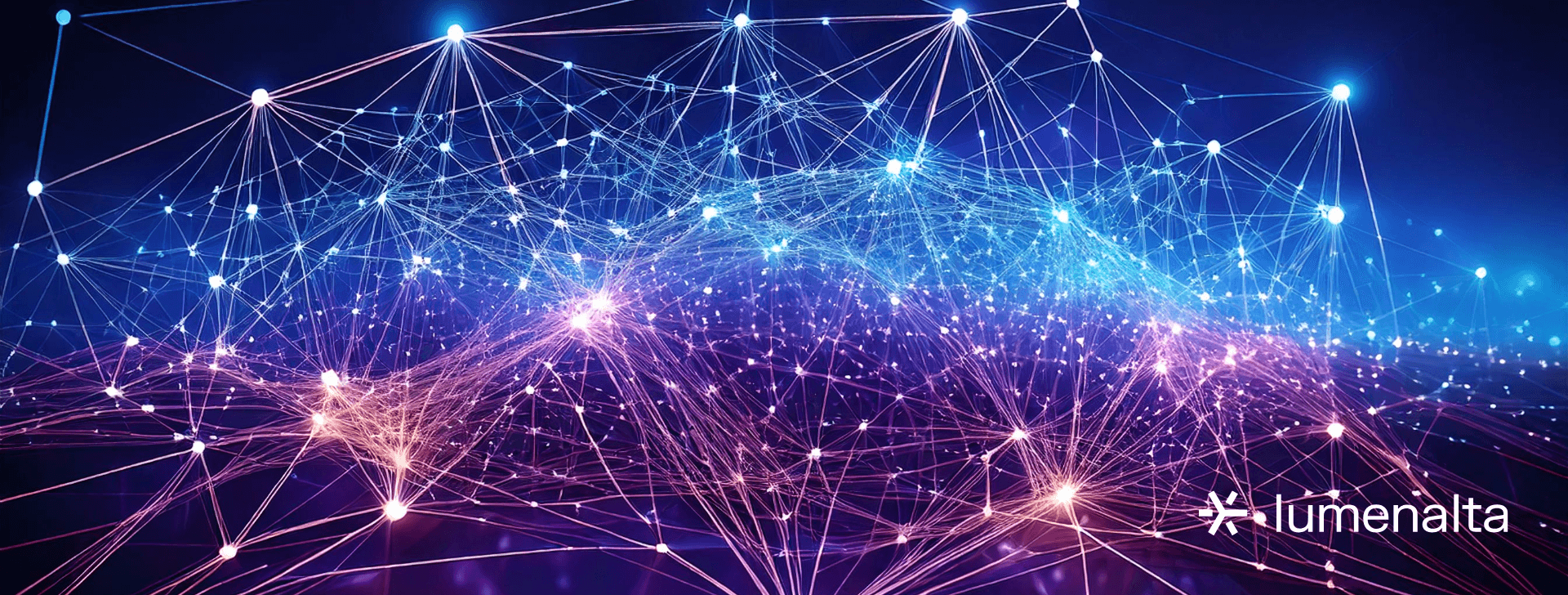
7 reasons why AI reasoning models accelerate time to value
FEB. 3, 2025
5 Min Read
AI reasoning models are redefining how businesses extract value from artificial intelligence.
Unlike traditional machine learning systems that rely on statistical correlations, these models apply structured logic and contextual awareness to improve decision accuracy and automation. Organizations utilizing these capabilities experience faster insights, reduced operational complexity, and more scalable AI-based processes.
Key takeaways
- 1. AI reasoning models improve decision accuracy by applying structured logic, reducing reliance on large datasets, and enhancing explainability.
- 2. These models accelerate time to value by validating faster AI deployment, reducing training data requirements, and improving adaptability to new challenges.
- 3. Businesses gain significant advantages in automation, workflow optimization, and real-time decision-making when implementing reasoning-based AI models.
- 4. Industries such as finance, healthcare, and cybersecurity benefit from reasoning models due to their ability to handle complex risk assessments, diagnostics, and threat detection.
- 5. AI reasoning models support long-term scalability by reducing model retraining needs, improving transparency, and adapting to growing business requirements.
What are AI reasoning models?
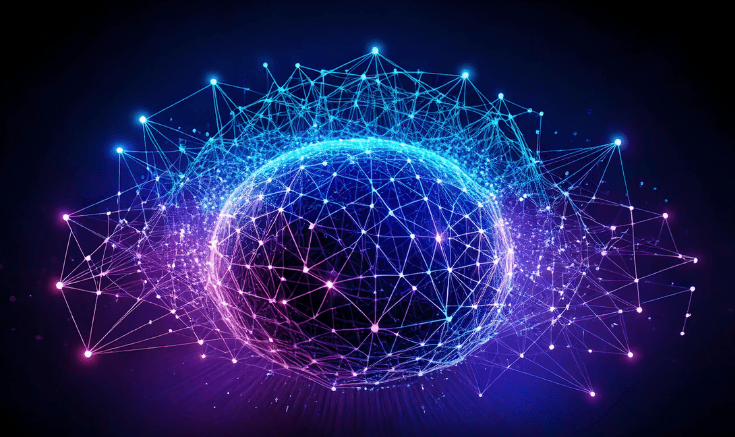
AI reasoning models are advanced systems designed to simulate human-like problem-solving and management capabilities. Unlike traditional machine learning models that rely on pattern recognition, these models apply logical inference, structured reasoning, and contextual understanding to interpret and generate responses.
These models use a combination of rule-based logic, probabilistic reasoning, and neural network architectures to process complex queries. By integrating multiple layers of analysis, they evaluate scenarios and adjust outputs based on situational context. With advancements like DeepSeek R1, which combines multiple reasoning techniques into a unified framework, AI is becoming more effective in automation, data-backed regulation, and enterprise applications.
“Unlike traditional machine learning models that rely on statistical correlations, AI reasoning models apply structured logic and contextual awareness to improve decision accuracy and automation.”
Types of AI reasoning models
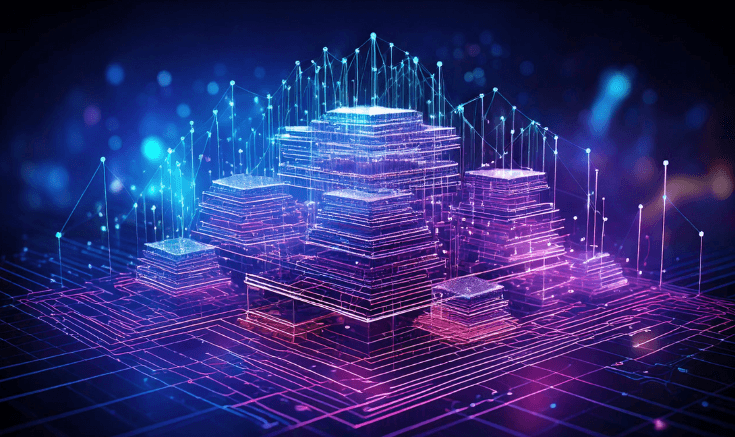
AI reasoning models use different approaches to process information, evaluate, and solve problems. Each type serves a unique function, making them suitable for various applications in automation, data analysis, and enterprise solutions.
- Deductive reasoning models: These models apply logical rules to understand specific content from general principles. They are commonly used in expert systems, rule-based engines, and automated theorem proving.
- Inductive reasoning models: By analyzing patterns in data, these models identify trends and make predictions. They are frequently employed in statistical analysis, forecasting, and anomaly detection.
- Abductive reasoning models: These models generate the most probable explanations for observed events, making them valuable in diagnostics, troubleshooting, and decision support systems.
- Probabilistic reasoning models: Using probability theory, these models evaluate uncertain data and compute likely outcomes. They are widely used in risk assessment, recommendation systems, and financial modeling.
- Commonsense reasoning models: Designed to understand everyday scenarios, these models simulate human intuition and general knowledge. They improve interactions in conversational AI and virtual assistants.
- Analogical reasoning models: These models draw comparisons between different situations to solve problems based on prior knowledge. They enhance case-based reasoning, creative problem-solving, and innovation strategies.
- Causal reasoning models: Focused on identifying cause-and-effect relationships, these models help in scientific discovery, policy analysis, and process optimization.
DeepSeek R1 reasoning is an example of a platform integrating these reasoning techniques to improve problem-solving. As AI continues to advance, combining multiple reasoning methods within a single model is becoming more common, enhancing the accuracy and adaptability of AI-powered solutions.
7 reasons why AI reasoning models accelerate time to value
AI reasoning models significantly reduce the time it takes for businesses to realize value from artificial intelligence. Either optimizing workflows, enhancing decisions, or automating complex processes, these models deliver measurable outcomes faster than traditional machine learning approaches.
1. Automating complex regulation
Traditional regulation processes require human expertise, extensive analysis, and time-consuming reviews. AI reasoning models streamline these workflows by evaluating data, applying logical inference, and generating accurate plans. This automation reduces bottlenecks in areas such as fraud detection, medical diagnostics, and supply chain optimization.
In high-stakes settings, immediate insights are crucial. AI reasoning models analyze vast datasets in real-time, ensuring decisions are based on up-to-date information. Organizations using these capabilities experience faster response times, reduced operational costs, and increased efficiency.
2. Enhancing accuracy with contextual understanding
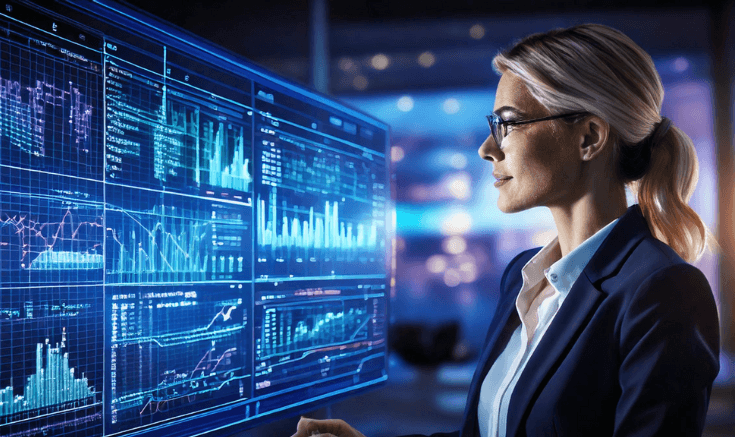
AI reasoning models incorporate structured logic and contextual awareness, improving the precision of responses. Unlike basic pattern recognition models, these systems process information within the appropriate context, reducing misinterpretations and incorrect outputs.
This capability is especially valuable in industries like financial services and healthcare, where slight errors can lead to significant consequences. From interpreting legal documents, analyzing medical records, and reviewing risk assessments, reasoning models ensure decisions are based on relevant contextual factors.
3. Reducing reliance on extensive training data
Many AI models require large datasets to achieve acceptable performance levels. AI reasoning models, however, integrate logical frameworks that allow them to function effectively with less training data. This reduces the time and resources needed to implement AI solutions, allowing businesses to deploy models faster.
Instead of continuously retraining models with new data, organizations can leverage AI reasoning models for scalable, adaptable automation. This efficiency is particularly beneficial for startups, enterprises with limited AI infrastructure, and industries with restricted data availability.
4. Improving adaptability to new challenges
Business settings constantly introduce new challenges, requiring AI systems to adapt quickly. AI reasoning models excel at applying logical inference and learned knowledge to unfamiliar scenarios, making them more resilient to changes.
Unlike traditional machine learning models that struggle with unseen data, reasoning models generalize better across different situations. This adaptability ensures AI-based solutions remain effective, reducing the need for frequent model updates and improving long-term usability.
5. Accelerating AI deployment and integration
Deploying AI solutions often involves lengthy development cycles, complex integrations, and extensive testing. AI reasoning models expedite this process by reducing the dependency on massive datasets, simplifying configuration, and enhancing interpretability.
Because these models incorporate structured reasoning, businesses can implement them without the prolonged optimization phases required by deep learning models. This speed advantage allows enterprises to capitalize on AI investments more quickly, leading to faster returns on technology adoption.
6. Increasing explainability and trust in AI outputs
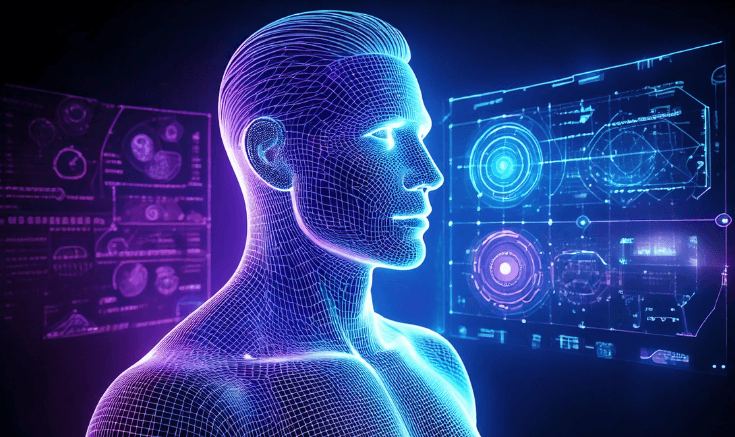
One of the biggest challenges in AI adoption is the lack of transparency in model decisions. AI reasoning models offer greater explainability by breaking down the logical steps behind each outcome. This clarity helps organizations build trust in AI processes, leading to higher adoption rates.
In regulated industries such as finance and healthcare, AI reasoning models provide traceable and auditable pathways to decisions. Stakeholders gain confidence in AI recommendations, helping with smoother regulatory compliance and stronger governance over automated processes.
7. Supporting real-time decision-making at scale
AI reasoning models allow real-time decision-making by processing vast amounts of data instantly. Industries such as e-commerce, cybersecurity, and autonomous systems benefit from this capability, as rapid analysis and response times are critical for operational success.
Reasoning models ensure decisions align with real-time conditions by detecting security threats, personalizing customer interactions, and optimizing logistics. This responsiveness drives efficiency, mitigates risks, and enhances user experiences across various applications.
AI reasoning models offer a direct path to faster value realization, eliminating inefficiencies associated with traditional AI deployments. Businesses adopting these models experience accelerated insights, improved decision accuracy, and reduced operational complexity.
"AI reasoning models significantly reduce the time it takes for businesses to realize value from artificial intelligence."
Future of AI reasoning models
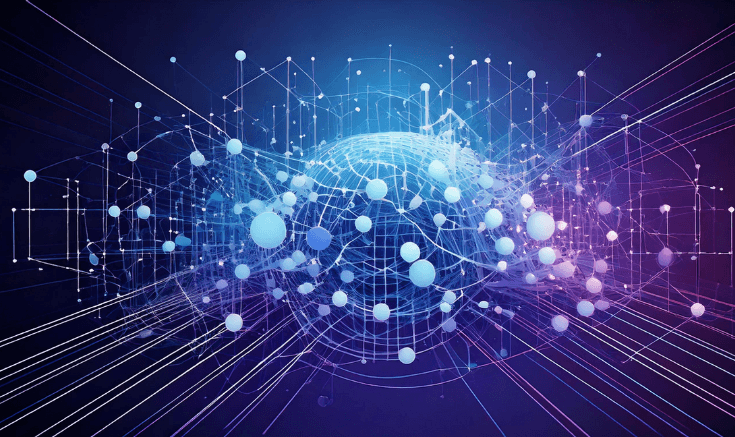
AI reasoning models are shaping the next phase of artificial intelligence by improving interpretability, adaptability, and management. As industries seek more reliable AI solutions, the focus is shifting toward models that combine multiple reasoning approaches to enhance accuracy and efficiency.
Advancements in DeepSeek reasoning, causal inference, and probabilistic modeling are making AI more effective at handling uncertainty and complex problem-solving. Future iterations of these models will integrate stronger knowledge representation techniques, allowing AI systems to function with a higher degree of logic and contextual awareness. This evolution will be critical in sectors like autonomous systems, financial risk assessment, and medical diagnostics.
Ongoing research is also addressing key challenges such as explainability, fairness, and scalability. AI reasoning models must be transparent enough to gain user trust while maintaining the flexibility needed to adapt to new challenges. As development continues, businesses implementing these models will gain an advantage through faster time to value and improved automation.
AI reasoning models are not just an enhancement to artificial intelligence—they are the foundation of a more efficient, accurate, and scalable decision process. By delivering faster insights, reducing complexity, and improving automation, these models help organizations to maximize results. At Lumenalta, we specialize in AI solutions designed to align with business objectives, ensuring intelligent decision support at every level. Let’s build a smarter future together.
table-of-contents
- What are AI reasoning models?
- Types of AI reasoning models
- 7 reasons why AI reasoning models accelerate time to value
- 1. Automating complex regulation
- 2. Enhancing accuracy with contextual understanding
- 3. Reducing reliance on extensive training data
- 4. Improving adaptability to new challenges
- 5. Accelerating AI deployment and integration
- 6. Increasing explainability and trust in AI outputs
- 7. Supporting real-time decision-making at scale
- Future of AI reasoning models
- Common questions about AI reasoning models
Common questions about AI reasoning models
What are reasoning models in AI, and how do they work?
How do AI reasoning models differ from traditional machine learning?
What industries benefit the most from AI reasoning models?
Can AI reasoning models improve explainability in AI systems?
What role do AI reasoning models play in real-time evaluation?
Want to learn how artificial intelligence can bring more transparency and trust to your operations?