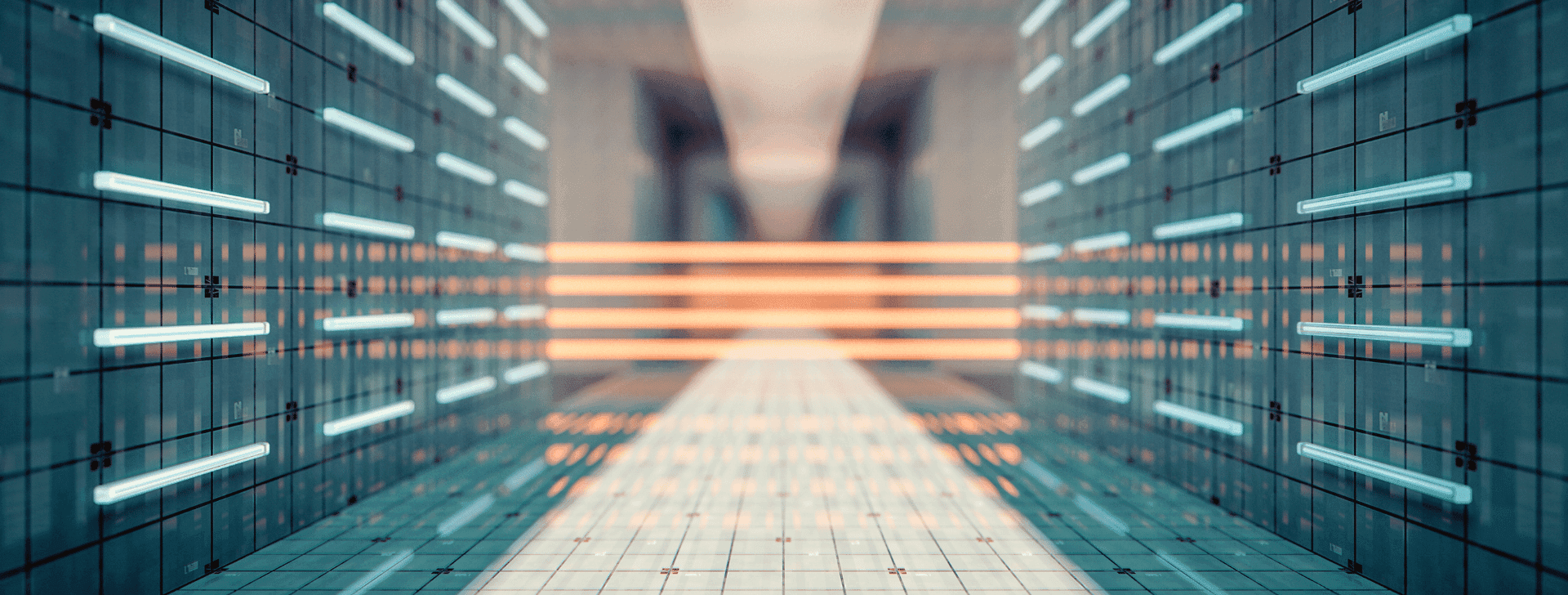
How to create a data governance framework that lasts
SEP. 24, 2024
10 Min Read
The growing popularity of GPT models has brought AI-powered tools to the forefront of digital transformation across industries.
As businesses scale and the adoption of AI and machine learning (ML) accelerates, a sustainable data governance framework is crucial to managing data complexity, quality, and regulatory requirements. This guide provides an in-depth look at creating a data governance framework that not only meets immediate needs but evolves with your organization to ensure long-term success.
Key takeaways
- 1. Data governance policies and procedures: Establishing clear guidelines and roles is essential for maintaining data integrity and ensuring compliance across the organization.
- 2. Metadata management enhances data usability: Effective metadata management supports data discovery, transparency, and accurate decision-making within the organization.
- 3. Scalability and integration are key challenges: A scalable data governance framework is necessary to handle growing data needs while ensuring seamless integration with existing systems.
- 4. AI's role in data governance is transformative: Leveraging AI for compliance monitoring and data quality management significantly enhances efficiency and reduces manual oversight.
- 5. Measuring success with KPIs: Tracking metrics like data quality, compliance rate, and cost savings helps organizations evaluate the impact of their data governance efforts.
Introduction to data governance
Data governance is about establishing a structured approach to managing data integrity, quality, and security throughout an organization. A well-designed data governance framework helps ensure that data remains a reliable and strategic asset for decision-making, enabling better outcomes and more informed strategies. For businesses, prioritizing data governance means laying the groundwork for consistent, secure, and valuable data usage across all operations.
What is a data governance framework?
A data governance framework is a set of policies, procedures, standards, and technologies that help organizations manage their data assets effectively. It includes the rules that dictate how data is collected, stored, used, and protected, ensuring data integrity, availability, and compliance with relevant regulations.
Data governance frameworks aim to:
- Ensure data accuracy, consistency, and completeness.
- Protect sensitive information and maintain privacy.
- Facilitate compliance with industry regulations and standards.
- Enhance data-driven decision-making capabilities.
A comprehensive data governance framework not only standardizes data management across the organization but also empowers businesses to leverage their data as a strategic asset, driving better decision-making and ensuring compliance in an increasingly data-centric world.
"Data governance is about establishing a structured approach to managing data integrity, quality, and security throughout an organization."
Key components of a data governance framework
Implementing a data governance framework involves a comprehensive set of components that work together to ensure that data is managed, protected, and utilized efficiently within an organization. Each component plays a specific role in establishing clear guidelines and maintaining data integrity, quality, and compliance. Below, we explore the fundamental elements that form the backbone of an effective data governance strategy.
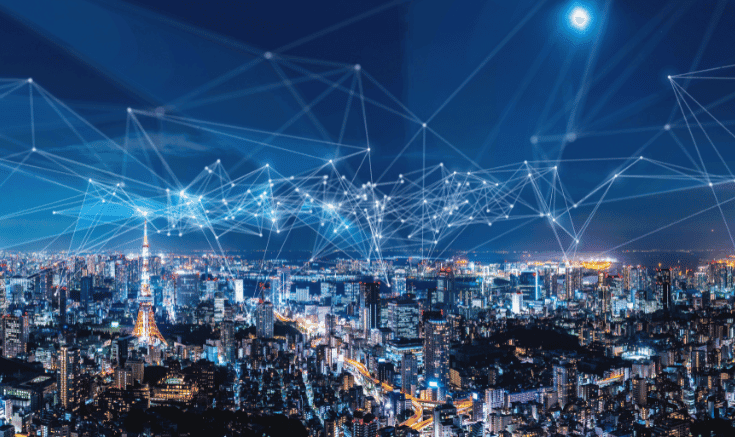
1. Data governance policies and procedures
Data governance policies provide the foundation for how data is handled within an organization. They define the principles, guidelines, and protocols for data management practices. These policies should cover aspects like data access controls, data privacy standards, data classification, and usage guidelines.
- Data quality standards: Establish benchmarks for data accuracy, consistency, and completeness.
- Access control policies: Define who has access to specific data and under what circumstances.
- Data classification: Organize data into categories based on its sensitivity and importance.
A well-structured data governance policy not only secures sensitive information but also ensures that your data operations align with both internal standards and regulatory requirements, paving the way for greater organizational trust and efficiency.
2. Metadata management
Metadata management is crucial for providing context to data, describing its source, structure, and meaning. This component helps in improving data discoverability and usability while ensuring transparency and traceability in decision-making.
- Data catalogs: Centralized repositories for metadata to facilitate easier data search and retrieval.
- Data lineage: The ability to track the flow of data from its origin through various transformations to ensure data integrity.
Robust metadata management is the backbone of any data governance framework, ensuring data transparency and empowering stakeholders to make informed decisions based on reliable information.
3. Data stewardship
Data stewardship involves assigning specific individuals or teams the responsibility of managing data assets in alignment with governance policies. Data stewards play a vital role in maintaining data quality and ensuring compliance with governance standards.
- Data integrity: Regularly monitor and address data inaccuracies or inconsistencies.
- Role-based access: Ensure that data access is limited to authorized personnel based on their roles.
Effective data stewardship not only enhances data quality but also fosters a culture of accountability, ensuring that data assets are managed responsibly and consistently across the organization.
4. Compliance and security management
This component focuses on ensuring that all data handling practices comply with industry regulations such as GDPR, CCPA, or HIPAA. Security measures are implemented to protect data from unauthorized access or breaches.
- Data encryption: Secure sensitive data both at rest and in transit.
- Audit trails: Maintain detailed logs to track data access and modifications for compliance purposes.
A strong focus on compliance and security management ensures that data governance practices are aligned with regulatory standards, minimizing risks and protecting the organization's reputation.
5. Data architecture and integration
Data architecture refers to the structured design and organization of data assets within an organization. This component is vital for integrating data from various sources, ensuring it is accessible and usable across the enterprise.
- Data models: Standardize how data is stored, accessed, and updated within systems.
- Data integration tools: Facilitate seamless data sharing and communication between different systems.
Data architecture and integration form the foundation for a unified data strategy, enabling smooth data flow and enhancing operational efficiency across the organization.
Understanding and implementing these key components of a data governance framework is essential for building a strong data-driven culture within any organization. When these elements work together, they create a cohesive approach that not only supports data integrity and compliance but also drives business growth and innovation. By focusing on these core areas, organizations can lay the groundwork for a sustainable and scalable data governance strategy that adapts to evolving technological and regulatory landscapes.
Benefits of data governance frameworks
Implementing a data governance framework delivers tangible advantages across multiple business areas:
- Enhanced decision-making: With high-quality, consistent data, decision-makers can rely on accurate information to drive strategic initiatives. According to research, 98% of organizations reported that AI-driven governance improved decision-making processes, enabling smarter business strategies.
- Improved data quality: A structured governance framework reduces errors and inconsistencies, ensuring reliable data for analytics and AI applications. In a recent study, 96% of organizations reported enhanced data quality and consistency, demonstrating the value of effective data governance.
- Regulatory compliance: Governance frameworks ensure adherence to data privacy laws like GDPR and CCPA, minimizing the risk of legal violations and building trust with customers and stakeholders.
- Increased transparency: Clear guidelines on data usage and access promote accountability across the organization, fostering a culture of data responsibility.
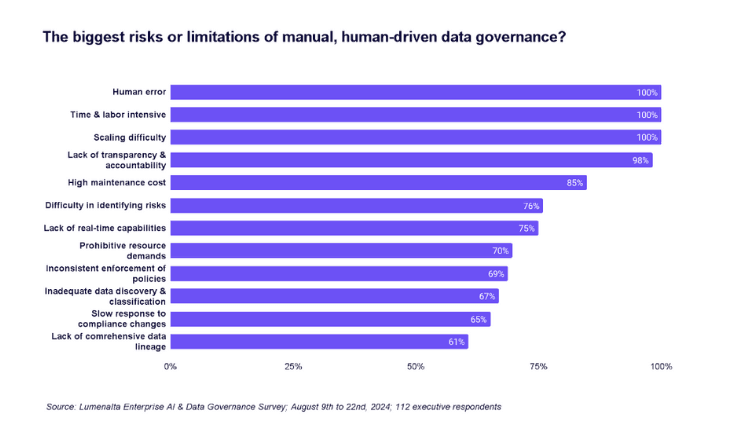
Steps to create a sustainable data governance framework
To build a data governance framework that adapts and grows with your organization, consider these expanded steps:
1. Define the objectives and scope
Intro: Start by clarifying the primary goals of your data governance framework. Knowing what you want to achieve will help you align your strategy with your business's broader objectives.
Action items:
- Identify the data assets that require governance.
- Define specific outcomes, such as data quality improvements or enhanced compliance.
- Establish clear success metrics.
Example: A financial services company may focus its objectives on achieving strict compliance with data protection laws like GDPR and reducing data-related risks.
2. Assemble a cross-functional data governance team
Intro: Effective data governance requires collaboration across departments to ensure that every stakeholder's needs are considered.
Action items:
- Include representatives from IT, data management, legal, compliance, and key business units.
- Assign roles like data stewards to manage data integrity.
- Set up a data governance council to oversee framework execution.
Example: Cross-functional teams help ensure that data governance aligns with both technical capabilities and business needs, avoiding silos and miscommunication.
3. Develop comprehensive data governance policies and standards
Intro: Policies and standards form the backbone of your data governance framework.
Action items:
- Draft policies for data quality, data privacy, and compliance.
- Regularly update these standards to align with evolving industry regulations.
- Ensure all employees are trained on these policies.
Example: Regular policy reviews can help companies in healthcare stay compliant with regulations like HIPAA while also managing sensitive patient data securely.
4. Invest in data management tools and technologies
Intro: Leveraging the right tools is critical for maintaining data governance at scale.
Action items:
- Implement tools for data cataloging, metadata management, and compliance tracking.
- Use AI-powered technologies to automate repetitive tasks and improve data accuracy.
Example: AI-based data quality tools can continuously monitor datasets for inconsistencies, ensuring data integrity in real-time applications.
By following these steps and adapting them to your organization's specific needs, you lay a strong foundation for a data governance framework that drives both immediate value and long-term resilience.
"Robust metadata management is the backbone of any data governance framework, ensuring data transparency and empowering stakeholders to make informed decisions based on reliable information."
Challenges in implementing a data governance framework
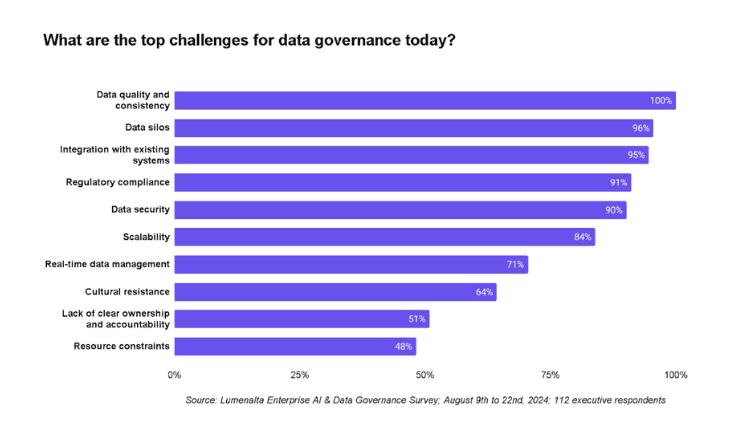
Data governance frameworks come with several challenges that organizations must address to ensure successful implementation. Let’s expand on each identified challenge:
1. Data quality and consistency
Maintaining high data quality is one of the most significant challenges organizations face. Inconsistent data can lead to poor decision-making, unreliable analytics, and compliance issues, which can ultimately impact the organization's credibility. Effective data governance requires ongoing data cleansing processes, validation techniques, and consistent monitoring to ensure data remains accurate and up-to-date.
2. Integration with existing systems
Data governance frameworks must seamlessly integrate with existing IT infrastructure and data management systems. Organizations often face compatibility issues when trying to align new governance protocols with legacy systems. To overcome this, companies should invest in flexible data integration tools that can bridge gaps between old and new technologies, ensuring a cohesive data ecosystem that supports strategic decision-making.
3. Scalability
As organizations grow, their data needs expand exponentially, making scalability a significant concern in data governance. Managing increasing data volumes without compromising quality or speed requires scalable governance solutions that adapt to evolving business requirements. Implementing scalable AI-driven tools can help automate repetitive tasks, optimize data management, and handle larger datasets efficiently.
4. Resource constraints
Limited resources and budget can often hamper the ability to implement a comprehensive data governance framework. Small and mid-sized enterprises may struggle to allocate sufficient funds for data management technologies and training. A strategic approach is to prioritize high-impact areas within data governance and gradually expand as more resources become available, ensuring that essential governance activities are never compromised.
Addressing these challenges requires a proactive approach, continuous optimization, and the integration of advanced technologies like AI to enhance scalability and data quality management, ensuring a resilient and effective data governance framework.
AI and data governance: An evolving landscape
AI is transforming how organizations approach data governance by automating processes, enhancing data quality, and ensuring compliance with evolving regulations. Below, we explore the key areas where AI plays a crucial role in modern data governance.
The role of AI in data governance frameworks
AI is playing an increasingly important role in modern data governance frameworks by automating tasks like data classification, anomaly detection, and regulatory compliance monitoring. By leveraging machine learning algorithms, AI can identify patterns and discrepancies within datasets that may go unnoticed by traditional methods. This proactive approach reduces errors and ensures that data remains accurate and compliant with industry standards.
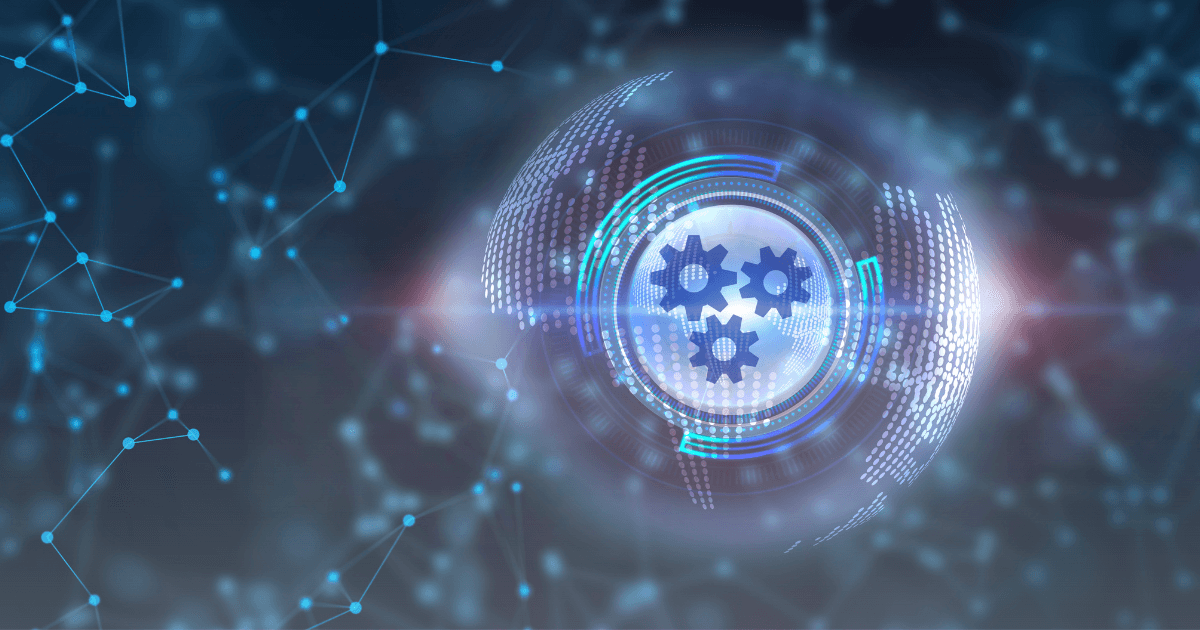
Integrating AI in data governance for long-term success
To leverage AI effectively, organizations must ensure that their data governance framework is adaptable and can accommodate emerging AI technologies. This means creating a flexible structure that allows for the integration of AI-driven tools, enabling faster response times to regulatory changes and enhancing overall data management efficiency. AI integration also helps streamline data processes, reducing the manual effort required to maintain high data standards.
AI-powered compliance monitoring
AI technologies can continuously monitor data against regulatory requirements, ensuring that compliance is managed effectively. Automated compliance monitoring reduces the risk of human error and ensures that any deviations from regulatory norms are promptly flagged and corrected. This capability is particularly valuable for industries with stringent data protection laws, like finance and healthcare, where maintaining compliance is crucial for business continuity.
Enhanced data quality management
AI tools provide real-time analytics and data quality checks, making it easier to maintain clean, accurate datasets for business intelligence. Machine learning algorithms can automatically detect anomalies, fill data gaps, and suggest corrections, which enhances the reliability of data for decision-making. By continuously optimizing data quality, organizations can derive more meaningful insights and make data-driven decisions with greater confidence.
As AI continues to evolve, its role in data governance will expand, enabling organizations to manage their data assets more effectively, scale their operations, and remain competitive in the digital landscape.
Measuring the success of your data governance framework
To ensure that your data governance framework delivers value, it's essential to track performance using key performance indicators (KPIs). Robust ROI measurement helps businesses align data strategies with organizational goals and provides tangible evidence to justify ongoing investments.
Key metrics for measuring the success of AI-driven data governance include:
- Data quality metrics: Assess improvements in accuracy, completeness, and consistency of data. According to research, 100% of organizations track these metrics as part of their governance efforts.
- Compliance rate: Monitor adherence to data privacy regulations like GDPR and CCPA, ensuring the organization stays compliant and reduces legal risks. 84% of organizations report improved compliance tracking through AI-powered governance tools.
- Cost savings: Measure reductions in data-related errors, manual processes, and operational inefficiencies. 92% of organizations track cost savings as a direct benefit of AI-driven governance.
- Efficiency gains: Evaluate the decrease in time spent on manual data management tasks by using automation and AI tools to streamline processes.
- Scalability: Track the ability to handle growing data volumes efficiently without compromising data quality. 88% of organizations reported improved scalability after implementing AI-enhanced governance systems.
By regularly measuring these KPIs, businesses can align their governance initiatives with strategic goals and ensure they deliver measurable outcomes. Tracking success through meaningful metrics provides a clear view of the ROI, enabling continuous improvement and building a strong case for further investment in AI-driven data governance.
Creating a data governance framework that lasts requires a strategic approach, commitment to data quality, and the integration of advanced technologies like AI. By building a robust framework that evolves with your business needs, you can ensure that your data remains a valuable asset, driving informed decisions and delivering measurable business outcomes.
For businesses ready to take their data governance to the next level, Lumenalta offers tailored solutions that align with your unique data needs, empowering your digital transformation journey.
Table of contents
- Introduction to data governance
- What is a data governance framework?
- Key components of a data governance framework
- Benefits of data governance frameworks
- Steps to create a sustainable data governance framework
- Challenges in implementing a data governance framework
- AI and data governance: an evolving landscape
- Measuring the success of your data governance framework
- Common questions about data governance frameworks
Common questions about data governance frameworks
What are the essential components of a data governance framework?
How does AI improve data governance frameworks?
Why is metadata management important in a data governance strategy?
How do scalable data governance solutions handle increasing data volumes?
What are the key benefits of a robust data governance framework?
Want to learn how data governance can bring more transparency and trust to your operations?