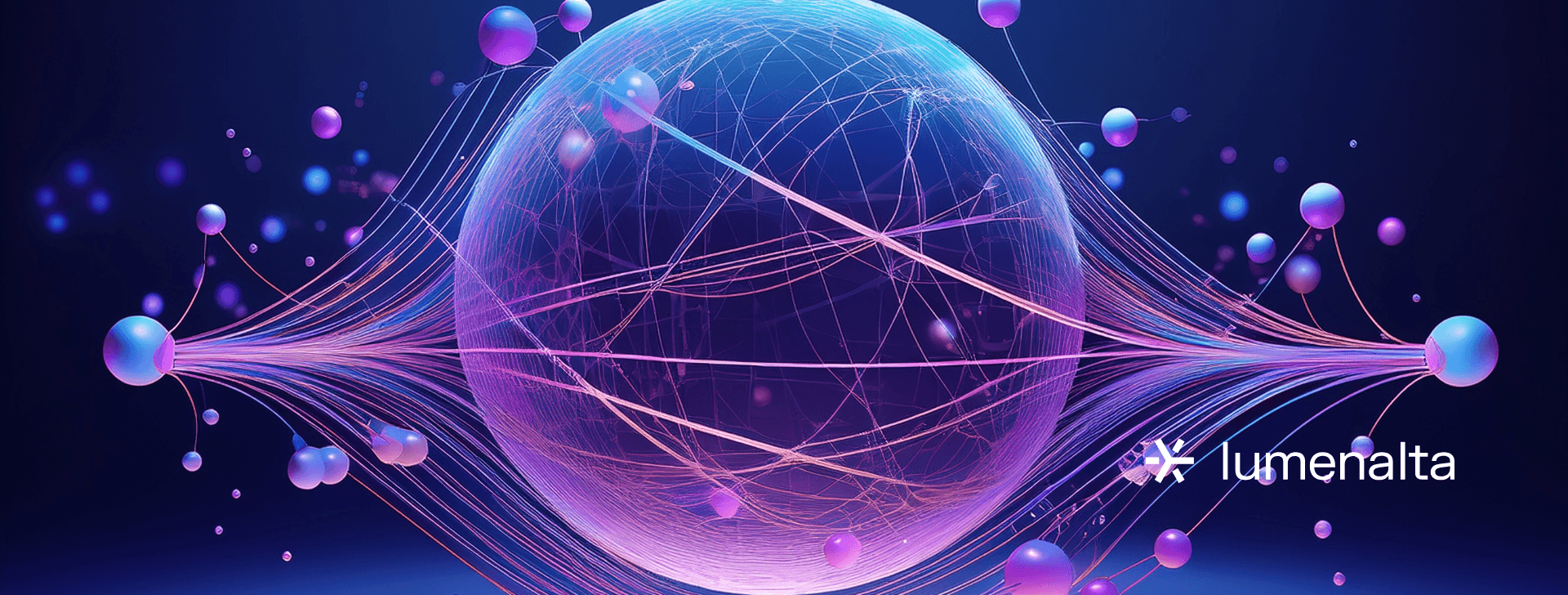
Understanding AI personalization in 2025
APR. 4, 2025
7 Min Read
Smart recommendations that grow with your customers’ unique habits save costs and fuel long-term loyalty.
AI personalization upgrades every touchpoint, giving you the power to adjust products or services on the fly based on real-time analytics. This approach highlights the importance of user data, cloud-native tools, and collaborative planning to drive ROI. Many organizations see that a targeted personalization AI strategy improves outcomes for both internal teams and end users alike.
key-takeaways
- 1. AI personalization relies on real-time analytics to craft meaningful customer interactions.
- 2. Tailored recommendations drive loyalty, expand revenue, and optimize resource spending.
- 3. Privacy compliance, data accuracy, and robust systems are vital for stable operations.
- 4. Iterative pilots and feedback loops confirm an approach’s viability before large-scale rollouts.
- 5. Forward-thinking stakeholders invest in predictive and context-aware personalization methods.
Understanding AI personalization
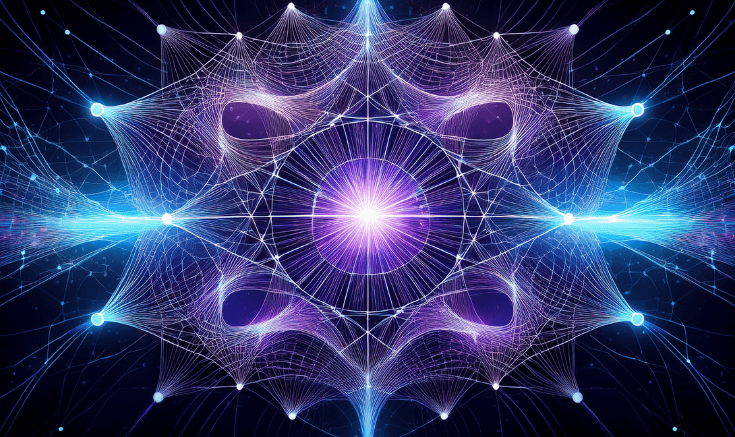
Artificial intelligence personalization refers to the real-time adaptation of products, services, or experiences based on individual user data and predictive analytics. It draws on tools such as machine learning (ML) models and big data to shape messages or recommendations that resonate with specific audiences. This approach moves beyond superficial targeting to deliver customized interactions that align with each user’s behavior and preferences. Decision-makers in sectors like financial services or logistics rely on this level of insight to reduce costs, boost engagement, and maintain steady growth.
Effective personalization with AI emerges from systems that monitor diverse data points such as past transactions, browsing behaviors, and location-based preferences. Teams develop user profiles that guide content strategies, product adjustments, or marketing initiatives. These methods often involve cloud-based platforms that scale as data volumes rise, ensuring uninterrupted processing. Many organizations accelerate implementation by collaborating with specialists who provide frameworks for integrating personalization capabilities into existing workflows.
“Organizations gain crucial advantages when personalization with artificial intelligence is prioritized.”
Why AI in personalization is important in 2025
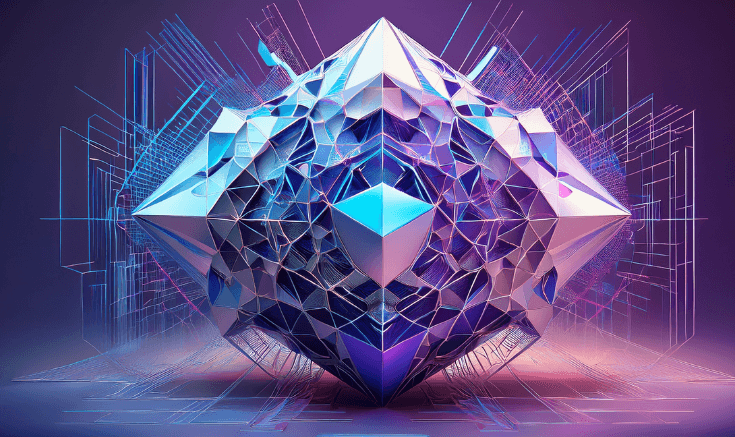
User expectations for individualized recommendations are rising, and outdated strategies that rely solely on demographics no longer suffice. Many organizations see that AI in personalization optimizes every customer interaction, which heightens loyalty and unlocks cross-selling possibilities. This growing demand for AI personalization is particularly visible in settings like retail, where tailored promotions improve revenue, or healthcare, where customized care plans increase patient satisfaction.
Time to value is another key driver. Projects that once required extended development cycles now benefit from agile integration with cloud services and third-party APIs. This reduces overhead and showcases immediate progress, an essential advantage for business decision-makers looking to streamline costs. AI and personalization tactics, especially when combined with robust governance practices, empower stakeholders to validate results early and refocus resources on the approaches that generate the highest ROI.
Key benefits of AI personalization
Organizations gain crucial advantages when personalization with artificial intelligence is prioritized.
- Enhanced customer loyalty: Providing each user with timely, relevant offerings increases retention rates.
- Measurable revenue growth: Suggestions powered by AI for personalization often guide users to products or services they are most likely to acquire.
- Faster time to market: Rapid analysis of user profiles guides development teams to deploy new features that align with real-time user feedback.
- Greater operational efficiency: Automated data analysis reduces manual work, allowing your workforce to focus on strategic projects.
- Streamlined decision processes: Real-time insights guide crucial choices on product roadmaps or campaign budgeting with evidence-based data.
- Improved cost-effectiveness: Access to data-based metrics ensures resources are distributed in ways that minimize wasteful spending.
Many organizations notice that these improvements go beyond one-time gains. AI personalization often triggers a culture shift, where teams become more aware of data value and continuously refine their engagement strategies.
Types of AI personalization across industries
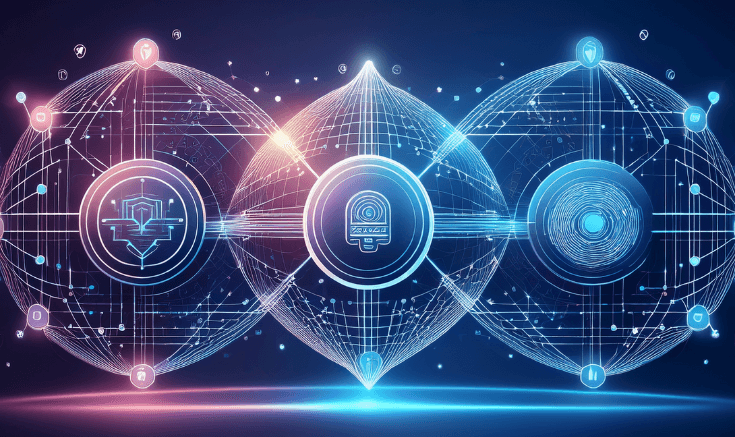
Different sectors implement personalization in AI to address unique user challenges. Real-time data insights guide how finance, healthcare, retail, or logistics organizations fine-tune their approaches.
Healthcare
Providers use personalization with AI to ensure that patients receive treatment plans tailored to their unique medical background. Automated alerts also suggest timely check-ups or preventive tests based on an individual’s health profile. This approach increases patient trust, especially when sensitive information is managed with strong security protocols. In many cases, personalized dashboards help both practitioners and patients track progress, anticipate potential concerns, and reduce readmission rates.
Retail
Omnichannel strategies with artificial intelligence personalization deliver targeted recommendations, loyalty perks, or dynamic pricing. E-commerce platforms track which items a user browses and then push tailored offers that align with browsing habits. This helps retailers move surplus inventory while giving shoppers a sense of exclusivity. Many organizations combine in-store data with online interactions, creating a 360-degree view of customers that can drive consistent experiences.
Financial services
Credit card providers, banks, and insurance firms adopt AI-powered personalization to refine how they present services to different segments. Some institutions use predictive analytics to match customers with the right credit or loan product at the correct time. Tailored financial tips or insights can also help clients improve their spending habits, enhancing brand loyalty. Fraud detection frameworks incorporate AI for personalization by differentiating between typical client activity and unusual patterns that demand further review.
Logistics
Operators in the supply chain and distribution track real-time order data to provide accurate delivery updates, packaging suggestions, or route optimizations. AI-powered personalization in this sphere might involve dynamic scheduling of drivers to address sudden spikes in demand. Managers also see cost savings when fleets are allocated efficiently based on known delivery patterns. Many find that this type of personalization reduces delays and fosters stronger client relationships.
Common challenges of implementing AI personalization
Adopting AI-based personalization can present hurdles that affect both technical and organizational readiness.
- Data quality and governance: Poorly managed data sets introduce errors into personalized outcomes.
- Integration with legacy systems: Existing infrastructures often lack the flexibility required for seamless AI deployment.
- Privacy and regulatory requirements: Personalization with AI must comply with rules that vary by region or industry.
- Talent and skill gaps: Specialist knowledge is needed to build, train, and maintain personalization AI models.
- Organizational alignment: Unclear objectives lead to conflicting priorities among teams.
- Scaling complexities: Memory, processing power, and data storage become expensive if not carefully planned.
These challenges can slow time to value if left unaddressed. Streamlined integration efforts, backed by a transparent roadmap, frequently resolve or minimize these issues.
Best practices for integrating AI personalization into your business
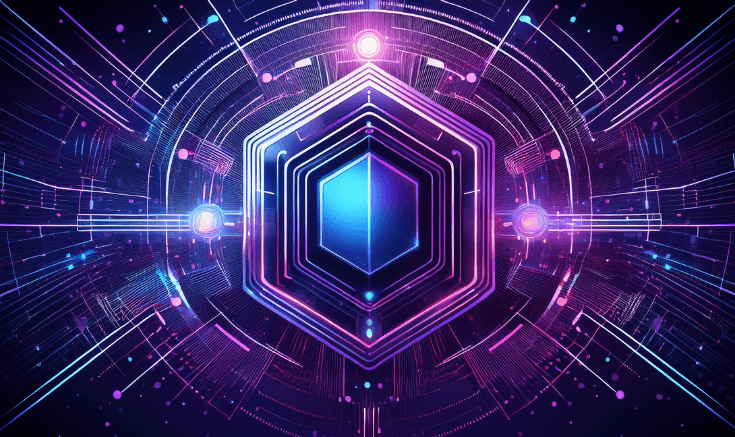
Leaders seeking consistent outcomes often combine methodical planning with user-focused design. Many teams gather cross-functional input early to minimize rework and facilitate smoother adoption. In many cases, an incremental rollout approach is more effective than a massive overhaul, allowing stakeholders to see measurable improvements sooner and refine future steps.
Design pilot programs
Focused pilot programs help verify whether your AI for personalization strategy aligns with user needs. Data scientists and frontline staff collaborate to define success metrics and gather feedback on initial releases. This process often reveals unanticipated system requirements or user behaviors that inform subsequent development. Pilots also offer a quick way to prove value to executive sponsors who care about speed to market.
Implement robust data strategies
Collection, cleansing, and analysis must follow consistent standards. Systems that unify data points across channels remove silos and reduce duplication. Security protocols, including encryption or tokenization, protect sensitive information while maintaining performance. Solid data management practices drive reliable outcomes across personalization and AI initiatives.
Maintain agile feedback loops
Teams that adopt short release cycles see faster results and can adapt to changes in user preferences. Frequent reviews with stakeholders prevent resource misallocation and confirm that each iteration meets defined goals. Decision-makers also appreciate the clarity that comes from frequent progress updates, which support more precise budgeting. This loop keeps your AI personalization strategy agile and grounded in real-time user data.
Measuring the impact of AI personalization on customer engagement
Quantitative data such as click-through rates, user time on the platform, or average purchase value indicate how personalization in AI strategies affects user decisions. Many organizations also track more nuanced metrics like churn reduction or the average time between repeat purchases. These insights help refine campaigns, product lines, or even user experience design.
Qualitative feedback is equally important. Surveys, focus groups, or social media monitoring reveal how customers feel about a brand’s personalization efforts. When combined with quantitative measures, these insights paint a comprehensive picture of the impact of AI personalization on engagement. Teams then identify which features to sustain, optimize, or discontinue based on data-backed evidence.
“Quantitative data such as click-through rates, user time on the platform, or average purchase value indicates how personalization in AI strategies affects user decisions.”
Future trends in AI personalization
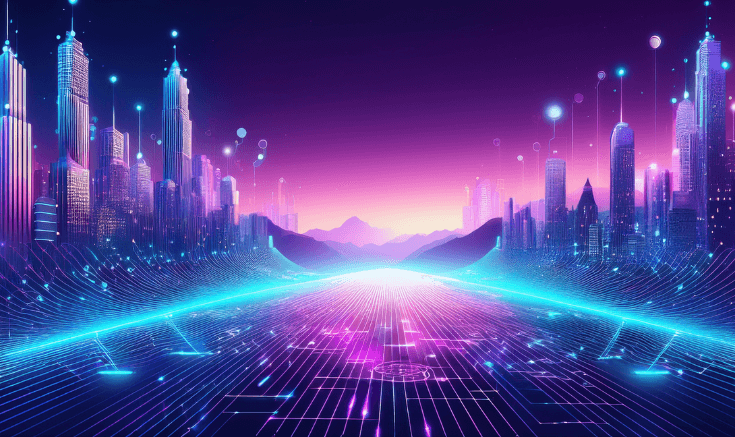
Ongoing progress in machine learning, sensor technology, and data analytics signals a rising need for advanced personalization methods.
- Context-aware recommendations: Systems that automatically adjust offers based on location or recent transactions.
- Voice and speech interactions: Personalization that understands tone or sentiment to tailor responses.
- Adaptive interfaces: User interfaces that shift layout or function based on historical preferences and usage patterns.
- Predictive service scheduling: Timely alerts that suggest when maintenance or additional products may be required.
- Cross-channel brand consistency: AI ensures seamless experiences across mobile apps, websites, and in-person interactions.
Technologies that facilitate these trends often integrate with existing AI frameworks, allowing faster adoption. Stakeholders who dedicate resources to these emerging innovations see strategic benefits, from cost savings to better customer satisfaction.
Long-term success in AI personalization requires a clear vision that aligns with strategic goals. Many organizations formalize iterative cycles to analyze data, enhance ML models, and expand personalization initiatives incrementally. This process reduces the risks associated with sudden, large-scale changes and keeps teams focused on delivering measurable business impact.
Alignment with leadership, effective training programs, and transparent collaboration set the tone for sustainable outcomes. Analysts and product owners often work closely with finance and operations to connect personalization metrics to ROI. The most successful teams continue refining their personalization approach, ensuring that they remain prepared for shifts in user expectations or operational priorities.
AI personalization fosters experiences that match the exact desires of your target audience and strengthens relationships at every step. Aligning the right people, processes, and technology leads to sharper insights, cost savings, and a more resilient business strategy. At Lumenalta, we specialize in crafting solutions that blend artificial intelligence with user-focused design, always keeping your success in mind. Let’s chart a brighter path together.
table-of-contents
- Understanding AI personalization
- Why AI in personalization is important in 2025
- Key benefits of AI personalization
- Types of AI personalization across industries
- Common challenges of implementing AI personalization
- Best practices for integrating AI personalization into your business
- Measuring the impact of AI personalization on customer engagement
- Future trends in AI personalization
- Common questions about AI personalization
Common questions about AI personalization
What is AI personalization, and how does it differ from traditional methods?
Does personalization in AI require large data sets?
Why is AI and personalization so critical for cost optimization?
How can AI for personalization stay compliant with privacy rules?
How quickly can you measure success with AI-based personalization?
Want to learn how personalization can bring more transparency and trust to your operations?