How fraud analytics help banks fight against financial crime
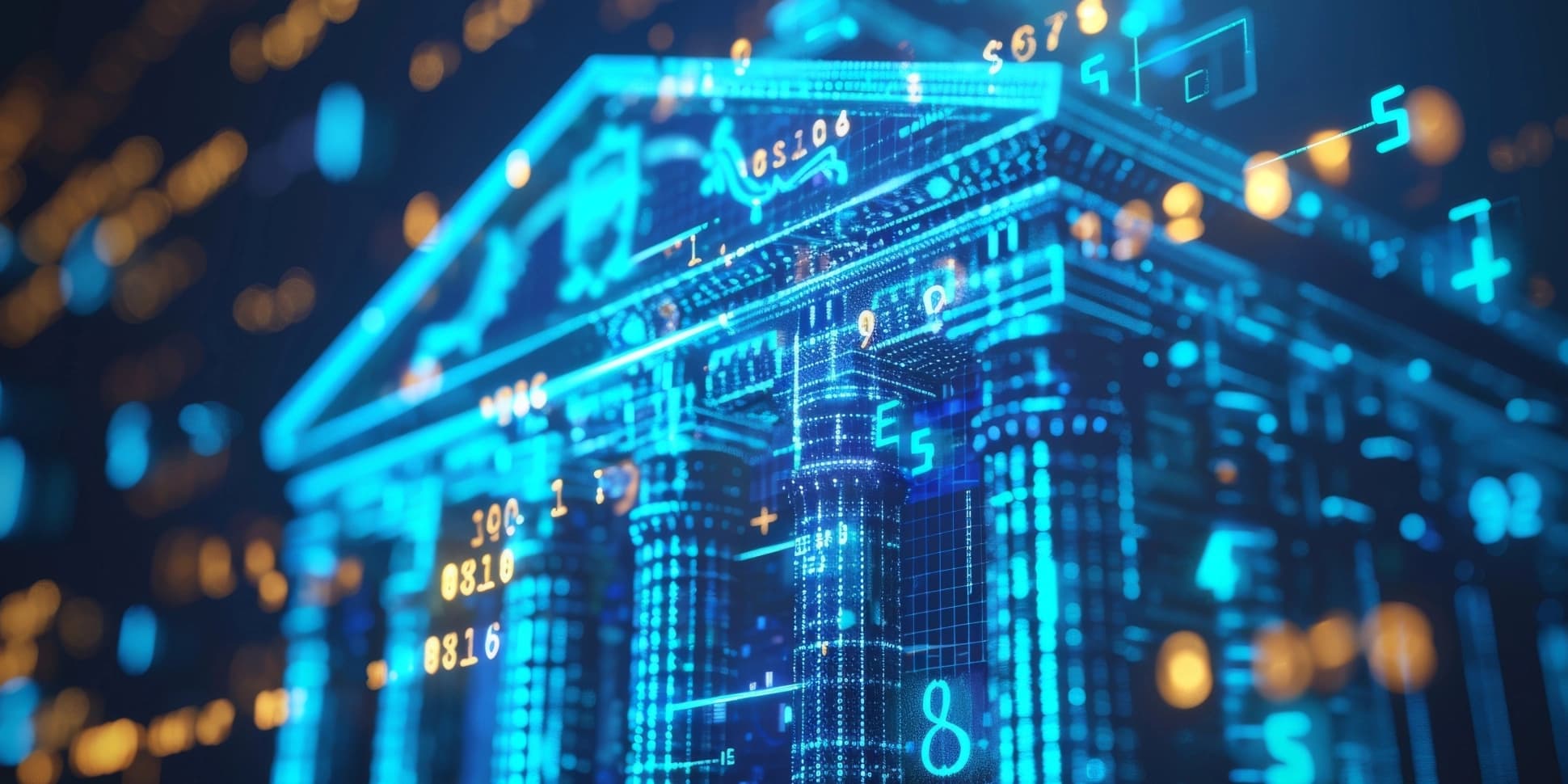
MAY. 22, 2024
Banks are turning to AI-powered fraud analytics solutions to combat fraud at scale.
Banks and fraudsters have been locked in a tug-of-war since the dawn of banking itself. As banks fortify their defenses, hackers get more sophisticated in their attacks.
Unfortunately, banks are losing ground. A recent report from Nasdaq found that “the scale and pervasiveness of financial crime is immense…fraud losses totaled $485.6 billion [in 2023] from a range of devastating fraud scams and bank fraud schemes, worldwide.”
To combat this surge, banks are turning to AI-powered fraud analytics solutions. These tools proactively identify anomalies and high-risk areas, giving banks the upper hand in the ever-evolving fight against financial crime.
The complex challenge of banking fraud
Fraud and data security breaches in banking are particularly insidious. Financial institutions have a mountain of data and transactions to keep track of, and spotting fraud can feel like finding a needle in a haystack.
What’s worse, these crimes tend to lack consistent patterns, making them even harder to detect. Whether it’s identity theft, cyberattacks, or money laundering, each scheme leaves well-camouflaged footprints disguised as legitimate transactions.
Think about a casino. They have entire teams solely dedicated to spotting unusual betting patterns and can typically root out cheating fairly quickly. But for banks, it’s a whole different ball game. You can have tons of tiny transactions that add up to a large money laundering operation, which is incredibly difficult to spot.
Humans alone are no match for modern fraud schemes — the sheer number of transactions is too much to handle. Plus, bank staff can add more value by spending their time on core business activities rather than sifting through thousands (or millions) of transactions.
Instead, banks are turning to AI-powered fraud analytics solutions to combat fraud at scale.
What is fraud analytics in banking?
Put simply, fraud analytics is the use of advanced data analysis tools and techniques to detect and prevent fraudulent activities within financial institutions. It leverages both historical and real-time data to identify, assess, and mitigate risks associated with fraud and data breaches.
Specific use cases for fraud analytics in banking
While each fraud scheme is unique, there are a few types that present the greatest threat to banks and their customers.
Here are some of the most common use cases for combating financial crimes via fraud analytics:
Account takeover prevention
In an account takeover (ATO) attack, a malicious actor gains unauthorized access to a user’s account, taking control to commit fraud, steal data, or engage in other harmful activities. It’s one of the most common types of fraud, with nearly one-third of people being victims of this attack.
By the time a bank registers that an ATO attack has taken place, it’s usually too late. Some funds may be recouped, but the bulk of the damage is already done.
That’s why proactive measures, such as AI-powered fraud analytics, are so valuable. They can detect suspicious login attempts, unusual activity patterns, and potential identity theft and stop an attack before it leads to significant financial and personal data losses.
Anomaly detection in wire transfers
Wire transfers are a major risk for money laundering and other illicit activities. Fraud analytics tools can flag large or unusual wire transfers for closer scrutiny.
By examining the context and patterns of these transactions, banks can uncover and prevent illegal activities, ensuring adherence to global anti-money laundering (AML) standards and other banking compliance regulations.
Payment fraud detection
Fraud analytics is indispensable in rapidly growing areas like online banking and e-commerce, where physical verification of cardholders is impossible.
These tools can identify potentially fraudulent transactions in real time, allowing banks to intervene before the transaction is completed.
Tailor-made solutions are ideal
These are just a few of the most common types of financial fraud — there are dozens more.
Since no two schemes are exactly alike, the usefulness of out-of-the-box tools is limited. To effectively combat these ever-evolving threats, banks need a custom-built defense strategy.
Here’s a breakdown of the steps required for building a robust financial fraud analytics program.
How to develop a banking fraud analytics program
1. Conduct a comprehensive data risk assessment
The first step is a thorough financial data analytics risk assessment. This isn’t a one-time exercise — it’s an ongoing process of continuously gathering and analyzing data to pinpoint areas that are more susceptible to fraud.
This step entails:
Data gathering and management
Banks first need to establish a system for collecting and storing vast amounts of data. This includes customer transactions, account details, device usage patterns, and historical fraud incidents.
This data needs to be meticulously organized and readily accessible for ongoing analysis.
Threat identification and prioritization
With the data consolidated and cleaned, banks can leverage AI’s predictive capabilities to identify potential security gaps and vulnerabilities.
For instance, a bank with a large online presence might prioritize identifying vulnerabilities related to ATO or online payment fraud. The identified vulnerabilities are then assessed for their potential impact on the bank, considering factors like the size of potential losses and the complexity of exploiting the weakness.
Rather than trying to boil the ocean, this prioritization allows banks to focus their resources on the areas that pose the greatest risk.
2. Develop an ongoing approach to fraud detection
Once vulnerabilities are identified, banks can shift their focus to detecting suspicious activities. Here are some of the techniques used at this stage:
Statistical analysis
Quantitative data models can identify anomalies in transaction patterns that may suggest fraudulent activities. For example, a sudden increase in high-value transactions in a short period could trigger a fraud alert.
Learning models
Machine learning (ML) algorithms learn from historical data to predict future behavior. This learning comes in two forms: supervised and unsupervised.
Related: How to avoid SaaS data fragmentation
Supervised learning models
Supervised learning models are trained on labeled datasets to recognize fraudulent transactions. This method tends to be more reliable but also requires significant human labeling effort.
Unsupervised learning models
Unsupervised learning, on the other hand, excels at spotting anomalies — patterns that deviate significantly from established behavior. While it minimizes human intervention, it can occasionally misinterpret normal fluctuations as suspicious activity.
Risk scoring and investigation
Once activities are flagged by the model, they’re assigned a risk score based on the severity of the anomaly. High-risk activities are then prioritized and forwarded to fraud analysts for a deeper investigation.
This investigation may involve contacting customers for verification, blocking suspicious transactions, or even involving law enforcement agencies.
Deep domain expertise
In most cases, banks can’t conduct a comprehensive risk assessment alone. Enlisting the help of a partner with deep domain expertise can help banks ensure their programs incorporate the latest and greatest fraud detection techniques.
Achieve best-in-class security with Lumenalta
AI-powered fraud analytics have given banks a potent tool for combating financial crime. But implementation can be complicated — the sheer volume of data, combined with constantly evolving fraud prevention techniques, means these systems need constant fine-tuning to work their best.
You need a partner to help you keep track of it all. That’s where Lumenalta comes in. Our team brings a wealth of software experience in financial services and can help you stay protected through a custom-built defense strategy.
You owe it to your stakeholders to keep your data and customer funds as secure as possible. Schedule a consultation today and see how Lumenalta can help you improve your security posture.
Read next: The place of AI in open banking solutions