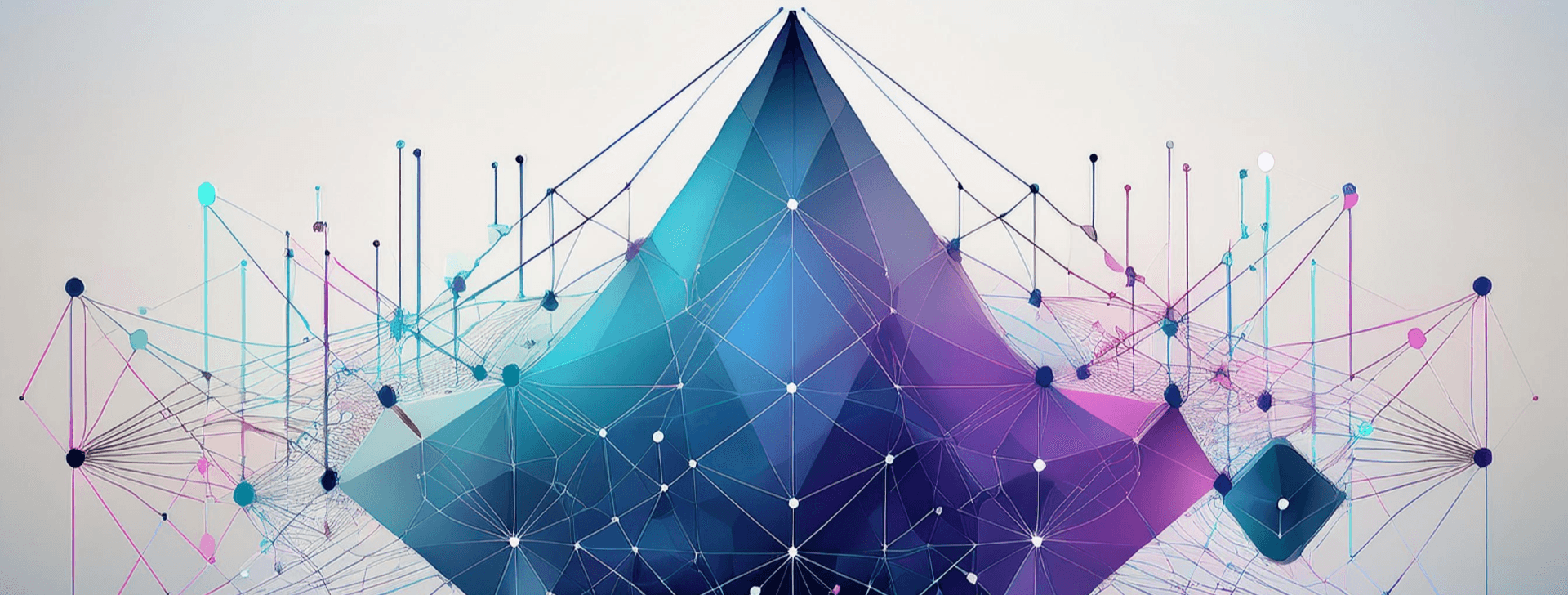
Data integrity vs data quality: Key differences and how to maintain both
NOV. 14, 2024
8 Min Read
Data is the foundation of modern business, but its true value hinges on both its quality and integrity.
Data is the pillar of modern business, but its true value hinges on both its quality and integrity. For organizations, data alone isn’t enough—it must be reliable and fit for purpose to drive effective regulation, compliance, and efficiency. Grasping the distinctions between data integrity vs data quality and the methods to uphold each is essential for utilizing data as a powerful tool. While closely related, data integrity and quality play distinct roles in ensuring that businesses can trust and act upon their data. These elements form the foundation of accurate analytics, compliant reporting, and strategic business insights.
Key takeaways
- 1. Data integrity ensures data accuracy, consistency, and security across its lifecycle, while data quality focuses on usability, completeness, and relevance.
- 2. Both data integrity and quality are essential for reliable decision-making, regulatory compliance, and building customer trust.
- 3. Tools like data quality assessment software and secure storage technologies support robust data governance frameworks that safeguard data integrity.
- 4. Emerging technologies, like AI and blockchain, are reshaping data integrity and quality management, with new regulations further raising standards for data reliability and compliance.
Understanding data integrity
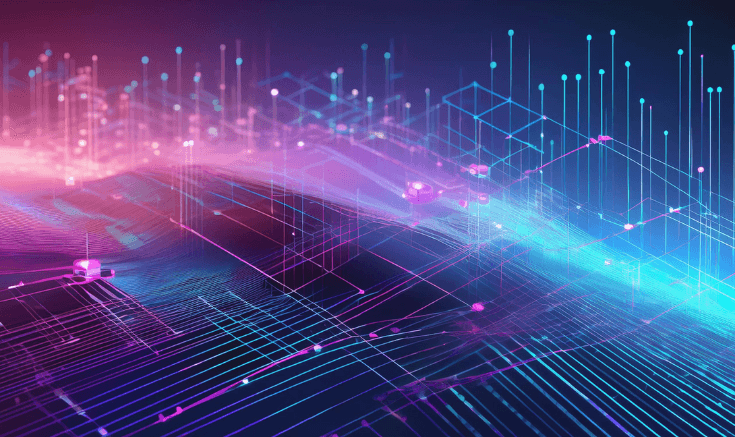
Data integrity refers to the accuracy, consistency, and security of data throughout its lifecycle, ensuring that data remains reliable and unaltered from creation to utilization. Maintaining data integrity is essential for building a trustworthy data environment that supports critical business operations, regulatory compliance, and informed evaluation. Companies enforce data integrity through several key principles, each of which reinforces the data’s reliability and usability.
Accuracy
Accuracy is fundamental to data integrity, as data must precisely represent the real entity or event it describes. Accurate data provides a solid foundation for governance, offering insights that reflect actual conditions rather than distorted information. Inaccurate data, on the other hand, can lead to flawed analytics, misguided strategies, and ineffective business operations. By prioritizing accuracy, companies ensure their data is not only correct but also valuable for analysis and planning.
Consistency
Consistency is another core element, requiring that data remains uniform across various systems, databases, and applications. In conditions where data flows between multiple channels, consistent data helps avoid discrepancies that could create confusion or lead to errors. For example, when customer information is consistent across different systems (e.g., CRM and ERP systems), it allows all teams to work with a unified view of the customer. Consistency is especially crucial for organizations with complex data ecosystems, as even minor discrepancies can have widespread effects on operational efficiency and accuracy.
Security
Data integrity is closely tied to data security, as protecting data from unauthorized access and tampering is essential for preserving its integrity. Data becomes vulnerable to unauthorized modifications without strong security measures, compromising its reliability and trustworthiness. By implementing robust access controls, encryption, and other security practices, companies ensure that only trusted individuals can view or modify sensitive data, safeguarding it from malicious actors or accidental changes.
Reliability
Reliability reinforces the long-term dependability of data, ensuring it remains accurate and consistent over time and across various applications. Reliable data is a stable foundation for analytics and reporting, letting businesses draw accurate insights that support effective management. When data is trustworthy, stakeholders across the organization can trust it as a dependable source, making it easier to align strategies and objectives based on shared information.
These principles form a framework for secure, trustworthy data that organizations can depend on for compliance, operational decisions, and customer interactions. By upholding data integrity, companies create a data environment that protects against errors and inconsistencies and fosters a culture of data reliability and trust. This, in turn, enhances their ability to leverage data as a strategic asset, strengthening both internal operations and external relationships.
“Data integrity refers to the accuracy, consistency, and security of data throughout its lifecycle, ensuring that data remains reliable and unaltered from creation to utilization.”
What constitutes data quality?
Data quality is a measure of the reliability, usability, and integrity of data, essential for making informed decisions, driving efficiency, and supporting strategic planning. Without high-quality data, analytics and evaluation frameworks are compromised, impacting outcomes. Quality data acts as a pillar for effective digital transformation and operational excellence, ensuring that every decision is backed by trustworthy information. Several core attributes define data quality, each playing a vital role in delivering actionable and reliable insights.
Completeness
High-quality data is complete, encompassing a large percentage of the necessary information to provide a comprehensive view. Incomplete data can lead to gaps in understanding and missed insights. For instance, missing customer data can result in an incomplete understanding of the target audience, limiting the success of marketing and customer service efforts. Complete data allows a more holistic perspective, allowing for accurate and well-rounded decisions.
Uniqueness
Data must be free from redundancies and extraneous entries. Unique datasets eliminate duplicate records, preventing misleading analytics. Redundant data can skew analysis and cause inefficiencies, particularly in applications where clean and concise data is critical, like customer segmentation or inventory management.
Validity
Quality data conforms to syntax, structure, and business rules, aligning with predefined standards and requirements. For example, correctly formatted email addresses in customer databases ensure communication accuracy while validating transaction data prevents inaccuracies in financial reporting. Valid data forms a solid foundation for dependable analysis.
Timeliness
Data value often diminishes over time. For real-time evaluation in sectors like retail, finance, or logistics, timely data is crucial to reflect recent developments and trends. Outdated information can distort insights and hinder responsiveness, whereas current data supports practical, immediate actions that align with present conditions.
Consistency
Data must be represented in a standardized manner across systems, ensuring uniformity and coherence. Consistency avoids discrepancies, particularly when data is shared across departments or used in multiple applications. For example, if customer data across marketing, sales, and support teams is inconsistent, it can hinder a cohesive customer experience. Standardizing data formats and representations fosters a single source of truth.
Each of these attributes—completeness, uniqueness, validity, timeliness, and consistency—collectively define data quality, allowing businesses to trust, leverage, and maximize the impact of their data. A commitment to data quality management empowers organizations to use data as a strategic asset, fostering a data-led culture where shared, accurate information drives management and operational success. Investing in data quality provides an edge, permitting organizations to respond to changes with agility and precision while setting a robust foundation for long-term growth.
Why data integrity and data quality are often confused
Data integrity and data quality are related concepts that overlap in areas like accuracy and consistency, but they serve fundamentally different purposes. Data integrity centers on safeguarding and reliability, ensuring that data remains unchanged, secure, and accessible only to authorized parties. Meanwhile, data quality emphasizes usability, ensuring data is relevant, complete, and applicable to the intended tasks. Confusion arises when organizations assume that secure, accurate data is inherently of high quality or, conversely, that robust integrity measures must protect high-quality data. Understanding these distinctions allows organizations to set more precise objectives for data governance and develop targeted strategies that align with their specific needs.
Scenarios where data quality is prioritized over data integrity
Data quality attributes such as relevance and timeliness may take priority in specific contexts. Here are examples where data quality is essential:
- Market research: High-quality data helps capture valuable insights into customer behavior and emerging trends. Completeness and relevance here are crucial for meaningful analysis.
- Product development: Data on customer preferences and needs is key to effective product design. Relevant, accurate data guides the development of features that meet market needs.
- Sales analytics: Accurate and timely data supports sales forecasting, letting teams adapt strategies in real-time. Data quality directly influences a business's ability to respond to market shifts.
Key differences between data integrity and data quality
Data integrity and data quality are both essential in creating a reliable data ecosystem, yet they serve different roles. Data integrity focuses on maintaining the accuracy, consistency, and security of data throughout its lifecycle, ensuring it remains protected from unauthorized alterations. This is crucial in contexts where data authenticity is non-negotiable, such as in compliance, security, and auditing.
Data quality, however, centers on the relevance and usability of data for specific applications. It assesses if the data is fit for purpose, supporting governance, analytics, and strategic planning. By ensuring data is complete, timely, and meaningful, data quality allows teams to leverage information effectively, converting raw data into actionable insights that guide business direction.
Each of these attributes plays a distinct role in supporting data-backed organizations. Data integrity safeguards trust in the data’s accuracy, while data quality empowers users to extract maximum value from it.
Attribute | Data integrity | Data quality |
---|---|---|
Definition | Accuracy, consistency, and security | Suitability of data for specific use |
Primary focus | Protecting data from unauthorized changes | Ensuring data’s relevance and completeness |
Main objective | Maintain data reliability | Improve data usability |
Applications | Compliance, security, auditing | Decision-making and analytics |
Measurement criteria | Consistency, accuracy over time, error prevention | Relevance, completeness, timeliness |
Impact of deficiencies | Inaccuracies can lead to security risks, compliance issues | Poor insights, flawed business decisions |
Validation methods | Checksums, data encryption, access controls | Data profiling, completeness checks, data cleansing |
Primary stakeholders | IT, compliance, security teams | Data profiling, completeness checks, data cleansing |
Data integrity and data quality are two sides of the same coin, each contributing to a reliable and effective data strategy. Integrity ensures data remains consistent, accurate, and secure, supporting trust in its authenticity and compliance with regulatory standards. Quality, in contrast, emphasizes the value and applicability of data, equipping teams to draw meaningful insights that support strategic regulation.
Organizations that can effectively balance data integrity and quality are better positioned to harness data as an asset. By implementing appropriate governance, validation, and monitoring, companies can cultivate a data environment that not only supports operational needs but also drives impactful outcomes. For modern data-powered organizations, both integrity and quality are essential to building a foundation of trust and utility in the information they rely on every day.
Areas where data integrity and data quality intersect
While serving different primary functions, data integrity and data quality overlap in several key areas that strengthen the overall reliability and usability of data within an organization. One of the main intersections is accuracy; both data integrity and quality emphasize the need for accurate data as it forms the foundation of reliable insights and effective management. Another shared area is consistency, which requires data to be uniform across different systems and over time, supporting seamless analytics and reporting processes. This consistency ensures a unified, trustworthy data source for the organization. Lastly, validation is critical for both integrity and quality. However, each with slightly different goals: validation for data integrity protects against unauthorized alterations, whereas validation for data quality ensures that data remains relevant and fit for its intended purposes.
Scenarios where data quality is more crucial than integrity
In certain cases, data quality becomes the main priority, particularly when usability, relevance, and timeliness of data are essential. For instance, in market research, high-quality data is critical for deriving meaningful insights into customer behavior and market trends. Here, completeness and timeliness help provide an accurate snapshot of the current perspective. Product development also depends heavily on relevant data to understand customer needs and preferences, guiding effective design and feature development. Accuracy alone isn’t sufficient—data must be relevant to varying customer requirements. Finally, sales analytics relies on real-time, accurate data to forecast demand and adjust sales strategies accordingly. In this context, timeliness is a vital quality attribute, helping teams adapt strategies to reflect the latest buying patterns and market shifts.
“Data integrity builds trust with customers, partners, and regulators by demonstrating the organization’s commitment to transparency and data protection.”
Importance of data integrity in business
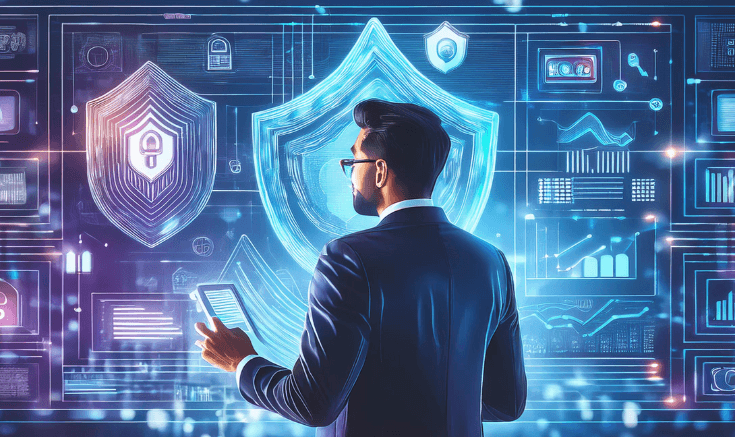
Data integrity is essential for ensuring accurate, consistent, and secure data throughout its lifecycle, and it plays a critical role in various business areas. Below is a breakdown of its importance across compliance, risk management, trust-building, and more.
Risk management
By ensuring data remains consistent and protected from unauthorized changes, data integrity helps organizations reduce vulnerabilities and prevent costly errors. Robust data integrity practices safeguard against operational disruptions and permit accurate analytics and regulation. For example, in financial reporting, even a minor data error can lead to substantial financial losses and impact investor confidence. By maintaining data accuracy and consistency, organizations minimize risk exposure and create a reliable foundation for governance.
Regulatory compliance
In regulated industries like finance, healthcare, and insurance, data integrity is crucial for meeting legal standards. Compliance with regulations such as the General Data Protection Regulation (GDPR), the Health Insurance Portability and Accountability Act (HIPAA), and the Sarbanes-Oxley Act (SOX) requires strict data integrity controls. Measures like secure access controls, audit trails, and regular validation checks ensure that data is accurate, traceable, and accessible only to authorized individuals. Failure to comply can result in severe penalties and legal repercussions. Prioritizing data integrity helps companies adhere to regulatory requirements and reinforces their reputation as responsible handlers of sensitive data.
Building trust
Data integrity builds trust with customers, partners, and regulators by demonstrating the organization’s commitment to transparency and data protection. Companies known for high data integrity foster stronger relationships, as customers feel more confident that their personal information and transaction history are handled securely and accurately. Partners also benefit, as they can rely on the organization’s data for collaborative evaluation. By upholding data integrity, organizations enhance brand loyalty, foster long-term partnerships, and solidify a positive reputation in the industry.
Preventing consequences of compromised data
Compromised data integrity can lead to serious consequences, including legal penalties, financial losses, operational disruptions, and reputational damage. Data breaches, unauthorized alterations, or system errors can compromise data reliability, leading to adverse outcomes. In sectors like finance, compromised data can result in inaccurate reporting, causing stock price drops or regulatory action. Remediation efforts, such as data recovery and investigations, are often costly and resource-intensive. Prioritizing data integrity helps organizations avoid these negative impacts, reinforcing a culture of accountability and resilience.
By proactively managing data integrity, businesses ensure reliable, trustworthy data that supports operational efficiency, compliance, and strong relationships with key stakeholders. This strategic approach to data management helps organizations build long-term value, enhance their positioning, and maintain a stable foundation for sustainable growth.
The role of data quality in evaluation
Data quality is a foundational element in effective evaluation processes within organizations. High-quality data ensures that the insights drawn from analytics are accurate, reliable, and actionable, supporting both day-to-day operations and long-term strategic planning. Here’s a closer look at how data quality influences management, the impacts of poor data quality, real applications, and the metrics used to assess quality in business contexts.
How data quality drives strategic regulation
Data quality provides the foundation for making well-informed strategic decisions. Reliable, accurate data allows decision-makers to assess various scenarios with confidence, set realistic goals, and pinpoint growth opportunities. For example, a company planning to enter a new market requires accurate market data to understand customer demographics, buying behaviors, and competitors. High-quality data ensures that these insights are based on current, comprehensive, and relevant information. Conversely, when data quality is lacking, regulation can become risky, as incomplete or incorrect data can lead to misguided strategies. With high-quality data, organizations can make strategic decisions that align with their goals and drive growth.
Moreover, data quality impacts an organization’s agility and responsiveness. Decision-makers who have access to up-to-date, accurate data can quickly identify emerging opportunities or threats, allowing the business to adapt more effectively. In industries like retail and finance, where trends can shift quickly, the ability to base decisions on reliable data allows the organization to stay aggressive and responsive to market changes.
The impact of poor data quality on business analytics
Poor data quality can hinder analytics, leading to flawed resolutions and costly business missteps. When data is incomplete, outdated, or contains inaccuracies, its insights are compromised, resulting in decisions that do not reflect the actual business condition. For instance, if sales data is missing or inaccurately reported, revenue forecasts and budgeting plans can be significantly impacted, causing the organization to misallocate resources or overlook key investment areas.
Inaccurate data also affects predictive analytics, which many organizations rely on to anticipate customer behavior, demand patterns, or market trends. If the data feeding these predictive models is of good quality, the forecasts will likely be accurate, undermining the value of analytics. Ultimately, poor data quality can lead to ineffective strategies, wasted resources, and missed opportunities for growth. Maintaining high data quality is essential for reliable analytics and strategic planning.
Real examples of data quality in action
Data quality is critical across multiple business functions, supporting operations, enhancing customer relationships, and allowing accurate financial planning.
- Inventory management: High-quality data allows businesses to optimize inventory levels, reducing the likelihood of overstock or stockouts. Accurate data on sales, demand, and supply chain performance facilitates better demand forecasting, ensuring the right stock levels at all times. For example, a retailer with real-time, accurate inventory data can adjust stock based on customer demand, reducing excess inventory and minimizing waste.
- Customer relationship management (CRM): Quality data within CRM systems supports personalized and targeted customer interactions. When customer data is accurate and comprehensive, it equips businesses to understand customer preferences, purchasing history, and engagement patterns. This leads to improved customer satisfaction and loyalty, as companies can tailor marketing and customer service efforts to individual needs.
- Financial forecasting: Reliable data is essential for effective financial planning, helping companies set realistic revenue goals, budget allocations, and investment plans. Accurate sales, expenses, and revenue data provide the foundation for reliable forecasting. For instance, a business that uses high-quality financial data for its quarterly forecasts can make better resource allocation decisions and adjust strategies to align with financial targets.
These examples illustrate how data quality directly contributes to business efficiency, customer satisfaction, and accurate financial planning, showcasing its value across varied areas of operation.
Metrics for assessing data quality in management
Organizations evaluate data quality using specific metrics to ensure that data consistently supports accurate and effective management. These metrics allow companies to determine the reliability of their data and identify areas for improvement:
- Accuracy: This metric evaluates if the data accurately represents the real entity or event it describes. For example, accuracy in customer records ensures that information such as addresses, contact details, and purchasing history is correct, supporting effective marketing and customer service efforts.
- Completeness: Completeness refers to the extent to which all necessary data is present. Missing data can lead to incomplete analyses and poor decisions. For instance, incomplete sales data could distort revenue forecasting, affecting budgeting and investment decisions.
- Relevance: Relevance assesses if the data aligns with the specific needs of the task or analysis. Data that is outdated or unrelated to the objective can lead to skewed insights. For instance, relevant data in product development ensures that customer feedback aligns with current trends and preferences.
- Timeliness: Timeliness measures how up-to-date the data is, ensuring it reflects current conditions. Timely data is essential in dynamic industries, such as retail and finance, where market trends change progressively. Real-time sales data, for instance, helps retailers adjust inventory based on present needs rather than outdated patterns.
By tracking these metrics, organizations can ensure that their data is of high quality, consistently supporting reliable and impactful governance. Regularly monitoring these attributes also helps identify and address quality issues before they disrupt critical business functions, ensuring that data remains a valuable asset in shaping strategies and achieving organizational goals.
“Artificial intelligence is enhancing data quality management by automating error detection, data categorization, and monitoring, making data governance more efficient and scalable.”
Strategies to ensure data integrity
Data integrity is critical for businesses that rely on accurate and secure information. A robust approach to data integrity includes strong security protocols, validation techniques, continuous auditing, and a culture that prioritizes data reliability.
Implementing robust data access and security controls
One of the most effective ways to protect data integrity is by implementing strict access and security controls. Techniques such as role-based access control (RBAC) allow organizations to limit data access to only those who need it, reducing the risk of unauthorized changes or accidental modifications. Additionally, encryption safeguards data by encoding it, ensuring that even if data is intercepted, it cannot be read without the decryption key. Multi-factor authentication (MFA) further strengthens data security by requiring multiple forms of verification before allowing access, creating a secure barrier around sensitive information.
Data validation techniques for integrity verification
Data validation is essential to maintaining data accuracy and preventing corruption. Validation techniques, including audits, cross-checks, and real-time data checks, help ensure data remains consistent and accurate throughout its lifecycle. For example, data audits provide a comprehensive review of records to identify inaccuracies or inconsistencies, while real-time checks detect anomalies instantly, allowing immediate corrective action. Automation enhances validation by continuously monitoring data, reducing manual errors, and adding an extra layer of protection, especially for critical data.
Conducting regular audits and monitoring
Regular audits and ongoing monitoring are proactive methods for identifying potential vulnerabilities in data management. Audits assess if data practices align with established integrity standards, highlighting areas needing improvement. Through continuous monitoring, organizations can detect unauthorized changes in real time, addressing issues before they impact broader operations. Audits and monitoring provide both retrospective analysis and real-time oversight, ensuring that data remains secure, accurate, and reliable.
Fostering a culture focused on data integrity
Building a culture that prioritizes data integrity helps ensure that all team members understand the importance of accurate and secure data. Training sessions on data handling best practices and integrity principles empower employees to manage data responsibly. By fostering a culture where data integrity is valued and upheld, companies reinforce organizational accountability, making data integrity a collective responsibility rather than a departmental task. This approach encourages employees to follow integrity practices and reinforces the organization’s commitment to reliable data management.
Tools and technologies for data integrity and quality management
To maintain high standards of data quality and integrity, organizations rely on a variety of tools and technologies. These solutions help streamline data management, enhance security, and improve overall governance by automating many critical processes.
Tools for data quality assessment
Data quality assessment tools such as Talend, Informatica, and Data Ladder are instrumental in identifying errors, duplicates, and inconsistencies within datasets. These tools offer actionable insights that empower organizations to improve data quality by providing metrics on data completeness, accuracy, and relevance. With these insights, companies can address quality issues proactively, ensuring their data supports accurate analytics, reporting, and management.
Technologies for data security and integrity
Technologies like encryption, access management tools, and blockchain are vital for maintaining data security and integrity. Encryption protects data by encoding it, making it unreadable without the proper decryption key, while access management tools control who can view or edit data, enforcing strict role-based access. Blockchain technology offers tamper-proof data storage by recording transactions in an immutable ledger, providing a highly secure method for data tracking and verification. These technologies help create a secure environment for data, protecting it from unauthorized alterations and ensuring its reliability over time.
Machine learning in data quality and integrity
Machine learning (ML) algorithms are used to enhance data quality and integrity by detecting and correcting issues before they become critical. ML models analyze patterns in data to identify anomalies, predict potential errors, and suggest corrective actions. For instance, predictive modeling can anticipate data quality issues based on historical patterns, allowing organizations to address them proactively. Machine learning also supports data integrity by automating consistency checks and validating data formats, reducing the need for manual intervention and enhancing overall data governance.
Utilizing automation for real-time monitoring
Automation is a powerful tool for maintaining continuous oversight of data quality and integrity. Automated monitoring systems conduct real-time checks, instantly detecting errors or inconsistencies and alerting data managers to potential issues before they impact operations. This approach not only improves efficiency by reducing the burden of manual checks but also allows for a faster response to data integrity or quality issues. By incorporating automation into data management processes, organizations can enhance their data governance practices, ensuring that data remains accurate, secure, and usable throughout its lifecycle.
These tools and technologies, when integrated effectively, provide a comprehensive framework for managing data integrity and quality. They authorize businesses to avoid potential issues, secure sensitive data, and optimize the value derived from their data assets.
Challenges in balancing data integrity and data quality
Balancing data integrity and quality is a complex yet essential challenge for organizations seeking reliable, actionable data. This balancing act requires careful consideration of various technical, operational, and strategic factors. Organizations often face trade-offs between ensuring data is consistently accurate and maintaining its overall usability and accessibility. For leaders, it’s about striking the right balance that authorizes data to drive business decisions while safeguarding its integrity across high-volume, resource-constrained, and various conditions. This guide highlights common challenges in maintaining data integrity and quality and presents actionable strategies to handle them effectively.
Recognizing trade-offs between data quality and integrity
- Challenge: Enhancing data quality, like integrating new data sources, can introduce risks to data integrity, potentially causing inconsistencies or data loss.
- Strategy: Define clear data governance policies that prioritize either quality or integrity based on the specific business need. For instance, strict integrity controls may be necessary for compliance, while flexibility for quality might be prioritized in analytics or customer insights.
Common pitfalls and solutions
- Challenge: Inconsistent standards and unclear communication between departments can lead to quality variations or compromise data integrity.
- Strategy: Standardize data protocols across all departments, ensuring uniformity in data handling, validation, and security. Regular cross-departmental training can also keep all team members aligned on data management best practices.
Balancing quality and integrity in high-volume environments
- Challenge: High data volumes increase the likelihood of processing delays, errors, and inconsistencies. Traditional manual data governance methods may not be effective at scale.
- Strategy: Implement scalable, automated systems for real-time monitoring, quality checks, and error detection. Automation helps manage large data volumes without compromising performance or the standards for data quality and integrity.
Overcoming budget and resource constraints
- Challenge: Budget limitations can make it challenging to implement thorough data governance practices, especially when advanced validation and monitoring tools are required.
- Strategy: Use automation to conduct routine quality checks and integrity validations, conserving time and resources. Prioritize high-impact data areas for quality and integrity safeguards, focusing on where accuracy and security matter most.
Ultimately, maintaining data integrity and quality is not a one-time task but an ongoing process requiring commitment, strategy, and adaptability. Organizations can achieve robust data practices that support informed evaluation by implementing clear governance, standardizing protocols, and using automation. While challenges such as resource constraints, scalability, and cross-departmental consistency may persist, a proactive approach to managing these issues ensures that data remains a valuable asset. As data continues to be a critical driver of innovation and growth, organizations prioritizing integrity and quality will prosper in a data-powered setting.
Future trends in data integrity and data quality
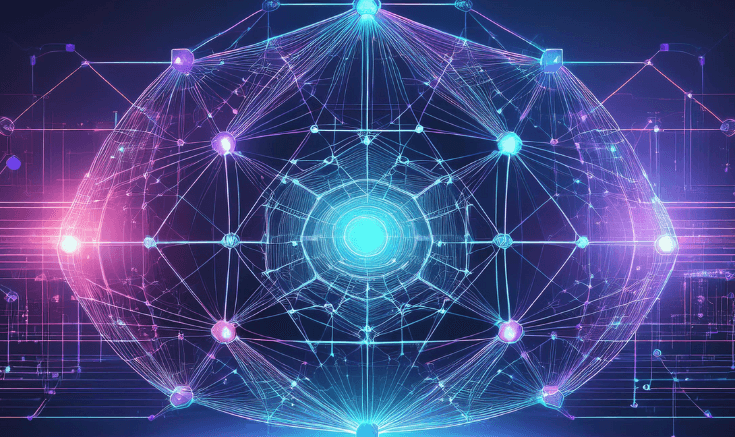
As organizations strive to keep pace with the growing setting, emerging technologies and regulatory frameworks are reshaping the way data integrity and quality are managed. Artificial intelligence (AI) is a key driver in this evolution, bringing efficiency and scalability to data quality management through automated error detection, data categorization, and real-time monitoring. These AI-based solutions streamline data governance by minimizing manual intervention and allowing rapid identification of issues across vast datasets. Blockchain and distributed ledger technologies are also gaining prominence as tamper-proof solutions for maintaining data integrity. By offering secure, traceable records, these technologies ensure that data remains unaltered and transparent throughout its lifecycle. Compliance regulations, such as the General Data Protection Regulation (GDPR) and the California Consumer Privacy Act (CCPA), also intensify the need for robust data integrity and quality standards. These regulations mandate stricter controls over data usage, accuracy, and protection, making it crucial for businesses to stay updated to avoid legal consequences.
As businesses handle the complexities of data integrity and quality, Lumenalta stands as a trusted partner, helping organizations use cutting-edge technology and strategic insights to build a resilient data foundation. By using AI-led data quality solutions, secure blockchain frameworks, and a proactive approach to regulatory compliance, Lumenalta empowers companies to convert their data into an advantage. Our commitment to data excellence ensures that every decision you make is supported by accurate, reliable, and compliant information, reinforcing trust, driving growth, and paving the way for sustainable success.
Table of contents
- Understanding data integrity
- What constitutes data quality?
- Why data integrity and data quality are often confused
- Key differences between data integrity and data quality
- Importance of data integrity in business
- The role of data quality in evaluation
- Strategies to ensure data integrity
- Tools and technologies for data integrity and quality management
- Challenges in balancing data integrity and data quality
- Future trends in data integrity and data quality
- Common questions about data integrity vs data quality
Common questions about data integrity vs data quality
What is the main difference between data integrity vs data quality?
How do companies maintain both data integrity and data quality?
Why are data integrity and data quality often confused?
Why is automation important in data quality and integrity management?
Want to learn how data strategy can bring more transparency and trust to your operations?