The future of data governance: 10 trends shaping AI and ML integration
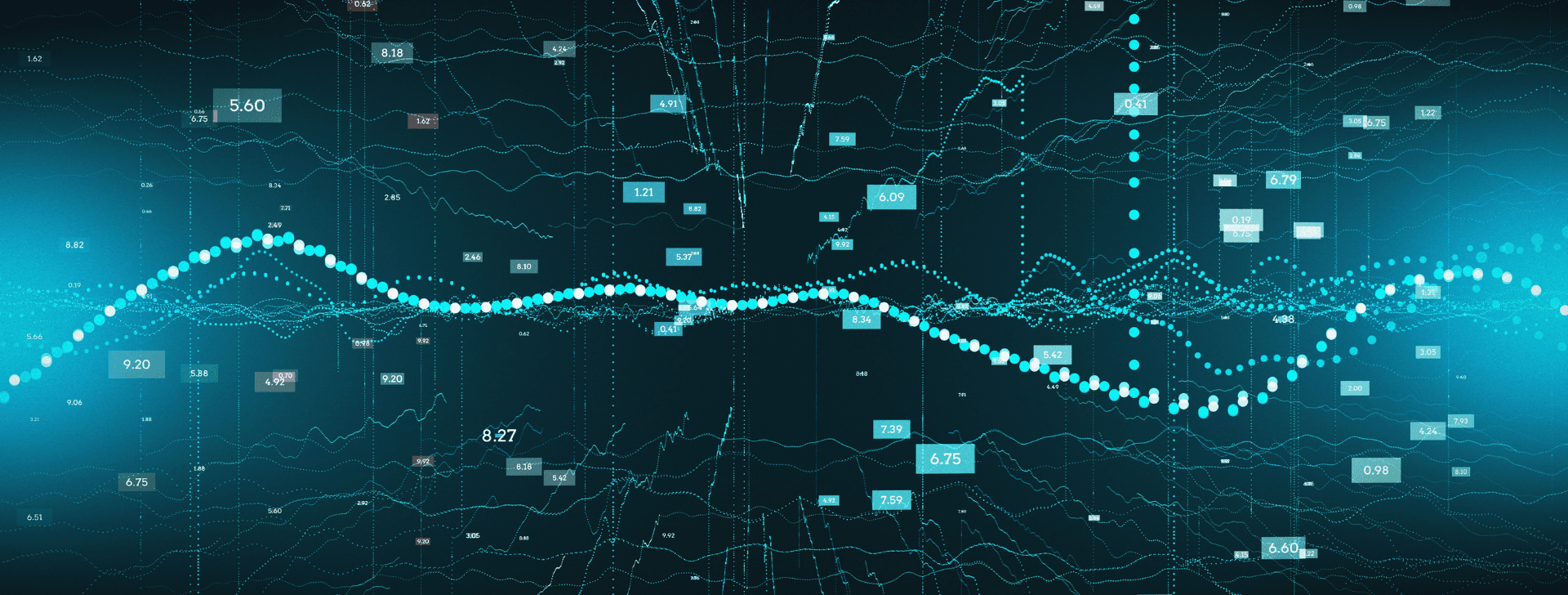
OCT. 11, 2024
Data governance frameworks must evolve to meet the demands of technologies, as artificial intelligence (AI) and machine learning (ML) continue to gain traction.
AI is rapidly transforming data management, with 96% of organizations reporting improved data quality and consistency. As AI adoption accelerates, effectively leveraging these technologies is essential for businesses seeking to maintain a competitive edge.
Let's explore the key trends driving the integration of AI and ML into data governance, offering insights into the strategies that will enable more effective data management and ensure long-term success.
Key takeaways
- 1. AI enhances data quality: AI-driven tools significantly improve data accuracy, consistency, and reliability, directly boosting decision-making capabilities.
- 2. Machine learning simplifies compliance: Integrating ML into compliance frameworks automates tasks, reduces costs, and ensures regulatory adherence.
- 3. Real-time data governance is essential: Organizations benefit from immediate insights through real-time data processing, which supports fast and accurate decisions.
- 4. Data ethics and transparency are vital: Embedding ethical principles in AI ensures responsible data use, transparency, and alignment with societal values.
- 5. Cloud and AI integration: Cloud-based solutions provide scalable and secure data governance, supporting AI-driven innovation and reducing infrastructure costs.
1. Emphasis on AI-driven data quality improvement
Data quality remains a cornerstone of AI and ML effectiveness, as even the most advanced models can falter without reliable data. AI-driven data governance focuses on enhancing the accuracy, consistency, and integrity of data, laying the foundation for high-quality analytics and decision-making. With growing data volumes and complexity, leveraging AI to monitor and refine data quality has become essential for organizations aiming to maximize the value of their AI investments.
- 96% of organizations have observed enhanced data quality and consistency by integrating AI into their data management systems.
- AI-driven tools can detect patterns and anomalies in real time, proactively addressing data quality issues before they impact decision-making.
- Automated data cleansing processes powered by AI help organizations reduce human error, ensuring that data remains consistent and reliable across the board.
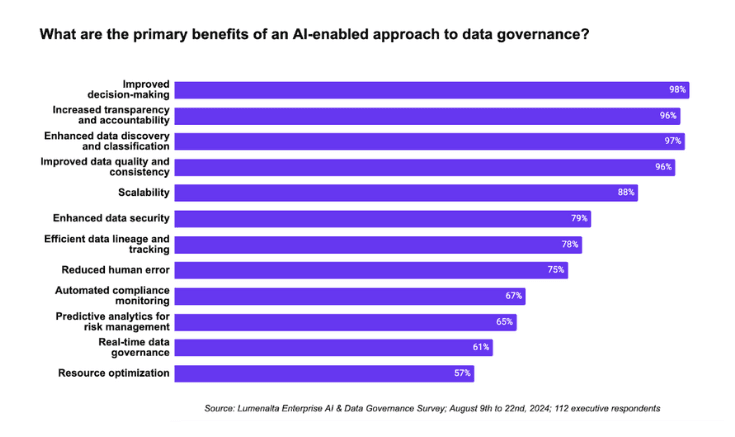
"Data quality remains a cornerstone of AI and ML effectiveness, as even the most advanced models can falter without reliable data."
2. Integration of machine learning in automated compliance
Compliance with data regulations is becoming increasingly complex as businesses handle larger datasets and stricter data protection laws. ML integration plays a pivotal role in automating compliance processes, making it easier for organizations to meet regulatory requirements without overwhelming their teams. By leveraging ML, companies can stay agile and responsive to new regulations, ensuring they avoid costly penalties and maintain customer trust.
- Machine learning algorithms can sift through vast datasets to automatically identify sensitive information and ensure it is handled in line with compliance requirements.
- Integrating ML in compliance reduces the need for manual intervention, cutting down operational costs associated with monitoring and enforcement.
- Advanced ML techniques can predict potential compliance breaches, enabling organizations to act proactively rather than reactively.
The ability to automate compliance tasks with ML not only minimizes operational risks but also boosts efficiency, allowing data teams to focus on higher-value activities that drive strategic growth.
3. Real-time data governance: Meeting the demand for insights
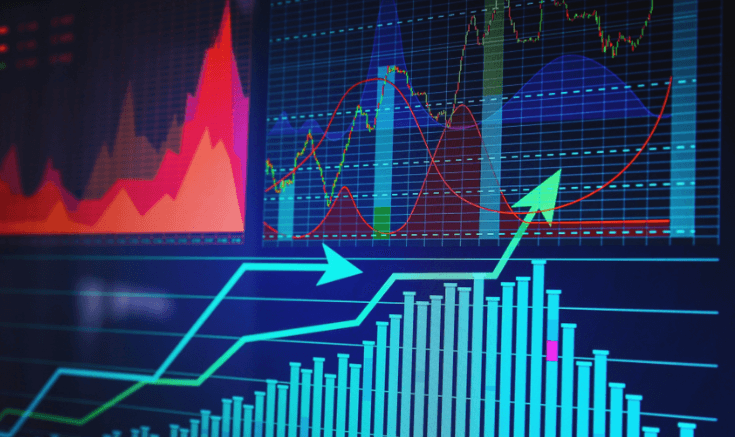
- Streaming data technologies allow continuous data flow, providing businesses with up-to-the-minute information crucial for fast-paced decision-making.
- AI-driven analytics can automatically flag issues or trends on the fly, helping organizations react promptly to new data.
- Timely data governance ensures seamless integration across multiple platforms, enhancing the accuracy and relevance of AI insights.
With responsive data governance in place, organizations can reduce latency in decision-making, improve operational responsiveness, and stay competitive in a rapidly evolving digital landscape.
4. Centralization vs. decentralization in data governance frameworks
Choosing between centralized and decentralized data governance models is a strategic decision that affects how data policies are implemented and enforced. While centralized models provide consistency and control, decentralized frameworks offer flexibility, allowing individual teams to tailor data management to their specific needs. As organizations increasingly adopt AI and ML technologies, the debate around centralization versus decentralization continues to evolve.
- Centralized data governance provides a uniform approach to data policies, ensuring consistency and minimizing compliance risks.
- Decentralized frameworks, such as data meshes, empower individual teams to manage data locally, fostering innovation and faster decision-making.
- Companies that blend both approaches can create a hybrid model, leveraging centralized policies for critical data while allowing decentralized teams to innovate.
Striking the right balance between centralization and decentralization ensures that businesses can maintain control over their data while enabling agile and innovative AI-driven solutions.
5. Enhancing data discovery and metadata management with AI
Data discovery and metadata management are increasingly becoming focal points of AI-driven data governance strategies. Effective data discovery ensures that organizations can quickly locate and utilize their data assets, while comprehensive metadata management facilitates accurate data classification and retrieval. AI significantly accelerates these processes, making data more accessible and actionable.
- AI tools can automatically generate and update metadata, ensuring that all data assets are accurately cataloged and easily searchable.
- Improved metadata aids in faster and more reliable data retrieval, supporting decision-making across the organization.
- Leveraging AI for metadata management helps maintain data accuracy and lineage, reducing the time and resources needed for manual updates.
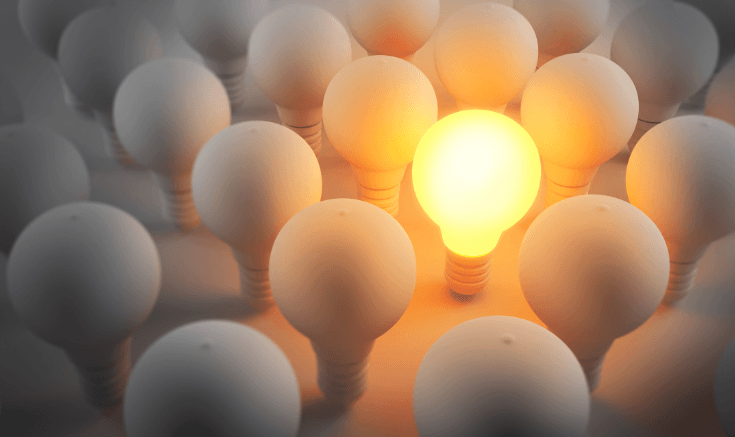
6. Data ethics and privacy regulations
With AI's growing influence, the focus on data ethics and privacy has intensified, driving the need for transparent and responsible data governance practices. Ethical considerations in AI involve minimizing biases, ensuring fair data usage, and respecting privacy rights, which are crucial for maintaining public trust and regulatory compliance. As regulations like GDPR and CCPA become stricter, data ethics is no longer just a best practice—it's a necessity.
- Ethical considerations in AI ensure that data is used responsibly, minimizing bias and discrimination in decision-making.
- Organizations must comply with privacy regulations like GDPR and CCPA to safeguard sensitive data and build user trust.
- Transparency in AI model operations and data handling practices is crucial for maintaining stakeholder confidence.
Embedding data ethics into AI practices not only helps avoid legal risks but also strengthens brand integrity, setting the foundation for sustainable AI adoption.
7. Cloud-based data solutions
Cloud technology has revolutionized data governance, providing scalable solutions that support AI and ML initiatives. By leveraging cloud-based data solutions, organizations can store, manage, and process large datasets more efficiently, facilitating faster AI-driven insights. These platforms also offer robust security features, ensuring data integrity and compliance in a cost-effective manner.
- Cloud platforms enable seamless data storage, access, and processing, facilitating real-time analytics and AI operations.
- Security protocols in cloud environments ensure that data remains protected against breaches and unauthorized access.
- With cloud-based tools, organizations can reduce infrastructure costs and focus more on innovation and AI advancements.
Investing in cloud-based data solutions enables organizations to build a future-ready infrastructure that supports their long-term AI and ML goals.
"A robust framework for measuring AI-driven data governance ROI enables continuous improvement and optimizes long-term business performance."
8. Investment in AI explainability and transparency
AI explainability and transparency are critical for building trust with stakeholders and meeting regulatory requirements. As AI models become more intricate, understanding how these systems make decisions is essential for accountability and ethical compliance. Organizations are increasingly turning to explainability tools to demystify AI processes and ensure they align with corporate values and societal expectations.
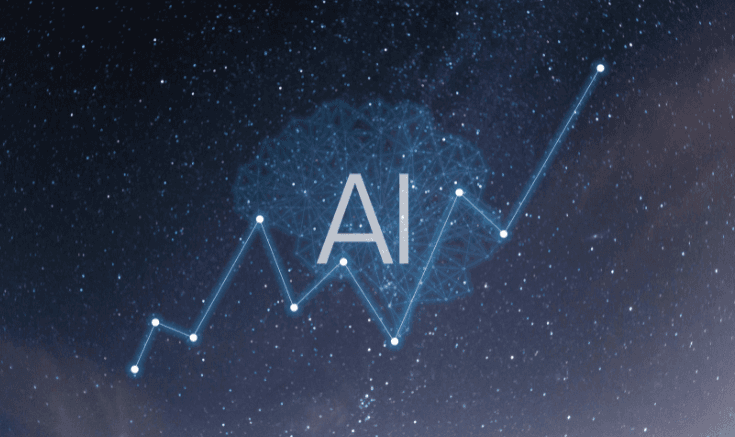
- AI explainability tools can break down complex models into understandable insights, aiding non-technical stakeholders.
- Investing in these tools helps ensure that AI decision-making processes are transparent and justifiable.
- Organizations that prioritize transparency are better positioned to meet regulatory standards and build consumer trust.
Fostering transparency in AI operations not only enhances compliance but also positions businesses as leaders in responsible AI innovation.
9. Adoption of data lineage and tracking technologies
Data lineage and tracking are crucial for understanding how data moves through systems, influencing AI model training and analytics accuracy. By implementing AI-driven lineage and tracking technologies, organizations can gain insights into data origin, transformations, and dependencies, which are vital for compliance and decision-making processes.
- Accurate data lineage helps trace the origin of data, track its transformations, and understand its current state.
- With AI-driven lineage tools, companies can detect inconsistencies and compliance issues faster and more accurately.
- Effective data lineage enhances data quality and supports reliable analytics, crucial for robust AI model training.
Understanding data lineage allows organizations to maintain data integrity, thereby improving the performance and reliability of AI-driven insights.
10. Measuring the ROI of AI-driven data governance
For organizations to sustain AI-driven data governance efforts, measuring the return on investment (ROI) is crucial. Quantifying the impact of AI on data management helps demonstrate the value of these technologies in reducing costs, enhancing decision-making, and improving compliance.
Our research highlights that 100% of organizations track data quality metrics, 92% measure cost savings, and 84% monitor compliance improvements. These metrics provide tangible evidence of AI's value in enhancing data governance processes and optimizing operational performance.
Businesses are increasingly focusing on key performance indicators (KPIs) to evaluate the success of their data governance strategies. Data quality metrics, cost savings from reduced manual processes, and compliance improvements are among the most important indicators of ROI. Tracking these outcomes not only justifies further investments but also helps ensure that AI efforts remain aligned with strategic goals.
By adopting a robust ROI framework, organizations can drive continuous improvement and optimize long-term performance. Monitoring KPIs such as data accuracy, compliance rates, and operational savings provides actionable insights for business leaders, ensuring that data governance initiatives deliver consistent value over time.
The trends shaping AI and ML integration into data governance indicate a shift towards more automated, scalable, and ethical frameworks. As AI technologies continue to evolve, their role in enhancing data governance becomes increasingly significant, offering organizations new ways to optimize their data management. Embracing these capabilities will not only streamline data processes but also drive greater business value and competitive advantage.
Companies that embrace these AI and ML trends will be positioned as leaders in the evolving landscape of data governance, ready to tackle challenges and seize new opportunities as they arise.
To explore how Lumenalta’s data solutions can support your journey towards AI-enhanced data governance, contact us today and take the first step towards transforming your data strategy.
Table of contents
- Emphasis on AI-driven data quality improvement
- Integration of machine learning in automated compliance
- Real-time data governance: meeting the demand for immediate insights
- Centralization vs. decentralization in data governance frameworks
- Enhancing data discovery and metadata management with AI
- Data ethics and privacy regulations
- Cloud-based data solutions
- Investment in AI explainability and transparency
- Adoption of data lineage and tracking technologies
- Measuring the ROI of AI-driven data governance
- Common questions about the future of data governance
Common questions about the future of data governance
What are the key trends shaping the future of data governance?
How does AI improve data quality in governance frameworks?
Why is machine learning important for compliance in data governance?
What role does cloud technology play in modern data governance?
How does real-time data governance benefit AI and ML integration?
Want to learn how artificial intelligence can bring more transparency and trust to your operations?