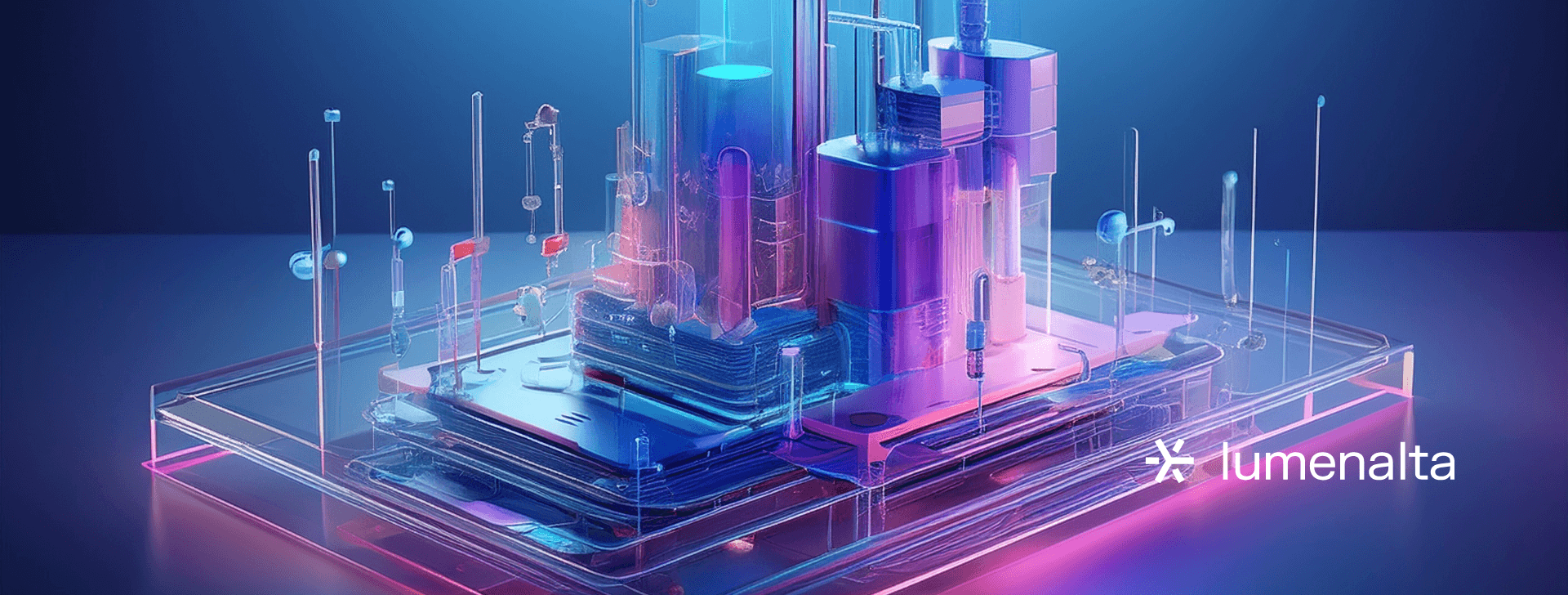
5 AI tools for front end development in 2025
APR. 27, 2025
3 Min Read
AI-based frameworks in front end development have redefined how user interfaces are built and maintained.
It is now possible to automate design checks, predict user behaviors, and streamline testing phases with minimal manual intervention. These advancements shift the conversation from tedious coding tasks toward strategic, revenue-driving enhancements. The results often include stronger user engagement, faster time to market, and measurable benefits for businesses seeking scalable solutions.
key-takeaways
- 1. Predictive ui personalization enhances user journeys with tailored experiences.
- 2. Intelligent code suggestions free developers to work on strategic features.
- 3. Automated design validation preserves brand consistency with minimal rework.
- 4. Conversational interfaces offer scalable engagement that reduces support overhead.
- 5. AI-based testing suites expedite release cycles and reduce production incidents.
5 essential AI tools enhancing front-end development
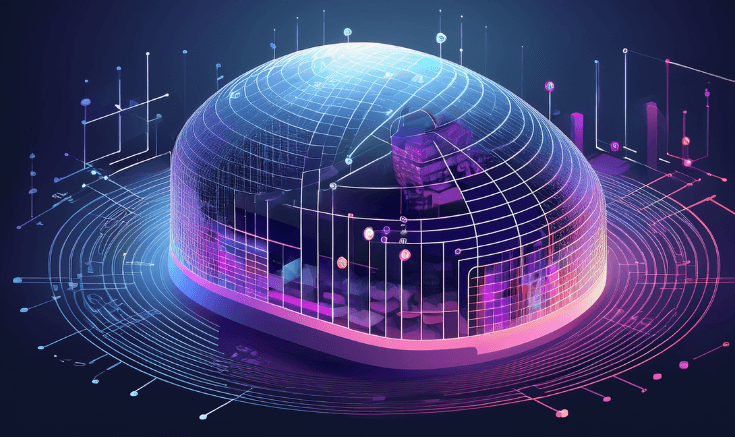
1. Predictive UI personalization
Developers searching for improved user satisfaction often start by integrating predictive UI personalization into their projects. This technique relies on data analytics and machine learning to suggest layouts, color schemes, and interactive elements that resonate with specific segments. A front-end developer AI tools suite with personalization capabilities can help anticipate customer preferences, cutting down on guesswork. Clear analytics dashboards translate these user preferences into actionable insights, ensuring that teams can balance cost-effectiveness with consistent design.
Teams benefit from immediate feedback on trends, which supports faster cycle times for new features. Personalized components also boost conversion rates, since visitors see custom elements that match their interests. Efficient personalization is more than a convenience; it helps front-end teams avoid overbuilding or overspending on features that lack appeal. Investing in predictive UI personalization can elevate brand impact and reduce wasted effort, making it easier to present a strong business case to stakeholders.
2. Intelligent code suggestions
Developers who want an automated approach to writing boilerplate code often turn to intelligent code suggestions. This category of AI tools for front-end developer tasks scans existing code bases, learns patterns, and offers snippets or entire functions that can be integrated directly into a project. The process saves time by eliminating repetitive tasks, which can be a key factor when aiming for quick time to value. Thoroughly tested code recommendations also enhance quality, reducing mistakes that would otherwise surface in production.
Implementation is user-friendly, especially when integrated into popular IDE (integrated development environment) platforms. A well-trained suggestion engine observes patterns in your unique project, rather than forcing you to adopt generic solutions. This approach supports adaptability because it leaves room for custom logic that aligns with business needs. Over the long term, intelligent code suggestions reduce manual overhead and allow professionals to focus on strategic improvements rather than mundane coding tasks.
3. Automated design validation
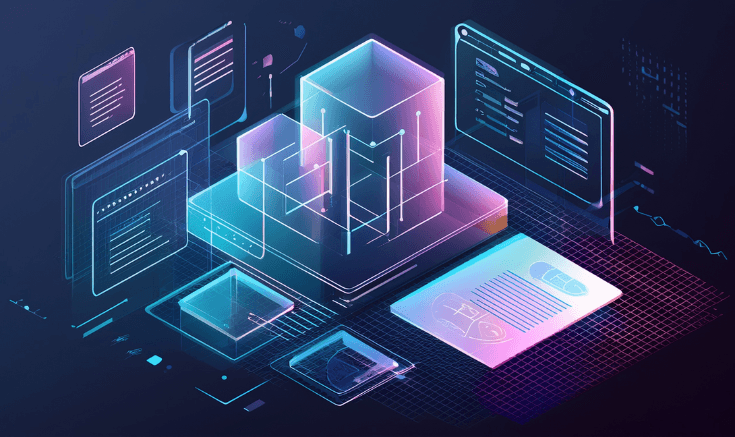
Teams that handle large-scale projects often use automated design validation to ensure brand consistency and maintain a polished user experience. This category of AI tools for front-end developer projects can interpret style guides or design mockups, then check the code base for alignment with those guidelines. Thorough validation routines confirm that spacing, typography, and responsive layouts match the intended blueprint. Design drift becomes less likely, which leads to cost savings by avoiding last-minute rework.
Accuracy in design consistency contributes to better stakeholder satisfaction, since visual elements remain cohesive across devices. These tools often include real-time feedback on code changes, helping developers refine layouts without lengthy revision cycles. As front-end demands become more complex, automated validation reduces the risk of launching a mismatched user experience. Teams gain a structured approach to design governance, improving trust among decision-makers who want a clear path to measurable business results.
4. Conversational interfaces
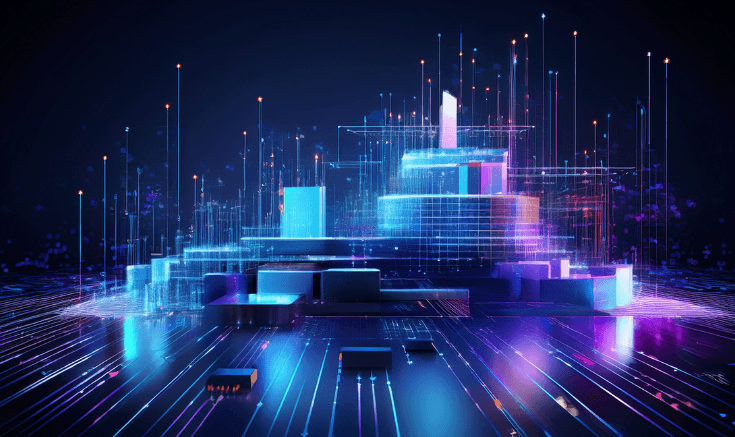
The process of embedding chat capabilities and voice-powered features can require significant effort, but conversational interfaces powered by an AI tool for front-end development simplify this task. Implementation involves plugging an AI-powered engine into chat widgets, voice interfaces, or interactive modules. This solution supports more intuitive interactions, which aligns with the rising demand for seamless user engagement. Business leaders appreciate the scalable potential of conversational interfaces because they can reduce manual support requirements.
Development time can be shortened since chatbots and voice features draw from pre-built AI models that recognize and respond to common queries. Front-end professionals can integrate these modules into existing workflows, freeing their attention for complex tasks such as data visualization or system interoperability. Many solutions offer robust analytics features to measure how users interact with the interface, generating insights that inform ongoing improvements. Introducing conversational interfaces can attract new audiences while boosting satisfaction among existing customers.
5. AI-backed testing suites
A front-end developer who juggles multiple frameworks may find that an AI-backed testing suite can detect functional and performance issues more efficiently than manual testing alone. These suites apply algorithms to pinpoint anomalies and predict potential failure points, supporting risk mitigation. Automated test scripts also help maintain consistency, which is essential for teams that operate at scale. Speed to market becomes easier to achieve because the most time-consuming parts of testing can be offloaded to an AI solution.
Predictive analytics within these suites highlight areas where breakage is likely, giving teams the option to focus on specific code blocks. That approach leads to higher quality deliverables with fewer production incidents. The result is an end-to-end workflow that addresses complex front-end scenarios without dragging down timelines. An AI-based testing suite underscores proactive quality assurance, reinforcing confidence in every release and improving returns for your organization.
“Teams benefit from immediate feedback on trends, which supports faster cycle times for new features.”
Challenges of integrating AI tools in front-end development
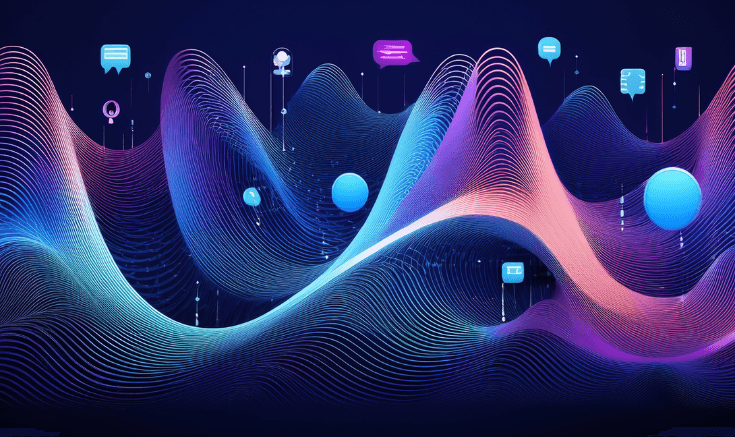
There are several hurdles that can slow progress or create unforeseen issues when incorporating an AI tool for front-end development. Teams sometimes encounter hidden costs, tech constraints, or internal resistance due to a lack of clarity around best practices. Identifying these potential pitfalls early helps create a smooth path to scalable AI integration. It also provides an opportunity to weigh priorities and ensure strong alignment among stakeholders.
- Data complexity: Existing databases might not have the clean, structured information needed for AI training.
- Model accuracy: Predictions or suggestions can produce errors when trained on incomplete or outdated data.
- Ethical considerations: Handling user data requires consistent adherence to privacy guidelines and regulatory requirements.
- Resource constraints: Legacy systems may demand significant reconfigurations before AI solutions can be deployed effectively.
- Skill gaps: In-house teams might need specialized training to manage advanced functionalities or interpret analytics.
Preparation can reduce the impact of these challenges and lead to a more effective front-end strategy. Evaluating tools based on your team’s abilities and your organization’s goals remains an essential part of any AI roadmap. Stakeholders who understand the scope of these potential obstacles are less likely to hesitate mid-implementation. Teams that plan thoroughly are in a strong position to adapt and secure long-term benefits.
“Identifying these potential pitfalls early helps create a smooth path to scalable AI integration.”
Strategies for effectively implementing AI tools in front-end projects
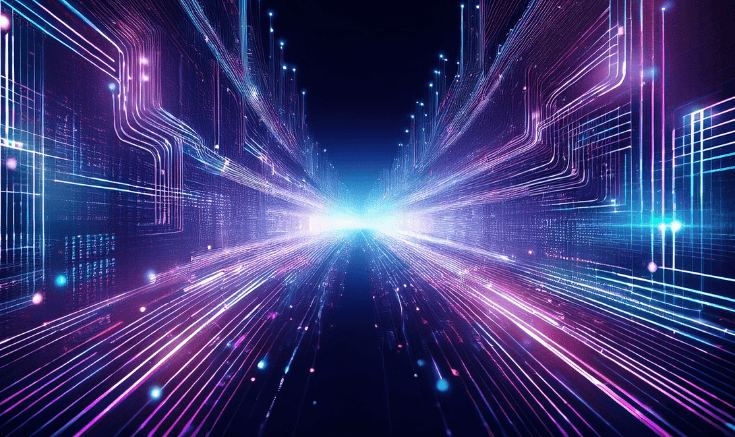
Seamless AI integration calls for careful coordination between developers, product managers, and business sponsors. Clear objectives help define the scope of each AI tool for front-end development, reducing the likelihood of wasted resources. Structured data or schema markup can improve how AI systems read and interpret information, leading to more accurate results. Incremental rollouts can preserve stability by allowing teams to observe outcomes and refine processes before scaling further.
A holistic approach aligns technology choices with measurable business outcomes. Thorough cost analyses can determine if a front-end developer AI tools solution justifies the investment, including any training or infrastructure upgrades. Tools that reduce time spent on maintenance or repeated tasks can accelerate overall project velocity, freeing teams to innovate in other areas. This balance between operational efficiency and user-centric features leads to a more effective and flexible development cycle.
Align AI solutions with specific use cases
Teams aiming for success often start by assigning AI capabilities to tasks that show immediate ROI. Examples include automated code reviews, design validations, or predictive content recommendations that enhance user engagement. This selective approach prevents feature bloat, helping you avoid overreliance on AI in areas that may not deliver significant returns. Focused use cases can also serve as proof-of-concept wins, making it easier to gain buy-in from stakeholders who prioritize tangible outcomes.
Maintain a culture of continuous learning
Developers benefit from regular workshops and resource-sharing sessions that highlight new techniques or emerging technologies. Open communication leads to broader awareness of how AI can simplify daily responsibilities or open new business opportunities. Training sessions and knowledge sharing contribute to greater trust in the toolset, which shortens feedback loops on new features. Over time, these efforts cultivate a setting where AI enhancements feel like a natural extension of the existing development process.
Evaluate performance metrics consistently
Every implementation benefits from consistent analysis of speed, usability, and design compliance. Tracking these metrics offers insights on how well AI solutions match project goals, guiding data-powered decisions. Dashboards and performance monitoring systems offer the visibility needed to adapt strategies when results begin to deviate from expectations. This vigilance ensures that both short-term and long-term benefits remain measurable, backing up claims of efficiency gains with clear evidence.
AI in front-end development opens up faster, more flexible growth without compromising user satisfaction. Deploying these capabilities can accelerate returns and reduce friction, keeping teams focused on what truly matters: achieving tangible outcomes. At Lumenalta, we support businesses with tailored digital solutions that align seamlessly with core objectives, ensuring every front-end transformation delivers measurable gains. Let’s chart a brighter path forward with front-end AI strategies that drive progress.
table-of-contents
- 5 essential AI tools enhancing front end development
- 1. Predictive UI personalization
- 2. Intelligent code suggestions
- 3. Automated design validation
- 4. Conversational interfaces
- 5. AI-backed testing suites
- Challenges of integrating AI tools in front end development
- Strategies for effectively implementing AI tools in front end projects
- Common questions about AI tools for front end development
Common questions about AI tools for front end development
What are the benefits of using an AI tool for front end development?
Are AI tools for front end developer tasks difficult to maintain?
How do front end developer AI tools impact project budgets?
Will automated testing suites replace manual testing?
How does AI improve user satisfaction in front end interfaces?
Want to learn how AI-led automation can lower costs and increase trust to your operations?