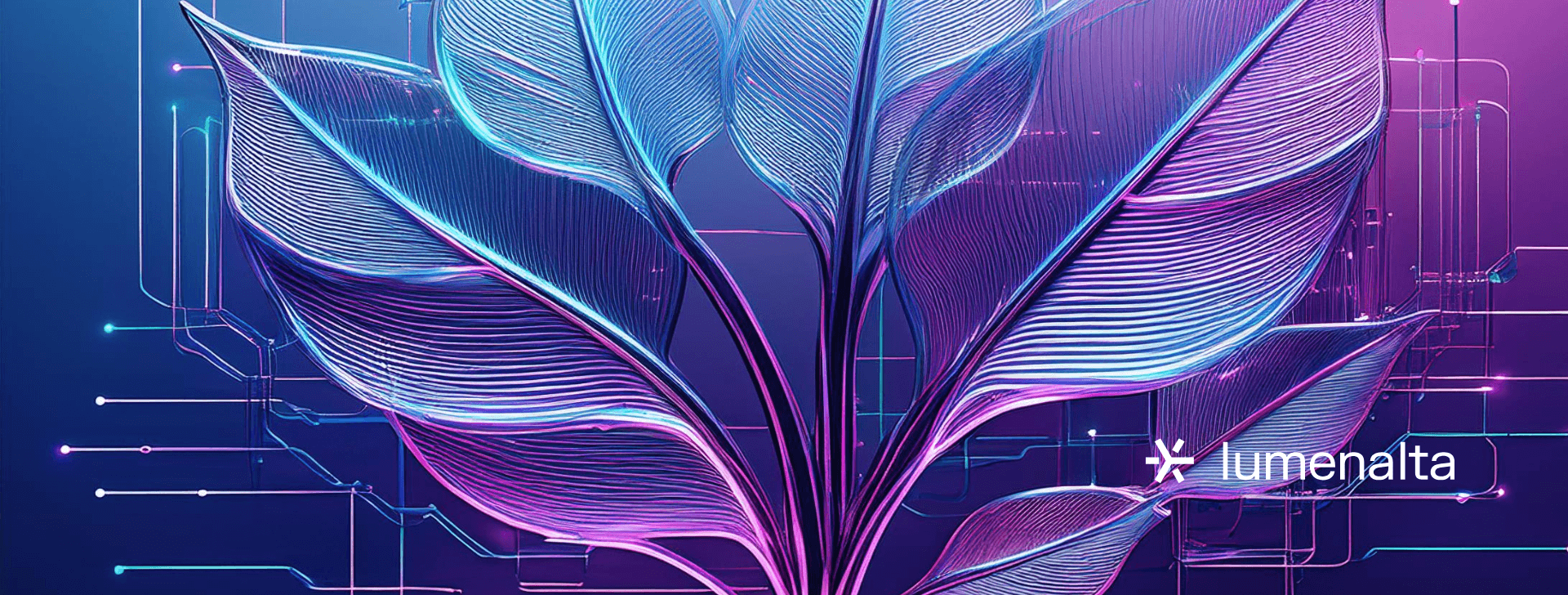
11 benefits of machine learning in agriculture
JAN. 4, 2025
6 Min Read
Agriculture is undergoing a profound modification with the adoption of machine learning, a powerful technology driving more precise and sustainable practices.
From optimizing resource use to facilitating data smart decisions, machine learning equips farmers and agribusinesses to meet the growing global need for food while minimizing environmental impact. This innovation is reshaping the future of farming by solving critical challenges and unlocking new opportunities.
Key takeaways
- 1. Machine learning revolutionizes agriculture by driving precision, efficiency, and sustainability.
- 2. Farmers can achieve better crop yields and resource optimization with data insights.
- 3. Advanced technologies detect diseases, manage weeds, and improve soil conditions.
- 4. Automated harvesting and price forecasting enhance scalability and profitability.
- 5. Adopting machine learning sustains agricultural operations for developing challenges.
What is machine learning in agriculture?
Machine learning is a branch of artificial intelligence focused on using data and algorithms to simulate human evaluation. In agriculture, this technology has significantly changed traditional farming by automating tasks, improving efficiency, and driving actionable insights from vast datasets. Farmers and agribusinesses can use machine learning to analyze environmental conditions, monitor crop health, and optimize resource use, all in real time.
The integration of machine learning in agriculture enhances operational scalability and helps mitigate challenges like unpredictable weather patterns, pest outbreaks, and fluctuating market demands. Using predictive models and advanced analytics lets machine learning empower stakeholders to make precise decisions, ensuring sustainability and profitability.
“Machine learning enhances crop yield predictions by analyzing vast datasets, including historical yield records, weather patterns, and soil conditions.”
11 benefits of machine learning in agriculture
Machine learning offers possibilities in agriculture by authorizing more efficient and scalable farming practices. Utilizing this technology ensures better outcomes for farmers, agribusinesses, and consumers by addressing critical challenges across the production lifecycle. From enhancing yield prediction to optimizing irrigation strategies, adopting machine learning in agriculture marks a significant leap toward sustainable and cost-effective solutions.
1. Improved crop yield prediction
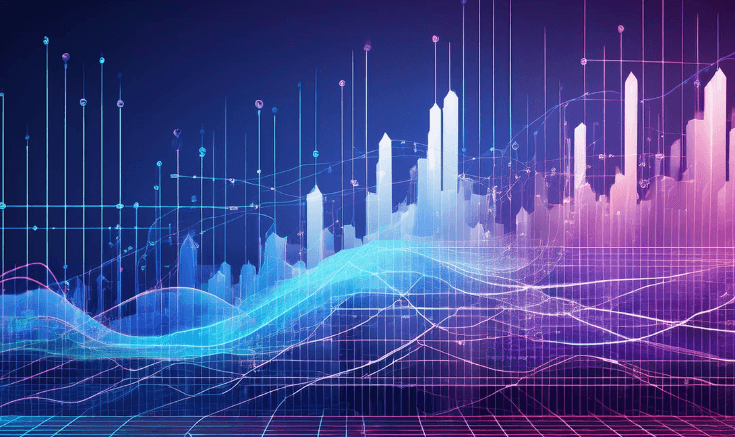
Accurately predicting crop yields is essential for meeting food production demands while minimizing waste. Machine learning enhances crop yield predictions by analyzing vast datasets, including historical yield records, weather patterns, and soil conditions. These algorithms identify patterns and correlations that would be impossible to detect manually, helping farmers anticipate harvest outcomes more effectively.
For example, predictive models can combine satellite imagery with localized data to forecast yield quantities across different regions. This insight allows farmers to allocate resources, such as labor and equipment, more effectively and to plan for potential supply chain fluctuations. With reliable crop yield predictions, producers can reduce uncertainty, stabilize pricing strategies, and optimize distribution networks to prevent food waste.
2. Enhanced disease and pest detection
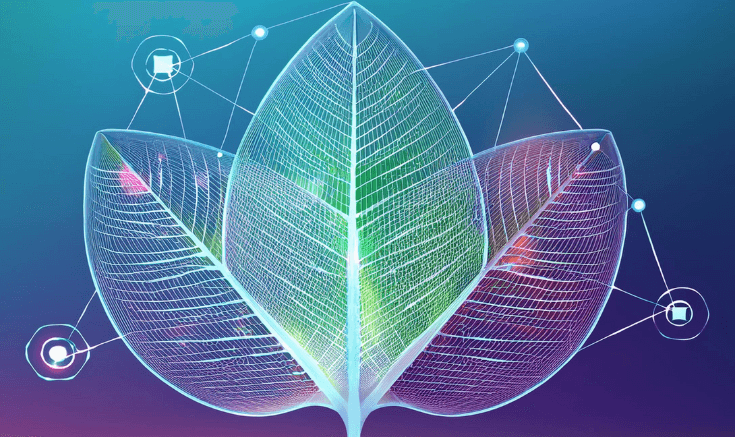
The early detection of crop diseases and pest infestations can significantly reduce losses and improve overall farm productivity. Machine learning systems analyze images captured by drones, satellites, or ground-level cameras to identify disease or pest activity signs. Advanced algorithms can distinguish between healthy and infected plants, often detecting issues long before they become visible to the human eye.
These systems also learn to recognize patterns in environmental conditions that correlate with outbreaks, allowing farmers to implement preventive measures. This proactive approach minimizes the need for excessive pesticide use, lowers operational costs, and ensures healthier crop production.
3. Efficient weed management
Weeds compete with crops for vital nutrients, water, and sunlight, often reducing yield. Traditional weed control methods, such as manual removal or blanket spraying of herbicides, can be labor-intensive, costly, and environmentally damaging. Machine learning offers precision weed management solutions by identifying and targeting specific weed species within fields.
Sophisticated algorithms allow autonomous machinery, such as smart sprayers, to apply herbicides only to areas where weeds are present. This targeted approach reduces chemical usage, cuts expenses, and minimizes environmental impact. Efficient weed management improves overall crop health, leading to higher productivity and better farmer returns.
4. Accurate crop recognition
Understanding which crops are growing in different areas is vital for large-scale agricultural planning, particularly in regions with varied farming practices. Machine learning systems analyze satellite and drone imagery to classify crops based on unique visual and spectral signatures. These technologies improve the accuracy of crop monitoring, even in mixed or overlapping planting areas.
Accurate crop recognition helps governments, researchers, and agribusinesses assess land-use patterns, forecast food supply levels, and identify opportunities for crop diversification. This capability also supports compliance with agricultural policies and trade agreements, ensuring seamless operations within the global market.
5. Optimized irrigation and water management
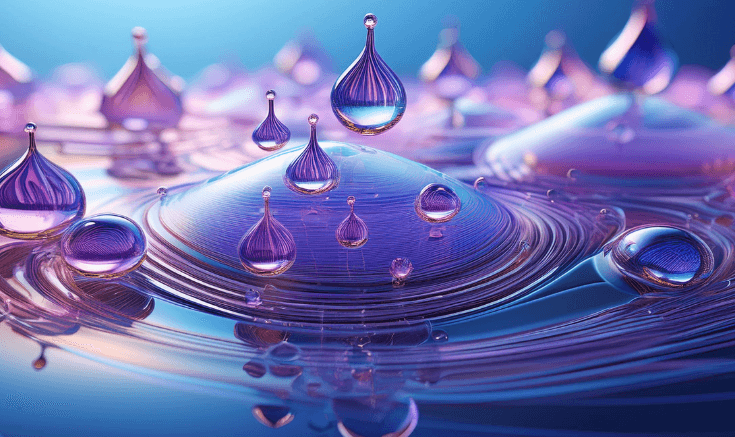
Water is one of agriculture’s most critical yet limited resources. Machine learning helps irrigation practices by analyzing weather data, soil moisture levels, and crop water requirements to recommend precise watering schedules. Automated irrigation systems integrated with machine learning algorithms adjust water distribution dynamically, reducing waste and ensuring optimal hydration for crops.
In regions prone to drought or water scarcity, these technologies are invaluable for sustaining production while conserving resources. Farmers benefit from reduced water bills, healthier crops, and improved long-term soil quality, ensuring sustainable agricultural practices for future generations.
“Automated irrigation systems integrated with machine learning algorithms adjust water distribution dynamically, reducing waste and ensuring optimal hydration for crops.”
6. Precision fertilizer and pesticide application
Applying fertilizers and pesticides uniformly across fields often leads to inefficiencies and environmental harm. Machine learning introduces precision agriculture techniques that identify specific zones within a field requiring intervention. These systems use real-time data from sensors, drones, and satellite imagery to assess soil nutrient levels, plant health, and pest activity, ensuring targeted application.
The precision application reduces the number of chemicals needed, lowering costs for farmers and minimizing runoff that harms surrounding ecosystems. Crops benefit from the required nutrients, improving growth rates and yield quality. Over time, this approach supports soil health, creating a sustainable foundation for future planting cycles.
7. Advanced weather monitoring and forecasting
Unpredictable weather patterns are a significant challenge for farmers. Machine learning enhances weather forecasting by analyzing massive datasets from meteorological sources, satellite imagery, and local climate sensors. These predictive models deliver highly accurate, localized forecasts, helping farmers plan critical activities such as planting, irrigation, and harvesting.
For example, if a forecast predicts excessive rainfall, farmers can adjust drainage systems or delay irrigation schedules to avoid waterlogging. Similarly, anticipating frost or heatwaves allows proactive measures to protect crops. Advanced weather monitoring ensures the timely making of decisions, reduces risks, and maximizes productivity under adjusting environmental conditions.
8. Automated harvesting
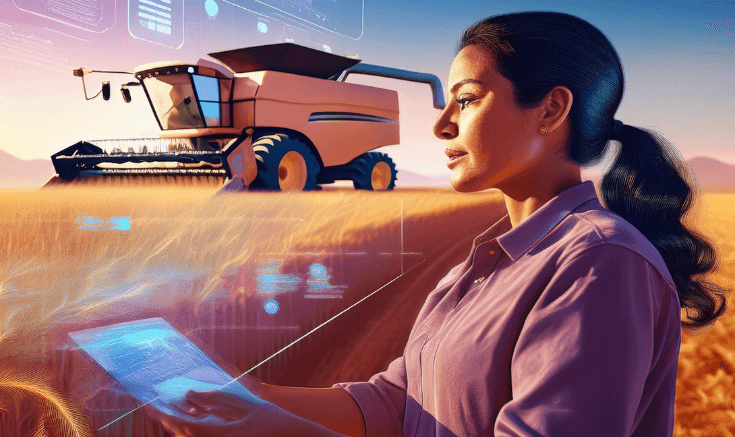
Labor shortages and high operational costs make manual harvesting unsustainable. Machine learning powers automated harvesting systems using advanced sensors and robotics to identify and pick ripe crops efficiently. These systems differentiate between crops and unwanted materials, ensuring clean harvests with minimal human intervention.
Automated harvesting increases speed and precision, reducing waste and damage to produce. Farmers can maintain consistent harvest quality while scaling operations to meet growing need. Integrating machine learning allows this technology to also adapt to different crop types and field conditions, offering flexibility and long-term scalability.
9. Livestock health and welfare monitoring
Maintaining animal health and welfare for livestock farming is critical for productivity and ethical practices. Machine learning applications analyze data from wearable sensors, cameras, and environmental monitors to track livestock health metrics, such as body temperature, activity levels, and feeding patterns.
Farmers receive alerts to intervene early when anomalies are detected, such as reduced activity or irregular eating habits. These insights prevent disease outbreaks, reduce mortality rates, and optimize feed efficiency, improving livestock productivity and profitability. Enhanced animal welfare also supports compliance with regulatory standards and consumer expectations for ethical farming.
10. Soil condition optimization
Soil health directly impacts crop yields, yet traditional soil management practices often lack precision. Machine learning systems use data from field sensors, drones, and satellite imagery to assess soil composition, pH levels, and nutrient availability. These insights help farmers determine the most effective crop rotation schedules, fertilizer blends, and soil amendments.
Optimizing soil conditions helps farmers to prevent over-farming, reduce erosion, and increase crop productivity. Healthy soils also contribute to long-term sustainability, ensuring that agricultural land remains fertile for future generations. Machine learning permits a proactive approach to soil management, reducing costs while boosting efficiency.
11. Price forecasting
Fluctuations in market prices for crops and livestock pose significant challenges for farmers and agribusinesses. Machine learning algorithms analyze historical market data, weather patterns, and consumer demand trends to predict price movements accurately. These forecasts let farmers decide when to sell their produce or invest in additional resources.
Price forecasting also supports contract negotiations and financial planning, reducing uncertainty in an often volatile market. Aligning production strategies with market conditions allows farmers to maximize profitability while maintaining an edge in the agricultural industry.
Machine learning’s capabilities in various areas exemplify its potential in agriculture. With data insights and precise applications, this technology addresses long-standing challenges while driving efficiency, profitability, and sustainability across farming operations.
Strategies for implementing machine learning in agriculture
Integrating machine learning into agricultural practices requires a structured approach that ensures successful adoption and measurable outcomes. Establishing clear objectives, selecting the right technology, and ensuring compatibility with existing systems are essential. Effective implementation maximizes the benefits of machine learning in agriculture while minimizing potential risks and inefficiencies.
Assessing operational needs and goals
Understanding the specific challenges faced by the farm or agribusiness is a critical starting point. Whether focusing on improving crop yields, enhancing resource efficiency, or strengthening supply chain resilience, identifying key priorities guides the selection and deployment of machine learning solutions. A detailed operational assessment helps pinpoint inefficiencies, allowing the technology to be tailored to specific requirements.
Investing in scalable data infrastructure
Data is the foundation of machine learning. Building or upgrading infrastructure to collect, store, and process data ensures seamless implementation. Farms can deploy sensors, drones, and Internet of Things (IoT) devices to gather high-quality, real-time data on soil health, weather conditions, crop status, and livestock. Investing in robust cloud platforms and analytics tools ensures scalability as operations grow.
Choosing the right machine learning tools
The selection of machine learning tools and platforms depends on the farm’s unique requirements. Open-source frameworks, custom-built algorithms, or industry-specific software solutions can be tailored to analyze data and deliver actionable insights. Collaboration with technology providers or experts ensures compatibility with current systems and the integration of advanced features like predictive modeling and automation.
Providing training for adoption
Introducing machine learning technology involves more than simply deploying new tools. Training farmers and agricultural workers builds confidence in using these systems effectively. Workshops, tutorials, and ongoing technical support ensure that users understand the technology’s capabilities and can make informed decisions based on the insights it generates.
Establishing continuous evaluation processes
Machine learning systems must adapt to conditions and objectives. Continuous monitoring and evaluation ensure that algorithms remain accurate and effective over time. Regularly reviewing performance metrics, updating datasets, and refining models enhance the system’s ability to address emerging challenges and opportunities.
Farms and agribusinesses that implement these strategies unlock the potential of machine learning and sustain their operations against growing industry demands. Combining technological innovation with practical planning creates a pathway for sustainable, scalable success in agriculture.
Business impact of machine learning in agriculture
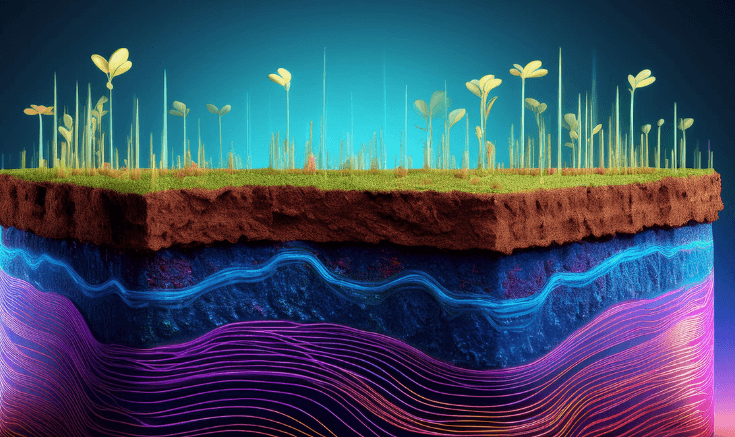
The adoption of machine learning in agriculture has redefined how businesses in the industry approach efficiency, profitability, and sustainability. Farms, agribusinesses, and stakeholders throughout the agricultural supply chain use this technology to solve complex challenges while uncovering new growth opportunities. Machine learning reduces resource waste, lowers operational costs, and delivers actionable insights that improve both short-term results and long-term planning.
The economic advantages include optimized use of inputs like water, fertilizers, and labor, directly impacting profitability. Additionally, machine learning strengthens supply chain resilience by allowing real-time monitoring of production metrics and external variables, such as weather and market trends. This empowers businesses to scale operations, meet consumer demands, and maintain dynamism in volatile markets. With its ability to bridge the gap between modern technology and traditional farming methods, machine learning is a pillar for future-ready agricultural strategies.
Machine learning is more than an innovation—it’s a tool for achieving scalable and more sustainable agricultural practices. This technology empowers farmers and agribusinesses to achieve lasting success by delivering actionable insights and precision solutions. At Lumenalta, we specialize in tailoring solutions that align with your business needs, ensuring measurable outcomes and future-ready strategies.
Let’s shape a brighter future together.
table-of-contents
- What is machine learning in agriculture?
- 11 benefits of machine learning in agriculture
- 1. Improved crop yield prediction
- 2. Enhanced disease and pest detection
- 3. Efficient weed management
- 4. Accurate crop recognition
- 5. Optimized irrigation and water management
- 6. Precision fertilizer and pesticide application
- 7. Advanced weather monitoring and forecasting
- 8. Automated harvesting
- 9. Livestock health and welfare monitoring
- 10. Soil condition optimization
- 11. Price forecasting
- Strategies for implementing machine learning in agriculture
- Business impact of machine learning in agriculture
- Common questions about machine learning in agriculture
Common questions about machine learning in agriculture
How does machine learning improve farming efficiency?
What are some examples of machine learning in agriculture?
Can small farms benefit from machine learning?
Is machine learning cost-effective for agriculture?
How does machine learning promote sustainable farming?
Want to learn how machine learning can bring more transparency and trust to your operations?