Benefits & challenges of data governance
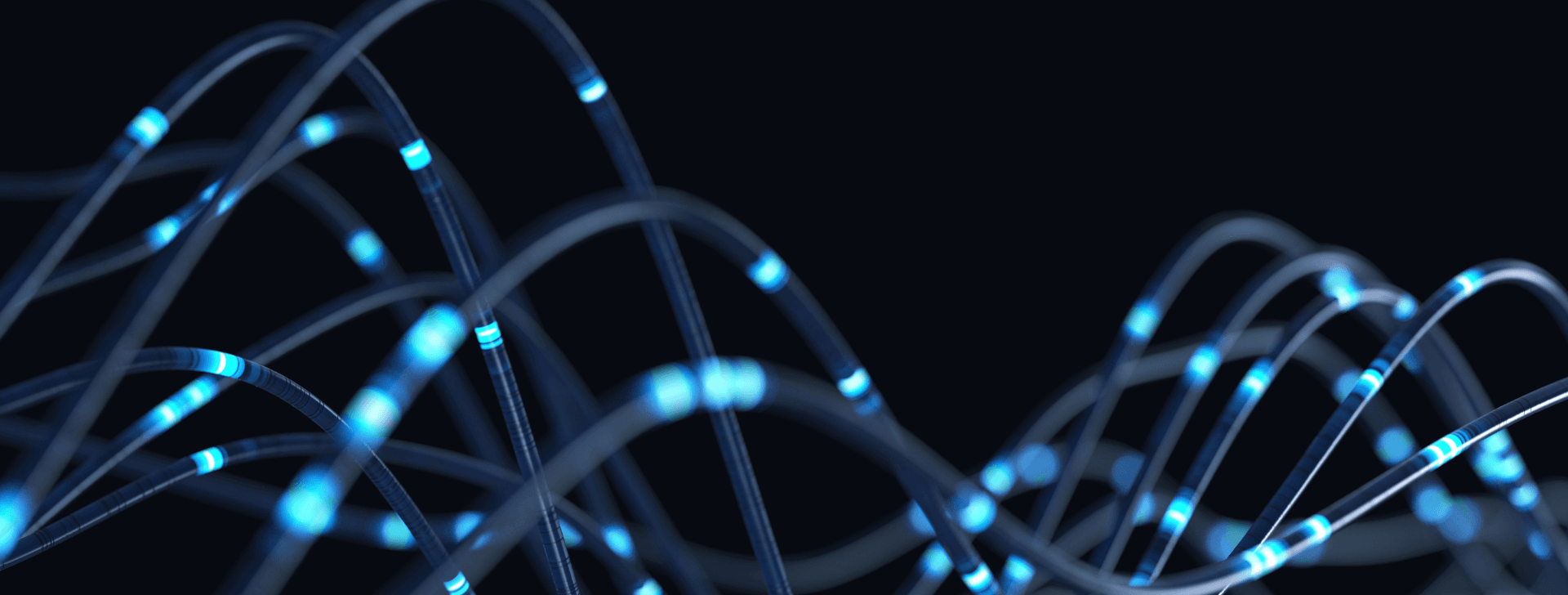
OCT. 9, 2024
Data governance is a cornerstone of modern data strategy, providing the framework for managing data integrity, security, and compliance.
As businesses grow more data-driven, understanding both the benefits of data governance and the challenges it presents is crucial to leveraging data for decision-making and innovation. Let’s explore how effective data governance can transform your business operations while also addressing common obstacles in its implementation.
Key takeaways
- 1. Effective data governance frameworks are essential for improving decision-making, enhancing data quality, and ensuring compliance with regulations.
- 2. Data governance pillars like data quality, metadata management, and data stewardship form the backbone of robust data strategies.
- 3. The biggest challenges in data governance include managing data silos, ensuring regulatory compliance, and overcoming resource constraints.
- 4. Scalability and real-time data processing are increasingly critical as businesses deal with larger volumes of structured and unstructured data.
- 5. Staying ahead in data governance involves adopting AI-driven tools and focusing on continuous improvement and adaptation to new trends.
What is data governance?
Data governance refers to the collection of processes, policies, roles, and standards that ensure effective data management within an organization. It covers the entire lifecycle of data, from acquisition to usage, ensuring that data remains accurate, consistent, secure, and compliant with regulations. The goal is to create a unified approach to handling data that aligns with business objectives and supports better decision-making.
Key components of data governance
The core components of a robust data governance framework typically include:
- Data quality and integrity: Ensuring that data is accurate, complete, and consistent.
- Data security and compliance: Protecting data from breaches and ensuring it adheres to industry regulations.
- Metadata management: Managing data about data to enhance its usability and transparency.
- Data lineage tracking: Understanding the origin, flow, and transformations of data.
- Data cataloging: Organizing data assets for easy access and utilization.
Each of these components plays a vital role in maintaining data governance standards, contributing to a data-driven culture within the organization.
Why data governance is important
Data governance is essential not only for maintaining the quality and security of your data but also for driving strategic business decisions. It empowers organizations to maximize the value of their data assets while ensuring compliance with regulatory requirements. Effective data governance helps businesses create a reliable and accessible data environment, promoting innovation and competitive advantage.
Pillars of data governance
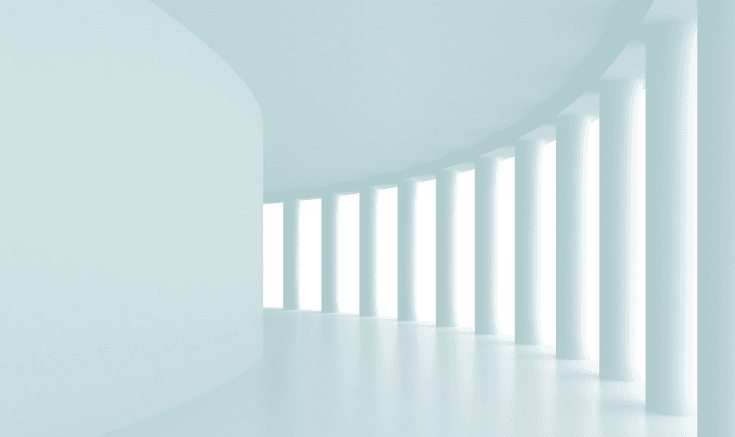
The effectiveness of data governance depends on several fundamental pillars that provide a structured approach to data management. These include:
Data quality
High-quality data forms the backbone of effective decision-making and strategic insight. It's not just about having data; it's about ensuring that data is accurate, complete, and relevant at every stage of its lifecycle. For Lumenalta, maintaining data quality involves robust processes for validation and cleansing, minimizing the risks associated with outdated or incorrect data.
By embedding these practices into the governance framework, organizations can rely on their data to deliver actionable insights, ultimately enhancing both operational efficiency and long-term strategic goals.
Data source management
Managing data sources is critical in creating a unified and cohesive view of organizational data. This process goes beyond simple consolidation; it involves mapping the journey of data from its origin to its application across various systems. Lumenalta emphasizes eliminating redundancies and ensuring that every piece of data is integrated accurately. When data flows seamlessly across an organization, it enables more precise analysis and reporting, breaking down silos and aligning teams toward common objectives.
Metadata management
Metadata plays a pivotal role in making data more accessible and meaningful to users of all skill levels. By organizing metadata effectively, Lumenalta helps businesses create a structured data environment where each piece of information is easily discoverable. This approach ensures that even complex datasets become intuitive, empowering users to harness data without needing extensive technical expertise. Metadata management, therefore, supports quicker decision-making processes by reducing the time spent searching for relevant information.
Data security and compliance
In an era of increasing data regulations and cybersecurity threats, robust data security is not a mere add-on; it's a necessity. Lumenalta’s approach to data security and compliance is about creating a framework that protects sensitive information through well-defined protocols and control mechanisms. This includes setting up role-based access, encryption, and continuous monitoring to stay aligned with evolving regulations like GDPR and CCPA. By prioritizing security and compliance, Lumenalta helps organizations build trust with their stakeholders, safeguarding both data and reputation.
Data stewardship
Data stewardship ensures that data governance policies are not only defined but also consistently applied. The role of data stewards is to bridge the gap between the technical teams and business units, ensuring that data management practices align with organizational goals. These stewards act as the custodians of data integrity, guiding the implementation of data policies and resolving any issues that arise. Their work is crucial in maintaining high standards of data quality and fostering a culture of accountability throughout the organization.
Transparency
Transparency in data governance is about creating a culture where data practices are openly communicated and understood by all stakeholders. When policies, processes, and data usage guidelines are clear, everyone in the organization can confidently engage with the data. Lumenalta promotes a governance model that not only tracks data management performance through visible metrics but also encourages continuous feedback and refinement. This openness builds trust, encouraging responsible data handling and better alignment with business objectives.
Benefits of data governance
Implementing data governance yields several business benefits, enhancing operational efficiency, improving decision-making, and driving competitive advantage. Here are some of the most significant benefits:
1. Improved decision-making and business insights
Data governance ensures that decision-makers have access to reliable and high-quality data, which directly supports better business decisions. Recent research highlights that a virtuous cycle emerges between AI and data governance: AI enhances data quality through automation and monitoring, while high-quality data improves AI model performance. This synergy allows organizations to leverage AI-driven governance for greater scalability and continuous optimization.
According to the Lumenalta whitepaper, 98% of surveyed organizations reported that AI-driven data governance improved their decision-making processes. This transparency and accountability lead to more informed strategic planning and forecasting.
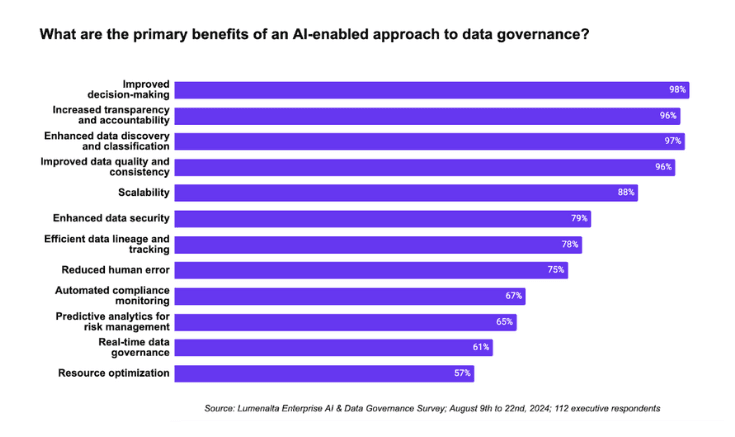
2. Enhanced data quality and consistency
Organizations with well-defined data governance frameworks experience significant improvements in data quality. By standardizing data handling practices, they reduce the risk of errors and discrepancies, leading to more consistent data across all platforms and applications. For example, 96% of companies in the Lumenalta survey saw improvements in data quality after implementing AI-driven governance.
3. Increased data security and compliance
Data breaches and non-compliance can lead to severe financial and reputational damage. Data governance helps to mitigate these risks by establishing clear protocols for data access and usage, ensuring that sensitive information is adequately protected. The whitepaper highlighted that 79% of organizations experienced enhanced data security through automated compliance processes.
4. Greater scalability in data management
As businesses scale and data volumes increase, managing this data can become complex and costly. Effective data governance frameworks streamline data processes, making it easier to handle growing data sets and integrate new data sources without compromising quality or efficiency. A notable 88% of surveyed organizations saw greater scalability after adopting AI-driven governance systems.
5. Operational efficiency and cost reduction
By automating data-related tasks and minimizing manual errors, data governance reduces operational inefficiencies and the costs associated with data management. The whitepaper underscored that 92% of companies track cost savings as a key metric in their data governance programs, with 100% tracking data quality improvements.
AI-driven applications in data governance
AI-powered tools are transforming governance processes by automating tasks that were once manual and time-consuming. Key AI use cases include:
- Automated data documentation: AI tools generate metadata and lineage tracking to improve data accessibility.
- Real-time quality monitoring: Machine learning algorithms identify inconsistencies and errors as data flows through pipelines.
- Automated compliance: Natural language processing (NLP) interprets regulatory requirements and flags compliance risks.
Data discovery with AI: Large Language Models (LLMs) enable natural-language queries, making data more accessible to business users.These AI-driven solutions reduce governance overhead while increasing accuracy, scalability, and compliance.
Challenges in data governance
Despite its benefits, implementing data governance is not without challenges. Organizations often face several obstacles that can hinder the effectiveness of their data governance initiatives.
1. Data quality and integrity issues
Maintaining high data quality remains one of the most significant challenges in data governance. Inaccurate or incomplete data can lead to poor decision-making and reduced business value. The whitepaper revealed that 98% of organizations faced data quality issues when integrating AI/ML into their processes, making it one of the top challenges in data governance today.
2. Data silos and integration complexities
Data silos occur when different departments within an organization use isolated data sets that aren't shared across the organization. This fragmentation can lead to inefficiencies, duplications, and a lack of comprehensive insights. Breaking down these silos to integrate data across various systems is a common challenge in achieving a cohesive data strategy.
3. Regulatory compliance and data security
Ensuring compliance with evolving regulations like GDPR, CCPA, and industry-specific standards is another major challenge. Organizations need to continuously monitor data practices to prevent violations, which can be resource-intensive. Moreover, as data breaches become more sophisticated, maintaining robust data security measures is critical yet challenging.
4. Cultural resistance to data governance
Implementing a data governance framework often requires a cultural shift within the organization. Employees may resist changes to established workflows, especially if they perceive the new processes as cumbersome or if there is a lack of understanding about the importance of data governance.
5. Resource constraints and lack of expertise
Establishing an effective data governance strategy requires skilled professionals and sufficient resources. Many organizations struggle to allocate the necessary budget and personnel, which can hinder their ability to implement and sustain comprehensive data governance practices.
Talent shortages and expertise gaps: 97% note that finding skilled professionals for AI governance remains a major barrier.
Big data governance challenges
With the rise of big data, the complexities of data governance have increased significantly. Managing large volumes of structured and unstructured data poses specific challenges that traditional data governance strategies may not adequately address.
Managing data volume and variety
The sheer volume and variety of big data make it difficult to maintain data quality and ensure consistent governance. Organizations must develop advanced data governance tools and techniques to manage these vast data sets effectively.
Real-time data processing
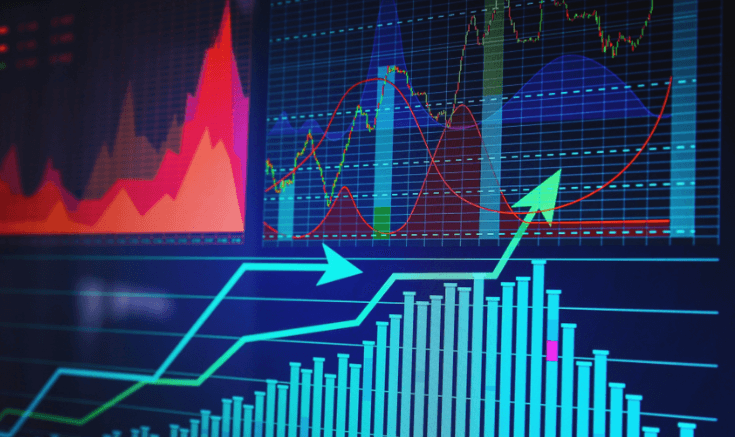
Big data analytics often involves real-time data processing, which requires agile and scalable data governance frameworks. Traditional data governance approaches may not be equipped to handle the speed and flexibility needed for real-time data applications.
Ensuring data privacy and compliance at scale
As big data initiatives grow, so do the challenges related to data privacy and compliance. Organizations must ensure that all data, regardless of its size or source, complies with legal and regulatory standards, which can be difficult to manage on a large scale.
Data governance best practices
To overcome challenges and maximize the benefits of data governance, organizations should consider the following best practices:
- Develop a clear data governance strategy: Outline specific goals, roles, and responsibilities to guide your data governance efforts.
- Promote a data-driven culture: Encourage data literacy and emphasize the value of data across all levels of the organization.
- Invest in technology and automation: Utilize data governance tools to automate routine processes, reduce manual errors, and enhance data quality.
- Regularly review and update governance policies: Adapt to new data sources, technologies, and regulatory requirements to ensure your data governance remains effective and relevant.
Measuring the success of data governance programs
Successful data governance isn’t just about processes and frameworks—it’s about tracking measurable outcomes. According to our research, 100% of organizations monitor data quality metrics, and 92% track cost savings as part of their AI-enabled governance programs. Key metrics include:
- Data quality improvements: Accuracy, completeness, and consistency metrics.
- Cost reductions: Savings from process automation and error reduction.
- Compliance metrics: Reduction in audit violations and improved regulatory adherence.
- Scalability: The ability to manage increasing data volumes without compromising quality.
Forward-thinking organizations prioritize business impact measurements, closing the gap between governance processes and ROI-focused outcomes. Tracking these metrics provides clarity on governance’s real value and enables continuous improvements.
Common mistakes in data governance
Organizations often make several common errors when implementing data governance. These include underestimating the need for data quality, failing to secure executive buy-in, and neglecting to continuously update governance policies as business needs evolve. Addressing these issues early on can significantly improve the success of your data governance initiatives.
Future of data governance
As data landscapes continue to evolve, the future of data governance will be shaped by advancements in AI and machine learning, as well as the increasing emphasis on real-time data analytics. Organizations that stay ahead of these trends will be better positioned to leverage their data assets for strategic decision-making. Learn more about the future of data governance in our dedicated blog, which dives deeper into emerging trends and what they mean for your data strategy.
Turning data governance challenges into opportunities
Data governance is a vital component of any organization's data strategy, offering numerous benefits such as improved decision-making, enhanced data quality, and robust security. While challenges like data silos, compliance issues, and cultural resistance may arise, they can be mitigated with a strategic approach and the right technology investments.
For businesses looking to navigate these complexities effectively, partnering with experts like Lumenalta can provide the guidance and tools necessary to develop a resilient data governance framework that aligns with your digital transformation goals.
"Data governance ensures that decision-makers have access to reliable and high-quality data, which directly supports better business decisions."
Table of contents
- What is data governance?
- Why data governance is important
- Pillars of data governance
- Benefits of data governance
- AI-driven applications in data governance
- Challenges in data governance
- Big data governance challenges
- Data governance best practices
- Common mistakes in data governance
- Future of data governance
- Turning data governance challenges into opportunities
- Common questions about data governance
Common questions about data governance
What are the key benefits of implementing data governance?
What are the main challenges in data governance?
How does data governance impact business decision-making?
What role does AI play in data governance?
What are the best practices for scalable data governance?
How can businesses prepare for the future of data governance?
Want to learn how artificial intelligence can bring more transparency and trust to your operations?