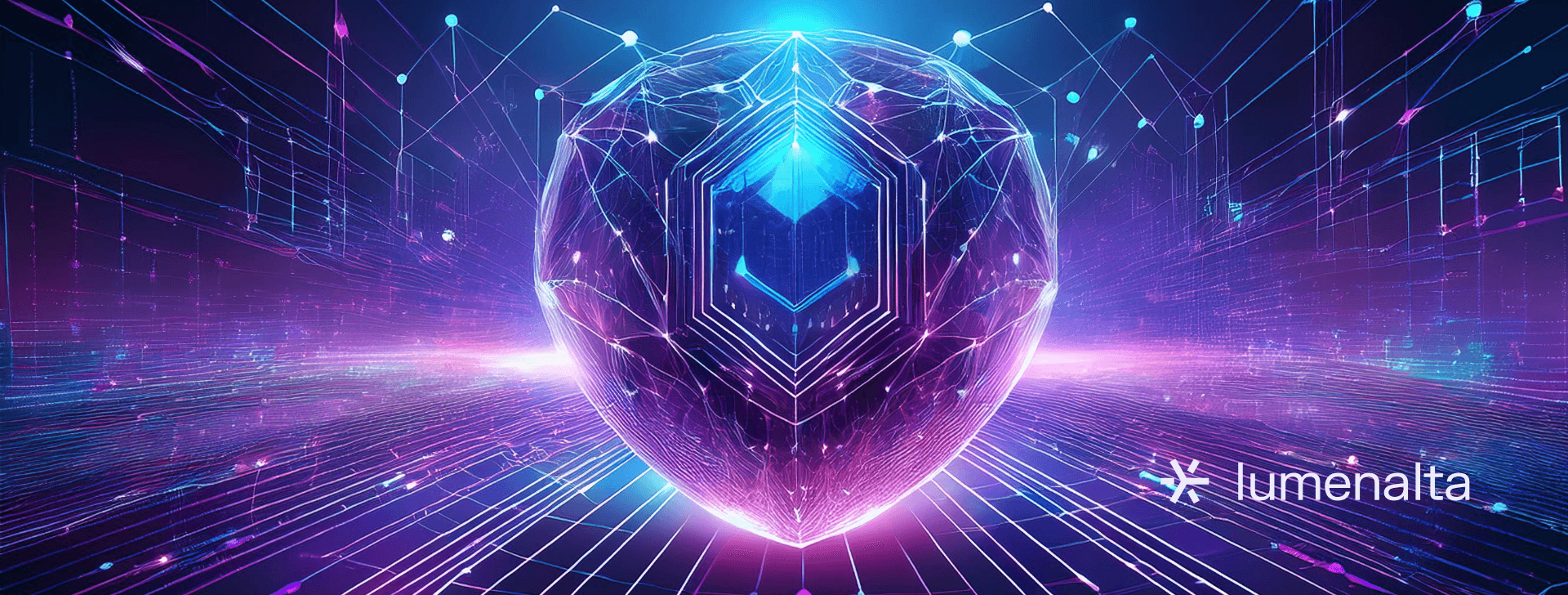
AI and cybersecurity
NOV. 30, 2024
6 Min Read
Artificial intelligence is redefining cybersecurity, enabling organizations to detect, prevent, and respond to threats with unprecedented speed and accuracy.
AI systems analyze vast datasets in real time, identifying anomalies and potential vulnerabilities that human analysts might overlook. This proactive approach is essential as cyber threats continue to evolve in complexity and frequency.
AI enhances security across multiple fronts, from threat detection and malware analysis to automated incident response. Machine learning models adapt to new attack patterns, while AI-driven tools streamline routine processes such as log analysis and vulnerability management. These capabilities free up human security professionals to focus on high-priority issues, improving both efficiency and resilience.
The integration of AI into cybersecurity is also paving the way for innovative applications like generative AI for threat simulations and automated patch management. However, it comes with challenges such as ethical concerns and the risk of adversarial AI. By addressing these issues head-on, organizations can use AI to build robust defenses
Key takeaways
- 1. AI enhances cybersecurity through real-time threat detection, automation, and predictive capabilities, making it essential for modern defense strategies.
- 2. Generative AI offers innovative applications like threat simulations and automated patching, while also posing new challenges like adversarial misuse.
- 3. AI-driven cybersecurity tools include intrusion detection systems, behavioral analytics, and automated response solutions that create a comprehensive defense.
- 4. Implementing AI effectively requires clear objectives, high-quality data, skilled personnel, ethical compliance, and seamless integration with existing systems.
- 5. Future developments in AI and cybersecurity will include predictive intelligence, quantum computing, and autonomous systems, reshaping how organizations approach security.
Understanding AI in cybersecurity
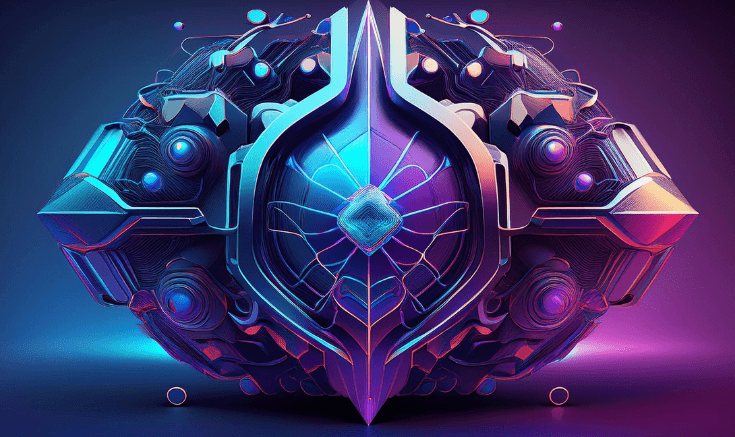
Artificial intelligence (AI) is transforming cybersecurity by enabling faster and more accurate detection of threats while automating complex defensive measures. By analyzing vast amounts of data in real time, AI can identify patterns that signify cyberattacks, allowing organizations to respond before damage occurs. This proactive approach is critical as cyber threats become more sophisticated and frequent.
AI is particularly effective in combating previously unseen threats. Through technologies like machine learning, AI adapts over time, learning from new data and improving its accuracy in identifying vulnerabilities or attack methods. This ability makes it a cornerstone for modern cybersecurity strategies across industries.
Moreover, AI enhances efficiency by automating routine tasks such as monitoring network activity, analyzing logs, and responding to lower-priority alerts. This automation not only reduces human error but also allows cybersecurity professionals to focus on high-level strategic initiatives. As organizations embrace digital transformation, leveraging AI in cybersecurity has shifted from being an advantage to a necessity.
"Artificial intelligence enables faster and more accurate detection of threats while automating complex defensive measures."
Key applications of AI in cybersecurity
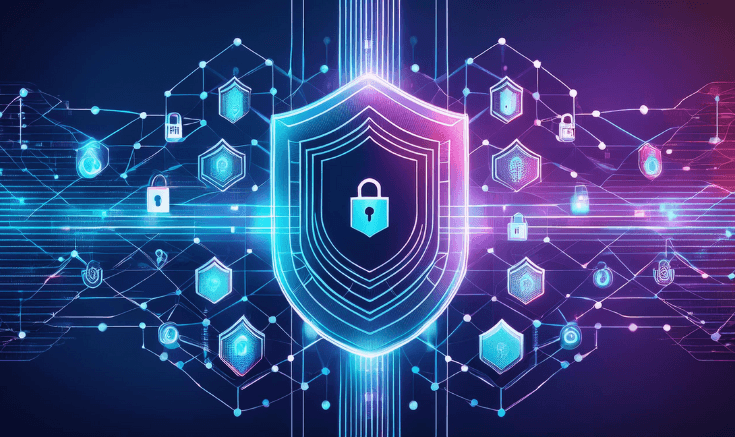
AI is redefining how organizations approach cybersecurity by offering solutions that are faster, more adaptive, and tailored to threats. Its applications span a broad spectrum, addressing challenges that traditional methods struggle to manage effectively.
Threat detection and prevention
AI excels in detecting and preventing cyber threats by analyzing network traffic patterns and identifying anomalies in real time. Machine learning models are trained on historical data to predict potential attacks, even when they mimic normal behaviors. This proactive detection minimizes damage by enabling immediate response to potential breaches.
Malware detection
Traditional malware detection often relies on signatures, making it ineffective against new or unknown variants. AI overcomes this limitation by leveraging behavioral analysis and predictive modeling. It identifies unusual activities, such as unauthorized file access, flagging potential threats without relying on existing databases. This ensures enhanced protection against zero-day exploits and polymorphic malware.
Fraud detection
AI-powered systems are invaluable in combating fraud in sectors like banking and e-commerce. By analyzing transaction patterns and user behaviors, AI can spot irregularities that signal fraudulent activities. This allows organizations to block suspicious transactions while reducing false positives, improving both security and customer experience.
Automated incident response
Responding to cyber incidents manually can be time-consuming and prone to human error. AI automates incident response processes, such as isolating affected systems, deploying patches, and restoring compromised data. This reduces downtime and ensures a faster recovery, limiting the impact of cyberattacks.
Endpoint security
As the number of connected devices grows, endpoint security becomes more challenging. AI enhances endpoint protection by monitoring device behavior, detecting unusual access patterns, and implementing adaptive defenses. This is particularly crucial in environments with a high volume of IoT devices.
By implementing AI across these applications, businesses not only bolster their defenses but also gain a strategic advantage in mitigating cybersecurity risks effectively.
Benefits and challenges of AI in cybersecurity
AI offers transformative benefits in cybersecurity, but it also introduces complexities that organizations must navigate carefully. Understanding these aspects is critical for leveraging AI effectively.
Benefits of AI in cybersecurity
- Enhanced threat detection: AI identifies threats in real time by analyzing vast datasets and detecting anomalies that traditional methods might miss. This reduces response times and prevents potential breaches.
- Proactive defense strategies: Machine learning models predict and counter emerging threats, ensuring systems are always prepared for attack methods like zero-day vulnerabilities and advanced persistent threats.
- Automation of routine tasks: AI streamlines repetitive tasks like monitoring logs and managing alerts, freeing up cybersecurity professionals to focus on more strategic issues.
- Improved accuracy: By minimizing false positives and negatives, AI reduces alert fatigue and ensures attention is directed toward genuine threats, increasing the overall efficiency of security operations.
- Scalability: AI adapts to growing network sizes and complexities, providing robust protection even as businesses expand or adopt new technologies such as IoT and cloud computing.
Challenges of AI in cybersecurity
- High implementation costs: Developing and deploying AI systems require significant investment in technology, infrastructure, and skilled personnel.
- Bias in algorithms: AI models depend on training data, and if the data is biased, it can lead to inaccurate threat detection or missed vulnerabilities.
- Adversarial attacks: Cybercriminals can exploit AI by feeding it misleading data, confusing the system, and bypassing defenses. These adversarial attacks pose a serious risk to security frameworks.
- Dependence on data quality: The effectiveness of AI in cybersecurity hinges on high-quality, diverse data. Inadequate or incomplete datasets can undermine its capabilities.
- Ethical and privacy concerns: Using AI to monitor user behavior raises questions about data privacy and the ethical implications of intrusive surveillance.
Balancing these benefits and challenges involves strategic planning, proper training, and continual updates to AI models. Organizations that effectively navigate these factors can build resilient cybersecurity systems capable of countering even the most sophisticated threats.
"Generative AI actively creates data, scenarios, or models that can simulate complex threat environments or strengthen defenses."
Generative AI in cybersecurity
Generative AI is transforming cybersecurity by enhancing how organizations identify, analyze, and respond to threats. Unlike traditional AI, which often focuses on detection and response, generative AI actively creates data, scenarios, or models that can simulate complex threat ecosystems or strengthen defenses.
One of its key applications is in creating realistic simulations for cybersecurity training and threat modeling. By generating potential attack scenarios, generative AI helps security teams prepare for a wide range of possibilities, from phishing attempts to ransomware. These simulations make it easier to anticipate attackers' strategies, improving readiness and response times.
Another significant use is in automating the creation of patches or updates to address vulnerabilities. Generative AI can analyze codebases, detect flaws, and propose fixes faster than manual methods. This accelerates the resolution of critical issues, reducing the window of exposure.
However, generative AI also has a dual-edge potential. Cybercriminals can use it to generate sophisticated phishing content, malware, or fake identities that evade traditional defenses. This underscores the need for ethical guidelines and robust countermeasures to prevent misuse.
Integrating generative AI with existing tools means organizations can achieve a deeper, more proactive cybersecurity posture, ensuring defenses evolve alongside emerging threats.
Types of AI cybersecurity tools
AI-powered tools are indispensable in modern cybersecurity, offering a diverse range of solutions tailored to specific challenges. These tools address everything from detecting anomalies to automating defensive responses, empowering organizations to safeguard their assets effectively.
- Intrusion detection systems (IDS): These AI-driven systems monitor network traffic and flag suspicious activities that indicate potential threats. By identifying unusual patterns, IDS solutions help prevent breaches before they escalate.
- Behavioral analytics platforms: These tools track user and system behaviors to identify anomalies that could signify insider threats or compromised accounts. They adapt to changes over time, offering dynamic protection.
- Endpoint protection software: AI enhances endpoint security by monitoring devices for malicious activities or unauthorized access attempts. These tools are crucial for securing a growing number of IoT devices and remote endpoints.
- Threat intelligence platforms: AI processes data from global threat databases, providing real-time insights into emerging risks. These platforms help organizations anticipate and prepare for upgraded cyberattacks.
- Automated incident response systems: Designed to reduce response times, these tools execute predefined actions like isolating affected systems or deploying patches when threats are detected, minimizing manual intervention.
- AI-powered firewalls: By analyzing data flows and behaviors, these advanced firewalls go beyond traditional rule-based systems to block sophisticated attacks that evade legacy protections.
The integration of these tools creates a layered cybersecurity strategy, where each solution complements the others. Together, they form a comprehensive defense capable of adapting to the growing complexity and scale of cyber threats.
"A thoughtful approach to these best practices builds a foundation for effective AI integration in cybersecurity."
Best practices for implementing AI in cybersecurity
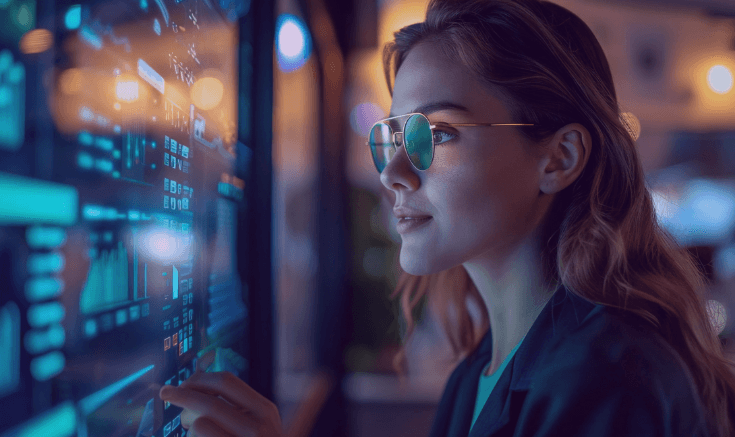
Effective implementation of AI in cybersecurity requires thoughtful planning and alignment with organizational goals. To maximize its benefits while minimizing risks, organizations must adopt a structured approach.
Define clear objectives
Establishing precise cybersecurity goals is the foundation for successful AI implementation. Organizations should begin by identifying specific vulnerabilities, such as the need for faster threat detection or reducing false positives in alert systems. Clear objectives not only guide the selection of the right AI tools but also help in measuring their success. For instance, if the goal is to improve endpoint security, organizations can focus on AI models tailored to monitor device behaviors. These objectives ensure that the deployment aligns with broader strategic needs, avoiding wasted resources on generic or mismatched solutions.
Prioritize data quality
The effectiveness of AI in cybersecurity is directly linked to the quality of the data it processes. High-quality, well-labeled datasets enable AI systems to learn and adapt accurately. In contrast, inconsistent or biased data can lead to false alarms or missed threats. Organizations should ensure that data sources are regularly updated and encompass diverse scenarios, including real-time threat simulations. Implementing data governance practices, such as anonymizing sensitive information and conducting audits, protects data integrity and enhances trust in AI outputs. Strong data management is not just a technical necessity but also a critical driver of AI’s effectiveness in securing systems.
Invest in workforce training
Adopting AI tools without adequately training the workforce can undermine their potential. Cybersecurity teams must be equipped to manage, interpret, and optimize AI solutions. Training programs should include understanding how AI models identify threats, recognizing potential biases, and learning to respond to AI-generated insights. Organizations can also foster collaboration between data scientists and security professionals, ensuring that AI tools are tailored to the organization’s specific needs. Moreover, ongoing education keeps teams updated on AI capabilities and threats, ensuring they remain adept at leveraging these technologies effectively.
Ensure integration with existing systems
AI solutions should complement and enhance current cybersecurity measures rather than disrupt them. For instance, integrating AI intrusion detection with traditional firewalls creates a layered defense that is both proactive and reactive. Compatibility is key; AI tools must seamlessly interact with legacy systems and workflows to avoid operational bottlenecks. Organizations should evaluate their existing infrastructure to identify integration challenges and ensure smooth interoperability. Properly integrated systems provide a unified security approach, where traditional and AI-enhanced tools work cohesively to address complex threats.
Address ethical and compliance concerns
Organizations must remain mindful of ethical and regulatory considerations when deploying AI. Transparency about how AI tools analyze data and make decisions is critical, especially in sensitive sectors like healthcare or finance. Compliance with data protection laws, such as GDPR or CCPA, ensures that artificial intelligence activities respect user privacy and legal standards. Ethical implementation also includes monitoring for biases within AI algorithms, which could inadvertently lead to discriminatory outcomes. Establishing clear policies on data use and system accountability not only mitigates risks but also strengthens stakeholder trust.
A thoughtful approach to these best practices builds a foundation for effective AI integration in cybersecurity. Organizations that prioritize strategic alignment, data quality, workforce readiness, system interoperability, and ethical compliance are better positioned to tackle the complexities of modern cyber threats.
Future trends and developments
The future of AI in cybersecurity points to an ecosystem where predictive and autonomous capabilities dominate. Predictive threat intelligence will play a pivotal role, enabling organizations to forecast vulnerabilities and preempt attacks based on global data patterns and historical behavior. Such advancements reduce reliance on reactive measures, positioning businesses to maintain a strategic advantage against threats.
Quantum computing is another transformative trend on the horizon. As this technology matures, it promises to redefine encryption and decryption capabilities, offering unprecedented speed in analyzing vast datasets. This innovation is expected to bolster defenses against sophisticated cyberattacks, especially in areas like secure communications and real-time threat analysis.
Automation will also extend beyond current applications, changing into systems capable of autonomous threat response. These mechanisms will detect, analyze, and neutralize threats in real time, minimizing human intervention and reducing response times significantly. Combined with advancements in biometric authentication, organizations will have more secure and efficient systems to protect against unauthorized access.
Ethical considerations and regulatory oversight are also set to shape the adoption of AI in cybersecurity. Governments and industry bodies will continue to refine policies to balance innovation with privacy and security concerns. Organizations that embrace these trends early will not only enhance their defenses but also position themselves as leaders in the future of cybersecurity.
AI in cybersecurity is not just a solution; it’s a strategic imperative for safeguarding your business. From proactive threat detection to automated responses, AI ensures you stay ahead of cyber risks. At Lumenalta, we specialize in crafting tailored AI cybersecurity solutions that help organizations to achieve resilience and trust. Together, we’ll secure a brighter future.
Table of contents
- Understanding AI in cybersecurity
- Key applications of AI in cybersecurity
- Benefits and challenges of AI in cybersecurity
- Generative AI in cybersecurity
- Types of AI cybersecurity tools
- Best practices for implementing AI in cybersecurity
- Future trends and developments
- Common questions about AI and cybersecurity
Common questions about AI and cybersecurity
How does AI improve cybersecurity?
What are the risks of using AI in cybersecurity?
Can AI replace human cybersecurity professionals?
How is generative AI used in cybersecurity?
What types of AI tools are commonly used for cybersecurity?
Want to learn how AI and cybersecurity can bring more transparency and trust to your operations?