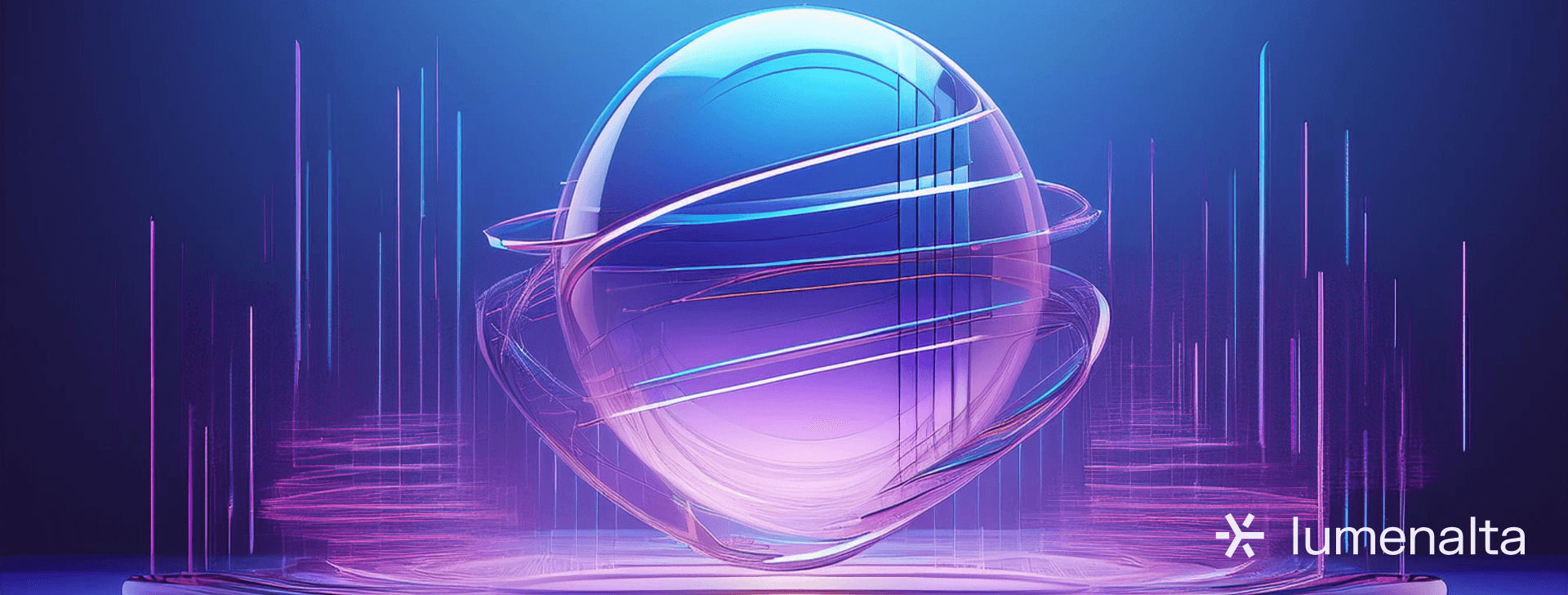
How to build a successful AI strategy
NOV. 27, 2024
6 Min Read
AI is more than a technology—it's a transformative enabler for business innovation and operational efficiency.
Crafting an effective strategy requires aligning your organization's goals with AI capabilities, ensuring that every initiative contributes meaningfully to your overarching objectives.
At its core, an AI strategy involves planning, implementing, and managing AI-driven systems to achieve specific business outcomes. This includes identifying the problems you aim to solve, selecting the right AI tools and technologies, and creating a roadmap for their deployment. It's also essential to recognize that AI solutions must be tailored to your industry, customer base, and operational processes, requiring a deep understanding of both technical and business domains.
Equally important is fostering a culture that embraces data-centric decisions. Organizations must prioritize data accessibility, quality, and security to maximize the impact of AI initiatives. By setting clear expectations, securing stakeholder buy-in, and emphasizing measurable results, you lay the groundwork for a strategy that integrates seamlessly with your existing processes while driving innovation.
Key takeaways
- 1. A successful AI strategy aligns AI capabilities with specific business objectives, enabling measurable and meaningful outcomes.
- 2. Robust data management, including quality and integration, is critical to the reliability and effectiveness of AI-driven initiatives.
- 3. Integrating AI across business operations optimizes efficiency, enhances customer experiences, and drives innovation.
- 4. Ethical considerations, such as transparency and fairness, are essential to responsible AI implementation and stakeholder trust.
- 5. Emerging trends like generative AI, decision intelligence, and edge computing are shaping the future of AI-driven business transformation.
Key components of an effective AI strategy
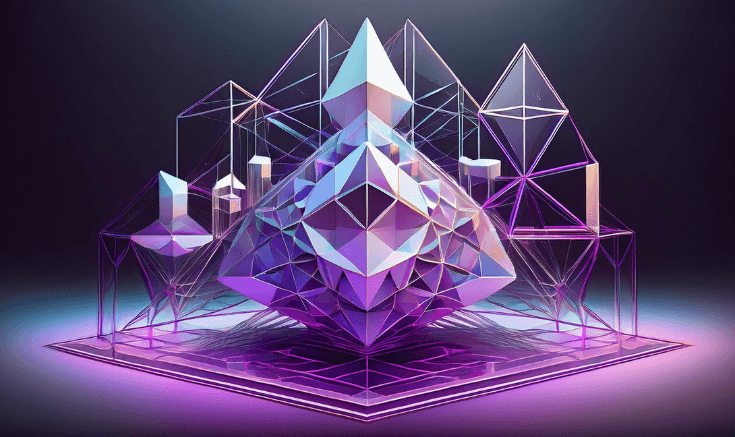
A well-rounded AI strategy incorporates several critical components that ensure it aligns with business objectives, remains adaptable, and delivers measurable value. These elements serve as the backbone for successful AI implementation, addressing both technical and organizational considerations.
Building a robust AI strategy begins with understanding the interplay between technology, people, and processes. Effective strategies focus not only on deploying AI tools but also on integrating them into existing workflows. By doing so, you minimize disruption and maximize the impact of your AI initiatives. Below are the key components that form the foundation of a successful AI strategy:
- Clear business goals: An AI strategy must align with your organization's overall objectives. Whether it's improving customer satisfaction, increasing operational efficiency, or driving revenue growth, AI initiatives should target specific and measurable goals. This ensures that investments yield tangible outcomes and support broader strategic priorities.
- Strong data infrastructure: High-quality, accessible, and well-managed data is the lifeblood of any AI system. Developing robust data pipelines and governance frameworks is essential for ensuring that AI models operate effectively. Focus on scalability, security, and compliance to future-proof your data infrastructure.
- Cross-functional collaboration: AI strategies succeed when diverse teams work together. Bringing business leaders, data scientists, and IT professionals into the conversation ensures that AI initiatives address real-world challenges and leverage technical expertise. Collaboration fosters alignment and accelerates implementation.
- Technological stack and tools: Choosing the right tools and platforms is critical. Consider scalability, ease of integration, and the ability to address specific use cases. Open-source technologies, cloud platforms, and custom-built solutions each have their place depending on your organizational needs.
- Ethical and responsible AI practices: Incorporating ethical guidelines and ensuring compliance with legal standards protects your organization from reputational and regulatory risks. Transparent algorithms, unbiased datasets, and accountability measures build trust with stakeholders.
- Continuous learning and adaptation: AI technologies and business environments evolve quickly. A successful strategy includes mechanisms for regularly reviewing and updating your approach. This adaptability ensures sustained relevance and effectiveness.
These components not only streamline the implementation process but also enhance the overall efficiency and scalability of AI systems. A strategy that incorporates these elements is well-positioned to deliver on its promises, driving meaningful change across the organization.
“Crafting an effective strategy requires aligning your organization's goals with AI capabilities, ensuring that every initiative contributes meaningfully to your overarching objectives.”
Aligning AI with business objectives
To ensure the success of an AI strategy, alignment with business objectives is paramount. AI initiatives are not standalone projects; they must seamlessly integrate with the broader goals of your organization, enhancing both operational and strategic outcomes. This requires a clear understanding of how AI capabilities translate into measurable business impact.
The first step in aligning AI with your objectives is identifying high-impact use cases. Focus on areas where AI can deliver significant value, such as optimizing supply chains, improving customer experience, or enabling predictive analytics for strategic decisions. Prioritizing these use cases ensures that AI efforts are directed toward achieving tangible results.
Equally important is stakeholder engagement. Leaders across departments must collaborate to define the role of AI in addressing their specific needs while contributing to company-wide goals. By involving key decision-makers early, organizations can build a unified vision that drives buy-in and accelerates implementation.
Lastly, aligning AI with business objectives requires a robust framework for tracking progress. Establishing key performance indicators (KPIs) and benchmarks allows you to measure the effectiveness of AI deployments, ensuring they deliver the intended outcomes and remain adaptable to priorities. When implemented thoughtfully, this alignment transforms AI from a technical tool into a strategic asset.
Developing an AI marketing strategy
An AI marketing strategy is essential for leveraging technology to optimize customer engagement, personalize experiences, and drive conversions. By integrating AI into your marketing efforts, you can unlock actionable insights from data, automate repetitive tasks, and improve decision-making processes.
- Personalization at scale: AI enables marketers to deliver highly personalized content and experiences. Using tools like predictive analytics, recommendation engines, and sentiment analysis, you can tailor messaging to individual preferences and behaviors. This enhances customer satisfaction and boosts brand loyalty while increasing conversion rates.
- Streamlining campaign management: AI-powered tools can automate and optimize various aspects of marketing campaigns. From dynamic ad creation to real-time bidding in programmatic advertising, these technologies reduce inefficiencies and maximize ROI. Automating routine tasks allows teams to focus on creative and strategic initiatives, driving greater impact.
- Leveraging data insights: AI excels at analyzing large volumes of customer and market data, uncovering patterns and trends that inform smarter marketing decisions. Tools like natural language processing (NLP) help identify emerging topics, while predictive models guide resource allocation and budget optimization for maximum returns.
These strategies emphasize the transformative role of AI in marketing. A well-developed AI marketing strategy doesn’t just enhance campaigns; it aligns them with business goals, ensuring a cohesive approach to growth and customer satisfaction.
Implementing AI strategy frameworks
Implementing an AI strategy requires a structured framework that ensures clarity, alignment, and scalability. A framework serves as the blueprint for deploying AI initiatives effectively while managing complexities and mitigating risks.
Critical components of an effective AI strategy framework include:
- Assessment and goal setting: Evaluate your organization’s readiness for AI by analyzing your data, technology, and workforce capabilities. Define clear goals that AI initiatives should achieve, ensuring they align with broader organizational priorities.
- Data strategy development: Establish a comprehensive approach to managing and utilizing data. This includes data collection, cleaning, integration, and governance. A strong data strategy ensures your AI models are built on reliable, relevant information.
- Technology and tool selection: Identify the right platforms, tools, and frameworks for your AI initiatives. Consider scalability, compatibility with existing systems, and the ability to support future growth.
- Talent and team alignment: Build cross-functional teams that bring together data scientists, engineers, business analysts, and domain experts. Collaboration between technical and business stakeholders is key to success.
- Deployment and integration: Implement AI solutions iteratively, starting with pilot projects. Gradually scale successful initiatives across the organization, ensuring smooth integration with workflows and systems.
- Performance monitoring and feedback loops: Use KPIs and performance metrics to measure success and identify areas for improvement. Regular reviews allow you to fine-tune models and processes to maximize impact.
An AI strategy framework transforms ambitious ideas into tangible outcomes, minimizing risks and streamlining implementation. It also fosters organizational alignment and collaboration, ensuring AI deployments are both innovative and practical. As AI continues to redefine industries, a robust framework positions your organization to adapt.
Data management in AI strategy
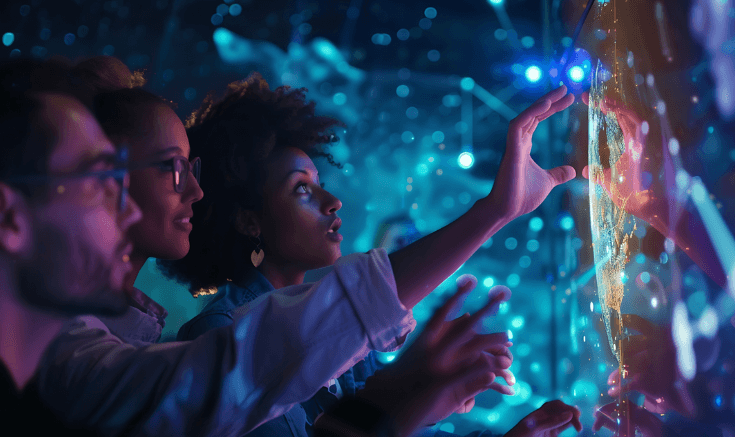
Effective data management lies at the heart of any successful AI strategy. Data is the fuel that powers AI models, and its quality, accessibility, and structure directly impact the performance and reliability of AI-driven initiatives.
The foundation of data management begins with robust data governance. This involves establishing clear policies for data collection, storage, and usage. Strong governance ensures that your data complies with regulatory standards and maintains consistency across systems, reducing the risk of inaccuracies or biases that could compromise AI outcomes.
Another critical aspect is data integration. Organizations often deal with fragmented data sources stored across disparate systems. Consolidating these into a unified, scalable infrastructure facilitates smoother AI implementation and allows for real-time insights. Whether through data lakes, warehouses, or hybrid solutions, the goal is to create a seamless flow of information.
Equally important is the preparation and curation of data. Cleaning and annotating datasets, removing duplicates, and ensuring relevance are vital steps in building reliable AI models. With these efforts, your data becomes more actionable, enabling AI systems to generate accurate predictions and recommendations.
A comprehensive data management strategy not only supports the immediate needs of AI projects but also future-proofs your organization. By prioritizing data quality and accessibility, you help your teams to innovate and scale AI initiatives with confidence, driving sustained business value.
AI integration across business operations
Integrating AI across business operations transforms organizational efficiency by embedding intelligence into workflows and processes. AI becomes a strategic enabler when seamlessly incorporated into core functions such as supply chain management, customer service, marketing, and product development.
- Enhancing operational efficiency: AI-driven automation streamlines repetitive tasks, freeing employees to focus on higher-value work. For example, AI-powered systems can manage inventory, optimize logistics, and predict demand, significantly reducing costs and improving resource allocation. By embedding AI into these operations, organizations gain both speed and accuracy, ensuring more agility.
- Improving customer interactions: Customer-facing operations benefit immensely from AI integration. Chatbots and virtual assistants provide real-time support, while sentiment analysis tools help organizations understand and respond to customer needs more effectively. By personalizing interactions and resolving issues faster, AI-powered customer service enhances satisfaction and builds stronger brand loyalty.
- Driving innovation in product development: AI’s ability to analyze large datasets and identify patterns accelerates the innovation process. In product development, AI tools can forecast trends, suggest design improvements, and simulate performance outcomes, enabling organizations to create more market-relevant offerings. This integration ensures that innovation is aligned with customer expectations.
Seamlessly integrating AI across business operations requires a clear strategy, robust infrastructure, and cross-functional collaboration. When done effectively, it not only improves efficiency but also drives innovation, elevates customer experiences, and solidifies a competitive edge.
“Seamlessly integrating AI across business operations requires a clear strategy, robust infrastructure, and cross-functional collaboration.”
Overcoming challenges in AI implementation
Implementing AI comes with its own set of challenges, ranging from technical hurdles to organizational resistance. Recognizing and addressing these obstacles early can pave the way for smoother adoption and more impactful outcomes.
Common challenges and strategies to overcome them:
- Data quality and availability: Poor data or fragmented sources can compromise AI performance. Robust data governance, cleansing practices, and integration are essential to ensure reliable insights.
- Integration with legacy systems: Outdated systems often lack compatibility with modern AI tools. Gradual modernization and flexible APIs help bridge the gap without overhauling existing infrastructure.
- Lack of skilled talent: Limited expertise in AI technologies can delay innovation. Upskilling existing teams and hiring specialized professionals address this gap effectively.
- Resistance to change: Employees may resist AI adoption due to concerns about job displacement or skepticism. Transparent communication and stakeholder involvement foster trust and collaboration.
- Ethical concerns and bias: Flawed datasets or opaque algorithms can lead to biased outcomes. Ethical guidelines and regular audits ensure fairness and accountability.
- Cost and resource constraints: High costs can deter organizations from scaling AI projects. Starting with pilot initiatives demonstrates value and secures future investments.
Successfully overcoming these obstacles requires a balanced approach that integrates technical readiness, cultural transformation, and ethical practices. By addressing these challenges head-on, organizations position themselves to utilize the transformative power of AI with confidence and clarity.
Ethical considerations and responsible AI use
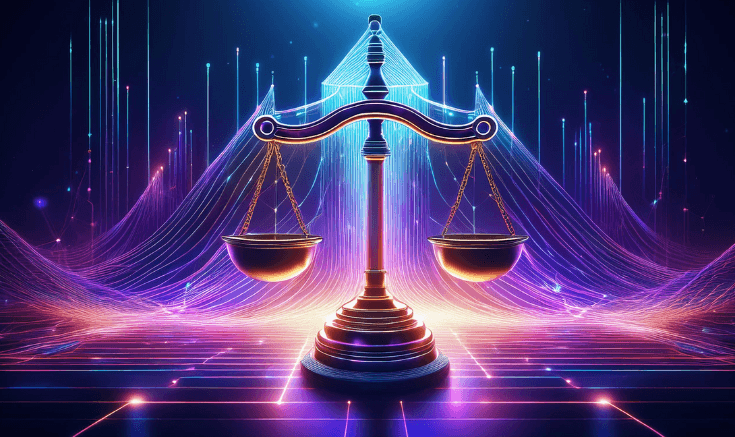
As AI becomes an integral part of business strategies, addressing ethical considerations is not optional—it’s a necessity. Responsible AI use safeguards against unintended consequences, fosters trust, and ensures compliance with regulatory standards. Organizations must prioritize ethical principles throughout the AI lifecycle, from development to deployment.
- Transparency: Decision-making processes in AI systems should be understandable and explainable to users and stakeholders. Ensuring transparency helps organizations build trust while making it easier to identify and address potential biases or errors in AI models.
- Accountability: Businesses must establish clear governance frameworks to oversee AI use, assigning responsibility for outcomes to specific teams or individuals. This reduces risks associated with ethical lapses and enables swift corrective actions when necessary.
- Diversity and inclusivity: AI systems trained on homogeneous datasets can perpetuate biases, leading to unfair outcomes. By incorporating diverse perspectives and datasets, organizations can create AI solutions that reflect the broader needs of their customers and society.
Ethical AI isn’t just about avoiding harm—it’s about building systems that actively contribute to equitable, fair, and sustainable outcomes. A commitment to these principles ensures that AI serves as a force for good, enhancing business operations while respecting societal values.
Measuring AI strategy success
Measuring the success of an AI strategy is essential to ensure it delivers on its promises and aligns with business objectives. Clear evaluation metrics provide insights into the effectiveness of AI initiatives, highlight areas for improvement, and justify continued investment.
- Defining key performance indicators (KPIs): The first step in measuring AI success is identifying relevant KPIs. These metrics should align with the specific goals of your AI initiatives, whether they aim to improve operational efficiency, increase revenue, or enhance customer satisfaction. For example, metrics like process automation rates, customer retention, or prediction accuracy can indicate how well AI is meeting expectations.
- Assessing ROI and business impact: Return on investment (ROI) is a fundamental measure of success. Beyond direct cost savings, ROI includes intangible benefits like improved decisions and industry advantages. By quantifying these outcomes, businesses can demonstrate the tangible value AI brings to their operations.
- Monitoring performance over time: AI systems require continuous monitoring to ensure they remain effective and adapt to changing conditions. Tracking metrics such as model accuracy, system uptime, and user adoption rates provides a comprehensive view of AI performance. Regular evaluations allow organizations to refine strategies and maintain alignment with business needs.
By leveraging these measurement practices, organizations can ensure their AI strategy delivers meaningful outcomes. Monitoring success not only validates the value of current initiatives but also informs future investments, enabling a cycle of continuous improvement and innovation.
“By focusing on these emerging trends and integrating them into their AI strategies, businesses can stay ahead of the curve, ensuring sustained growth and a competitive advantage in an AI-driven world.”
Future trends in AI for business
The future of AI in business is defined by transformative technologies that are reshaping industries and driving innovation. Staying ahead of these trends ensures that organizations remain innovative.
- Generative AI: This technology is enabling businesses to produce sophisticated content and simulations, from personalized marketing campaigns to product design prototypes. By enhancing creativity and streamlining operations, generative AI offers significant time and cost savings.
- Decision intelligence: Combining real-time data processing, predictive analytics, and machine learning, decision intelligence improves decisions by delivering actionable insights. This capability is particularly impactful in dynamic environments where agility and accuracy are critical.
- Edge AI: By processing data closer to its source, edge AI reduces latency and enhances security. This trend is particularly relevant in sectors like logistics, healthcare, and manufacturing, where making decisions in real-time is crucial. Businesses adopting edge computing gain operational agility while meeting the demand for faster, more secure processes.
By focusing on these emerging trends and integrating them into their AI strategies, businesses can ensure sustained growth.
AI is more than just a technological innovation—it’s a strategic lever for driving transformation, efficiency, and growth. By embedding AI into your operations, you unlock unparalleled potential for innovation. At Lumenalta, we specialize in building tailored AI solutions that align with your unique business goals. Discover how our expertise can empower your organization to lead with intelligence and precision. Let’s build a brighter path forward, together.
Table of contents
- Key components of an effective AI strategy
- Aligning AI with business objectives
- Developing an AI marketing strategy
- Implementing AI strategy frameworks
- Data management in AI strategy
- AI integration across business operations
- Overcoming challenges in AI implementation
- Ethical considerations and responsible AI use
- Measuring AI strategy success
- Future trends in AI for business
- Common questions about AI strategy
Common questions about AI strategy
What is an AI strategy, and why is it important?
How can AI be used to enhance business decision-making?
What role does data play in developing an AI strategy?
What industries benefit most from AI strategies?
How do ethical considerations impact AI implementation?
Want to learn how AI strategies can bring more transparency and trust to your operations?