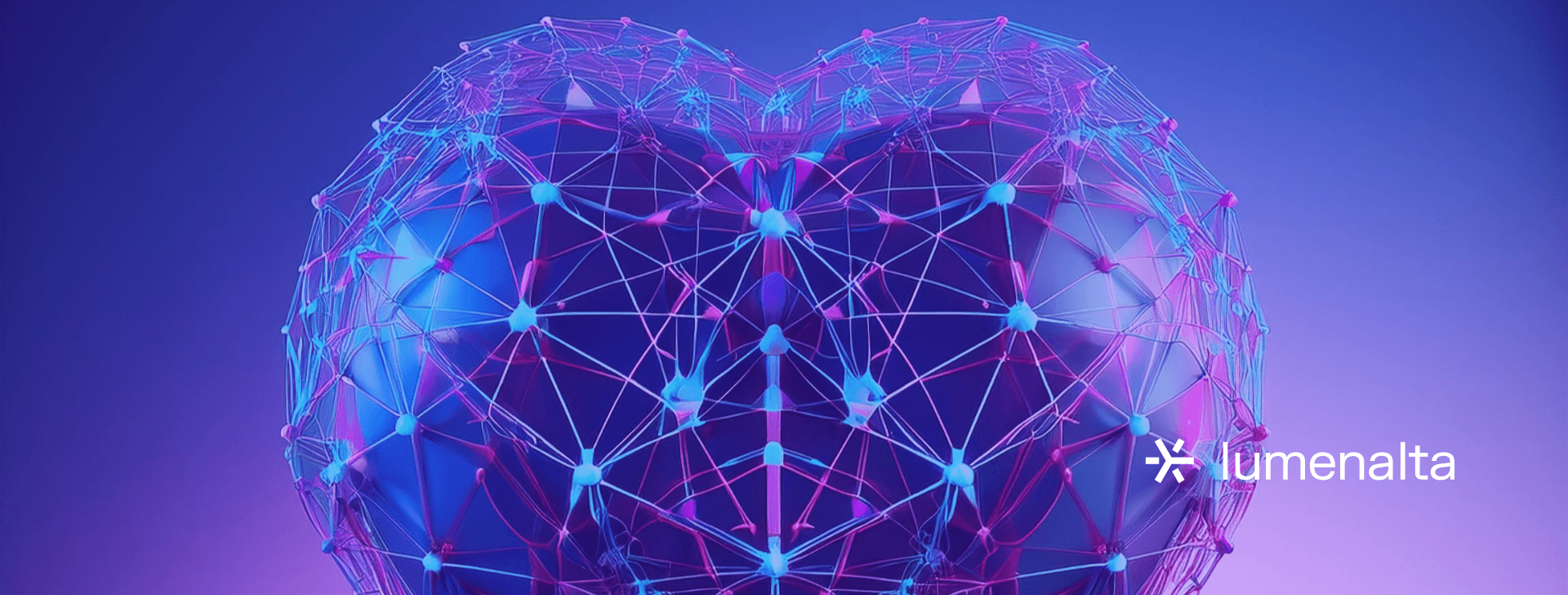
17 benefits of machine learning in healthcare
DEC. 14, 2024
9 Min Read
Machine learning is reshaping healthcare, driving precision, efficiency, and innovation at every level of care delivery.
Its applications are transforming patient outcomes, operational workflows, and the discovery of life-saving treatments. By leveraging advanced algorithms and data analysis, machine learning empowers healthcare organizations to tackle complex challenges, improve care quality, and build sustainable solutions for the future.
Key takeaways
- 1. Machine learning enables earlier and more precise disease diagnosis, helping healthcare providers intervene before conditions worsen.
- 2. Personalized treatment plans, powered by machine learning, ensure tailored care and better patient outcomes.
- 3. Predictive analytics support real-time health monitoring and proactive interventions, transforming chronic disease management.
- 4. Machine learning enhances operational efficiency, reducing healthcare costs and streamlining administrative workflows.
- 5. Advanced algorithms enable faster drug discovery and accurate medical imaging analysis, driving innovation in healthcare.
What is machine learning in healthcare?
Machine learning in healthcare refers to the application of advanced algorithms and computational models to analyze complex medical data, enabling insights and predictions that were previously unattainable. Machine learning empowers healthcare providers to deliver more precise, efficient, and personalized care by leveraging patterns and trends within massive datasets. The use of machine learning in healthcare spans various areas such as diagnostics, treatment planning, drug discovery, and administrative operations, making it an invaluable tool for modern medicine.
One of the core strengths of machine learning is its ability to process vast amounts of structured and unstructured data—such as electronic health records, medical imaging, and genomic sequences—with unparalleled speed and accuracy. This capability supports earlier disease detection, predictive analytics for patient outcomes, and enhanced operational efficiency. Additionally, machine learning facilitates continuous learning, adapting its models as new data emerges, ensuring ongoing healthcare delivery improvements. As a result, machine learning is transforming healthcare into a more proactive and data-centric industry, significantly improving patient and provider outcomes.
17 benefits of machine learning in healthcare
The adoption of machine learning in healthcare is creating groundbreaking opportunities to address long-standing challenges while significantly enhancing patient outcomes. This innovation enables healthcare providers to deliver faster, more accurate, and cost-effective services. From personalized treatments to predictive analytics, the capabilities are vast and varied, helping transform the way the industry operates. Healthcare organizations are using machine learning to optimize resources, improve diagnostic accuracy, and streamline operational workflows, all while fostering better patient experiences.
Each advancement led by machine learning not only improves the quality of care but also helps mitigate costs, strengthen data security, and unlock new paths for innovation. With its ability to analyze massive datasets efficiently, machine learning is bridging the gap between clinical expertise and actionable insights.
1. Earlier and more accurate disease diagnosis
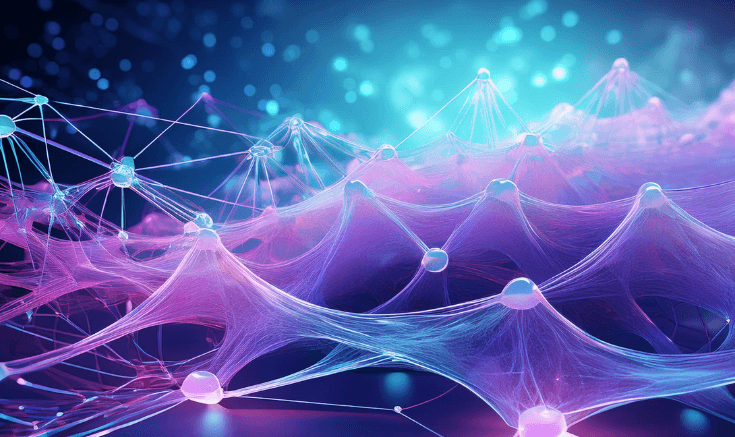
Machine learning is redefining diagnostic processes by enabling earlier and more precise detection of diseases. Its models analyze complex patient data patterns, identifying subtle indicators of conditions that might otherwise go unnoticed. This precision is particularly crucial for early-stage diseases like cancer or rare genetic disorders, where timely intervention can save lives.
For example, predictive algorithms trained on medical imaging data, such as CT scans or MRIs, can detect anomalies accurately. These tools assist radiologists and ensure consistent and unbiased interpretation of test results. Focusing on granular details, they help clinicians make informed decisions faster, leading to better outcomes. Additionally, as these models learn and improve with exposure to more data, their diagnostic capabilities become reliable over time.
"Machine learning models excel at analyzing complex patterns in patient data, identifying subtle indicators of conditions that might otherwise go unnoticed."
2. Personalized treatment plans
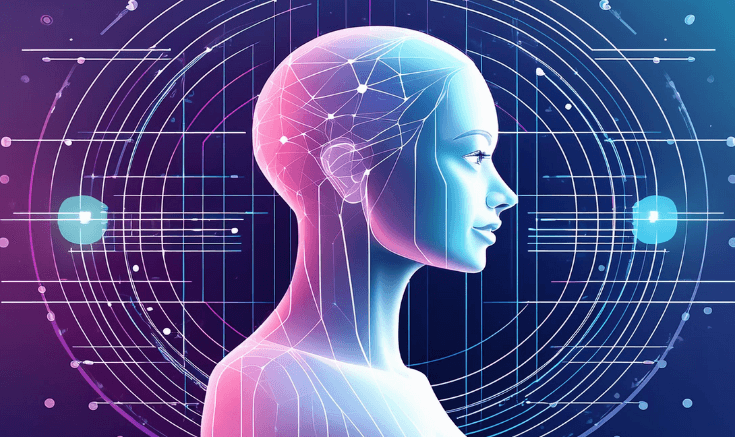
The ability to tailor medical treatments to individual patients is another key benefit of machine learning in healthcare. Treatment decisions often rely on various factors, including genetic information, medical history, and lifestyle habits. Machine learning enables a comprehensive analysis of these variables to suggest optimized care pathways for every patient.
For instance, pharmacogenomics, which involves understanding how genes affect a person’s drug response, has gained immense traction through machine learning. Algorithms can predict which medications will work best for a specific patient, minimizing trial-and-error approaches and improving treatment effectiveness. This precision ensures patients receive interventions designed to maximize efficacy while reducing potential side effects.
3. Accelerated drug discovery and development
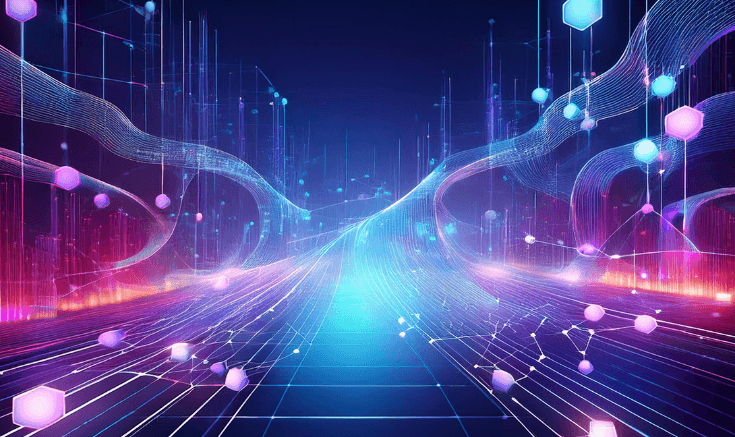
Developing new medications is traditionally a lengthy and expensive process. Machine learning is helping pharmaceutical companies shorten the drug discovery timeline by identifying potential compounds and testing their efficacy with higher accuracy. By analyzing vast biochemical datasets, algorithms can predict how a drug will interact with target proteins, reducing the need for labor-intensive and time-consuming trials.
The process also extends to repurposing existing drugs for new conditions. Machine learning has helped identify overlooked medication uses, providing new treatment options for patients faster than conventional methods. These advances lower development costs, increase the speed of innovation and bring vital therapies to market more quickly.
4. Enhanced medical imaging analysis
Medical imaging is one of the most transformative applications of machine learning in healthcare. Algorithms can analyze images such as X-rays, ultrasounds, and pathology slides with precision that rivals or exceeds that of human experts. Machine learning models detect conditions ranging from fractures to life-threatening diseases like strokes or cancer.
This technology ensures quicker turnaround times for diagnoses, especially when radiologists are overwhelmed. By flagging high-priority cases, machine learning allows healthcare providers to allocate their time and expertise more effectively, ultimately improving the speed and quality of care.
5. Improved patient care and outcomes
Machine learning improves patient care by providing actionable insights that improve both short-term and long-term health outcomes. Tools that leverage predictive analytics can foresee potential complications or identify patients at high risk of deteriorating health. This capability helps healthcare teams proactively intervene and adjust care plans as needed.
For instance, wearable devices that collect continuous data on heart rate, blood pressure, or glucose levels use machine learning to analyze trends in real time. These insights enable timely adjustments to medication or lifestyle interventions, empowering patients to take an active role in their health while reducing the burden on healthcare systems.
6. Efficient clinical trial processes
Clinical trials often delay due to inefficient patient recruitment, data collection, and monitoring processes. Machine learning optimizes these elements by identifying suitable study candidates, predicting trial outcomes, and monitoring participant adherence. Algorithms can analyze patient databases to match individuals with appropriate trials, speeding up recruitment.
Additionally, machine learning ensures data collected during trials is standardized and easily interpretable. Real-time monitoring tools detect anomalies or adverse events, enabling researchers to respond promptly and improve the trial's safety and efficacy.
7. Predictive analytics for patient health
Healthcare providers are leveraging predictive analytics powered by machine learning to foresee health trends and outcomes. These tools analyze patterns in patient data to anticipate potential health issues before they arise, allowing clinicians to implement preventative measures. For example, algorithms can predict hospital readmission risks, helping teams develop strategies to reduce them.
These capabilities are particularly impactful in managing chronic diseases, where early intervention significantly improves quality of life and reduces healthcare costs. By predicting patient needs, machine learning supports more proactive and targeted care delivery.
8. Streamlined administrative tasks
Administrative inefficiencies burden healthcare systems and divert resources away from patient care. Machine learning is helping to streamline repetitive, time-consuming tasks such as billing, appointment scheduling, and medical coding. These tools automate routine workflows, freeing up valuable time for healthcare professionals to focus on direct patient care.
For example, natural language processing algorithms can review medical records and automatically assign correct billing codes, reducing errors and accelerating insurance claim processes. Similarly, chatbots powered by machine learning assist patients with scheduling appointments, answering common queries, and providing follow-up reminders. These advancements lead to significant cost savings and improved operational efficiency for healthcare providers.
9. Cost reduction in healthcare delivery
Healthcare organizations face immense pressure to manage costs while maintaining high standards of care. Machine learning reduces costs by optimizing resources, enhancing operational workflows, and minimizing waste. Predictive analytics helps allocate staff, equipment, and facilities based on anticipated demand, reducing inefficiencies.
Additionally, automated tools detect patterns indicative of fraudulent insurance claims, saving millions in unnecessary payouts. By addressing these challenges, machine learning helps healthcare providers operate more efficiently, making quality care more accessible to patients.
10. Enhanced data privacy and security
The increasing digitization of healthcare data has raised concerns about privacy and security. Machine learning offers robust solutions to protect sensitive patient information. Advanced algorithms identify potential threats, detect anomalies in network traffic, and prevent unauthorized access to data.
One key innovation is anomaly detection, where machine learning models monitor systems for unusual activities that could indicate a cyberattack. Additionally, encryption techniques powered by machine learning ensure data is securely transmitted and stored, meeting regulatory requirements and building trust among patients and providers.
11. Real-time health monitoring
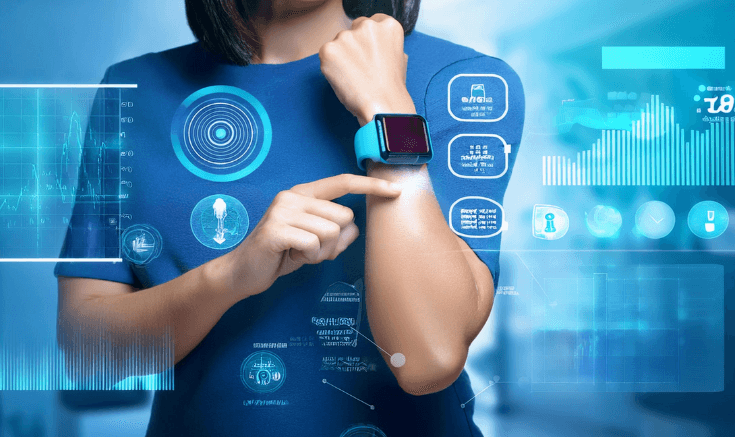
The rise of wearable devices and Internet of Medical Things (IoMT) has made real-time health monitoring a reality. Machine learning processes data collected from sensors, such as heart rate, oxygen saturation, or glucose levels, to provide actionable insights. These tools alert patients and healthcare providers to potential health risks, enabling timely interventions.
For example, machine learning algorithms can analyze heart rate variability and detect arrhythmias, prompting users to seek medical attention before complications arise. This proactive approach improves patient outcomes while reducing the likelihood of emergencies.
12. Optimized resource allocation in hospitals
Efficient resource allocation is critical for ensuring hospitals run smoothly, especially during periods of high demand. Machine learning assists administrators in predicting patient admission rates, optimize bed management, and ensure critical equipment availability. Algorithms analyze historical data alongside real-time metrics to forecast needs accurately.
For instance, predictive models help determine the staffing levels required during flu season or other health crises. This foresight prevents overcrowding, minimizes wait times, and ensures patients receive prompt care without compromising quality.
13. Improved chronic disease management
Managing chronic conditions, such as diabetes, hypertension, and COPD, requires continuous monitoring and personalized care plans. Machine learning enhances this process by analyzing patient data to identify trends, predict potential complications, and suggest tailored interventions. These tools empower patients and healthcare providers to take a more proactive approach to disease management.
Remote monitoring systems, powered by machine learning, track patient progress and generate alerts for deviations from expected patterns. This ensures timely adjustments to treatment, reducing hospital visits and improving the overall quality of life for individuals with chronic conditions.
14. Faster and more accurate genetic analysis
Advancements in genomics have introduced new possibilities for understanding genetic predispositions to diseases. Machine learning accelerates genetic analysis by identifying patterns within DNA sequences that indicate risk factors or hereditary conditions. These insights enable clinicians to recommend targeted preventative measures or treatments.
For example, machine learning models identify genetic markers linked to conditions like Alzheimer’s or certain cancers, helping patients take preemptive action. Faster genetic analysis also aids researchers in exploring new therapies and understanding the underlying causes of complex diseases.
15. Enhanced fraud detection in healthcare claims
Fraudulent claims cost healthcare systems billions of dollars annually. Machine learning algorithms excel at detecting suspicious patterns in billing and insurance data, reducing the likelihood of fraud. These tools flag inconsistencies, such as duplicate claims or exaggerated charges, for further investigation.
Automated fraud detection not only saves money but also increases the efficiency of claims processing. By catching fraudulent activity early, machine learning ensures resources are directed toward legitimate claims and improves trust in healthcare systems.
16. Improved surgical planning and outcomes
Surgical procedures demand precision and careful planning. Machine learning supports surgeons by analyzing patient data, imaging, and past outcomes to recommend optimal approaches for each case. Tools like robotic-assisted surgery systems use machine learning to enhance accuracy and minimize risks.
Machine learning-powered devices provide real-time feedback during procedures, helping surgeons make critical adjustments. This technology reduces complications, shortens recovery times, and improves patient satisfaction.
17. Better public health monitoring and epidemic prediction
Machine learning is pivotal in public health by identifying patterns and trends that inform policy decisions. Predictive models analyze data from multiple sources, such as hospital records, social media, and environmental sensors, to forecast disease outbreaks and epidemics. Early detection allows authorities to allocate resources effectively and implement preventative measures.
For example, machine learning was instrumental in monitoring and predicting the spread of COVID-19, enabling governments to respond swiftly. These capabilities continue to improve global preparedness for future public health challenges.
The transformative power of machine learning in healthcare is undeniable, reshaping the industry by addressing challenges that once seemed insurmountable. These applications enhance diagnostic accuracy and treatment personalization and create efficiencies that benefit patients and providers alike. As the industry adopts these technologies on a broader scale, the potential to deliver cost-effective, scalable, and patient-centric solutions continues to grow.
Machine learning fosters a future where healthcare systems can predict, prevent, and treat conditions with precision and agility. Its integration into critical areas, from real-time monitoring to resource optimization, ensures more equitable access to quality care worldwide. This progress underscores how machine learning empowers the healthcare industry to meet evolving demands while improving individual and public health outcomes.
"Wearable devices that collect continuous data use machine learning to analyze trends in real time, enabling timely adjustments to medication or lifestyle interventions."
Implementing machine learning in healthcare
The successful integration of machine learning into healthcare requires careful planning, robust infrastructure, and a patient-first approach. Healthcare providers must prioritize creating systems that collect and process vast amounts of data securely and efficiently. This often involves leveraging cloud platforms, advanced analytics, and interoperable health information systems to ensure seamless data exchange.
Equally important is the collaboration between clinicians, data scientists, and technologists. These partnerships ensure that machine learning models are technically sound and aligned with real-world clinical needs. Training healthcare teams to interpret and utilize insights generated by these algorithms is critical to maximizing their impact. Ethical considerations like patient consent and transparency must remain central to every implementation effort, fostering trust and compliance with regulatory standards.
Scalability is another essential aspect, as healthcare organizations look to expand machine learning applications across departments and facilities. Pilot programs, rigorous testing, and continuous feedback loops help fine-tune these tools, ensuring they deliver consistent, high-quality results. Thoughtful implementation strategies ensure that machine learning enhances care delivery and strengthens operational efficiency and patient satisfaction.
Challenges of machine learning in healthcare
Adopting machine learning in healthcare is driving significant advancements, but it has obstacles. These challenges highlight the complexity of implementing technology in a domain that prioritizes patient safety, data integrity, and regulatory compliance. While the benefits of machine learning in healthcare are clear, ensuring its successful integration requires addressing several critical hurdles.
- Data quality and availability: Machine learning models use comprehensive datasets to function effectively. In healthcare, data often exists in fragmented silos or in inconsistent formats, making integration and analysis difficult. Missing or inaccurate data can reduce the accuracy and reliability of predictions.
- Regulatory compliance: Healthcare is a highly regulated industry, and compliance with standards such as HIPAA (Health Insurance Portability and Accountability Act) or GDPR (General Data Protection Regulation) is essential. Ensuring that machine learning tools adhere to these frameworks adds complexity to their deployment.
- Algorithm bias: Bias in machine learning algorithms remains a pressing concern. Training models on datasets that are not diverse or representative can lead to biased predictions, disproportionately affecting specific patient groups and undermining equitable care.
- Ethical concerns: Using machine learning in healthcare raises questions about patient privacy, informed consent, and decision transparency. Clear guidelines are needed to address these issues while maintaining trust between providers and patients.
- Integration with existing systems: Many healthcare organizations operate with legacy systems that are not easily compatible with modern machine learning tools. Integrating these solutions without disrupting workflows requires significant time, resources, and expertise.
- Cost and resource constraints: Implementing machine learning solutions often involves substantial investment in technology, infrastructure, and talent. Smaller healthcare providers or those in resource-limited settings may find these barriers challenging to overcome.
- Interpretability and trust: Clinicians and patients may struggle understanding how machine learning algorithms arrive at their recommendations. Building interpretable and explainable tools is crucial for fostering confidence and widespread adoption.
- Scalability challenges: Expanding machine learning solutions across large healthcare networks requires robust frameworks and processes. Ensuring consistency and maintaining performance at scale remains a technical and operational challenge.
Addressing these challenges ensures smoother adoption and strengthens the foundation for scalable, sustainable, and impactful machine learning solutions. These efforts pave the way for more inclusive, efficient, and trustworthy healthcare systems, enabling technology to support clinicians and patients more effectively. Success lies in identifying and overcoming these barriers to create a future where machine learning is seamlessly integrated into everyday care.
Business impact of machine learning in healthcare
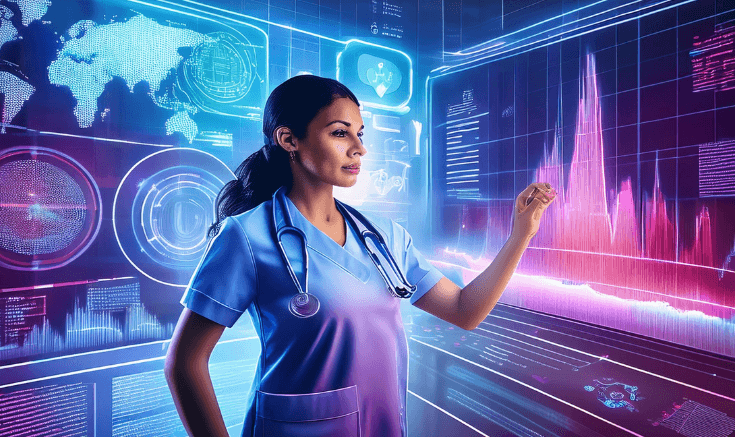
Machine learning is redefining the healthcare industry by delivering measurable outcomes that drive operational efficiency and patient-centered care. Organizations leveraging this technology are seeing transformative results in key areas, including cost management, service scalability, and enhanced clinical evaluation. Analyzing and acting on large datasets enables healthcare providers to make better-informed decisions, resulting in improved care quality and optimized resource allocation.
One of the most significant impacts of machine learning is its ability to reduce costs while maintaining or even enhancing care standards. Predictive models streamline operations by anticipating patient demand, reducing unnecessary procedures, and identifying high-risk cases early. These efficiencies translate into tangible financial savings for hospitals and clinics. Machine learning also enhances the patient experience by enabling personalized treatment plans, real-time monitoring, and proactive care approaches. This alignment of technology with patient needs fosters trust and satisfaction.
On an organizational level, machine learning supports scalability and innovation, empowering providers to respond to evolving healthcare demands. Solutions such as automated administrative tools and advanced diagnostic systems reduce manual workloads, allowing teams to focus on delivering quality care. These improvements enhance operational performance and position healthcare organizations as leaders in technological innovation, attracting investment and improving competitiveness in the market.
As the healthcare industry continues integrating machine learning, the focus on measurable outcomes ensures that investments yield sustainable benefits. Organizations prioritizing data strategies are better equipped to adapt to future challenges while maintaining a commitment to quality care and patient well-being. The growing adoption of machine learning underscores its potential to reshape healthcare, delivering both immediate and long-term value for patients, providers, and stakeholders.
Machine learning in healthcare is not just a technological innovation—it’s the key to delivering smarter, more efficient, and patient-focused care. Enhancing evaluation, optimizing operations, and unlocking personalized treatment possibilities enables machine learning to empowers healthcare providers to drive meaningful results. At Lumenalta, we specialize in developing tailored machine learning solutions that address critical healthcare challenges and deliver measurable impact.
Let’s build a brighter path forward together.
table-of-contents
- What is machine learning in healthcare?
- 17 benefits of machine learning in healthcare
- 1. Earlier and more accurate disease diagnosis
- 2. Personalized treatment plans
- 3. Accelerated drug discovery and development
- 4. Enhanced medical imaging analysis
- 5. Improved patient care and outcomes
- 6. Efficient clinical trial processes
- 7. Predictive analytics for patient health
- 8. Streamlined administrative tasks
- 9. Cost reduction in healthcare delivery
- 10. Enhanced data privacy and security
- 11. Real-time health monitoring
- 12. Optimized resource allocation in hospitals
- 13. Improved chronic disease management
- 14. Faster and more accurate genetic analysis
- 15. Enhanced fraud detection in healthcare claims
- 16. Improved surgical planning and outcomes
- 17. Better public health monitoring and epidemic prediction
- Implementing machine learning in healthcare
- Challenges of machine learning in healthcare
- Business impact of machine learning in healthcare
- Common questions about machine learning in healthcare
Common questions about machine learning in healthcare
What is machine learning in healthcare?
How does machine learning benefit patient care?
What role does machine learning play in medical imaging?
Is machine learning secure for healthcare applications?
How does machine learning impact healthcare costs?
Want to learn how machine learning can bring more transparency and trust to your operations?