These AI-powered systems analyze vast amounts of data to produce human-like responses, making them valuable tools for industries looking to scale operations efficiently. Organizations that integrate these models into their workflows benefit from reduced manual effort, faster processing times, and cost-effective automation. With continuous advancements in artificial intelligence, large language models are becoming more refined, supporting complex use cases that improve accuracy and business impact.
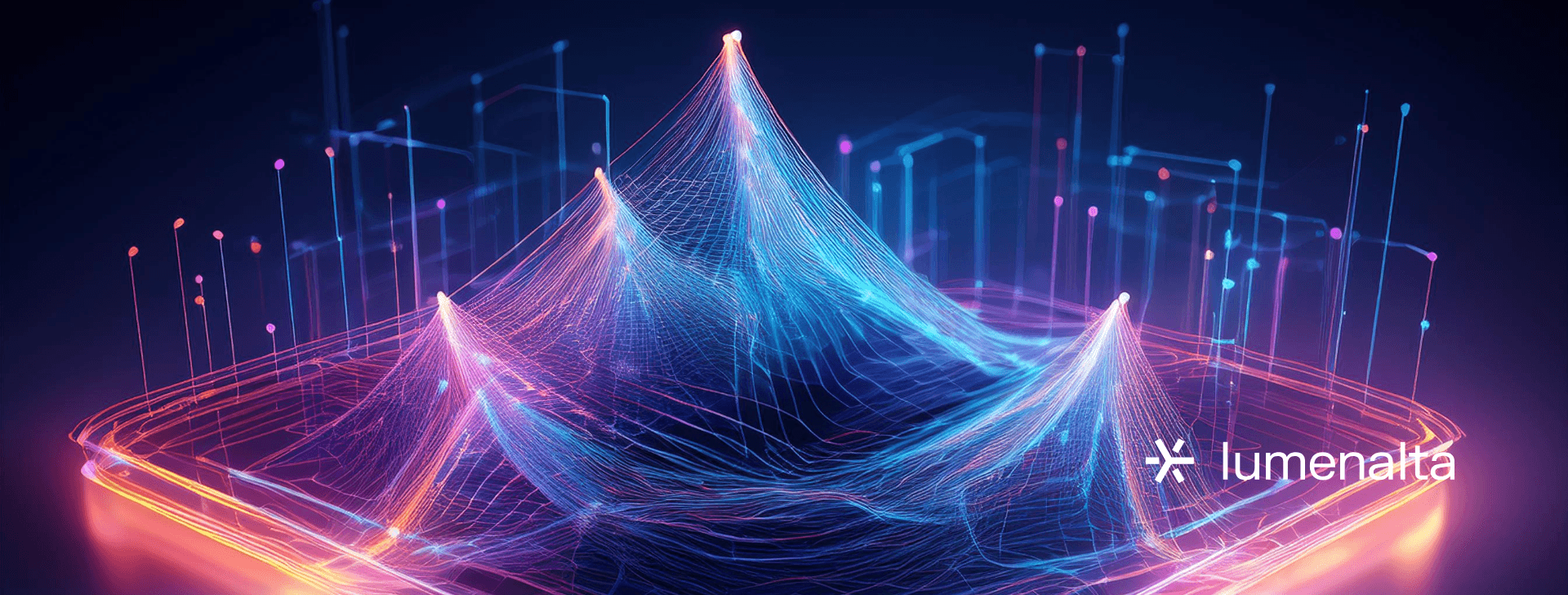
7 surprisingly powerful large language model applications
JAN. 23, 2025
7 Min Read
Large language model applications are modifying how businesses automate processes, generate content, and improve customer interactions.
Key takeaways
- 1. Large language model applications automate complex text-based tasks, improving efficiency and reducing manual effort in industries such as healthcare, finance, and software development.
- 2. Conversational AI, automated content creation, and research assistance are among the most impactful use cases for businesses looking to scale operations.
- 3. Ethical considerations, including bias mitigation and data privacy, must be prioritized to maintain fairness and compliance when deploying AI solutions.
- 4. Multimodal AI is expanding the capabilities of large language models, allowing them to process text, images, and voice in a unified system for improved accuracy.
- 5. Companies strategically integrating AI-powered tools gain measurable benefits, including cost savings, faster response times, and enhanced customer interactions.
What are large language model applications?
Large language model applications power many text-based artificial intelligence tools across industries today. These AI-led systems analyze massive datasets, predict text, and generate human-like responses with a high degree of accuracy. Organizations use them to automate workflows, improve efficiency, and enhance user interactions. As businesses look for scalable and cost-effective solutions, these applications play a key role in reducing manual tasks while maintaining quality and precision.
These applications support various functions, from answering customer inquiries to generating high-quality written content. Advanced implementations include real-time language translation, automated coding assistance, and intelligent research tools that process vast amounts of information in seconds. Industries such as healthcare, finance, and retail rely on these capabilities to improve communication, streamline operations, and extract insights from unstructured data.
The ability to process and generate text at scale has made large language model applications an essential tool for organizations aiming to improve efficiency and deliver personalized experiences. Businesses that adopt these solutions often gain measurable benefits, such as faster response times, lower operational costs, and improved customer engagement. As artificial intelligence advances, these applications will become even more precise, adaptable, and valuable in addressing real business challenges.
"The ability to process and generate text at scale has made large language model applications an essential tool for organizations aiming to improve efficiency and deliver personalized experiences."
How LLM applications work
Large language model applications function through advanced artificial intelligence techniques that allow them to process and generate human-like text. These systems rely on deep learning methods to analyze patterns in language, predict word sequences, and construct coherent responses based on context. Unlike traditional programs that follow predefined rules, these models adapt to a wide range of language tasks, making them highly effective for automation, content generation, and conversational AI.
Neural networks power these models by recognizing complex relationships between words and phrases across extensive datasets. Training a large language model requires processing billions of text samples to develop an understanding of syntax, grammar, and context. Transformer architectures, designed to process words in relation to one another rather than in isolation, contribute to the accuracy and fluency of responses. Once trained, these models can be fine-tuned to specialize in specific tasks, such as summarizing long documents, assisting with programming, or analyzing customer sentiment.
Many industries rely on large language model applications for improved efficiency, cost reduction, and faster processing times. These systems assist with automating repetitive tasks, generating insights from unstructured text, and enhancing user interactions. Organizations that integrate these applications into their workflows benefit from increased productivity and scalable solutions that reduce reliance on manual processes. As artificial intelligence advances, large language models will provide even greater accuracy, adaptability, and business value.
7 powerful large language model applications
Large language model applications provide advanced automation and efficiency across a wide range of industries. These AI-powered systems analyze vast amounts of text, generate human-like responses, and assist with tasks that traditionally require significant manual effort. From customer interactions to content creation and compliance management, these applications help organizations scale operations while reducing costs. Companies that integrate these solutions into their workflows often experience faster processing times, improved accuracy, and better resource allocation.
The ability to process language with precision has made these models an essential tool for businesses looking to optimize productivity. The following types of LLM application highlight some of the most impactful ways artificial intelligence supports necessary business functions.
1. Conversational AI and chatbots
AI-backed chatbots and virtual assistants handle inquiries, provide customer support, and assist with sales interactions. These systems understand natural language, detect intent, and generate personalized responses, improving communication without human intervention.
Organizations that use AI-powered chatbots reduce response times, streamline service operations, and maintain consistency across multiple communication channels. Automated conversations allow support teams to focus on complex requests while providing users with quick and accurate assistance. Advanced implementations also include voice-based assistants that improve accessibility and engagement.
2. Automated content creation
AI-powered content generation tools assist with writing marketing copy, reports, product descriptions, and long-form articles. These applications analyze context, structure responses, and create well-formatted text that aligns with brand messaging and industry requirements.
Companies that rely on content marketing benefit from automated writing solutions that reduce production time while maintaining quality. Scalable content creation supports digital campaigns, enhances search engine visibility, and improves audience engagement. AI-generated writing tools also help businesses maintain consistency across multiple platforms and adapt messaging for different markets.
3. Code generation and software development assistance
Large language models improve coding efficiency by generating code snippets, detecting errors, and suggesting optimizations based on best practices. These applications assist software engineers in writing and debugging programs, reducing the time spent on repetitive development tasks.
AI-assisted development tools provide real-time recommendations, allowing developers to focus on complex problem-solving. These applications also contribute to better collaboration by simplifying documentation and making it easier to understand existing codebases. Organizations that integrate AI-powered coding assistants reduce errors and accelerate software development timelines.
4. Language translation and localization
Artificial intelligence improves translation accuracy by analyzing text context, tone, and cultural nuances. These applications support multilingual communication by adapting content for different audiences while maintaining message clarity.
Companies that operate across global markets use AI-powered translation tools to localize websites, customer support responses, and marketing materials. Automated translation enhances accessibility, improves audience engagement, and reduces reliance on manual translation services. Large language models provide a scalable solution for businesses expanding into new regions while maintaining consistent communication.
5. Research and data analysis
Large language models assist professionals with processing extensive datasets, summarizing key insights, and extracting relevant information from complex documents. These applications enhance research efficiency by analyzing reports, industry studies, and online sources with greater speed and accuracy.
Industries such as finance, healthcare, and academia use AI-powered research tools to identify patterns, detect trends, and generate detailed reports. Automating research tasks allows professionals to focus on strategy and critical analysis rather than spending time on manual data processing. AI-assisted research applications continue to improve as these models gain access to more refined datasets and advanced processing techniques.
6. Sentiment analysis and customer insights
Organizations use AI-powered sentiment analysis tools to evaluate customer opinions, analyze feedback, and assess online discussions. These applications detect emotions, track sentiment shifts, and give businesses insights into public perception.
Analyzing customer sentiment helps companies refine marketing strategies, improve product development, and enhance user experiences. Automated tools process large volumes of text, identifying emerging trends and customer preferences. AI-powered sentiment analysis supports brand management efforts and provides actionable insights that help businesses adjust their strategies to meet audience expectations better.
7. Legal and compliance automation
Legal professionals and compliance teams use large language models to review contracts, identify key clauses, and summarize legal documents. These applications analyze legal texts with precision, reducing the time required for manual contract review and regulatory checks.
Automating compliance tasks helps organizations maintain accuracy while minimizing risks associated with human oversight. AI-powered tools assist with contract drafting, policy analysis, and due diligence, improving efficiency in legal operations. Companies that adopt these solutions reduce administrative burdens and optimize compliance processes.
Large language model applications continue improving efficiency across multiple industries, providing organizations with scalable content generation, communication, and automation solutions. The ability to analyze text at scale, generate precise responses, and automate complex workflows allows businesses to reduce costs, improve response times, and enhance customer experiences. As artificial intelligence technology advances, these applications will become even more refined, offering increased accuracy and deeper integrations with business systems.
Ethical considerations and challenges in LLM applications
Large language model applications provide significant benefits, but their widespread use introduces ethical concerns and operational challenges. Organizations adopting these technologies must address bias, misinformation, data privacy, and unintended consequences. Without proper oversight, these systems may generate inaccurate or misleading information, affecting business outcomes and public trust.
Bias remains a significant concern in artificial intelligence. Large language models learn from vast datasets, which may contain imbalances that influence their outputs. If training data reflects historical or systemic biases, AI-generated responses can unintentionally reinforce stereotypes or provide skewed results. Addressing these biases requires continuous monitoring, various datasets, and responsible fine-tuning to improve fairness and accuracy.
Data privacy presents another challenge. Large language models process and store significant amounts of text, raising concerns about how sensitive information is handled. Businesses and developers must implement strict security measures to protect user data and comply with regulations. Failing to do so may expose organizations to legal risks and damage customer relationships.
Misinformation is another risk associated with AI-generated content. These models predict text based on statistical probabilities, sometimes leading to incorrect or misleading outputs. Organizations that use AI-generated content in public-facing applications should incorporate verification steps to reduce errors and maintain credibility. Human oversight remains essential in ensuring AI-generated responses align with factual accuracy and ethical standards.
The automation of content and decision-support processes also raises concerns about transparency and accountability. Users interacting with AI-generated responses may not always be aware they are engaging with an automated system. Defining AI’s role in workflows and maintaining human oversight helps build trust and prevent reliance on unverified outputs.
Ethical considerations must be a priority for organizations using large language model applications. Responsible deployment requires a balanced approach that incorporates transparency, fairness, and compliance with industry regulations. As artificial intelligence develops, ongoing evaluation and improvements will help mitigate risks while maximizing these applications' benefits.
"Automated conversations allow support teams to focus on complex requests while providing users with quick and accurate assistance."
Best practices for implementing LLM applications
Successful implementation of large language model applications requires careful planning, ethical considerations, and a structured approach to integration. Organizations that adopt these solutions should focus on accuracy, efficiency, and compliance to maximize the benefits while mitigating risks.
- Define clear objectives: Establish the primary use case for the application, whether it is for customer service automation, content generation, or internal process optimization. Setting measurable goals ensures the technology aligns with business priorities.
- Choose the right model and architecture: Different language models offer varying levels of complexity and customization. Organizations should evaluate open-source and proprietary models to determine which best meets their needs regarding scalability, accuracy, and security.
- Mitigate bias and inaccuracies: Large language models learn from extensive datasets that may contain biases. Regular audits, mixed training data, and model fine-tuning help reduce unintended bias and improve response fairness. Implementing human oversight ensures generated content aligns with ethical standards.
- Prioritize data security and compliance: AI applications process vast amounts of text, some of which may include sensitive information. Strong encryption, strict access controls, and adherence to industry regulations protect data privacy and reduce security risks. Compliance with standards such as GDPR or HIPAA is essential for organizations handling regulated information.
- Integrate human oversight: While AI enhances automation, human intervention remains critical for monitoring outputs and making final decisions. Establishing review processes prevents the spread of misinformation and ensures content aligns with business policies.
- Optimize for performance and cost-efficiency: Running large-scale AI models requires significant computational resources. Organizations should balance performance with cost by selecting efficient deployment methods based on workload demands, such as cloud-based services or on-premise solutions.
- Monitor and continuously improve the system: AI applications require ongoing evaluation to maintain accuracy and relevance. Establishing feedback loops and user testing allows businesses to refine outputs, address weaknesses, and enhance the overall user experience.
Implementing large language model applications effectively supports business growth, improves efficiency, and enhances customer engagement. A strategic approach that combines transparency, security, and ethical oversight helps organizations unlock the full potential of these AI-powered solutions while reducing potential risks.
Understanding large language models in 2025
In 2025, large language models (LLMs) are becoming more refined, offering businesses enhanced efficiency, automation, and customer engagement. Advancements in contextual accuracy, multimodal capabilities, and ethical safeguards enable AI to support industry-specific applications in law, healthcare, finance, and software development. Instead of relying on broad datasets, specialized models trained on domain-specific data provide more precise and relevant outputs, optimizing workflows and reducing errors.
Multimodal AI expands the scope of LLMs by integrating text, image, and audio processing, enhancing automation in document analysis, voice-to-text, and content moderation. Ethical considerations remain central, with businesses prioritizing fairness, transparency, and regulatory compliance through bias mitigation and responsible AI frameworks. As adoption grows, companies leveraging AI strategically gain cost savings, increased productivity, and improved customer interactions, positioning themselves for scalable growth in the market.
Large language model applications offer more than automation—they provide businesses with more competent, faster, and scalable solutions for content generation, analysis, and customer engagement. As artificial intelligence continues to improve, these applications will play an even greater role in optimizing operations. At Lumenalta, we specialize in AI-based solutions that align with business goals, helping organizations maximize efficiency and improve outcomes. Let’s build a future where AI works seamlessly for you.
table-of-contents
- What are large language model applications?
- How LLM applications work
- 7 powerful large language model applications
- Ethical considerations and challenges in LLM applications
- Best practices for implementing LLM applications
- Understanding large language models in 2025
- Common questions about powerful large language model applications
Common questions about large language model applications
What are the most impactful large language model applications?
How do large language models improve efficiency?
What challenges exist when implementing large language model applications?
Can AI-generated content be as reliable as human writing?
How will large language model applications develop in the future?
Want to learn how AI, LLM, ML can bring more transparency and trust to your operations?