data lineage in AI-driven data governance
Implementing data lineage strategies is crucial for ensuring data reliability and traceability, especially in industries where regulatory compliance and data accuracy are paramount.
- Transparency in data transformation: AI enables detailed tracking of how data evolves over time, making it easier to identify and rectify issues.
- Audit readiness: Automated lineage tracking simplifies the auditing process, ensuring that all data handling practices comply with industry regulations.
- Data quality assurance: Maintaining a clear lineage helps organizations verify data accuracy, enhancing the reliability of analytics and reporting.
- Root cause analysis: AI tools can quickly pinpoint the source of data errors, reducing downtime and improving data trustworthiness.
- Regulatory compliance: Data lineage is essential for meeting compliance standards like GDPR and HIPAA, where understanding data flow and access points is critical.
With AI-enhanced data lineage, organizations gain unprecedented visibility into their data processes, ensuring that every transformation, usage, and modification is accurately documented and compliant with industry standards.
"Leveraging AI to streamline data governance frameworks not only addresses traditional data management challenges but also creates new opportunities for scalable, compliant, and high-quality data processes."
Use cases for AI in data governance across departments
Implementing AI in data governance can have transformative impacts on various departments within an organization. By tailoring AI applications to specific scenarios, companies can address unique challenges in sales, marketing, operations, and IT with precision and efficiency.
AI for sales data management
AI can enhance the effectiveness of sales teams by automating data-related tasks and providing actionable insights.
- Real-time sales intelligence: AI systems can analyze customer data to generate insights into buying patterns, enabling personalized engagement strategies that increase conversion rates.
- Automated data entry and CRM updates: Reducing manual data entry by automating the process ensures that sales teams focus more on customer interactions rather than administrative tasks.
- Predictive analytics for sales forecasting: AI algorithms can predict future sales trends by analyzing historical data, helping businesses optimize inventory and align their sales strategies with market demand.
AI in marketing for hyper-personalization
In marketing, AI-powered data governance ensures that campaigns are not only personalized but also aligned with privacy regulations.
- Customer segmentation and targeting: AI-driven analytics can categorize customers based on behavior and preferences, leading to more targeted marketing efforts.
- Real-time personalization: AI algorithms can tailor marketing messages in real time, increasing engagement by delivering relevant content at the right moment.
- Data compliance in campaigns: Leveraging AI helps marketers ensure that all customer data used in campaigns complies with privacy laws such as GDPR and CCPA.
AI for predictive maintenance in operations
AI enables a proactive approach in operational environments, significantly reducing equipment downtime and maintenance costs.
- Sensor data analysis: AI models process large volumes of sensor data from machinery to identify anomalies and predict potential breakdowns.
- Maintenance scheduling: Automated systems can schedule maintenance activities based on predictive analytics, optimizing asset performance and extending equipment life.
- Reducing operational costs: By predicting failures before they occur, AI reduces the need for emergency repairs and minimizes disruptions in operations.
AI in IT for data security and risk management
The IT department can leverage AI to enhance data security protocols and streamline risk management.
- Threat detection and prevention: AI-driven systems monitor network activities and detect suspicious behavior patterns in real time to prevent cyberattacks.
- Automated incident response: AI tools can quickly react to security breaches by isolating affected systems and alerting relevant stakeholders.
- Data access management: Machine learning models can enforce dynamic access controls, ensuring that sensitive data is accessible only to authorized personnel.
Integrating AI into these departmental functions not only enhances data governance but also drives efficiency and innovation across the entire organization. Tailored AI applications transform data from a static asset into a dynamic tool for strategic growth.
AI use cases in data governance across departments
Implementing AI in data governance offers transformative opportunities by automating workflows, improving data quality, and enhancing the customer experience across various business functions. Here are some key examples:
- Automating internal workflows: AI-driven data governance tools streamline routine tasks, such as data entry, documentation, and quality monitoring. This automation reduces human error, saves time, and allows employees to focus on strategic initiatives instead of manual processes.
- Scaling content generation: AI assists in generating and managing content at scale by organizing metadata, automating classification, and ensuring that all content adheres to governance policies. This capability supports content-rich industries such as media, e-commerce, and marketing, where real-time content updates are crucial.
- Enhancing customer experiences through AI-assisted search: With AI-enhanced data governance, companies can leverage natural language processing (NLP) to offer intelligent search experiences. AI ensures that data powering search engines is accurate, complete, and compliant, leading to more personalized and relevant search results. This is particularly valuable in industries like retail, banking, and telecommunications, where enhanced search functionality directly impacts customer satisfaction and engagement.
Business benefits of AI-enabled data governance
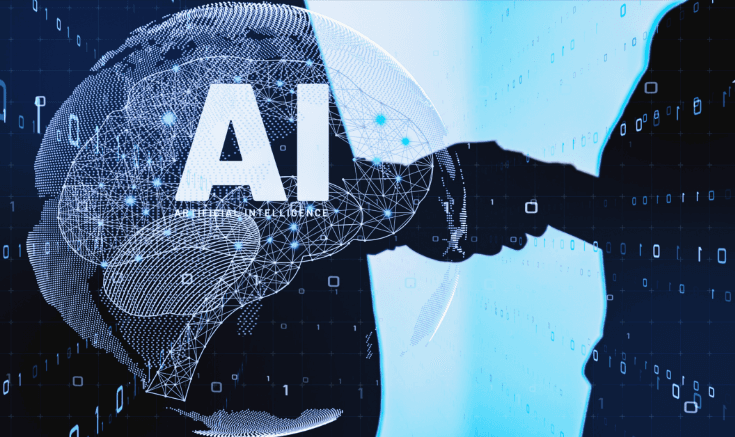
AI-driven data governance offers a wide array of benefits that revolutionize how organizations manage, analyze, and leverage their data. By integrating AI, companies can transform data into a strategic asset that drives innovation, reduces costs, and improves decision-making capabilities.
1. Enhanced data quality and accuracy
One of the most significant benefits of AI in data governance is its ability to consistently improve data quality and accuracy.
- Automated data cleansing: AI tools can automatically detect and correct errors in data sets, such as duplicates, missing values, and inconsistencies, without the need for manual intervention.
- Data standardization: AI algorithms ensure that data formats remain consistent across various sources and systems, which is crucial for accurate analysis and reporting.
- Real-time quality monitoring: AI continuously monitors data quality in real time, instantly identifying anomalies and ensuring data remains reliable for decision-making.
2. Improved decision-making capabilities
AI enhances decision-making by providing accurate and actionable insights from vast data sets, enabling businesses to make data-driven choices.
- Predictive analytics: AI models analyze historical data trends to forecast future scenarios, helping companies anticipate market changes and adapt their strategies.
- Data-driven strategies: The insights derived from AI analytics enable businesses to base their strategies on factual data rather than intuition, reducing risks and increasing the likelihood of success.
- Faster decision-making: AI processes data much faster than traditional methods, allowing decision-makers to respond quickly to emerging trends or challenges.
3. Increased scalability and flexibility
Scalability is a key benefit of AI-driven data governance, allowing organizations to manage growing data volumes efficiently without compromising on performance.
- Seamless integration: AI platforms easily integrate with existing data infrastructures, enabling businesses to expand their capabilities as data demands grow.
- Elastic data management: AI solutions adjust dynamically to handle varying data loads, providing flexibility to scale up or down based on organizational needs.
- Support for big data: AI-driven tools are designed to process large datasets in real time, ensuring that even massive data volumes do not overwhelm governance processes.
4. Cost efficiency and resource optimization
By automating routine data management tasks, AI helps reduce costs and optimize resource allocation within the organization.
- Reduced manual labor: Automation minimizes the need for human intervention in data entry, validation, and cleansing, freeing up resources for more strategic tasks.
- Lower operational costs: AI-driven efficiencies translate into significant cost savings by streamlining data processes and reducing time spent on manual tasks.
- Optimized resource utilization: Resources can be redirected from repetitive data tasks to value-generating activities like innovation and strategic planning.
5. Advanced analytics and business insights
AI provides sophisticated analytical capabilities that transform raw data into meaningful business insights, driving innovation and growth.
- Advanced data visualization: AI-powered analytics tools create intuitive visualizations that make complex data easier to understand, helping stakeholders grasp insights quickly.
- AI-driven market analysis: AI tools analyze market trends and customer behaviors, enabling businesses to develop targeted marketing strategies and product offerings.
- Real-time business intelligence: Organizations gain real-time insights into performance metrics, allowing them to make agile decisions based on the latest data.
6. Strengthened compliance and risk management
AI enhances an organization’s ability to adhere to regulatory standards and manage risks effectively by automating compliance processes.
- Automated compliance monitoring: AI systems continuously monitor data activities to ensure that they align with industry regulations like GDPR, HIPAA, or CCPA.
- Risk detection and mitigation: Machine learning models identify potential compliance risks and trigger alerts before they escalate into costly issues.
- Improved audit trails: AI tools generate comprehensive audit trails, documenting every data transaction, which is crucial for compliance reviews and legal defenses.
The diverse benefits of AI-enabled data governance go beyond operational efficiencies; they empower organizations to harness data strategically, unlocking new opportunities for growth, innovation, and competitive advantage.
Challenges in AI-driven data governance
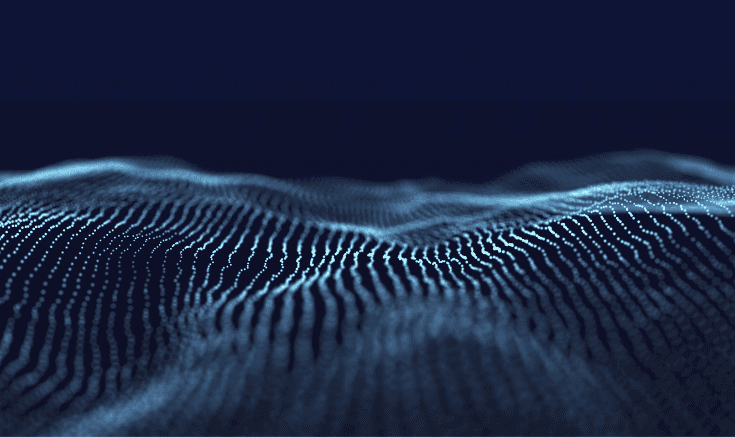
While AI-driven data governance offers numerous advantages, it also presents challenges that businesses must address to leverage its full potential effectively. These challenges range from ethical concerns to technological integration issues.
1. Data privacy and ethical concerns
Data privacy is a top priority for organizations, and AI technologies can sometimes pose risks if not properly managed.
- Bias in AI models: AI systems can inadvertently introduce biases into data analysis, leading to skewed results and potential ethical issues.
- Data privacy regulations: Navigating strict regulations like GDPR and CCPA can be challenging when using AI, as data handling practices must be meticulously documented and transparent.
- Transparency in AI decisions: Ensuring AI's decision-making processes are transparent and explainable is crucial for maintaining stakeholder trust and compliance.
2. Integration with legacy systems
Integrating AI-driven data governance solutions with existing legacy systems can be a significant challenge for many organizations.
- Compatibility issues: Legacy systems may not be compatible with modern AI tools, leading to delays and increased costs in implementation.
- Data silos: Fragmented data sources across legacy systems can hinder AI’s ability to provide a unified view of the data landscape.
- Cost of upgradation: Upgrading legacy infrastructure to accommodate AI capabilities can be expensive and require substantial resource investment.
3. Managing AI biases and transparency
AI biases can distort decision-making processes and lead to unintended consequences if not properly managed.
- Algorithmic bias: AI models trained on biased data sets may reinforce existing prejudices, impacting decision-making in areas like hiring or customer targeting.
- Lack of explainability: Black-box AI models make it difficult to understand how decisions are made, raising transparency concerns for regulators and stakeholders.
- Accountability issues: Establishing clear accountability for AI-driven decisions is crucial for mitigating risks and ensuring responsible AI deployment.
4. High implementation costs
The initial investment in AI technologies can be prohibitive for many organizations, especially smaller enterprises.
- Infrastructure costs: Implementing AI-driven data governance requires significant investment in computing power, data storage, and integration tools.
- Training and development: Organizations need to invest in upskilling their workforce to manage and operate AI technologies effectively.
- Return on investment (ROI) concerns: Calculating the ROI of AI-driven data governance can be complex, making it difficult to justify the initial outlay.
5. Skill gaps in workforce
The lack of skilled professionals in AI and data science can be a bottleneck for organizations seeking to implement AI-driven data governance.
- Talent shortage: There is a global shortage of data scientists and AI specialists capable of building and managing AI-driven data governance systems.
- Training requirements: Employees require comprehensive training to understand AI tools and how to use them effectively within data governance frameworks.
- Adaptability issues: Resistance to adopting new technologies can slow down the transition to AI-enabled data governance.
6. Data silos and fragmented data ecosystems
Data silos within organizations can prevent seamless data flow and limit the effectiveness of AI in data governance.
- Inconsistent data formats: Different departments may store data in varied formats, making it difficult to integrate and analyze using AI tools.
- Communication barriers: Lack of coordination between teams exacerbates data silos, reducing the overall efficiency of data governance.
- Reduced data accessibility: Fragmented data systems limit AI's ability to provide holistic insights, hindering data-driven decision-making.
Understanding these challenges and addressing them proactively is essential for maximizing the impact of AI-driven data governance and ensuring a smooth transition to advanced data management practices.
"AI provides sophisticated analytical capabilities that transform raw data into meaningful business insights, driving innovation and growth."
The role of change management and organizational readiness in AI-driven data governance
Implementing AI-driven data governance requires more than just the right technologies; it demands a comprehensive change management strategy and organizational readiness to ensure smooth adoption and long-term success. Without these elements, even the most advanced AI solutions may fail to deliver their full potential.
Why change management matters
AI-driven data governance transforms traditional processes by introducing automation, real-time monitoring, and intelligent decision-making frameworks. However, the transition can strain teams balancing both legacy systems and new AI tools, as highlighted by research in the whitepaper. Nearly half of surveyed organizations maintain legacy systems alongside AI/ML implementations to ensure smooth integration. This dual focus can create operational bottlenecks and resistance to change, making effective change management essential.
Key elements of change management in AI-driven governance:
- Stakeholder engagement: Involving key stakeholders from the outset ensures alignment with strategic objectives and builds trust across departments. Engaged leadership plays a critical role—according to the whitepaper, cultural buy-in and centralized ownership are essential for sustainable data governance efforts.
- Training and upskilling: Employees must be equipped with the skills and knowledge required to operate AI-driven tools effectively. With 97% of organizations reporting complexity as a major barrier to AI adoption, continuous learning programs are crucial for reducing disruptions and enabling teams to adapt quickly to new technologies.
- Clear communication: Open communication channels are essential to convey the benefits and goals of AI-driven data governance. Teams must understand how these changes will impact their roles and contribute to business outcomes like enhanced data quality and decision-making, reported by 96% and 98% of organizations respectively.
Organizational readiness for AI-driven governance
A high level of organizational readiness is necessary to integrate AI into governance frameworks seamlessly. Readiness involves more than just technical preparedness—it requires a shift toward data-centric and agile operations. Organizations must:
- Evaluate current infrastructure: Ensuring that existing systems can integrate with AI tools is essential for success. As mentioned in the whitepaper, 33% of organizations still lack compliance and audit management tools, which can complicate AI adoption.
- Establish cross-functional collaboration: AI-driven data governance affects multiple departments, including IT, operations, and compliance. Building cross-functional teams ensures all perspectives are considered, helping address challenges such as data silos and alignment across systems.
- Monitor progress and iterate: Change management is not a one-time activity but an ongoing process. Regular monitoring, supported by KPIs such as data quality metrics and compliance rates, ensures governance strategies evolve to meet changing business and regulatory needs.
The impact of successful change management
When change management is executed effectively, organizations can realize the full benefits of AI-driven data governance. Employees feel empowered, workflows become more efficient, and governance efforts align with strategic goals. Additionally, organizational readiness ensures that enterprises can scale governance frameworks efficiently as data volumes grow and technologies evolve.
By focusing on cultural alignment, centralized ownership, and continuous improvement, organizations can ensure their AI-driven governance initiatives deliver sustained business value—creating a foundation for innovation, compliance, and long-term success.
Future trends in AI-enabled data governance
The future of AI in data governance is evolving rapidly, with several emerging trends set to reshape how organizations manage and leverage their data.
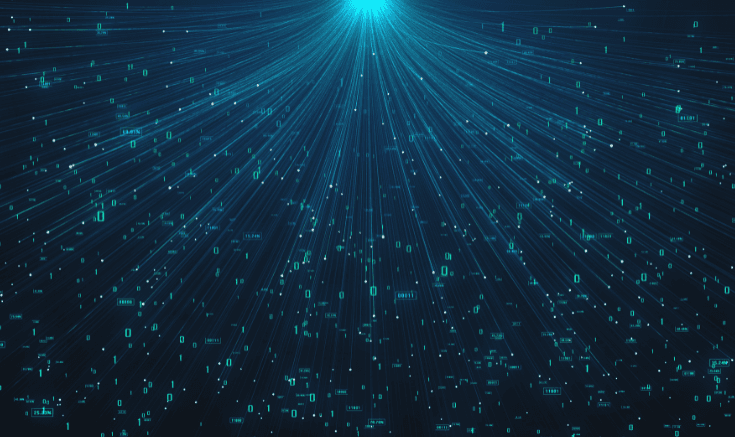
1. Real-time data processing and governance
As businesses demand more agility, dynamic data processing has become a critical component of data governance strategies.
- Immediate data insights: AI tools that process data as it arrives enable organizations to make faster, data-driven decisions.
- Automated data actions: Adaptive governance systems automatically trigger responses to data events, reducing latency in decision-making.
- Improved operational efficiency: Real-time analytics streamlines operations, enabling quick adaptations to changing market conditions.
2. Federated learning models
Federated learning is emerging as a key trend that allows AI models to train on decentralized data without compromising privacy.
- Data privacy enhancements: Federated learning processes data locally, minimizing the need to share sensitive information across networks.
- Collaborative learning: Multiple organizations can collaborate on AI model training without directly sharing their data, promoting innovation.
- Security-driven insights: This approach reduces the risks associated with data breaches, making it ideal for industries with stringent privacy requirements.
3. Synthetic data generation
The creation of synthetic data is becoming more prevalent as organizations look to enhance AI model training while protecting privacy.
- Data scarcity solutions: Synthetic data helps overcome challenges related to the lack of available real-world data for training AI models.
- Enhanced model accuracy: AI models trained on synthetic data can achieve higher accuracy, as they have more diverse and varied datasets.
- Privacy compliance: Generating synthetic data reduces reliance on actual sensitive data, making it easier to comply with data protection laws.
4. Automated compliance management
AI is set to revolutionize compliance management by automating the monitoring and enforcement of regulatory standards.
- Proactive risk detection: AI tools identify compliance risks in real time, allowing businesses to take preventive measures before violations occur.
- Audit simplification: Automated systems streamline the audit process by providing clear, accurate records of all data activities.
- Regulatory adaptability: AI systems can quickly adapt to new regulations, ensuring continuous compliance with evolving legal standards.
5. Data mesh architecture
Data mesh is transforming how organizations think about data governance by decentralizing data management across business units.
- Distributed data ownership: Each business unit takes ownership of its data, fostering better accountability and data quality.
- Scalable architecture: The data mesh approach supports scalability by organizing data into logical domains managed independently.
- Enhanced collaboration: Teams can collaborate more effectively, using domain-specific knowledge to manage and utilize their data assets.
6. AI-powered data privacy solutions
As data privacy regulations become more stringent, AI-driven tools are being developed to offer robust privacy protection measures.
- Data masking and anonymization: AI tools automate the process of masking or anonymizing sensitive data, protecting user privacy.
- Secure data sharing: AI enables secure methods for data sharing, ensuring that information is only accessible to authorized entities.
- Compliance with global standards: Advanced AI solutions ensure that data privacy practices align with international regulations, reducing the risk of non-compliance.
AI is reshaping the landscape of data governance by automating critical processes and enhancing data quality, security, and compliance. Enterprises that embrace AI-driven data governance gain not only operational efficiencies but also a strategic advantage in making data-driven decisions. As data volumes continue to grow and regulations evolve, integrating AI into data governance frameworks will be essential for sustaining business innovation and achieving competitive differentiation.
Lumenalta’s AI-driven solutions are designed to guide your organization through this transformative journey, ensuring that your data governance strategy is both innovative and resilient. Explore how our services can modernize your enterprise data governance today.
Table of contents
- Why data governance needs AI today
- Key components of AI-driven data governance
- AI and data lineage: Tracking data across its lifecycle
- Use cases for AI in data governance across departments
- Business benefits of AI-enabled data governance
- Challenges in AI-driven data governance
- The role of change management and organizational readiness in AI-driven data governance
- Future trends in AI-enabled data governance
- Common questions about data governance
Common questions about data governance
How does AI improve data governance in enterprises?
What are the main benefits of AI in data governance?
What challenges do businesses face with AI-driven data governance?
What are the future trends in AI and data governance?
Why is data lineage important in AI-driven data governance?
Want to learn how artificial intelligence can bring more transparency and trust to your operations?