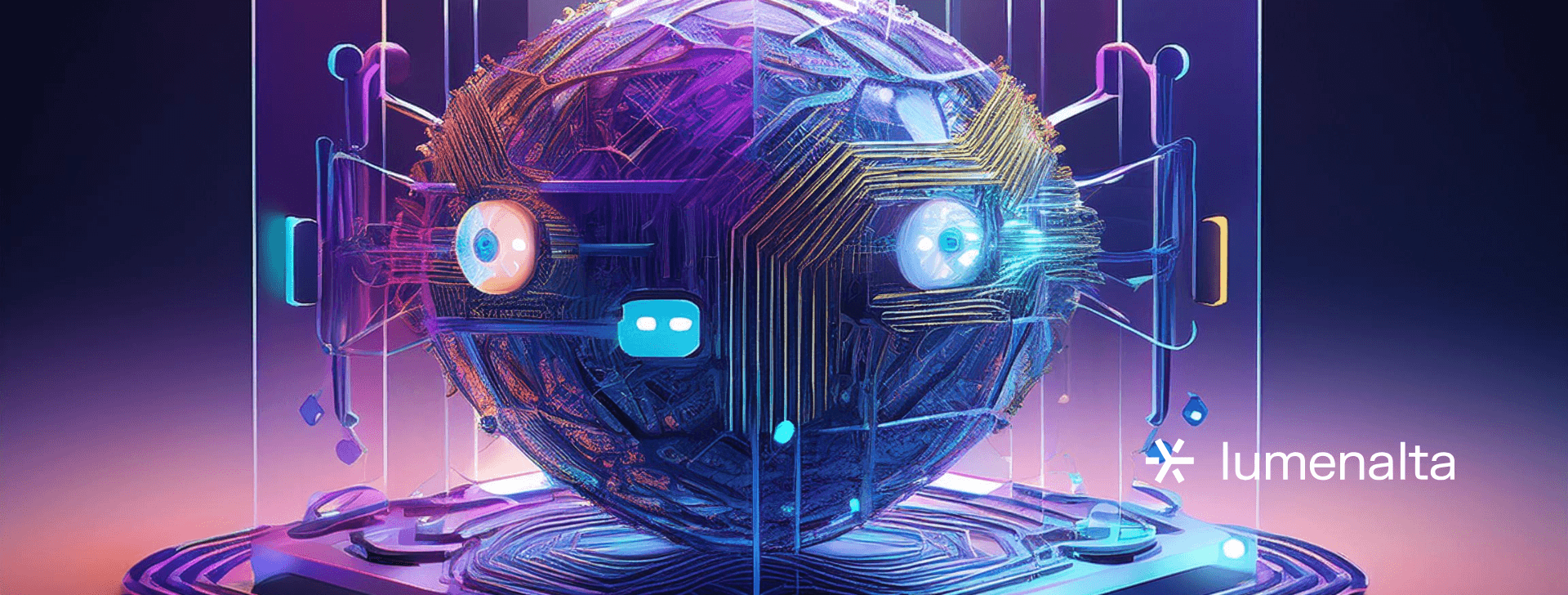
7 benefits of machine learning in cybersecurity
DEC. 21, 2024
6 Min Read
Organizations face an escalating challenge to secure their systems and data as cyber threats become more sophisticated and persistent.
Machine learning is transforming cybersecurity by enabling faster, smarter, and more adaptive defense strategies that mitigate risks and protect critical infrastructure. Its ability to analyze vast amounts of data in real time and predict potential threats before they occur makes it a pivotal tool in safeguarding digital settings.
Key takeaways
- 1. Machine learning identifies and mitigates cyber threats in real time, ensuring faster detection and prevention.
- 2. Automated processes powered by machine learning improve security efficiency and reduce operational costs.
- 3. Adaptive defense mechanisms allow systems to develop and address emerging threats with precision.
- 4. Advanced authentication methods enhance identity security by leveraging behavioral analysis and biometrics.
- 5. Large-scale data analysis through machine learning ensures compliance and robust protection for sensitive systems.
What is machine learning in cybersecurity?
Machine learning in cybersecurity represents a transformative approach to managing and mitigating threats. At its core, machine learning involves the use of algorithms that allow systems to learn from data, identify patterns, and make informed decisions without explicit programming. In cybersecurity, this capability plays a vital role in proactively identifying vulnerabilities, detecting anomalies, and responding to potential threats faster than traditional methods.
Machine learning empowers cybersecurity systems to adapt to new and emerging threats by analyzing vast quantities of data in real time. Unlike conventional rule-based approaches that depend on predefined instructions, machine learning continuously refines its understanding of what constitutes malicious activity by studying patterns from historical data and live network interactions. This adaptability ensures that cybersecurity measures remain robust even as attack vectors advance.
The integration of machine learning into cybersecurity doesn't just improve detection accuracy—it enables a proactive defense strategy. Systems equipped with machine learning can recognize subtle signs of cyberattacks before they fully materialize, helping organizations avoid significant breaches, reduce response times, and maintain business continuity in the face of persistent threats. This makes machine learning a critical tool in the arsenal for protecting sensitive data and maintaining operational security.
"Machine learning empowers cybersecurity systems to adapt to new and emerging threats by analyzing vast quantities of data in real time."
7 benefits of machine learning in cybersecurity
The integration of machine learning into cybersecurity has shifted the way organizations approach digital defense. Cyberattacks are no longer simple or predictable, requiring solutions that go beyond traditional tools. Machine learning addresses this challenge with unmatched capabilities in pattern recognition, adaptability, and real-time response. Its ability to process vast amounts of data and identify subtle indicators of threats makes it indispensable. Organizations relying on static, rule-based systems often struggle to keep up with changing attack strategies. Machine learning fills this gap, offering a dynamic and intelligent defense that minimizes risk while ensuring operational continuity.
1. Enhanced threat detection and prevention
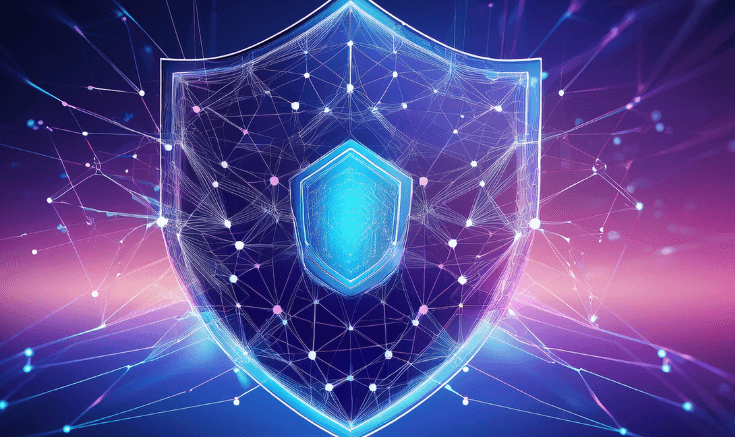
Machine learning excels at identifying complex and cyber threats. Traditional detection systems often rely on predefined rules, making them ineffective against new or sophisticated attacks. Machine learning, on the other hand, analyzes historical and real-time data to detect subtle patterns that signal malicious activity. This proactive approach minimizes the risk of attackers exploiting unknown vulnerabilities.
For example, machine learning algorithms can differentiate between legitimate user behavior and anomalous activity, even when the differences are minute. These systems constantly refine their models, ensuring they remain effective against updated tactics like phishing or advanced persistent threats. The measurable impact is clear: organizations can prevent breaches before they occur, reducing downtime and financial losses associated with reactive measures.
2. Faster data analysis and response times
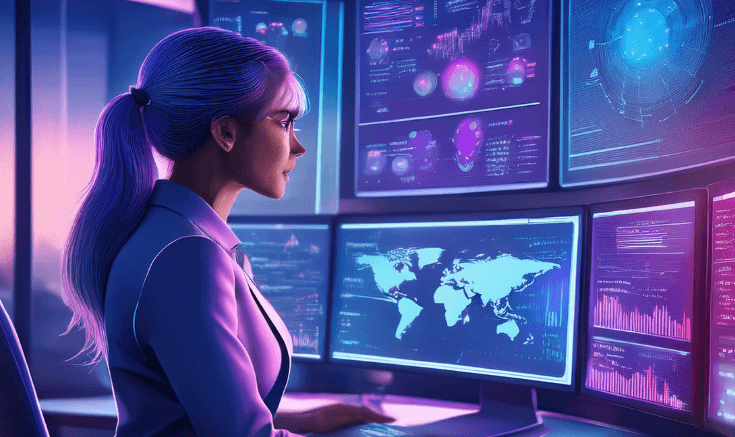
Cybersecurity demands real-time responsiveness, especially as threats become more aggressive. Machine learning accelerates data analysis by processing millions of logs, network events, and user activities within seconds. This capability enables teams to identify and address vulnerabilities faster than ever before.
For instance, instead of manually sifting through massive amounts of data, machine learning tools can provide instant insights into high-priority threats. This automation empowers security teams to respond to incidents as they unfold, limiting their impact. Faster response times lead to better outcomes, ensuring critical systems remain operational even during an attempted breach.
3. Automation of cybersecurity processes
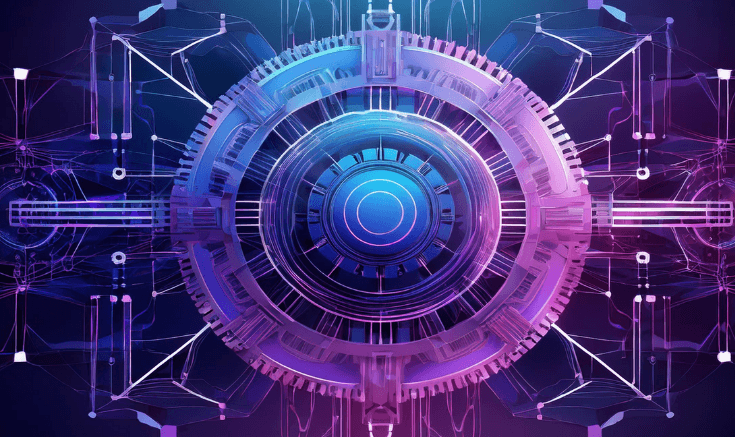
Manually managing cybersecurity operations is both time-consuming and resource-intensive. Machine learning automates critical tasks like vulnerability assessments, malware detection, and threat intelligence gathering. This automation frees up security professionals to focus on strategic initiatives rather than repetitive, low-value tasks.
An example is the automation of phishing email detection. Machine learning models can analyze email content, sender behavior, and recipient interactions to flag potential phishing attempts automatically. This approach reduces the risk of human oversight while maintaining high levels of protection. The result is a more efficient and cost-effective cybersecurity operation.
4. Improved accuracy and reduced human errors
Human errors in cybersecurity can result in devastating breaches. Regardless of oversight or fatigue, mistakes can leave systems vulnerable. Machine learning improves accuracy by removing the subjectivity and inconsistency inherent in manual processes. These algorithms detect threats that might be missed by even the most vigilant human analyst.
Additionally, the ability of machine learning to process vast datasets ensures comprehensive coverage. It identifies correlations that would take human teams weeks or months to uncover. By minimizing reliance on manual processes, organizations can build a more reliable security posture that safeguards against inadvertent mistakes.
5. Adaptive and proactive defense systems
Static cybersecurity solutions struggle to keep up with the fluid nature of cyber threats. Machine learning introduces adaptability, enabling defense systems to develop in real time. These systems not only respond to existing threats but also predict potential attack vectors based on current trends and behaviors.
This adaptability is particularly effective against zero-day vulnerabilities—attacks that exploit previously unknown weaknesses. Machine learning detects anomalies and initiates preventative measures, protecting systems before attackers can gain a foothold. The result is a proactive defense strategy that minimizes exposure to new risks and ensures long-term security.
6. Advanced authentication mechanisms
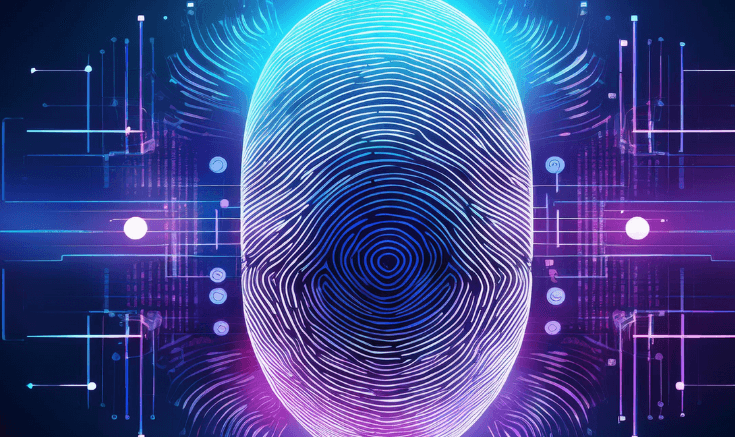
Securing user identities is one of the cornerstones of modern cybersecurity. Machine learning enhances authentication by analyzing biometric data, user behavior, and access patterns. Unlike traditional password systems, these mechanisms are difficult for attackers to bypass or replicate.
For example, systems that use machine learning for behavioral analysis can detect subtle irregularities in typing speed, mouse movements, or even mobile device usage. If an attacker attempts to mimic a legitimate user, the system flags the activity as suspicious. This layered approach strengthens user authentication, reducing the likelihood of unauthorized access.
7. Efficient handling of large-scale data
As organizations grow, so does the volume of data they generate. Managing and securing this data at scale presents significant challenges. Machine learning performs best in settings with vast amounts of information, processing large-scale data efficiently and accurately.
Capabilities such as these are especially critical in industries like finance and healthcare, where compliance with strict data security regulations is mandatory. Machine learning tools provide actionable insights from massive datasets, ensuring businesses remain compliant while identifying potential security gaps. The efficiency gained from these tools translates to improved operational performance and reduced costs.
This innovative technology empowers businesses to take control of their cybersecurity, turning potential vulnerabilities into opportunities for stronger defenses. The benefits of machine learning extend beyond immediate protection; they establish a foundation for future-proofing cybersecurity strategies. As cyber threats grow in complexity, the role of machine learning becomes even more vital, providing the precision and scalability needed to safeguard sensitive systems and data. Organizations leveraging this technology are better positioned to stay ahead of attackers, secure critical assets, and build trust with stakeholders, ensuring long-term resilience.
"Adaptive and proactive defense systems equipped with machine learning detect anomalies and initiate preventative measures, protecting systems before attackers can gain a foothold."
Strategies for implementing machine learning in cybersecurity
Successful integration of machine learning into cybersecurity requires a structured and strategic approach tailored to an organization’s unique needs and challenges. A clear understanding of existing vulnerabilities and operational goals is essential before adopting any machine learning tools. Organizations should begin by identifying areas where traditional methods fall short, such as detecting advanced threats or managing vast amounts of data in real time. This foundational assessment helps guide the selection of machine learning models that align with specific cybersecurity objectives.
Once foundational requirements are established, collaboration between cybersecurity experts and data scientists ensures effective implementation. These professionals must train machine learning models using high-quality, representative datasets. The success of machine learning in cybersecurity heavily relies on the accuracy and diversity of data used during training. Organizations should regularly update datasets to include new threat signatures and behavioral patterns, ensuring the system remains adaptable to attack vectors. Additionally, employing robust validation techniques is critical to avoid overfitting, which can hinder real-world performance.
Integration with existing systems is another key consideration. Machine learning tools should complement current cybersecurity infrastructure rather than replace it entirely. Seamless interoperability allows organizations to maximize the value of both traditional security methods and advanced machine learning capabilities. Clear governance policies should guide the deployment of machine learning, including periodic evaluations to measure effectiveness and identify improvement areas. Investments in user education further ensure that security teams are equipped to interpret outputs and make informed decisions, fostering a cohesive and proactive cybersecurity strategy.
Implementing machine learning in cybersecurity is not a one-time effort but an ongoing process of refinement. Regular updates, continuous monitoring, and adaptive strategies are vital for maintaining effectiveness in the face of shifting cyber threats. Organizations that commit to this iterative process position themselves for long-term success, leveraging machine learning to build a more resilient and future-ready cybersecurity framework.
Measuring success of machine learning in cybersecurity
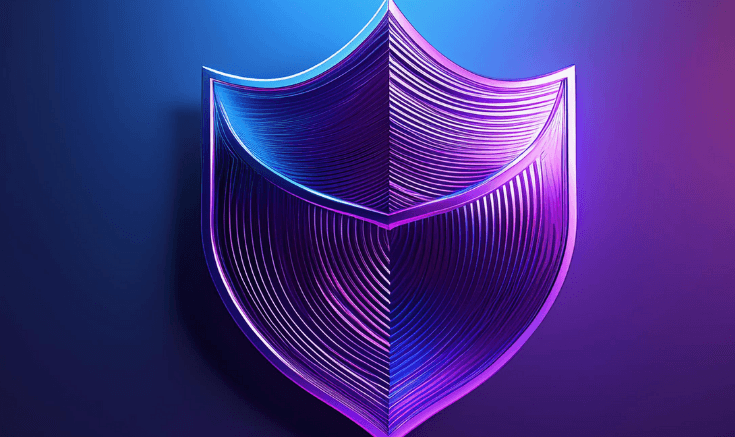
Measuring the impact of machine learning in cybersecurity requires organizations to focus on key performance indicators that reflect both short-term effectiveness and long-term resilience. While machine learning provides cutting-edge capabilities, its value must be quantifiable to ensure ongoing investment and optimization. Tracking metrics such as detection accuracy, response time, and operational efficiency allows businesses to assess the real-world performance of their cybersecurity solutions. Establishing a robust framework for measurement ensures that machine learning delivers tangible improvements to threat management and protection strategies.
Detection accuracy and false positive rates
Detection accuracy is one of the most critical metrics for evaluating the effectiveness of machine learning models in cybersecurity. Algorithms must identify and categorize threats with precision while minimizing false positives that could disrupt operations. Consistent monitoring of accuracy rates enables organizations to identify weaknesses in their models and refine them accordingly. Systems that maintain high accuracy over time not only bolster defenses but also reduce the workload for human security teams, ensuring resources are allocated to real threats rather than unnecessary investigations.
Response time and incident resolution
Speed in identifying and responding to threats is essential for mitigating damage during an attack. Machine learning's ability to process vast amounts of data in real time is a key factor in reducing response times. Metrics such as time-to-detection and time-to-resolution are useful benchmarks for measuring success. These indicators help organizations understand how quickly their systems react to anomalies and how effectively they mitigate the impact of an incident. Faster response times contribute directly to minimizing downtime and safeguarding critical systems.
Operational efficiency and cost savings
Operational efficiency is a measurable outcome that reflects how machine learning improves overall cybersecurity processes. Metrics such as automated task completion rates and resource utilization highlight the extent to which machine learning has reduced reliance on manual interventions. These efficiencies often translate to significant cost savings, as fewer human resources are needed for routine tasks. Tracking financial metrics alongside performance indicators provides a comprehensive view of the return on investment from machine learning adoption in cybersecurity.
Machine learning’s success in cybersecurity lies in its ability to deliver measurable improvements across critical areas. A clear framework for tracking detection accuracy, response times, and operational efficiency ensures that organizations can optimize their solutions while demonstrating value to stakeholders. As cyber threats grow in complexity, the ability to quantify and enhance machine learning performance will remain essential for maintaining a secure and resilient digital infrastructure.
Machine learning brings unparalleled precision and adaptability to cybersecurity, empowering businesses to stay ahead of threats. With solutions that enhance security and scalability, it’s not just a defense mechanism—it’s a pathway to resilient operations. At Lumenalta, we develop tailored cybersecurity solutions that integrate advanced machine learning, aligning with your goals for a safer and smarter future.
Let’s build a stronger defense together.
table-of-contents
- What is machine learning in cybersecurity?
- 7 benefits of machine learning in cybersecurity
- 1. Enhanced threat detection and prevention
- 2. Faster data analysis and response times
- 3. Automation of cybersecurity processes
- 4. Improved accuracy and reduced human errors
- 5. Adaptive and proactive defense systems
- 6. Advanced authentication mechanisms
- 7. Efficient handling of large-scale data
- Strategies for implementing machine learning in cybersecurity
- Measuring success of machine learning in cybersecurity
- Common questions about machine learning in cybersecurity
Common questions about machine learning in cybersecurity
What is the role of machine learning in cybersecurity?
How does machine learning reduce cybersecurity risks?
Can small businesses benefit from machine learning in cybersecurity?
What are examples of machine learning in cybersecurity?
Is machine learning alone enough to secure systems?
Want to learn how machine learning can bring more transparency and trust to your operations?