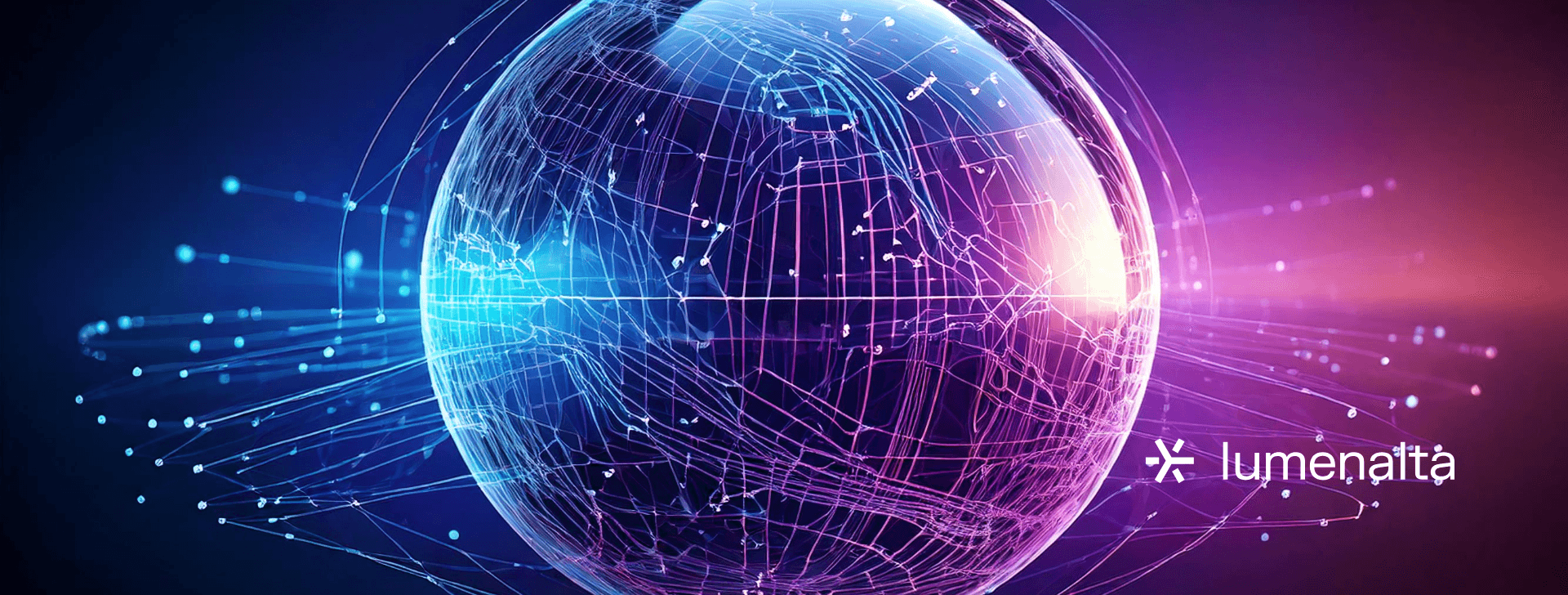
7 examples of predictive analytics in customer services
NOV. 29, 2024
7 Min Read
Predictive analytics is transforming customer service by shifting from reactive problem-solving to proactive decision-making.
With tools that analyze historical data and identify patterns, businesses can forecast customer needs, prevent issues before they arise, and deliver highly personalized support experiences. This approach enhances customer satisfaction while streamlining operations, enabling organizations to allocate resources more effectively and resolve challenges with greater precision. By anticipating behaviors and tailoring interactions, predictive analytics not only builds stronger customer relationships but also positions companies as leaders in service innovation.
"Predictive analytics enables businesses to go beyond reactive problem-solving by delivering proactive, tailored support."
Key takeaways
- 1. Predictive analytics transforms customer service by enabling proactive problem-solving and personalized interactions.
- 2. Anticipating customer needs and preferences increases satisfaction and loyalty while driving operational efficiency.
- 3. Advanced tools such as machine learning and sentiment analysis enhance the accuracy and scope of predictive models.
- 4. Implementing predictive analytics requires clear goals, quality data, scalable platforms, and cross-team collaboration.
- 5. Real-time analytics and ethical data practices are critical for future advancements in customer service.
What is predictive analytics in customer support?
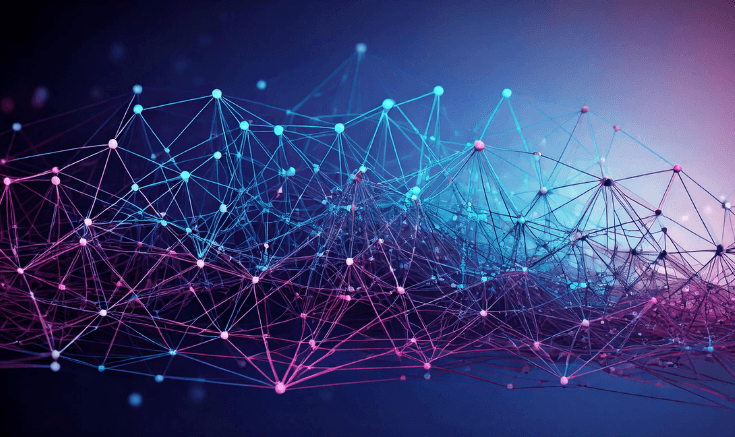
Predictive analytics in customer support refers to the use of advanced statistical algorithms and machine learning to forecast customer behaviours, preferences, and potential issues before they occur. By analyzing historical data and identifying patterns, this technology enables support teams to anticipate needs, enhance customer satisfaction, and optimize operations.
At its core, predictive analytics enables businesses to go beyond reactive problem-solving by delivering proactive, tailored support. For example, instead of merely addressing a customer complaint, predictive models can alert the team to recurring issues, allowing them to intervene before a problem arises. This approach not only improves customer experience but also increases operational efficiency by minimizing downtime and resource wastage.
By integrating predictive analytics into customer support, organizations gain actionable insights that help them prioritize high-impact tasks, allocate resources effectively, and maintain stronger, long-term relationships with their customers.
Examples of predictive analytics in customer services
Predictive analytics has emerged as a cornerstone of modern customer service strategies, offering a proactive and data-driven approach to addressing customer needs. Unlike traditional reactive methods, predictive analytics enables organizations to foresee challenges, predict outcomes, and craft tailored solutions that resonate with their customers. By analyzing historical data, customer behaviour patterns, and external factors, this powerful tool equips businesses with insights to enhance decision-making and elevate customer experiences.
Incorporating predictive analytics into customer services allows companies to go beyond fixing problems as they arise. Instead, they can anticipate customer needs, personalize interactions, and optimize internal processes to deliver faster, more effective solutions. This capability is especially valuable in competitive markets, where customer loyalty hinges on seamless, personalized, and proactive service.
Below are seven detailed examples showcasing how predictive analytics is reshaping customer services across industries. These applications highlight the potential of leveraging advanced analytics to improve customer retention, operational efficiency, and overall satisfaction.
1. Anticipating customer needs
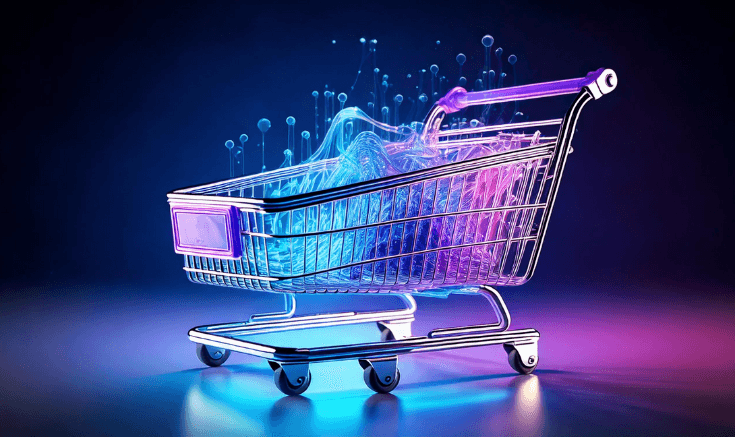
Predictive analytics leverages historical purchase data, browsing behaviour, and demographic information to predict what a customer is likely to need next. For instance, subscription services can analyze usage patterns to determine when a customer might run out of a product, sending timely reminders or auto-replenishment options. Similarly, travel companies can predict when a customer might plan their next trip based on previous bookings and seasonal trends.
- Industry application: Retailers use this capability to send proactive suggestions for add-ons or replacements, like offering printer ink shortly before it runs out.
- Technology in action: Machine learning algorithms analyze trends and calculate probabilities of certain needs arising, helping businesses align their inventory and marketing strategies.
Key benefit: By anticipating needs, businesses can increase revenue through upselling and cross-selling while ensuring customers feel valued and understood.
2. Personalizing customer interactions
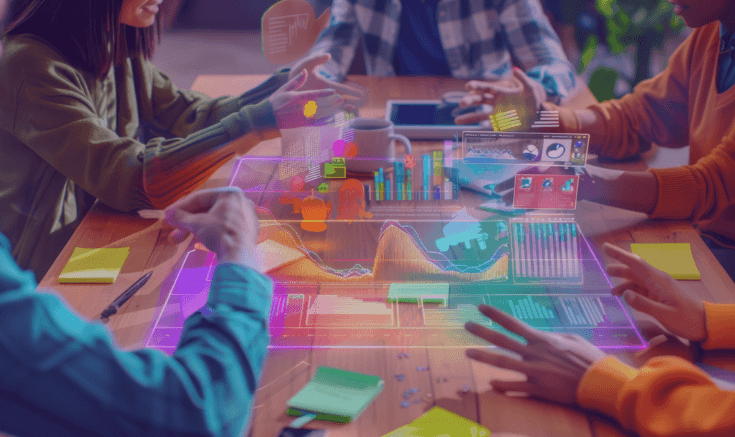
Personalization is no longer a luxury but an expectation, and predictive analytics makes it scalable. Advanced algorithms assess a customer’s preferences, purchase history, and behavior to provide unique recommendations, content, or communication strategies. In streaming services, for instance, predictive analytics suggests content based on viewing habits, which enhances user satisfaction and engagement.
- Industry application: Hospitality providers use predictive models to customize room preferences, meal options, and loyalty rewards based on past stays.
- Technology in action: Natural language processing (NLP) helps create personalized email campaigns or chat responses tailored to individual customer profiles.
Key benefit: Personalized interactions drive higher engagement, improve customer retention, and create memorable service experiences.
3. Predicting and reducing customer churn
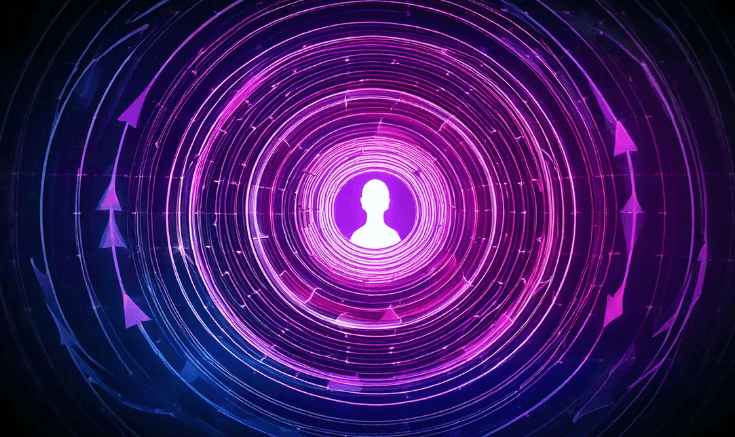
Customer churn can be detrimental to a business’s growth, but predictive analytics enables proactive measures to retain customers. By analyzing variables such as declining purchase frequency, reduced website visits, or customer complaints, businesses can identify early warning signs of dissatisfaction. These insights allow timely interventions, such as personalized offers or outreach from support teams.
- Industry application: Telecom companies use predictive analytics to identify subscribers at risk of cancelling services and offer retention incentives like discounts or feature upgrades.
- Technology in action: Predictive models integrate multiple data sources to assign churn scores to customers, prioritizing those at the highest risk for retention campaigns.
Key benefit: Reducing churn not only preserves revenue but also lowers acquisition costs, as retaining a customer is more cost-effective than acquiring a new one.
4. Enhancing resource allocation
One of the operational challenges in customer service is managing resources effectively during peak times. Predictive analytics provides actionable forecasts of customer interaction volumes by analyzing past data, seasonal trends, and event-based spikes. This ensures optimal staffing, reduces wait times, and prevents overloading support teams.
- Industry application: Call centers use predictive analytics to staff appropriately during holiday shopping seasons or new product launches, balancing costs and service quality.
- Technology in action: Time series forecasting models analyze historical ticket volumes and external variables, such as promotions or product launches, to refine staffing schedules.
Key benefit: Better resource allocation minimizes operational inefficiencies, ensures faster response times, and enhances the overall customer experience.
5. Detecting fraudulent activities
Fraud detection is critical in maintaining customer trust and protecting business operations. Predictive analytics models analyze patterns in transaction data to identify anomalies that suggest potential fraud. These systems learn from historical fraud cases to continuously improve their accuracy in detecting threats.
- Industry application: Financial services deploy predictive models to flag unusual transactions, such as unauthorized access to accounts or sudden, high-value purchases.
- Technology in action: Real-time analytics engines monitor transactions as they occur, cross-referencing them against customer profiles and historical behaviors for fraud detection.
Key benefit: Identifying fraud early mitigates financial losses, secures customer accounts, and fosters a sense of safety and reliability.
6. Automating support ticket categorization
Predictive analytics optimizes ticket handling by analyzing customer inquiries and automatically categorizing them for the most appropriate support team. This reduces manual sorting, speeds up resolution, and improves accuracy. For example, emails containing phrases related to billing issues can be routed directly to the finance team.
- Industry application: IT service desks use predictive analytics to identify ticket severity and assign priority levels automatically.
- Technology in action: Machine learning algorithms process text data from customer tickets, identifying keywords, sentiment, and context to classify and assign tickets efficiently.
Key benefit: Streamlined ticket categorization reduces response times, enhances first-call resolution rates, and ensures a smoother customer experience.
7. Improving product or service quality
Predictive analytics can detect patterns in customer feedback, service interactions, and complaints to identify areas for improvement in products or services. For instance, if multiple customers report similar issues with a product, predictive models can flag it as a recurring problem, prompting proactive measures to address it.
- Industry application: Automotive companies use predictive analytics to identify defects in vehicles based on maintenance reports and warranty claims.
- Technology in action: Sentiment analysis tools process customer feedback, helping businesses understand product reception and pinpoint potential flaws.
Key benefit: Proactively resolving issues enhances customer satisfaction and reduces reputational risks associated with unresolved complaints.
"Streamlined ticket categorization reduces response times, enhances first-call resolution rates, and ensures a smoother customer experience."
Predictive analytics implementation and best practices
Successfully implementing predictive analytics in customer service requires a strategic approach that balances technology, data, and organizational alignment. By focusing on clear objectives and following best practices, businesses can maximize the value of predictive analytics while avoiding common pitfalls.
Define clear goals and outcomes
Before deploying predictive analytics, it’s critical to establish well-defined goals. These may include reducing customer churn, improving service response times, or increasing customer satisfaction. Clear objectives ensure that the analytics models are designed to address specific business challenges, delivering measurable results.
Invest in quality data
The accuracy and reliability of predictive models depend heavily on the quality of data used. Businesses must prioritize data integrity by cleaning, normalizing, and structuring their data sources. Additionally, integrating diverse data sets, such as customer behavior logs, transaction histories, and social media interactions, enriches the predictive model’s output.
Adopt scalable technology platforms
Selecting the right technology stack is crucial for deploying predictive analytics effectively. Cloud-based platforms with built-in analytics capabilities or AI tools enable scalability and real-time processing. Such systems can adapt to growing data volumes and evolving business requirements, ensuring long-term usability.
Focus on model interpretability
While complex machine learning models can deliver high accuracy, they must also be interpretable. Customer service teams benefit from tools and dashboards that present analytics findings in an easy-to-understand format, allowing them to make informed decisions quickly.
Train and empower staff
Predictive analytics tools are only as effective as the teams using them. Organizations must invest in training programs to equip customer service representatives and managers with the skills to interpret analytics insights and incorporate them into their workflows. Collaborative efforts between analytics experts and service teams ensure smooth adoption.
Monitor and iterate continuously
Predictive models are not static; they require ongoing monitoring and refinement to maintain accuracy. Regularly reviewing model performance, incorporating new data, and adjusting algorithms help ensure the analytics continue to meet business goals.
Key considerations for implementation
- Data privacy compliance: Adhere to regulations like GDPR or CCPA to safeguard customer data and build trust.
- Cross-departmental collaboration: Align analytics efforts with marketing, operations, and IT teams to ensure consistent strategies and data sharing.
- Customer-centric design: Ensure that predictive analytics initiatives are designed with the customer’s experience in mind, prioritizing their needs and preferences.
By following these best practices, organizations can unlock the full potential of predictive analytics, transforming their customer service operations into proactive, value-driven processes.
The growing role of predictive analytics in customer service
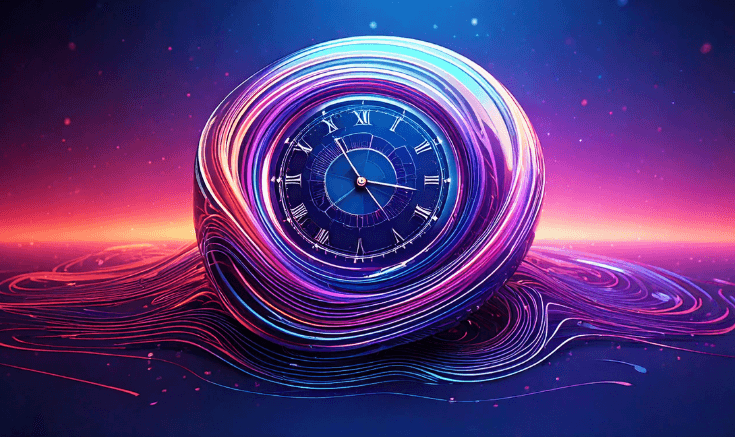
The future of predictive analytics in customer services lies in real-time insights and increasingly advanced AI capabilities. Organizations are beginning to shift from batch data processing to real-time analytics, allowing them to respond instantly to customer needs. This transition enhances the ability to provide context-aware and proactive support across various industries, including e-commerce, financial services, and healthcare.
As artificial intelligence and machine learning continue to evolve, predictive models are becoming more sophisticated. These advancements enable businesses to recognize complex patterns, delivering highly accurate forecasts and deeper personalization for customers. Additionally, sentiment analysis is emerging as a critical feature, using text, speech, and behavioural data to predict emotions and ensure empathetic responses to customer concerns.
Ethical AI and data privacy are also becoming focal points, as companies work to maintain transparency and comply with regulations like GDPR and CCPA. Future solutions will need to prioritize customer trust while leveraging analytics effectively. Moreover, the integration of predictive analytics with omnichannel platforms ensures consistent, seamless experiences across all customer touchpoints.
These innovations collectively promise a transformative impact, positioning predictive analytics as a cornerstone for delivering exceptional customer service in the years to come.
Predictive analytics in customer service is redefining how businesses understand and support their customers. By equipping teams with the tools to anticipate needs and deliver personalized solutions, predictive models pave the way for stronger relationships and more efficient operations. At Lumenalta, we specialize in building tailored predictive analytics solutions that align with your goals, enabling your team to excel in delivering proactive and impactful customer experiences. Explore how our expertise can help your business lead in customer support innovation. Let’s create a brighter path together.
table-of-contents
- What is predictive analytics in customer support?
- Examples of predictive analytics in customer services
- 1. Anticipating customer needs
- 2. Personalizing customer interactions
- 3. Predicting and reducing customer churn
- 4. Enhancing resource allocation
- 5. Detecting fraudulent activities
- 6. Automating support ticket categorization
- 7. Improving product or service quality
- Predictive analytics implementation and best practices
- The growing role of predictive analytics in customer service
- Common questions about predictive analytics in customer services
Common questions about predictive analytics in customer services
What industries benefit most from predictive analytics in customer service?
How does predictive analytics improve customer satisfaction?
What is required to implement predictive analytics in customer support?
Are predictive analytics tools suitable for small businesses?
What role does AI play in predictive analytics for customer service?
Want to learn how predictive analytics in customer services can bring more transparency and trust to your operations?