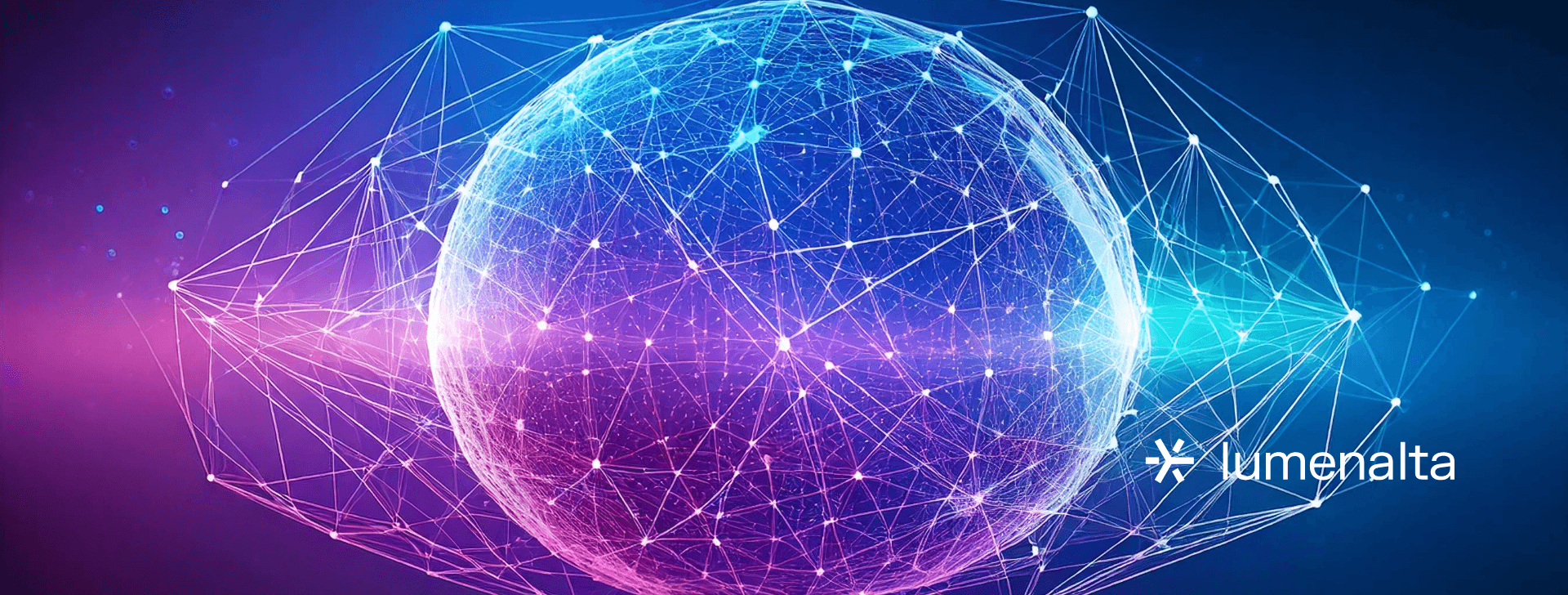
13 benefits and challenges of machine learning
DEC. 13, 2024
Machine learning has become a key enabler for organizations seeking to achieve smarter, faster, and more scalable operations.
Its ability to process vast datasets, identify patterns, and improve outcomes without explicit programming has greatly shifted how businesses approach efficiency and innovation.
From delivering personalized customer experiences to optimizing workflows and revealing actionable insights, machine learning empowers you to make strategic decisions that drive measurable impact.
As businesses continue to integrate this technology into their operations, understanding its potential and the challenges it presents is essential to unlocking its full value.
Key takeaways
- 1. Machine learning provides unparalleled accuracy by analyzing vast datasets and continuously refining its outputs.
- 2. Personalized customer experiences driven by machine learning foster stronger engagement and long-term loyalty.
- 3. Automation powered by machine learning reduces resource consumption and improves productivity.
- 4. Predictive analytics and cost-efficient solutions help businesses optimize operations and anticipate future challenges.
- 5. Organizations adopting machine learning gain a competitive edge through scalability, adaptability, and innovative capabilities.
What is machine learning?
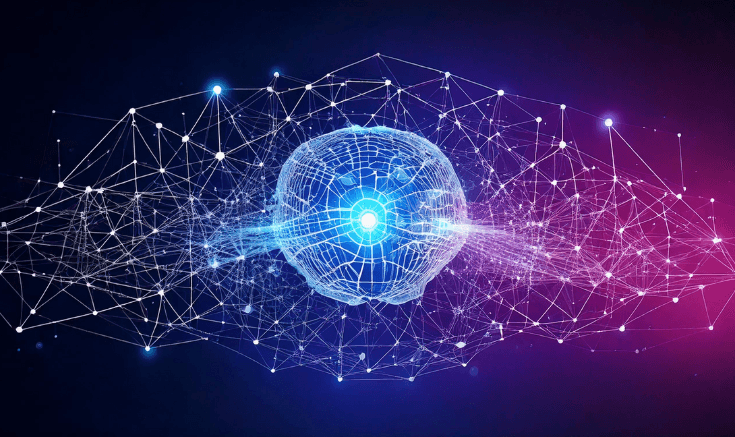
Machine learning is a branch of artificial intelligence that enables systems to learn, adapt, and improve their performance by analyzing data without requiring explicit programming. It uses advanced algorithms and statistical models to identify patterns within datasets, allowing machines to perform tasks that were once reserved for humans.
These tasks include image recognition, language translation, predictive analytics, and anomaly detection, among others. Machine learning leverages three main approaches—supervised learning, where models are trained on labeled data; unsupervised learning, which identifies patterns in unlabeled data; and reinforcement learning, which learns through trial and error to optimize outcomes.
This technology significantly reduces manual intervention by automating repetitive processes, streamlining operations, and improving accuracy across industries. In healthcare, machine learning identifies anomalies in medical imaging to enhance diagnostics. In retail, it delivers personalized recommendations based on customer behavior. Logistics companies rely on machine learning to optimize delivery routes and predict inventory demands.
As machine learning models continuously analyze data, they refine their predictions, providing actionable insights that enable businesses to make smarter decisions and improve operational efficiency. This adaptability makes machine learning especially valuable for handling complex computations at scale, optimizing resource allocation, and solving challenges that traditional methods struggle to address.
Automating decision processes lets organizations unlock opportunities to innovate, reduce costs, and enhance scalability, creating long-term value for businesses worldwide.
7 benefits of machine learning
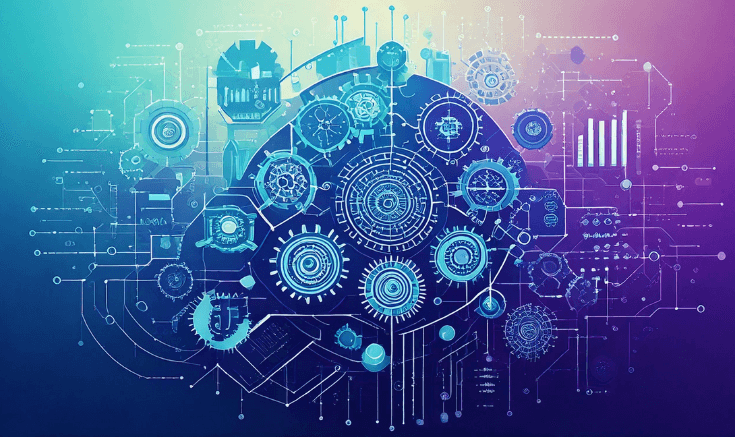
Machine learning provides organizations with the tools to enhance accuracy, automate processes, and achieve greater operational efficiency. Its ability to analyze complex datasets and deliver actionable insights allows businesses to make smarter decisions while reducing costs. From tailoring customer experiences to scaling operations with minimal effort, machine learning supports critical business priorities such as improving workflows, forecasting trends, and optimizing resource allocation. Companies leveraging machine learning are better equipped to meet demands and discover new opportunities.
1. Improved accuracy and insights
Machine learning delivers remarkable accuracy by identifying patterns in complex datasets. Unlike traditional approaches, which are limited by static algorithms, machine learning models continuously adapt to new data, refining their outputs over time. Industries like healthcare use machine learning to detect anomalies in medical imaging with unparalleled precision, improving diagnostic reliability. Organizations gain deeper insights that enhance operational strategies and mitigate risks through the accuracy provided by machine learning.
"Machine learning delivers remarkable accuracy by identifying patterns in complex datasets and refining outputs through continuous adaptation."
2. Tailored customer experiences
Personalization has become essential for engaging and retaining users. Machine learning algorithms analyze user preferences, behaviors, and historical data to customize offerings, such as product recommendations on retail platforms or tailored content on streaming services. This technology creates experiences that resonate with individual customers, fostering long-term loyalty and increasing user satisfaction. Businesses that deploy machine learning for personalization achieve stronger connections with their audiences, driving consistent growth.
3. Automating routine tasks
Organizations often lose time and resources managing repetitive tasks that could be automated. Machine learning streamlines operations by automating functions such as fraud detection, report generation, and email filtering. For example, financial systems use machine learning to flag unusual activity in real time, reducing the need for manual oversight. Automating routine tasks increases efficiency and frees employees to focus on more impactful work, contributing to overall business growth.
4. Predictive capabilities for better planning
Machine learning excels at forecasting future trends, enabling organizations to prepare for potential challenges and opportunities. Businesses rely on machine learning to predict inventory needs, optimize supply chains, and anticipate customer behavior based on historical and current data. Manufacturing companies use predictive maintenance to identify potential equipment failures before they occur, avoiding costly disruptions. Machine learning provides the foresight necessary for effective resource allocation and improved outcomes.
5. Efficiency and cost optimization
Improving operational efficiency is critical for staying ahead, and machine learning offers a solution by identifying inefficiencies and reducing costs. In logistics, machine learning optimizes delivery routes, saving time and resources, while in manufacturing, it minimizes equipment downtime through advanced predictive maintenance. This ability to optimize workflows helps organizations allocate resources more effectively and achieve significant cost savings, boosting profitability.
6. Competitive edge through innovation
Innovation often defines the success of organizations in evolving markets, and machine learning accelerates this by enabling the development of advanced solutions and services. Chatbots powered by machine learning enhance customer service experiences, while supply chain optimization tools use real-time data analysis to improve delivery times and inventory management. Autonomous systems, such as self-driving vehicles, depend on machine learning to process and act on vast amounts of data. Integrating machine learning positions organizations to lead in their sectors through new capabilities and offerings.
7. Seamless scalability and flexibility
Scaling operations while maintaining efficiency can be challenging for growing businesses. Machine learning systems are inherently adaptable, allowing them to handle larger datasets and more complex tasks without diminishing performance. Cloud-based platforms equipped with machine learning models support applications ranging from customer service automation to enterprise-level data analysis. This flexibility enables organizations to meet evolving demands while maintaining the agility needed to succeed in industry circumstances.
Adopting machine learning enables organizations to improve accuracy, deliver innovative solutions, and streamline operations across departments. The benefits range from automating repetitive tasks to achieving cost optimization and scalability, ensuring businesses operate with agility and precision. As industries rely on data to drive results, machine learning empowers companies to maximize their potential, future-proof their operations, and achieve measurable success. Embracing this technology positions organizations to lead in their markets, delivering consistent value while addressing the challenges of modern business conditions.
6 challenges of machine learning
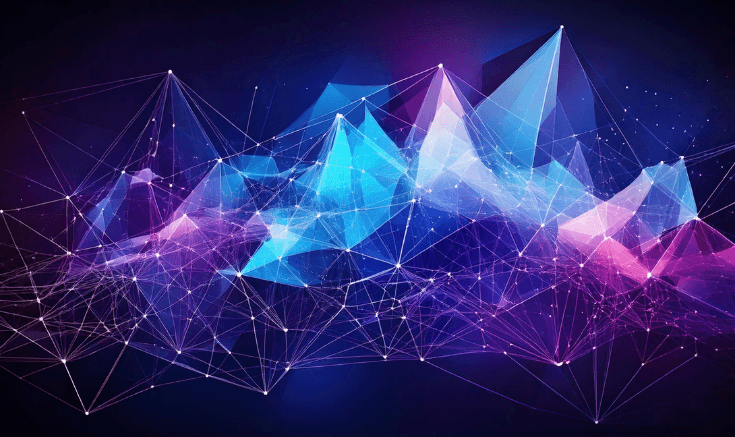
Machine learning offers transformative capabilities, but its adoption demands careful consideration of critical challenges. High-quality data is the foundation of machine learning systems, yet inconsistencies, missing information, and accessibility issues often pose roadblocks. The need for significant computational power, combined with high costs and expertise gaps, further adds to the complexity. Ethical concerns, such as bias and transparency, must also be addressed to build fair and reliable systems that earn stakeholder trust. For organizations to maximize the benefits of machine learning, mitigating these challenges is essential to ensure accuracy, scalability, and long-term impact.
1. Data quality and quantity requirements
Machine learning systems rely heavily on large volumes of high-quality data to deliver accurate and reliable outputs. Poor data quality, inconsistencies, or missing information can lead to flawed predictions and reduced effectiveness. Moreover, organizations may face difficulties collecting sufficient data to train robust models, especially in industries where proprietary information or privacy concerns limit accessibility. Addressing this challenge involves investing in data preprocessing techniques, establishing strong data governance policies, and utilizing synthetic data generation to supplement existing datasets.
2. High computational costs
The computational demands of training machine learning models can be prohibitively expensive, particularly for deep learning algorithms that require significant processing power and memory. These costs include hardware investments, such as GPUs or TPUs, and ongoing cloud infrastructure expenses. Small and medium-sized enterprises often find these costs a barrier to entry. Strategies like optimizing model architecture, using transfer learning, and leveraging open-source tools can help reduce computational overhead and make machine learning more accessible.
3. Complexity and lack of interpretability
Machine learning models, especially deep learning systems, are often described as "black boxes" due to their lack of transparency. Understanding how these models arrive at specific predictions or decisions can be challenging, making it difficult to build trust among stakeholders or address regulatory compliance requirements. This complexity poses a significant hurdle in industries like healthcare and finance, where accountability and explainability are crucial. Solutions such as adopting explainable AI techniques, simplifying model structures, or using interpretable models like decision trees can help improve transparency without compromising performance.
4. Ethical concerns and bias
Bias in machine learning algorithms can lead to unfair or discriminatory outcomes, especially if the training data reflects existing social inequalities or stereotypes. Ethical concerns also arise when algorithms make decisions that impact individuals, such as approving loans or hiring candidates, without clear oversight. Tackling this issue involves implementing bias detection and mitigation techniques, diversifying training datasets, and establishing ethical AI frameworks to guide development and deployment.
5. Security vulnerabilities
Machine learning systems are susceptible to adversarial attacks, where malicious actors manipulate input data to deceive models. For instance, attackers can subtly alter images or data inputs to produce incorrect outputs, compromising the system's reliability. Additionally, machine learning models often require access to sensitive data, increasing the risk of breaches or unauthorized access. Enhancing security measures through techniques like adversarial training, encryption, and robust access controls can mitigate these risks and safeguard machine learning implementations.
6. Skill gap and expertise dependency
Developing and maintaining machine learning solutions requires specialized knowledge in fields like data science, algorithm design, and software engineering. The shortage of skilled professionals often slows down machine learning adoption, particularly for smaller organizations without access to extensive resources. Investing in training programs, collaborating with external experts, and leveraging pre-built machine learning solutions can help bridge the skill gap and accelerate adoption.
The challenges of machine learning—ranging from data quality and ethical concerns to computational costs and security vulnerabilities—are significant but not insurmountable. Confronting these obstacles head-on enables organizations to unlock the full potential of machine learning, driving innovation, improving efficiency, and achieving measurable business outcomes.
Machine learning and AI
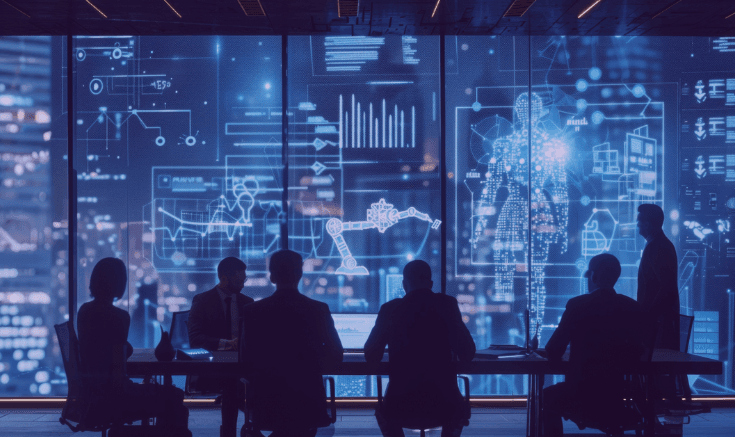
Machine learning and artificial intelligence (AI) work hand-in-hand to create systems that can adapt, learn, and deliver results that enhance operational performance. While AI focuses on creating systems capable of reasoning and solving problems, machine learning enables these systems to learn autonomously from data, improving their performance over time. Together, these technologies are transforming industries through applications that optimize workflows, reduce costs, and unlock new opportunities. From automating customer interactions to improving safety in autonomous systems, the combined power of AI and machine learning is redefining how businesses achieve measurable success.
AI-powered tools such as chatbots use machine learning algorithms to analyze user data, delivering accurate and tailored responses in real time. These tools enhance customer satisfaction while freeing up resources, making service delivery both scalable and cost-effective. In industries such as transportation, AI complements machine learning’s ability to identify patterns by enabling advanced processes, as seen in self-driving vehicles that improve safety through precise and timely actions. Similarly, healthcare organizations use machine learning models alongside AI-backed insights to analyze medical data, streamline diagnostics, and enhance patient outcomes.
The integration of AI and machine learning empowers businesses to make faster, data-led decisions, helping them address challenges such as resource allocation, demand forecasting, and risk management. These technologies work seamlessly with existing systems, allowing organizations to scale their operations without major infrastructure overhauls. For example, machine learning models optimize supply chains, ensuring products are delivered efficiently, while AI enhances evaluation through real-time analysis of market trends. This synergy creates future-proof strategies that enable businesses to remain competitive and adaptive to market changes.
As industries adopt AI and machine learning solutions, the measurable impact becomes clear. Businesses achieve greater efficiency, reduce operational risks, and improve scalability, all while identifying untapped opportunities for growth. From finance and logistics to retail and healthcare, organizations are unlocking innovation and achieving consistent, long-term results.
"The integration of AI and machine learning empowers businesses to make faster, data-led decisions, helping them address challenges such as resource allocation, demand forecasting, and risk management."
Getting started with machine learning
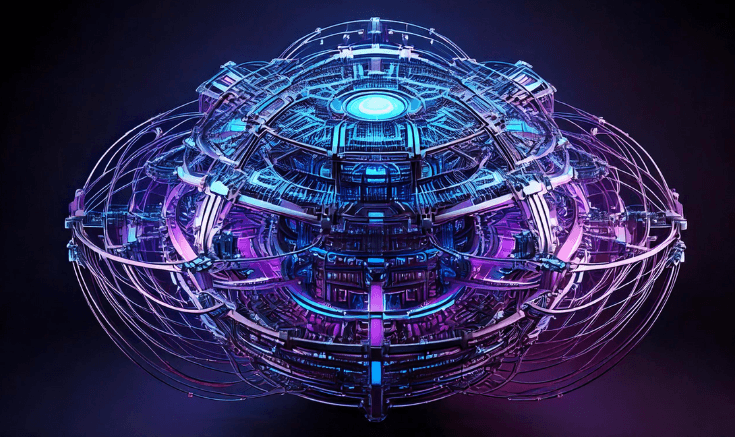
Implementing machine learning begins with a clear understanding of your business goals and the challenges you aim to address. Identifying specific use cases where machine learning can deliver measurable benefits, such as improving customer engagement or optimizing operations, is the first step in the journey. Start small by piloting machine learning models on a manageable dataset, ensuring the results align with your desired outcomes before scaling.
Organizations should focus on building a strong foundation in data management, as high-quality data is essential for the success of machine learning models. Establishing robust data pipelines, leveraging cloud-based platforms, and collaborating with experienced data scientists can help streamline the process. Pre-built machine learning tools and frameworks offer an excellent starting point, enabling businesses to implement solutions without requiring deep technical expertise. With the right strategy and resources, businesses can utilize the power of machine learning to unlock efficiencies, and drive innovation.
Machine learning is not just about algorithms—it’s about creating smarter, faster, and more impactful solutions for your business. Making use of its power equips organizations to unlock efficiency, scalability, and innovation tailored to their unique needs. At Lumenalta, we specialize in delivering machine learning solutions that align seamlessly with your goals, enabling you to lead in your industry.
Let’s build a brighter future together.
table-of-contents
- What is machine learning?
- 7 benefits of machine learning
- 1. Improved accuracy and insights
- 2. Tailored customer experiences
- 3. Automating routine tasks
- 4. Predictive capabilities for better planning
- 5. Efficiency and cost optimization
- 6. Competitive edge through innovation
- 7. Seamless scalability and flexibility
- 6 challenges of machine learning
- 1. Data quality and quantity requirements
- 2. High computational costs
- 3. Complexity and lack of interpretability
- 4. Ethical concerns and bias
- 5. Security vulnerabilities
- 6. Skill gap and expertise dependency
- Machine learning and AI
- Getting started with machine learning
- Common questions about machine learning
Common questions about machine learning
What are the top benefits of machine learning for businesses?
How does machine learning differ from artificial intelligence?
What industries benefit the most from machine learning?
What challenges do companies face when implementing machine learning?
How does machine learning support innovation?
Want to learn how machine learning can bring more transparency and trust to your operations?