With the financial sector relying on accurate, efficient, and scalable technologies, NLP has become essential for gaining a measurable edge in operations, investment strategies, and compliance. Its ability to automate labor-intensive processes, identify patterns, and deliver real-time insights makes it indispensable in meeting today’s challenges while unlocking future potential.
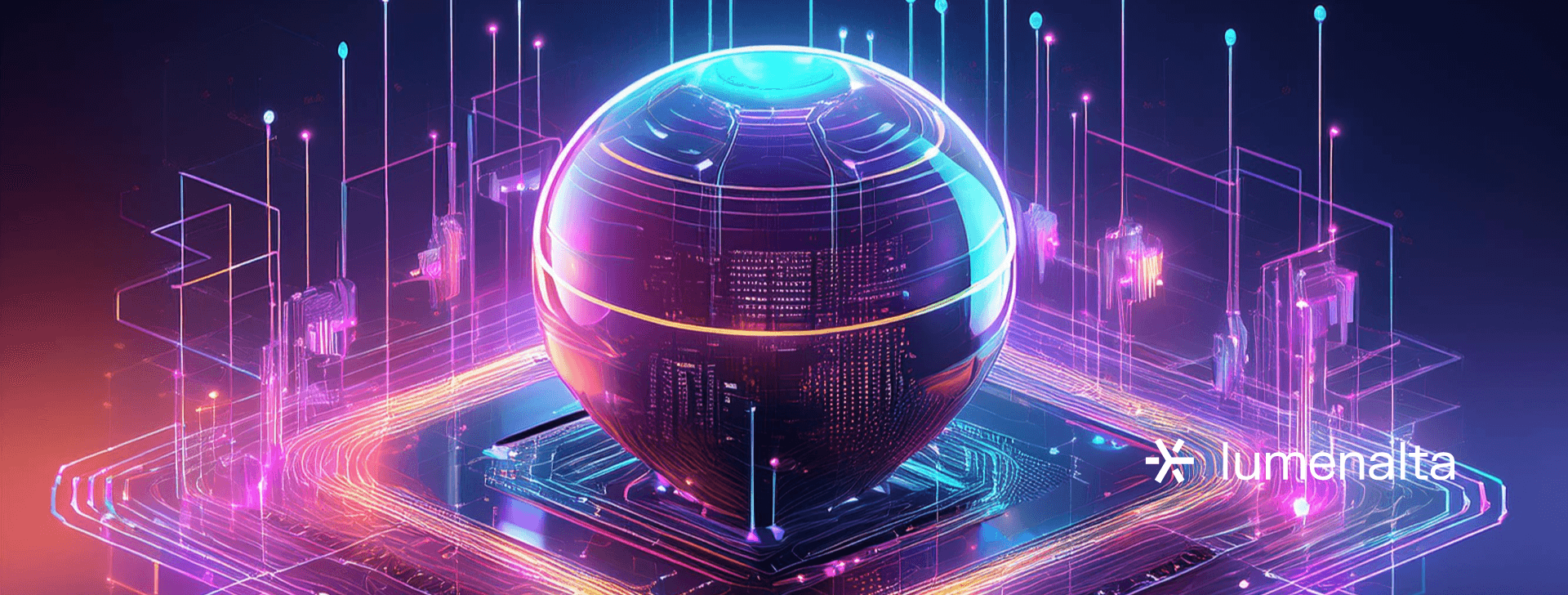
7 applications of NLP in finance
JAN. 19, 2025
6 Min Read
Natural language processing (NLP) reshapes how financial institutions process and analyze data, turning previously unmanageable volumes of unstructured information into actionable insights.
Key takeaways
- 1. NLP allows financial institutions to automate labor-intensive tasks, saving time and reducing operational costs.
- 2. Sentiment analysis with NLP provides actionable insights into market trends and public perception, supporting strategic adjustments.
- 3. NLP-powered tools improve risk management by identifying vulnerabilities in contracts and regulatory filings, reducing exposure to financial risks.
- 4. Financial document summarization enhances productivity by extracting key insights from unstructured data, improving time-to-value.
- 5. Customer interactions benefit from NLP-powered chatbots, which streamline responses and improve satisfaction while reducing resource requirements.
Understanding NLP in finance
Natural language processing (NLP) has emerged as a pillar of technological advancement in finance, allowing firms to better analyze and manage the vast quantities of text-based data they generate daily. In the financial sector, where accuracy, timeliness, and informed analysis are critical, NLP bridges the gap between unstructured language data and actionable insights. Its ability to interpret and process human language allows financial institutions to stay ahead in an industry where decisions are based on nuanced and complex information.
Organizations in finance are tasked with analyzing an overwhelming amount of data, including news reports, earnings calls, financial statements, and regulatory filings. Reviewing these materials through traditional manual processes is resource-intensive and often subject to errors or missed opportunities. NLP technology automates this process, offering scalable, precise methods to extract relevant information from text sources. Using machine learning in finance helps firms analyze sentiment, assess risk, and improve accuracy—far beyond what manual processes can achieve.
For financial institutions, the value of NLP extends to improving scalability and operational efficiency. It is an essential tool for managing large datasets, reducing processing times, and providing insights that drive measurable business outcomes. From identifying emerging market trends to improving the clarity of financial reporting, NLP offers future-proof strategies that empower organizations to meet their goals more effectively. Its use is no longer confined to niche applications but is central to how financial firms operate in a data-backed economy.
"Sentiment analysis uses NLP to interpret the tone and sentiment behind text, allowing financial institutions to assess market trends, investor sentiment, and public perception."
Benefits of natural language processing in finance
Natural language processing is reshaping the financial sector by introducing cost-effective, scalable, and data-led approaches to solving complex challenges. Financial institutions manage immense textual data, including financial reports, regulatory filings, news updates, and customer interactions. The ability to analyze and interpret this data efficiently can unlock untapped business opportunities, optimize resource allocation, and drive measurable returns. NLP offers innovative solutions to automate processes, reduce manual workloads, and enhance the accuracy and speed of operations. Its growing role in finance is no longer limited to data analysis but extends to improving customer satisfaction, mitigating risks, and supporting compliance efforts.
- Improved operational efficiency: Automating the processing of text-heavy resources like earnings reports, contracts, and market analyses significantly reduces manual intervention. This improvement in efficiency allows financial teams to focus on higher-value strategic priorities, ultimately saving time and operational costs.
- Enhanced accuracy in data analysis: NLP algorithms excel at identifying trends, detecting risks, and analyzing vast datasets with precision. Unlike traditional methods prone to human error, NLP offers consistently accurate insights, granting firms to minimize costly risk management, portfolio management, and compliance mistakes.
- Scalable solutions for large datasets: Financial services generate terabytes of data daily, requiring scalable methods to process this information quickly. NLP systems can process these datasets in real time, making managing tasks like analyzing regulatory updates or summarizing financial statements at scale easier.
- Actionable insights for investment strategies: Financial markets are influenced by various factors, including news sentiment, global events, and economic indicators. NLP tools analyze these factors to provide actionable insights, helping portfolio managers identify trends that maximize returns for investors and mitigate potential risks.
- Real-time insights to seize opportunities: NLP-powered systems process market data, news, and sentiment metrics in real time, providing valuable insights that can shape market strategies. Organizations gain the ability to react swiftly to emerging trends, improving agility and strategic alignment with market dynamics.
- Enhanced customer interactions: NLP grants financial institutions to deliver better customer service through chatbots and virtual assistants. These tools can resolve inquiries efficiently, improve response times, and address issues precisely, creating a seamless and positive customer experience.
- Simplified regulatory compliance: Financial institutions face stringent regulatory requirements. NLP tools automate document analysis and align processes with regulatory standards, reducing compliance-related risks and freeing up resources that can be redirected to other priorities.
NLP is becoming indispensable for financial firms seeking measurable business impact through better resource management, accurate analytics, and improved customer experiences. Its scalability and efficiency allow organizations to achieve faster results, future-proof their strategies, and unlock previously untapped potential. As NLP advances, it will remain an essential tool for optimizing operations and delivering value in the financial sector.
7 applications of NLP in finance
Natural language processing is modifying finance, offering innovative ways to process unstructured data, enhance operational efficiency, and maximize business impact. The financial sector generates massive amounts of text-based information daily, including regulatory filings, news reports, contracts, and customer communications. This sheer volume makes manual processing time-intensive, error-prone, and inefficient. NLP solutions address these challenges by automating analysis, identifying critical trends, and supporting faster, more accurate insights. Its use reshapes financial institutions' management of risk, serving customers, and making informed strategic decisions.
1. Sentiment analysis
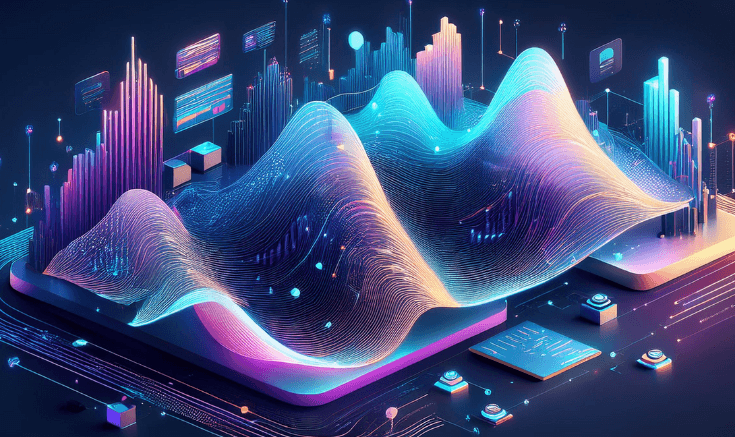
Sentiment analysis helps financial institutions interpret the emotional tone behind text sources like news articles, earnings call transcripts, and social media content. By analyzing these sources, organizations can gauge public perception, investor sentiment, and potential market movements. This capability empowers firms to make strategic decisions aligned with emerging trends.
A financial services firm could analyze how news about a specific company or sector is being received by the public. Positive sentiment might signal growth potential, while negative sentiment could warn of risks. Insights like these allow portfolio managers and analysts to adjust investment strategies accordingly.
This application of NLP delivers measurable business value by providing timely and actionable insights, allowing firms to adapt more effectively to shifting market conditions while optimizing opportunities for returns.
2. Financial document summarization
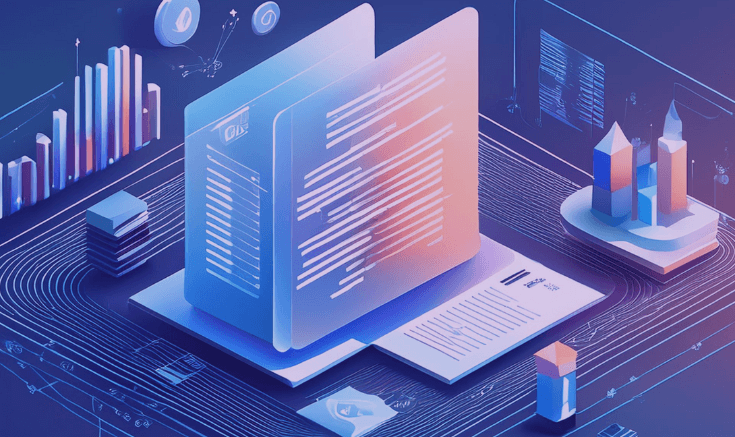
Financial institutions are inundated with extensive documents, including regulatory filings, annual reports, and analyst research. NLP-powered document summarization tools streamline the review process by extracting key details and presenting them in concise formats. This approach reduces time-to-value and improves operational efficiency without sacrificing accuracy.
Investment analysts, for instance, need to quickly extract critical metrics from earnings reports to guide decisions. NLP tools can identify and summarize performance data, risks, and other essential insights, letting analysts focus on strategic priorities rather than time-consuming manual reviews.
3. Risk assessment and management
Risk management is a crucial aspect of financial operations, requiring detailed analysis of contracts, market data, and regulatory updates. NLP helps identify risks that might otherwise go unnoticed by analyzing vast datasets and detecting language patterns indicative of potential issues.
For example, a bank reviewing loan agreements can use NLP to flag terms that suggest higher risk profiles or identify inconsistencies in regulatory compliance. These insights allow institutions to proactively address vulnerabilities, reduce financial exposure, and ensure operational stability.
NLP supports more scalable and precise risk assessments, helping organizations mitigate financial and regulatory risks while maintaining governance and compliance standards.
4. Chatbots and customer service
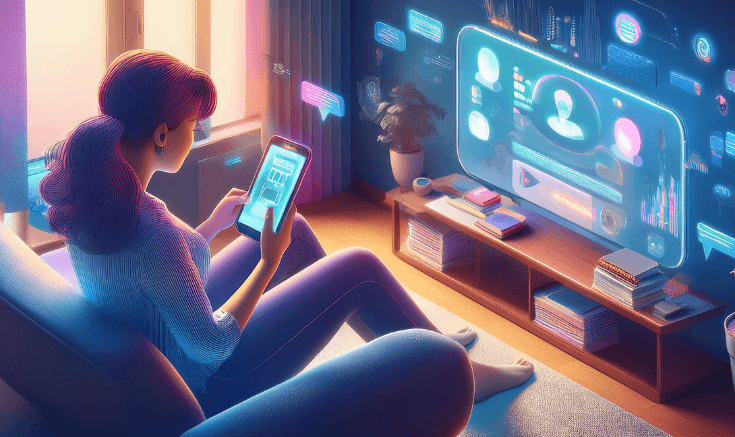
NLP powers intelligent chatbots and virtual assistants, modernizing how financial institutions manage customer interactions. These tools process customer inquiries, provide instant responses, and resolve common issues efficiently, reducing the need for human intervention.
For example, a chatbot can help customers check account balances, understand loan eligibility, or resolve transaction disputes. Beyond improving response times, NLP-led tools personalize interactions, increasing customer satisfaction and loyalty.
5. Regulatory compliance
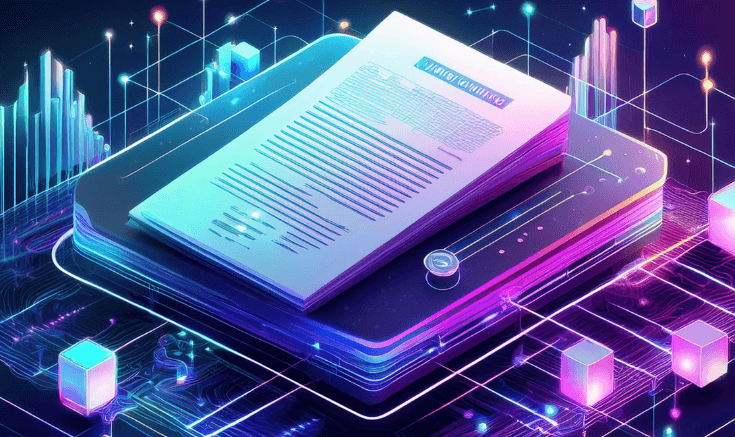
Financial institutions face stringent compliance requirements, and failing to meet them can result in significant penalties. NLP simplifies compliance efforts by analyzing regulatory texts, monitoring policy updates, and ensuring documentation aligns with requirements.
For instance, an NLP system could review and validate compliance reports to identify missing data or flag potential non-conformance with regulatory standards. These tools reduce the likelihood of human error and improve the speed and accuracy of compliance reporting processes.
This application of NLP saves time and minimizes risks related to regulatory violations, supporting smoother governance and operational continuity.
6. Portfolio management
Portfolio management requires continuous monitoring of market conditions, news sentiment, and investment performance. NLP tools analyze these data sources to provide actionable insights, helping portfolio managers optimize allocations and identify profitable opportunities.
A portfolio manager, for example, might use NLP to scan global news for sentiment shifts or identify market events that could affect specific assets. This information permits quicker, more informed adjustments to portfolio strategies.
The ability to process various data streams in real time helps organizations improve portfolio outcomes, maximize returns, and mitigate potential losses.
7. Market research
Market research relies heavily on data collection and analysis, and NLP streamlines this process by automating the extraction of trends and patterns from large volumes of text. Organizations use this information to identify untapped business opportunities and guide investment or product strategies.
NLP can analyze everything from consumer sentiment in social media discussions to detailed insights in industry reports. These findings allow firms to identify growth opportunities or emerging market risks hidden in unstructured data.
NLP applications in finance continue to expand, offering solutions that improve scalability, enhance customer satisfaction, and optimize strategic processes. Whether used to automate repetitive tasks, identify risks, or unlock new opportunities, NLP delivers measurable value to financial institutions. Its ability to turn vast amounts of unstructured data into actionable insights positions it as a foundation of modern finance, helping firms stay ahead in an industry where precision and agility are essential.
Challenges integrating NLP in finance
While natural language processing delivers transformative benefits in finance, implementing it effectively requires addressing several significant challenges. These obstacles often stem from the complexity of financial data, stringent compliance requirements, and high expectations for precision and scalability. Organizations seeking to integrate NLP into their financial operations must carefully handle these challenges to unlock their full potential while ensuring seamless functionality and measurable business impact.
- Handling unstructured and complex data: Financial data comes in different formats, including earnings reports, contracts, legal filings, and customer communications. NLP models must be trained to process and understand this highly specialized and often technical language, which requires substantial training data and model development investments.
- Ensuring data quality and consistency: The accuracy of NLP tools relies heavily on the quality of input data. In finance, datasets often contain errors, inconsistencies, or outdated information, which can reduce the effectiveness of NLP algorithms and lead to inaccurate outcomes. Standardizing and cleansing data before applying NLP is critical but can be resource-intensive.
- Addressing regulatory and compliance risks: Financial institutions operate under strict regulatory frameworks, and any errors in NLP-based processes could expose firms to significant legal and financial risks. Adapting NLP tools to meet specific compliance requirements and keeping them updated with growing regulations is a continuous challenge.
- Managing costs of implementation: Building and deploying NLP systems tailored to financial use cases requires significant investment in infrastructure, data engineering, and skilled personnel. Smaller institutions may struggle to allocate the resources necessary to develop robust NLP capabilities, limiting their ability to compete with larger players.
- Overcoming interpretability issues: Many NLP models, particularly those based on deep learning, are often seen as "black boxes," making it difficult to understand how specific decisions or outputs are generated. This lack of transparency can pose challenges in high-stakes financial contexts where explainability is crucial for compliance and risk management.
- Ensuring scalability: Financial institutions deal with massive volumes of data that NLP models must process in real time or near-real time. Scaling these models to handle enterprise-level data while maintaining high performance and low latency requires robust infrastructure and optimization strategies.
- Aligning NLP outcomes with business objectives: Implementing NLP effectively requires aligning its outputs with organizational goals, such as improving operational efficiency, maximizing returns, or mitigating risks. Without clear objectives, NLP initiatives can fail to deliver measurable business value.
While these challenges are significant, they are not insurmountable. Financial institutions that invest in high-quality data, robust infrastructure, and a skilled workforce can successfully integrate NLP into their operations. Addressing these hurdles lets organizations gain an advantage, reduce risks, and achieve faster time-to-value in their financial processes.
Financial institutions handle extensive documentation daily, from financial statements to regulatory filings and investment research. NLP-based document summarization tools can process these lengthy texts and produce concise, accurate summaries."
Measuring ROI of NLP in finance
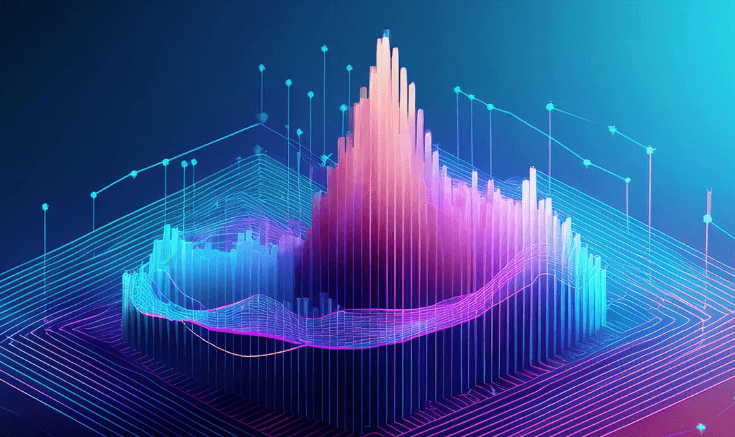
Measuring the return on investment (ROI) of natural language processing in finance requires a structured approach that highlights both quantitative and qualitative benefits. Financial institutions often adopt NLP to reduce costs, increase operational efficiency, and generate new revenue streams. Evaluating the success of these implementations ensures that the technology aligns with organizational goals and delivers measurable business outcomes.
Cost reduction is one of the most precise indicators of ROI for NLP in finance. Automating resource-intensive processes such as analyzing regulatory filings or financial documents helps organizations save both time and labor. For example, deploying NLP tools for processing quarterly reports can cut analysis time by significant margins while improving accuracy. These efficiencies free up human resources to focus on activities that add excellent value to the organization.
Revenue generation provides another key metric for assessing ROI. NLP-powered tools such as sentiment analysis platforms and market research solutions offer actionable insights into consumer preferences, market trends, and economic shifts. These insights allow portfolio managers to make data-led adjustments to investment strategies, increasing profitability and maximizing returns for investors.
Precision and risk mitigation also play a vital role in evaluating NLP’s financial impact. Automating the identification of anomalies in contracts, regulatory documents, or market data reduces the potential for costly errors. Detecting risks earlier in the process minimizes financial losses and contributes to more reliable operational workflows, making risk management more efficient and impactful.
Scalability highlights NLP's value in financial operations. Organizations can process larger datasets at a fraction of the time and cost associated with manual workflows. Real-time capabilities allow firms to adapt to shifting market conditions and manage their operations without sacrificing quality or increasing resource footprint.
Beyond financial metrics, organizations benefit from improved customer satisfaction when integrating NLP. Chatbots and virtual assistants powered by NLP create seamless customer interactions by addressing inquiries and resolving issues efficiently. Enhancing the client experience helps foster loyalty and retention, which has long-term financial implications.
Comprehensive ROI analysis considers tangible benefits, such as cost savings and revenue growth, and intangible gains, such as scalability and customer experience enhancements. Financial institutions that approach NLP integration with clear objectives and measurable key performance indicators can maximize the value of this technology and align it with their broader strategic goals. NLP offers an opportunity to deliver quantifiable business outcomes while preparing financial firms for future challenges and opportunities.
Natural language processing drives more intelligent, faster, and more effective financial operations by delivering automation, insights, and scalability. Turning complex challenges into measurable business outcomes permits NLP to empower organizations to focus on delivering results. At Lumenalta, we design tailored NLP solutions that align with your goals, ensuring you stay ahead. Let’s create a brighter path forward.
Table of contents
- Understanding NLP in finance
- Benefits of natural language processing in finance
- 7 applications of NLP in finance
- 1. Sentiment analysis
- 2. Financial document summarization
- 3. Risk assessment and management
- 4. Chatbots and customer service
- 5. Regulatory compliance
- 6. Portfolio management
- 7. Market research
- Challenges integrating NLP in finance
- Measuring ROI of NLP in finance
- Common questions about NLP in finance
Common questions about NLP in finance
What is natural language processing in finance?
How does NLP improve investment strategies?
What are the scalability benefits of NLP in finance?
How does NLP support regulatory compliance in finance?
What are the measurable outcomes of using NLP in finance?
Want to learn how NLP can bring more transparency and trust to your operations?