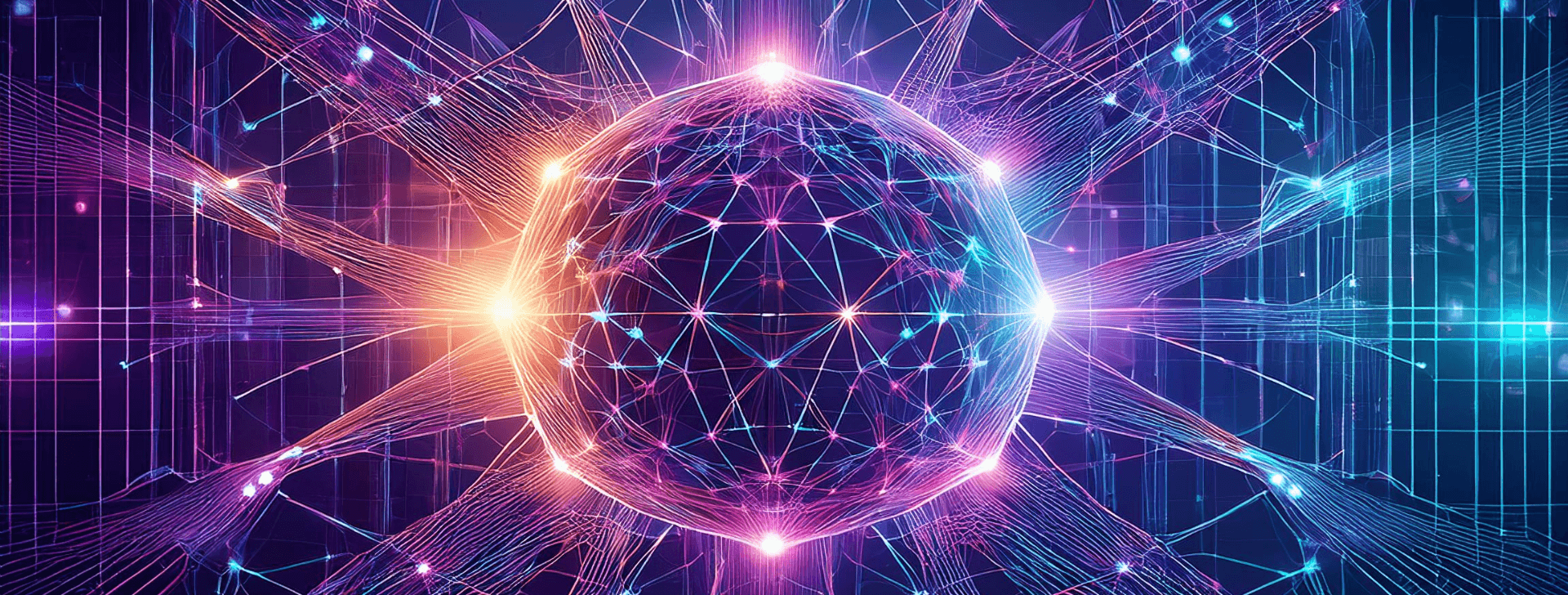
15 differences between artificial intelligence vs machine learning
DEC. 7, 2024
6 Min Read
Artificial intelligence and machine learning are redefining how businesses solve problems, optimize operations, and create long-term value.
These technologies are not just tools; they are transformative forces that enhance decisions, drive innovation, and deliver measurable impact. Organizations adopting these technologies strategically can unlock untapped potential, achieve operational excellence, and secure an innovative advantage in their industry.
Key takeaways
- 1. Artificial intelligence mimics human intelligence, enabling businesses to tackle complex, multi-dimensional challenges effectively.
- 2. Machine learning excels at identifying data patterns and providing precise, data-driven insights for operational improvements.
- 3. Combining artificial intelligence and machine learning enhances scalability, cost-efficiency, and measurable impact across industries.
- 4. Tailored implementation strategies ensure alignment with business objectives, delivering faster returns and long-term adaptability.
- 5. Businesses investing in these technologies future-proof their operations while unlocking untapped growth opportunities and improving overall efficiency.
Understanding AI and machine learning
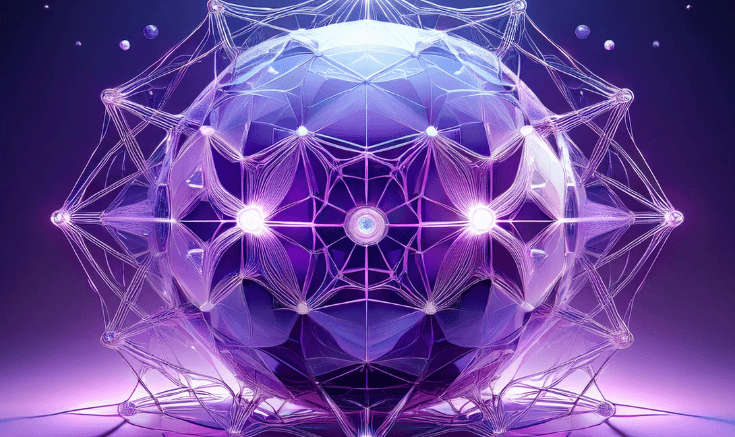
Artificial intelligence and machine learning have transformed how organizations approach problem-solving and innovation. Artificial intelligence refers to the simulation of human intelligence in machines that can perform tasks like reasoning, learning, and evaluation. It serves as an umbrella term for technologies that allow automation and intelligent interactions. Machine learning, a subset of artificial intelligence, focuses on developing algorithms that allow computers to learn from and make predictions based on data.
AI systems aim to replicate and extend human capabilities, from natural language processing to robotics. Machine learning emphasizes creating models that grow through data exposure, often outperforming traditional programming approaches. These technologies power solutions ranging from predictive analytics to conversational interfaces, helping businesses enhance productivity and deliver more personalized customer experiences.
Organizations across industries leverage AI and machine learning to automate repetitive processes, reduce errors, and gain actionable insights from vast datasets. As these technologies develop, they are shaping the future of innovation by empowering businesses to solve complex challenges, optimize operations, and find new opportunities for growth.
"Artificial intelligence refers to systems designed to mimic human intelligence, such as reasoning, learning, and adapting."
Business impact of machine learning vs artificial intelligence
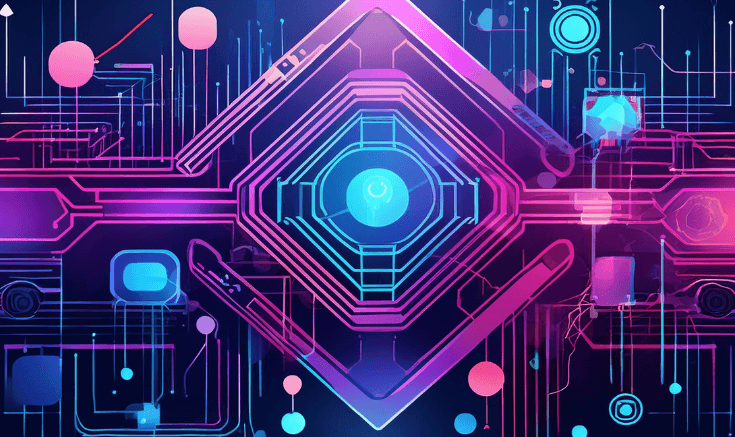
The distinction between machine learning and artificial intelligence has a profound impact on how businesses strategize and deploy technology solutions. Artificial intelligence focuses on mimicking human cognitive abilities to solve complex problems, often involving strategic reasoning, pattern recognition, or decision processes. Machine learning, on the other hand, is data-centric, allowing systems to improve and adapt based on patterns within the data they analyze.
AI offers businesses the ability to address broad challenges, such as developing conversational interfaces, streamlining operations, or creating autonomous systems. Its ability to simulate human-like reasoning and interaction makes it invaluable for industries requiring quick responses, such as healthcare diagnostics or financial fraud detection. Machine learning delivers specific, scalable, and predictive insights by identifying trends or anomalies that human analysts might overlook. Its applications span from real-time customer personalization to inventory forecasting in retail.
AI and machine learning modernize businesses by accelerating time to value. AI-led automation reduces operational inefficiencies, while machine learning permits data strategies that reveal untapped growth opportunities. Both technologies improve cost-effectiveness and scalability, offering a clear pathway to measurable business impact and improved return on investment (ROI).
15 key differences between artificial intelligence vs machine learning
The main differences between artificial intelligence and machine learning highlight their distinct capabilities, approaches, and applications. Artificial intelligence seeks to replicate human cognition, solving broader challenges by simulating perception, learning, and reasoning. Machine learning, as a subset of artificial intelligence, uses algorithms to analyze data, make predictions, and improve based on experience. Understanding these distinctions allows businesses to optimize strategies, reduce costs, and capitalize on untapped opportunities, ensuring scalable, sustainable operations.
Each difference outlined below offers a unique perspective on how these technologies deliver measurable business outcomes. While artificial intelligence permits transformative innovation and strategic insights, machine learning provides precision and efficiency, addressing operational needs. Businesses can make informed decisions on implementation, balancing immediate benefits with long-term returns.
1. Scope and definition
Artificial intelligence represents a comprehensive framework encompassing cognitive functions like problem-solving, reasoning, and regulation. Its applications include autonomous systems, robotics, and virtual agents. Machine learning focuses on a narrower scope, emphasizing algorithmic learning from data to make predictions or classifications. Businesses looking to address specific, data-centric tasks often benefit from machine learning's focused approach, while artificial intelligence tackles broader, multifaceted challenges.
Adopting artificial intelligence offers strategic advantages by authorizing systems to function autonomously across many scenarios. Machine learning adds operational efficiency by streamlining tasks like fraud detection or customer segmentation. Each approach aligns with different organizational needs, enhancing the ability to innovate and compete.
2. Problem-solving approach
Artificial intelligence operates by mimicking human problem-solving, integrating various methodologies such as neural networks, rule-based systems, and natural language processing. It often addresses multi-dimensional challenges requiring contextual understanding. Machine learning solves specific problems by identifying patterns and building predictive models. These models continuously adapt, delivering scalable solutions for repetitive or analytical tasks.
This difference allows organizations to choose tailored strategies. Artificial intelligence can guide long-term planning or strategic transformation, while machine learning supports day-to-day operations by automating data analysis and prediction. Both approaches amplify the organization's capacity to overcome challenges with precision and agility.
3. Speed to market
Artificial intelligence implementations, due to their complexity, typically require longer development cycles involving multiple layers of customization. Machine learning solutions, often built on pre-existing frameworks or libraries, accelerate time to deployment, ensuring businesses can address needs. This speed is crucial for companies seeking immediate returns.
Organizations prioritizing speed to value benefit from machine learning’s modular nature, which supports faster prototyping and scaling. While artificial intelligence delivers transformative outcomes over time, machine learning ensures a more immediate impact, reducing lead times and operational delays.
4. Cost-effectiveness and scalability
Artificial intelligence requires significant upfront investment, including advanced infrastructure and multidisciplinary expertise. Machine learning, with its data-focused approach, often proves more cost-effective, particularly for businesses with constrained resources. Scalable solutions built on machine learning allow organizations to grow and adapt without incurring prohibitive costs.
For businesses, balancing initial expenses with long-term gains is critical. Machine learning offers a cost-efficient entry point, with its ability to process data quickly and scale on demand. Artificial intelligence drives strategic growth by automating complex processes, justifying higher upfront investments for businesses with broader goals.
5. Data requirements
Artificial intelligence can function with minimal data in scenarios where rule-based or expert systems dominate. Machine learning, however, relies heavily on large datasets for training and improving accuracy. Data quality and quantity directly influence machine learning models' effectiveness, making robust data pipelines essential.
Organizations with established data ecosystems often see faster results from machine learning. For businesses with limited data access, artificial intelligence solutions using structured knowledge bases may provide an alternative pathway. Investing in comprehensive data strategies ensures technology adoption aligns with operational realities.
6. Decision-making capabilities
Artificial intelligence allows machines to replicate human decisions by synthesizing data, contextual knowledge, and predefined rules. Machine learning focuses on generating predictions and insights through pattern recognition. While artificial intelligence extends human reasoning into complex domains, machine learning enhances precision in operational contexts.
Businesses using artificial intelligence often gain strategic clarity in unstructured, high-stakes scenarios. Machine learning empowers organizations with actionable insights, implementing real-time optimizations that improve performance. They address both strategic and tactical needs.
7. Adaptability to new scenarios
Artificial intelligence systems, particularly those equipped with advanced algorithms, adapt to scenarios by interpreting diverse inputs. Machine learning requires retraining or fine-tuning when introduced to new conditions or datasets, limiting its flexibility. Businesses seeking long-term adaptability may favor artificial intelligence for its dynamic capabilities.
Machine learning excels in stable settings where patterns remain consistent. Artificial intelligence offers greater resilience for industries experiencing frequent changes, ensuring systems progress with emerging requirements—the ability to adapt positions artificial intelligence as a foundation for lasting strategies.
8. Business potential identification
Artificial intelligence provides a broad lens for identifying untapped opportunities across industries, integrating multiple data sources, and interpreting trends. Machine learning focuses on immediate operational potential, discovering actionable insights within defined parameters. Both approaches empower organizations to discover new growth areas.
AI-backed systems are ideal for long-term strategic planning, allowing companies to forecast market trends and anticipate shifts. Machine learning supports immediate optimization by revealing performance gaps and inefficiencies. They maximize the ability to unlock hidden business potential.
9. Implementation complexity
Artificial intelligence systems involve complex architecture and integration across multiple functions, requiring expertise and resources. Machine learning’s modular nature simplifies implementation, particularly for specific use cases like fraud detection or recommendation engines. This difference impacts both timeframes and budgets for organizations adopting these technologies.
Machine learning’s accessibility lets businesses experiment and deploy quickly, even with limited resources. Artificial intelligence delivers more comprehensive solutions for broader digital transformations, albeit with greater technical requirments. Choosing the right implementation strategy aligns technology investments with operational goals.
10. Return on investment (ROI)
Artificial intelligence delivers strategic ROI by modifying workflows, automating processes, and allowing innovation. Machine learning achieves operational ROI by addressing immediate challenges with data precision. Both approaches align with business objectives, whether optimizing efficiency or driving growth.
Organizations can measure ROI through tangible outcomes, such as cost reductions, productivity gains, and revenue growth. Artificial intelligence provides cumulative benefits over time, while machine learning ensures quicker returns through its targeted problem-solving approach. This dual impact enhances overall value creation.
11. Future-proofing capabilities
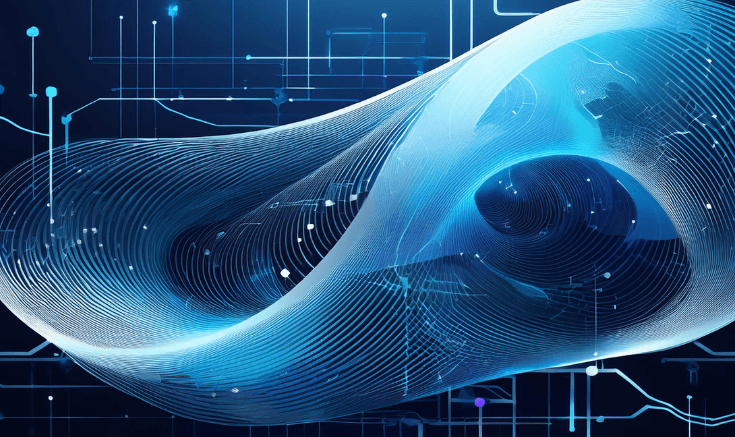
Artificial intelligence is designed to handle use cases, integrating advancements in technology seamlessly. Machine learning addresses present needs but requires ongoing adjustments to remain relevant. Organizations looking to sustaintheir operations benefit from artificial intelligence’s versatility.
Integrating artificial intelligence ensures businesses stay proactive amid technological disruptions. Machine learning complements this by addressing specific challenges, maintaining operational stability. These technologies create robust systems capable of navigating future complexities with confidence.
12. Change management requirements
Adopting artificial intelligence often requires cultural and operational shifts, reshaping workflows and decision processes. Machine learning’s impact is less disruptive, integrating more smoothly into existing systems. Effective change management ensures both technologies deliver their intended value.
Stakeholder alignment is essential when implementing artificial intelligence, particularly in industries with complex regulations or workflows. Machine learning offers a more incremental approach, permitting gradual adoption. This balance supports seamless transitions and sustained operational improvements.
13. Governance and ethical considerations
Artificial intelligence raises broader concerns, such as ensuring unbiased decisions and maintaining accountability. Machine learning emphasizes data governance, focusing on the integrity and reliability of inputs. Addressing these considerations is essential for building trust and compliance.
Organizations implementing artificial intelligence must prioritize transparency and ethical design, particularly in sensitive applications. Machine learning requires robust data policies to avoid biases and errors. Proactive governance ensures both technologies align with organizational values and regulatory standards.
14. Measurable business impact
Artificial intelligence drives impact by automating processes and delivering strategic insights. Machine learning creates measurable outcomes by improving operational efficiency and generating actionable insights. These technologies amplify an organization’s ability to achieve its objectives.
Quantifying impact helps organizations demonstrate value to stakeholders, whether through increased revenue, cost savings, or improved customer satisfaction. Artificial intelligence allows long-term innovation, while machine learning enhances day-to-day performance, delivering tangible results.
15. Human involvement
Artificial intelligence seeks to minimize human intervention in repetitive or complex tasks, enhancing efficiency. Machine learning requires active participation for training, validation, and tuning, ensuring model accuracy. Each approach balances automation with human oversight.
Organizations benefit from artificial intelligence’s ability to reduce cognitive workload, freeing employees to focus on strategic priorities. Machine learning empowers teams with tools for informed decisions, creating synergy between human expertise and technological capabilities.
Understanding these distinctions helps businesses tailor technology investments to their needs, delivering measurable outcomes and strategic advantage.
"Machine learning offers quicker ROI with solutions designed to solve specific problems efficiently and effectively."
How AI and machine learning work together to maximize ROI
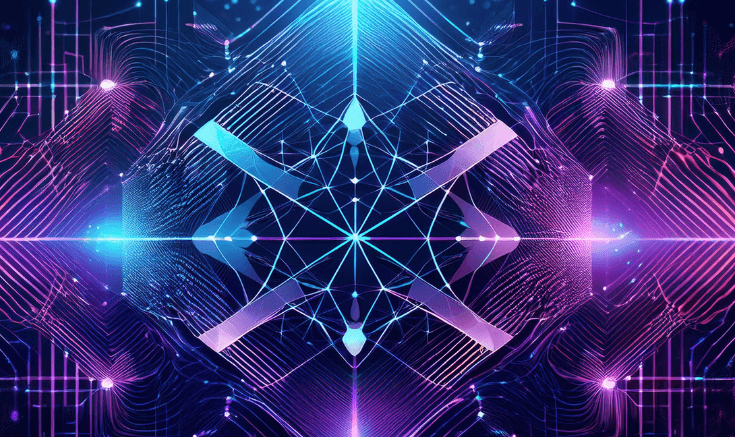
Artificial intelligence and machine learning, when combined, create a synergistic effect that enhances business operations and accelerates returns on investment. Artificial intelligence sets the strategic foundation by simulating human-like reasoning and evaluation across a range of applications. Machine learning strengthens this framework by implementing systems to learn from data, improve performance, and adapt to market conditions. These technologies optimize workflows, showing untapped business potential, and deliver measurable impact across industries.
AI systems leverage machine learning models to refine predictions, automate repetitive tasks, and streamline processes. For instance, predictive maintenance in manufacturing relies on machine learning algorithms to identify patterns in equipment data, while artificial intelligence ensures that the system interprets and applies insights to prevent failures. This collaboration not only reduces downtime but also cuts operational costs, improving scalability and profitability.
Businesses benefit from this combination by addressing both immediate operational challenges and long-term strategic objectives. Machine learning’s adaptability complements AI’s ability to guide overarching decisions, ensuring solutions remain relevant and reliable. From improving customer experiences to optimizing supply chains, integrating these technologies drives sustainable growth and maximizes returns for stakeholders.
Applications of artificial intelligence vs machine learning
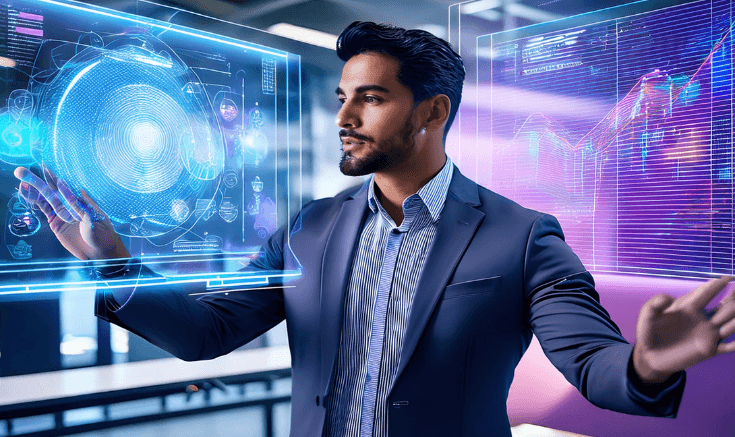
Identifying the right applications for artificial intelligence and machine learning is crucial for businesses aiming to optimize operations and unlock their full potential. Artificial intelligence excels in complex, multi-dimensional tasks that require reasoning, learning, and making decisions. Machine learning offers precision and efficiency for data insights and real-time optimizations. These technologies create opportunities to address challenges, improve scalability, and maximize returns for businesses across industries.
- Healthcare: Artificial intelligence assists with diagnosing complex conditions using medical imaging, such as detecting tumors or anomalies in radiology scans. Machine learning enhances patient care by predicting disease risks through patterns in medical history, and offering targeted treatment recommendations that improve outcomes.
- Finance: AI enhances fraud detection by analyzing large datasets in real time to flag suspicious activity. Machine learning strengthens credit risk assessments and optimizes algorithmic trading by identifying subtle market trends and patterns in financial behavior.
- Retail: Artificial intelligence personalizes the shopping experience through intelligent chatbots and recommendation engines that analyze customer preferences. Machine learning optimizes inventory management and demand forecasting, ensuring cost efficiency and timely restocking.
- Manufacturing: AI increases production efficiency with predictive maintenance tools that monitor equipment performance and prevent downtime. Machine learning supports supply chain optimization by analyzing real-time data to streamline operations and minimize waste.
- Logistics: Artificial intelligence automates route optimization and fleet management, reducing delivery times and fuel costs. Machine learning enhances last-mile delivery solutions with real-time adjustments based on traffic conditions and delivery constraints.
- Marketing: AI automates large-scale marketing campaigns, allowing segmentation and hyper-personalized outreach. Machine learning tracks customer behaviors, refining targeting strategies that maximize engagement and conversions.
Understanding these applications equips businesses with actionable insights to deploy AI and machine learning where they create the most value. These technologies solve industry-specific challenges while improving cost-effectiveness, scalability, and measurable impact. Whether focusing on automation, customer personalization, or supply chain efficiency, using AI and machine learning ensures businesses stay innovative and prepared for future opportunities.
Implementing AI and ML strategies
Artificial intelligence and machine learning have the potential to greatly shift business operations, but effective implementation requires a clear roadmap aligned with organizational goals. Strategies must be tailored to achieve speed to market, ensure cost-efficiency, and unlock opportunities for scalability and growth. Businesses must focus on creating solutions that not only address current challenges but also adapt to future needs. Success hinges on a structured approach that integrates these technologies seamlessly while delivering measurable outcomes that justify investment.
Building a data foundation
A strong data foundation ensures AI and machine learning solutions deliver accurate, actionable insights. This includes establishing robust data governance policies, integrating siloed datasets, and ensuring data quality. Organizations should prioritize secure storage, accessibility, and compliance to maximize the value extracted from their data.
Choosing the right use cases
Selecting the most impactful use cases aligns AI and machine learning initiatives with strategic goals. Organizations should evaluate high-impact opportunities, such as automating repetitive processes, optimizing supply chains, or delivering personalized customer experiences. Focusing on measurable outcomes ensures the investment drives tangible business results.
Ensuring scalability and adaptability
AI and machine learning solutions must be scalable to meet growing business needs and adaptable to shifting market conditions. Organizations can achieve this by utilizing cloud-based infrastructure and modular development approaches. Solutions that adapt with organizational demands reduce costs and improve long-term ROI.
Building stakeholder alignment
Effective adoption requires stakeholder buy-in across departments. This involves communicating the benefits of AI and machine learning while addressing potential concerns, such as job displacement or implementation complexity. Transparent collaboration ensures alignment and accelerates adoption.
Measuring outcomes and ROI
Establishing clear metrics and KPIs ensures organizations can track the success of their AI and machine learning initiatives. Metrics should align with business goals, such as cost reductions, improved efficiency, or increased customer satisfaction. Regular evaluation and optimization ensure continued impact.
Adopting AI and machine learning redefines how organizations operate, allowing more competent evaluation, faster processes, and new revenue opportunities. These technologies empower businesses to stay aggressive by enhancing efficiency, improving customer experiences, and unlocking new avenues for innovation. When properly implemented, AI and machine learning strategies become key drivers of business success, fostering long-term adaptability and sustained impact across industries.
Artificial intelligence and machine learning are powerful technologies driving efficiency, scalability, and growth across industries. These tools allow businesses to address challenges with precision, reveal new opportunities, and sustain their operations. At Lumenalta, we craft tailored AI and machine learning strategies that align with your goals, ensuring you achieve measurable success.
Let’s build smarter solutions now.
Table of contents
- Understanding AI and machine learning
- Business impact of machine learning vs artificial intelligence
- 15 key differences between artificial intelligence vs machine learning
- 1. Scope and definition
- 2. Problem-solving approach
- 3. Speed to market
- 4. Cost-effectiveness and scalability
- 5. Data requirements
- 6. Decision-making capabilities
- 7. Adaptability to new scenarios
- 8. Business potential identification
- 9. Implementation complexity
- 10. Return on investment (ROI)
- 11. Future-proofing capabilities
- 12. Change management requirements
- 13. Governance and ethical considerations
- 14. Measurable business impact
- 15. Human involvement
- How AI and machine learning work together to maximize ROI
- Applications of artificial intelligence vs machine learning
- Implementing AI and ML strategies
- Common questions about differences between artificial intelligence vs machine learning
Common questions about differences between artificial intelligence vs machine learning
What is the primary difference between artificial intelligence and machine learning?
How can businesses use artificial intelligence effectively?
Why is machine learning important for scalability?
What industries benefit the most from artificial intelligence?
What are the key challenges of implementing machine learning?
Want to learn how artificial intelligence & machine learning can bring more transparency and trust to your operations?