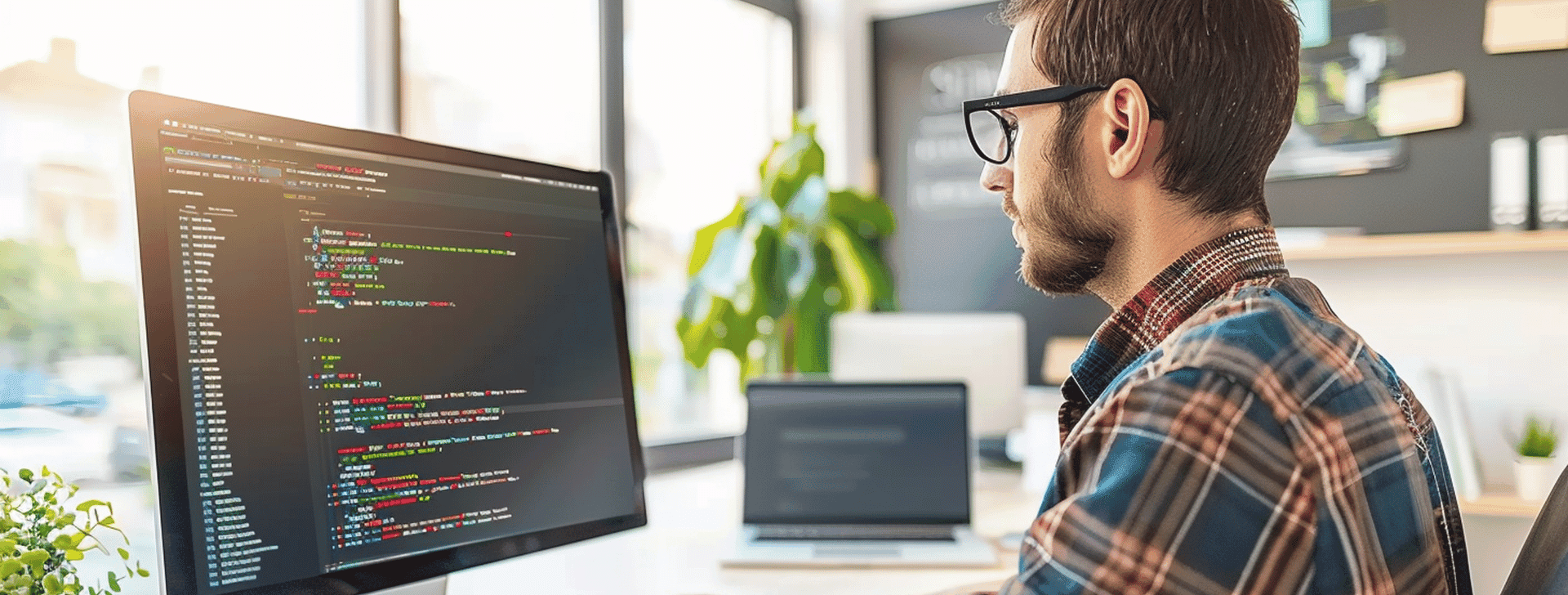
AI’s limitations: 5 Things artificial intelligence can’t do
AUG. 27, 2024
11 Min Read
Artificial Intelligence (AI) has revolutionized industries, from healthcare to finance, transforming how organizations operate.
However, despite its impressive advancements, AI still faces significant limitations. Business decision-makers (BDMs) and industry professionals must be aware of these limitations when considering AI-driven solutions for their organizations. This article outlines the boundaries of AI technology, shedding light on its potential, challenges, and current shortcomings.
Key takeaways
- 1. AI can lack true understanding and creativity, making it highly effective for data analysis but unsuitable for tasks that require nuanced decision-making.
- 2. AI systems rely heavily on high-quality data; poor data introduces bias and inaccuracies, which can lead to flawed outcomes in critical industries.
- 3. Explainable AI (XAI) enhances AI transparency, allowing users to understand how decisions are made, crucial for sectors like healthcare and finance.
- 4. Human-AI collaboration is essential for overcoming AI's limitations, leveraging human creativity alongside AI's data-driven capabilities.
- 5. Ethical concerns such as data privacy, security, and bias are major challenges for AI adoption, highlighting the need for governance and regulation.
The current state of AI
Artificial Intelligence has evolved rapidly over the last decade, becoming integral to a wide range of industries, from healthcare to retail. Today, AI powers everything from recommendation algorithms on streaming platforms to sophisticated machine learning models in financial services. However, it is essential to recognize that the current state of AI, while powerful, still faces limitations that stem from its underlying architecture and operational dependencies.
AI's current applications predominantly rely on narrow AI, or weak AI, which excels in performing specific tasks—such as image recognition, natural language processing, and data analysis. Narrow AI is a step toward broader applications but is limited by its specialization. It cannot function beyond its pre-defined capabilities, making it vastly different from human intelligence. General AI, which would perform any intellectual task a human can, remains a distant, theoretical concept at this stage.
A breakdown of key advancements and limitations in the current state of AI:
Strengths:
- Data processing: AI can analyze vast amounts of data far faster than humans, making it indispensable in industries like finance and healthcare.
- Automation: Repetitive tasks are easily automated, increasing efficiency and reducing human error in industries such as manufacturing.
- Predictive modeling: AI excels at forecasting and trend analysis in sectors like retail and logistics.
Limitations:
- Contextual understanding: AI struggles with understanding nuance or context, often resulting in errors in decision-making that require human intervention.
- Creativity: While AI can generate content or ideas, it lacks genuine creativity and cannot innovate outside the scope of its programming.
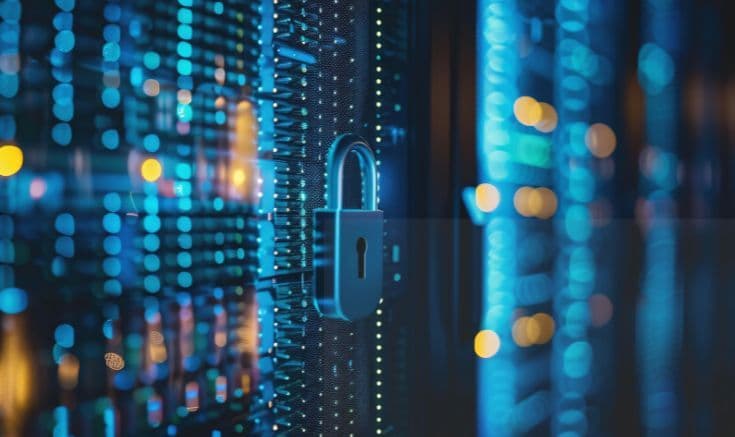
Key aspects of the current AI landscape
Data analysis
- Current capabilities: Fast processing and analysis of large datasets
- Limitations: Limited by data quality and contextual gaps
Natural language processing (NLP)
- Current capabilities: Used in chatbots, virtual assistants
- Limitations: Struggles with complex, multi-context conversations
Automation
- Current capabilities: High efficiency in repetitive tasks
- Limitations: Cannot handle complex, dynamic workflows
Predictive modeling
- Current capabilities: Accurate predictions based on historical data
- Limitations: Dependent on accurate, unbiased data
"AI's current applications predominantly rely on narrow AI, or weak AI, which excels in performing specific tasks—such as image recognition, natural language processing, and data analysis."
Understanding the limitations of AI
While AI has made substantial strides, it is not without its constraints. Despite popular belief, AI is far from replicating human cognition. Some key limitations of AI include:
- Lack of true understanding: AI systems, even the most advanced, lack human-like comprehension. They process vast amounts of data and identify patterns, but they don't "understand" in a way that humans do.
- Dependency on data quality: AI relies heavily on large datasets. If the input data is biased, incomplete, or of poor quality, AI's output will reflect these issues, often leading to inaccurate or misleading conclusions.
- Inability to reason beyond programming: AI operates within the constraints of its programming. It cannot think creatively or make decisions outside of the parameters set by its developers, limiting its application in scenarios that require out-of-the-box thinking.
- Ethical and privacy concerns: AI raises significant ethical questions, especially regarding privacy. As organizations collect more data to fuel AI models, concerns about how this data is used and who has access to it become more prominent.
- Lack of emotional intelligence: AI lacks the ability to understand and respond to human emotions, making it less effective in areas that require empathy, such as customer service or human resources.
These limitations are crucial for businesses to consider when integrating AI into their operations. Let’s delve deeper into each challenge and its implications.
1. Lack of true understanding: AI vs human cognition
At its core, AI processes information in ways that mimic human intelligence, but it doesn't possess genuine understanding. A computer can be trained to recognize images of cats or dogs, but it does not "know" what a cat or dog is. It simply analyzes patterns within data to make predictions. This is fundamentally different from how humans learn and understand the world around them.
- Why it matters: AI's inability to grasp context can lead to errors in areas where nuanced understanding is required, such as legal analysis or medical diagnoses.
- Real-world example: In legal tech, AI can analyze contracts for clauses, but without human oversight, it may miss context or nuance that could lead to significant legal ramifications.
This lack of understanding poses a major limitation, especially in industries where decision-making is complex and multifaceted.
2. Dependency on data quality: garbage in, garbage out
AI systems are only as good as the data they are trained on. If the data used to train an AI model is flawed, the system will produce equally flawed results—a concept known as “garbage in, garbage out.”
- Implications: Poor-quality data can introduce biases into AI models, leading to skewed or unethical outcomes. For example, if an AI system used for hiring is trained on biased historical data, it may perpetuate gender or racial biases.
- Data-driven decision-making: AI's effectiveness hinges on access to vast, high-quality datasets. However, gathering such data can be challenging, especially for smaller organizations with limited resources.
Businesses must invest in high-quality data collection and management practices to ensure that AI outputs are accurate and reliable.
3. Inability to reason beyond programming: limits of creativity
Creativity and flexibility are hallmarks of human intelligence, but AI lacks the ability to reason beyond its programmed constraints. While AI can solve complex problems and automate routine tasks, it is confined by the instructions it is given.
- Challenges: AI cannot engage in creative problem-solving or adapt to novel situations without explicit programming. This limits its use in fields requiring innovation, such as research and development (R&D) or strategy planning.
- Case study: In marketing, AI can analyze data to optimize ad placement but cannot generate a creative advertising campaign that resonates emotionally with customers.
For businesses that rely on innovation and adaptability, AI's rigidity is a notable drawback.
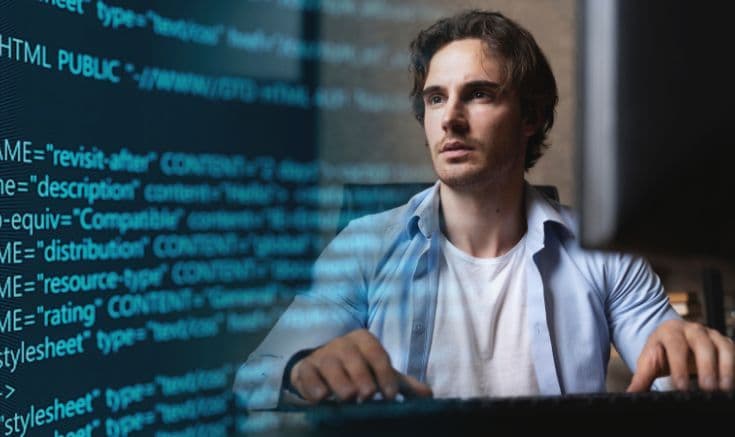
4. Ethical and privacy concerns: managing AI responsibly
As AI systems grow more pervasive, ethical concerns become harder to ignore. AI's capacity to collect and analyze personal data raises significant privacy issues, and its deployment in decision-making can lead to unintended biases.
- Privacy issues: AI systems that analyze consumer behavior, for instance, often gather sensitive data that could be misused if not properly managed.
- Bias in AI: AI systems have been known to produce biased outcomes, especially when trained on historical data that reflects societal biases. This has led to controversy in sectors like law enforcement, where AI tools have been used to predict criminal behavior, often leading to discriminatory outcomes.
5. Lack of emotional intelligence: limits in human interaction
Another critical limitation of AI is its inability to understand or respond to human emotions. In industries where empathy is essential, such as healthcare or customer service, AI falls short. Chatbots, for instance, can handle routine queries but often struggle with more complex emotional interactions that require human understanding.
- Implication: AI-driven customer service solutions may be effective for answering straightforward questions, but they are less successful in resolving customer complaints that require empathy and emotional intelligence.
- Case study: In healthcare, AI can assist in diagnosing diseases, but it cannot provide the emotional support that patients often need when facing difficult diagnoses.
Lack of true understanding
- Description: AI can process data but lacks the ability to "understand" like humans.
- Business impact: Limits AI's effectiveness in areas requiring complex decision-making.
Dependency on data quality
- Description: AI relies on high-quality data to function accurately.
- Business impact: Poor data leads to inaccurate predictions and biased outcomes.
Inability to reason creatively
- Description: AI operates within predefined parameters and lacks creative problem-solving.
- Business impact: Restricts AI's use in innovation-driven industries.
Ethical and privacy concerns
- Description: AI's use of data raises significant ethical and privacy issues.
- Business impact: Increases the need for robust data governance and ethical oversight.
Lack of emotional intelligence
- Description: AI cannot process or respond to human emotions effectively.
- Business impact: Limits its role in customer service or other human-centric roles.
Challenges of AI
Despite AI’s impressive capabilities, it faces several challenges that inhibit its widespread adoption and effectiveness. These challenges span technical, ethical, and operational dimensions, making AI a tool that requires careful management and oversight. In addition to ethical concerns and resource demands, the limitations of artificial intelligence also impact its ability to perform in dynamic environments.
Key challenges:
- Data privacy and security: AI systems rely on large datasets, raising privacy issues. Companies must comply with regulations like GDPR.
- Bias and fairness: AI can perpetuate biases present in its training data, leading to unfair outcomes, especially in recruitment and financial services.
- Interpretability: AI models often operate as black boxes, making it hard for users to understand decision-making processes.
- Resource intensiveness: AI systems require significant computational power, which increases energy costs and environmental impact.
Gartner predicted in 2018 that 85% of AI projects will fail to deliver their intended outcomes due to issues such as data bias and improper algorithms. Addressing these challenges requires a balanced approach, integrating AI with human oversight to ensure ethical and effective use.
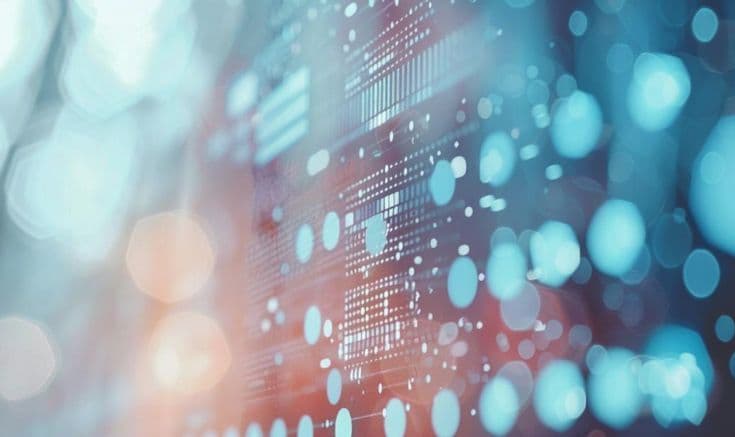
Overcoming the limitations of AI
Although AI has clear limitations, researchers and businesses are developing methods to mitigate these shortcomings. By employing emerging technologies and refining existing methodologies, AI can become more robust, interpretable, and ethically sound. Below are strategies that organizations can adopt to overcome some of AI's current limitations:
"AI's limitations do not diminish its value, but they do underscore the importance of understanding where AI fits best."
- Explainable AI (XAI): One of the most promising advancements is the development of explainable AI (XAI). XAI aims to make AI models more transparent by providing understandable explanations for AI decisions. This is critical in sectors like healthcare and finance, where accountability is essential.
- Human-AI collaboration: Rather than viewing AI as a replacement for human intelligence, organizations should focus on using AI to complement human expertise. A hybrid approach balances AI's data-driven capabilities with human creativity and emotional intelligence.
- Enhanced data governance: Implementing strong governance frameworks is key to ensuring that AI systems are built on high-quality, unbiased data, thus mitigating risks related to bias and inaccuracies.
- AI regulation and ethical frameworks: Governments and organizations are working to develop regulatory frameworks that guide the ethical use of AI. These frameworks will be crucial in areas such as data privacy and algorithmic fairness.
- Continuous learning systems: Unlike static AI systems, continuous learning models evolve based on new data, making them more adaptable and effective over time.
- Hybrid models: Combining AI with rule-based systems can help address ethical and legal reasoning issues, especially in industries where compliance and transparency are critical.
Artificial intelligence is undeniably a powerful tool that has transformed industries and will continue to shape the future of business. However, it is crucial to understand that AI has limitations that organizations must consider when implementing AI-driven solutions. These limitations, from data dependency and privacy concerns to biases and lack of creativity, highlight the need for a balanced approach that combines human oversight with technological innovation.
AI's limitations do not diminish its value, but they do underscore the importance of understanding where AI fits best. By recognizing these boundaries and leveraging emerging solutions like explainable AI and continuous learning systems, organizations can maximize the benefits of AI while mitigating its risks.
Common questions about AI limitations
What are the main limitations of AI in business?
How can explainable AI (XAI) improve AI adoption?
What are the ethical concerns associated with AI?
How can businesses overcome AI's limitations?
Can AI replace human creativity and problem-solving?
Why is data quality important for AI?
Want to learn how artificial intelligence can bring more transparency and trust to your operations?