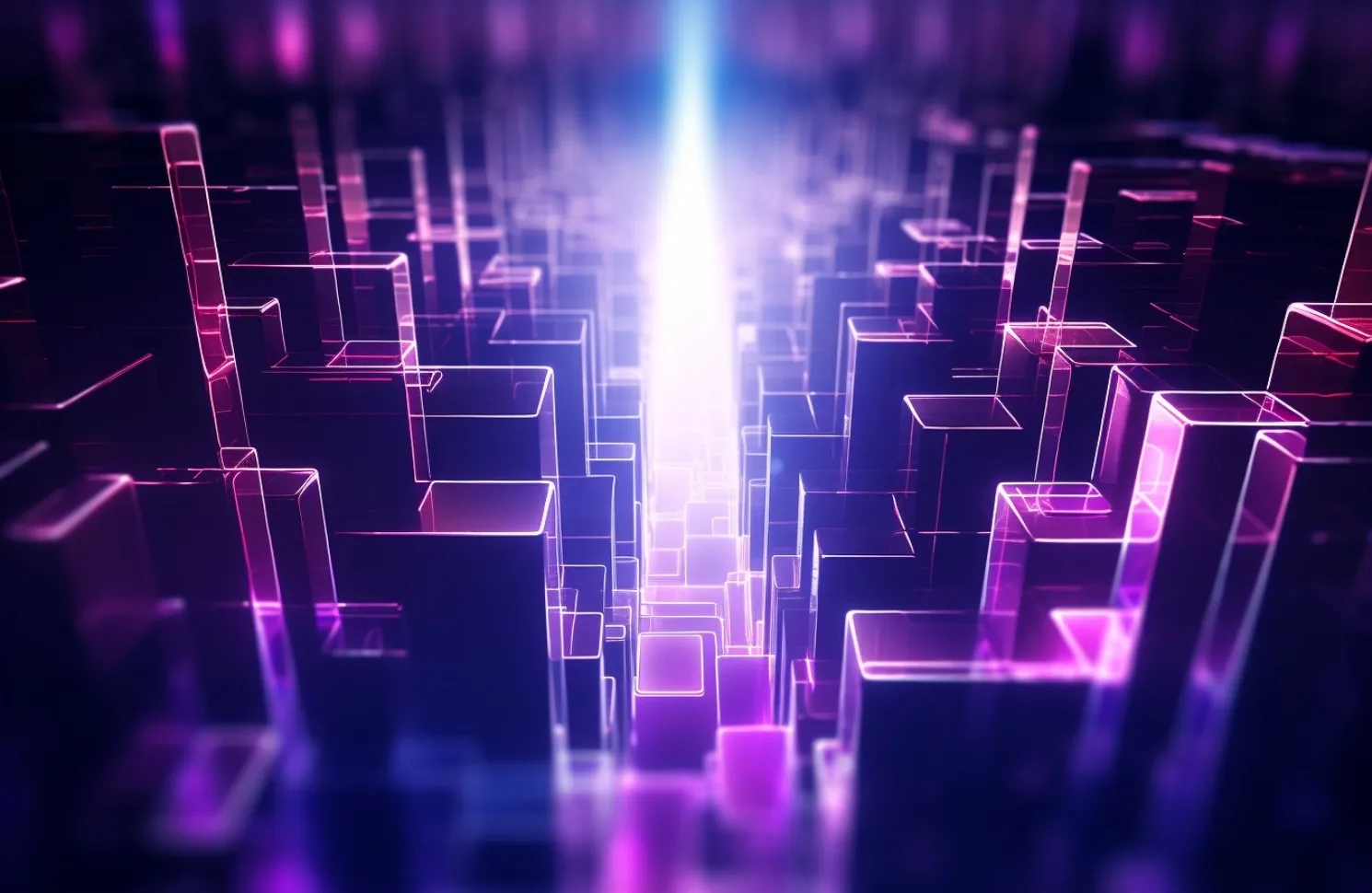
Is your business AI-ready? 10 questions to assess AI fitness
APR. 26, 2024
2 Min Read
Business leaders want to adopt AI and machine learning quickly. But are their companies ready?
Business data insights, such as analytics, customer feedback, and trends fuels decision-making and customer satisfaction. Companies knew this even before computers. But it is especially true since the rise of business software and databases, which have made it easier and cheaper for every company to collect more data.
Now, there’s artificial intelligence. Business leaders want to adopt AI and machine learning quickly to improve employee productivity, customer engagement, and research.
But are their companies AI-ready?
“Business leaders are excited by the promise of this technology, but they express key concerns about safely integrating it into their organizations. There are worries about vulnerabilities stemming from inaccuracies and misinformation, human error, and internal and external data breaches.”
- The Harris Poll, published by Insight Enterprises
We explain the technical and organizational requirements for developing effective AI-powered tools and generating high-quality predictive and generative outputs. If you’re struggling to manage your data, these questions will help you get up to speed. And if you already have good data practices, you’re halfway there.
AI brings new concerns for data storage, processing, AI governance, and organizational practices. We ask ten questions to determine if our clients are AI-ready. They fall into three categories:
- Determine if your data and technology are AI-ready
- Make your technology compliant, secure, and private
- Ensure organizational buy-in, deliverable objectives, and skills
Determine if your data and technology are AI-ready
We always start by assessing the tech. Machine-learning AI has no frame of reference beyond its models and training data. Quality data and well-defined, tested algorithms produce the most successful results.
“If an e-commerce company is using AI to personalize product recommendations, they must have clean data that their recommendation engine can easily access. If their data is messy or incomplete, the AI system might recommend irrelevant products, which could lead to lost sales and unhappy customers.”
- Bernard Marr, best-selling author of Generative AI in Practice
1. Do you have enough quality data?
Machine learning AI depends on large amounts of quality data. AI models process and analyze data quality to look for meaningful relationships and patterns, then use these patterns to generate knowledge and make decisions.
The better the data, the better the business insights, and the better the decisions.
Good data helps AI models provide expert insights and make reliable and autonomous decisions. Bad AI data does the opposite. Inaccurate or incomplete data gives a computer false impressions, and too little data, unrepresentative samples, or poor data quality mislead machines and businesses.
The solution is not to amass as much data as possible, though. While big data is indeed beneficial, having the right amount is also important. It has to cover all use cases.
Imagine a data set of English literature with only British authors. That’s a lot of literature, and it captures a good sample — Shakespeare and JK Rowling, for example. But, no Hemingway? No Jane Austin or Stephen King?
2. Is your data infrastructure powerful and fast?
A slow, complex database or system will impede any business AI initiative. Many organizations still run legacy systems that are not built for AI. Custom software and proprietary data sources make machine learning difficult.
Engineers need easy access to large amounts of data to build and train their models. Their algorithms use size and speed to capture usage feedback, which they use to retrain and refine their models.
Many machines run in parallel for lengthy training and testing, requiring powerful processors to perform intensive operations without impeding your business’s daily processes.
3. Is your data centralized and easily accessible?
Creating an AI-ready infrastructure involves more than buying hardware. It often involves replacing the infrastructure with considerable-sized data storage that cloud services offer. The key to creating a powerful AI data source is to build a single truth source in a centralized location.
Database technologies like a data lake or data lakehouse provide structures to combine your company data and give data scientists query tools, formatting flexibility, and access to metadata. For example, the raw format of data lakehouses gives data scientists the flexibility to work with any AI model.
Centralizing data comes from having an organization-wide integration engine that integrates your data. Data integration involves planning, engineering resources, and a proper infrastructure that should scale for new and updated data as your business grows.
It should also run in real time. We can assess your current technology and provide a roadmap and engineering resources to help you build an integrated, AI-ready system.
Make your technology compliant, secure, and private
While data quality is a precondition for AI integration, regulations, and strong security protect your data.
4. Do you follow regulatory and compliance laws and guidelines?
AI regulation is nascent and rapidly evolving. To create responsible AI, businesses must prioritize compliance to protect against financial and reputational risks. Understanding how to navigate the increasing number of local and international laws regulating AI is crucial.
Your company is subject to regulations on the use of personal data. For example, there’s the EU General Data Protection Regulation (GDPR) and the California Consumer Privacy Act (CCPA).
New regulations that target artificial intelligence, such as Europe’s AI Act, regulate AI applications directly. EU regulators will examine how your AI impacts users and whether its outputs are transparent and explainable. They will also look at the quality of its models and data.
Consider the example of metadata. Food companies must say what’s in their products, and regulators now want companies to explain their algorithms. For instance, the EU AI Act requires that regulators be able to trace the data, and the same goes for users and content creators who want to know what data a machine uses to generate results.
Complying with these regulations takes time, effort and specialized knowledge. What do you label, and how? We can handle the technical, legal and ethical considerations, and help you build scalable controls for successful compliance and AI governance.
5. Are you protecting personal and proprietary information?
AI can pose a serious risk to sensitive financial and customer data. You want to ensure your employees do not leak customer and business secrets to ChatGPT and other generative AI applications; otherwise, such mistakes could result in reidentification. Your AI models should be built to avoid accidental information discovery.
Two issues are at play here:
- Protection of proprietary information from entering public models like ChatGPT. You can do this by making an in-house GPT or teaching employees not to share secrets.
- Cybersecurity. Organizations must implement comprehensive cybersecurity safeguards to protect the data infrastructure from compromise.
The data used to train AI models usually includes names, addresses and financial information. It might also include business contracts and source code, depending on the use case. This proprietary data could fall into the wrong hands without proper security, leading to disastrous legal, economic and reputational harm.
Many industry standards and engineering methodologies reinforce a secure infrastructure.
Keeping up to date with the newest cyberattacks is critical to security. We can help you use security testing techniques, such as tools that simulate hackers, to find and fix system flaws.
6. Do your customers understand how you use AI?
AI adoption is already driving how organizations design their applications and personalized experiences. Yet, some customers still have reservations. They worry their data will fall into the wrong hands or don't trust AI algorithms to make decisions for them.
For example, banks and online retailers use AI to personalize services or detect fraud. Machine learning based on personalized data enables organizations to engage more knowingly with the public.
However, customers and regulators want to know what sensitive data the organization uses and understand the reasoning behind the decisions. These organizations must be open to questions about how their models work.
Financial services are starting to use chatbots that rely on private financial data to offer investment tips to their customers. Compliant institutions must audit their algorithms to prevent abuse and avoid bias, and they must inform their customers, regulators, and employees. Such conscientious compliance builds trust.
Ensure organizational buy-in, deliverable business objectives, and skills
Businesses need help in developing their organization's AI strategy. Studies show that 70% of companies are unhappy with their AI projects, and 87% of their data science projects never go into production. The message here is that good tech only gets you so far — changes to organizational practices matter just as much.
7. Do you have AI expertise, experience, and talent?
AI is a young technology that requires mature skills and experience. Spreading AI knowledge and skills across the organization is critical. But finding talent is not easy.
We can give you access to AI professionals who specialize in AI technologies and will help drive your projects and digital transformation. They will also transfer their knowledge as they work, to enable you to continue independently.
For example, say you want to build a product recommendations platform. Your product teams will decide on the catalog recommendations, data engineers will collect and change the data, and data scientists will analyze the data and use AI models.
We can partner with you to unite these professionals and reinforce their experience and expertise. Your team will be complemented by our developers, product analysts, and designers, who will leverage the latest technology and tools to help solve your company's problems.
We not only offer the technical expertise to build AI, but we also understand the soft skills needed to manage AI projects and train employees to use new tech.
Read our case studies to see examples of our client work.
8. Have you set clear business objectives and obtained strategic alignment?
As you may have guessed, AI adoption comes with a cost. You'll need to work with AI experts, train your employees, and update your IT equipment and software. AI readiness requires a shift in your organization, which can be costly and may encounter employee resistance and low adoption if you don’t first obtain executive buy-in.
Balancing costs and benefits is crucial for this top-level engagement. Executives want to understand and define the goals AI technologies can achieve. They do not want to allocate budget to AI projects just because they seem interesting.
Lumenalta includes executive teams at the outset, developing plans to ensure AI integration meets the organization’s strategy.
9. Are you overemphasizing short-term wins at the expense of long-term thinking?
Companies often jump into AI without understanding what it can do for them. They may choose the wrong solution or define an overly broad scope, setting themselves up for failure.
Successful organizations act on what AI teaches them and learn from their data, applying these lessons in their business operations and digital transformation. They create a virtuous cycle in which everyone in the organization evolves.
Starting small is the right approach. Tightly scoped AI projects build a foundation for the future and provide confidence to take on longer-term business objectives. The feedback cycle is based on data generated by an AI system, where today’s results teach us what to do differently tomorrow.
It always comes back to data. The first version’s feedback informs the next version, teaching the machines and the data engineers how to fine-tune their learnings.
Anticipating future needs is essential. An online marketplace might use AI technologies to predict customer behavior, expecting immediate results. But if the results come in too quickly, the organization might not be ready to respond. They may not understand their customers’ new buying patterns.
Should they diversify and adapt their inventory? When? Is it time to add AI to their sales, stock, and shipping logistics? Machine-learning AI is helping businesses manage the sudden changes in their markets and internal operations.
10. Do you have a roadmap or a future scalability plan?
AI-ready companies take an approach that expands their projects and plans. They may follow a design-first, build-next process or go agile. For this, they need to develop a roadmap of the project’s method and goals that includes timelines and resource allocations.
Many organizations do well following the agile Minimum Viable Product (MVP) method. MVP allows companies to release small projects and make improvements during the software development cycle.
Smaller releases enable businesses to validate or invalidate assumptions and learn what clients need. Eventually, the MVP pilot becomes the full product over time as the company releases future iterations of its algorithms.
Determining requirements and scope right at the outset is essential. Because AI capabilities are rapidly evolving, an AI project must involve planning for the long term, which means identifying top business goals and investing in the tech and staff needed to scale and grow your digital transformation.
So, are you ready for AI?
Succeeding with AI relies on the skills and effective collaboration of data scientists, developers, designers and product analysts. AI doesn’t come out of the box—they must know how to tailor these new technologies reasonably to your objectives.
We assess your AI readiness by focusing first on your data, evaluating its quality and the processes that collect and centralize it. But we know quality data is only the foundation. Implementing AI places new demands on your infrastructure, security, and organization.
Answering these ten questions helps us ensure you’re ready to transform your organization for the AI future.