Investors and financial institutions are adopting AI-powered tools to process market data more accurately, identify trends, and execute trades more efficiently. With AI’s ability to continuously analyze real-time data, portfolio managers gain an edge in making informed investment decisions while minimizing inefficiencies. Integrating AI into portfolio management is not just a technological upgrade—it’s a strategic necessity for optimizing financial outcomes in a complex market.
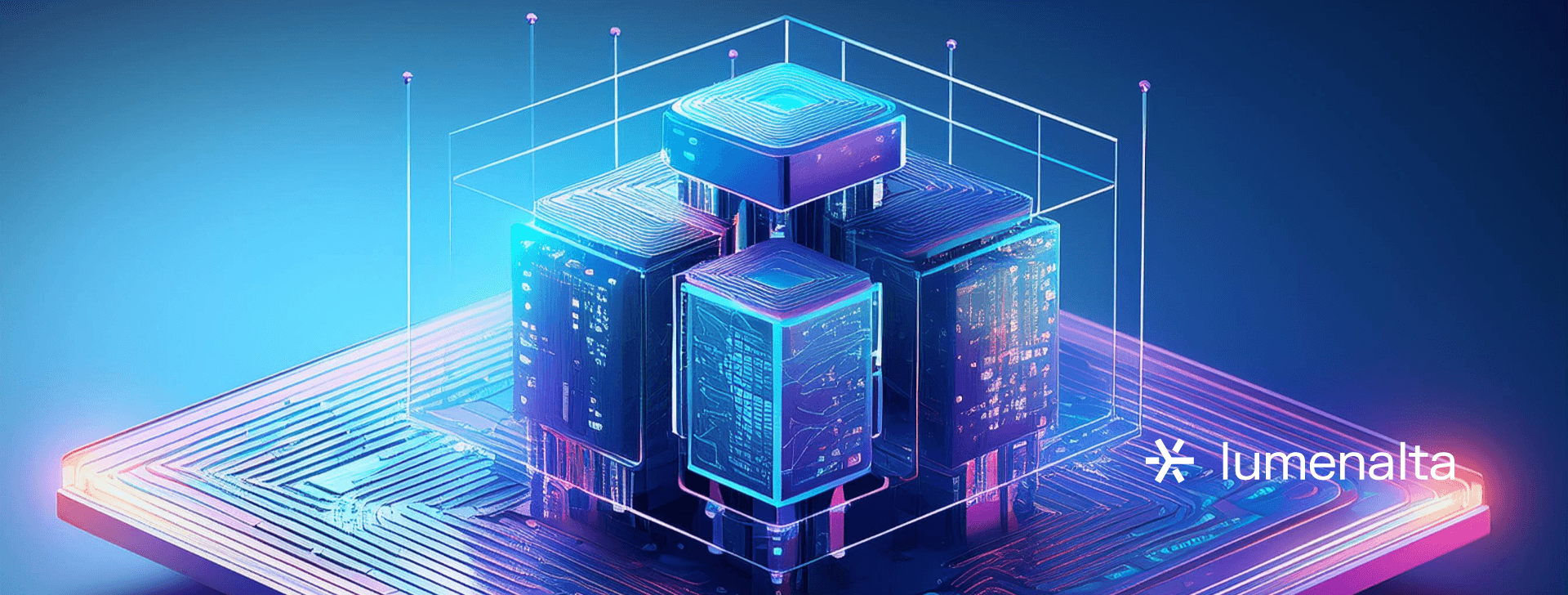
The impact of AI for portfolio management in 2025
JAN. 11, 2025
7 Min Read
AI is reshaping portfolio management by automating investment strategies, refining risk assessments, and enhancing asset allocation.
Key takeaways
- 1. AI-powered portfolio management enhances investment accuracy, automates processes, and optimizes risk management strategies.
- 2. Machine learning and predictive analytics improve asset allocation by analyzing market data in real time.
- 3. Automated trade execution enables investors to capitalize on opportunities without delays.
- 4. AI-powered risk assessment models help mitigate financial exposure and adjust portfolios accordingly.
- 5. Scalable AI solutions provide long-term investment optimization for individual and institutional investors.
How does AI in portfolio management work?
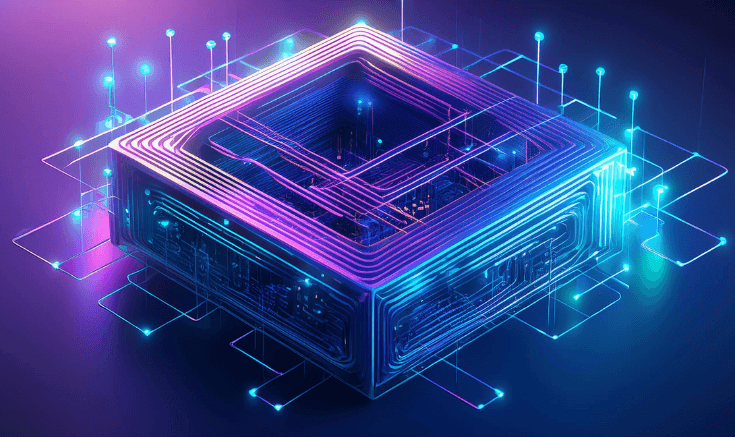
AI in portfolio management uses advanced algorithms, machine learning models, and real-time data analytics to optimize investment decisions, minimize risk, and improve returns. Traditional methods rely on human analysts and historical trends, but artificial intelligence processes vast amounts of data identifies patterns, and adjusts portfolios more precisely.
Portfolio optimization AI leverages predictive modeling, sentiment analysis, and quantitative finance techniques to enhance evaluation. These tools analyze financial indicators, global economic trends, and market sentiment in real time to provide actionable insights. AI-powered systems also automate rebalancing, ensuring portfolios align with investment goals and risk tolerance.
Artificial intelligence in portfolio management continuously refines strategies based on live market data, reducing inefficiencies and human bias. This adaptability allows investors to respond to market fluctuations more effectively, achieving better long-term financial outcomes.
“AI is reshaping portfolio management by automating investment strategies, refining risk assessments, and enhancing asset allocation.”
Differences between traditional portfolio management and AI in portfolio management
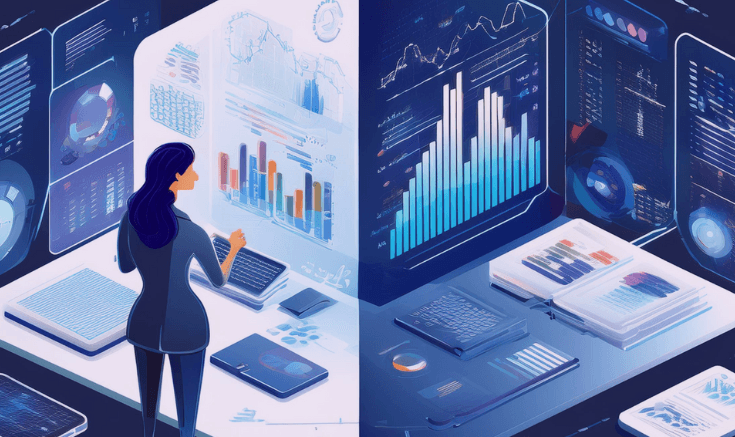
Traditional portfolio management depends on human expertise and past data trends to guide investment decisions. Analysts examine economic conditions, corporate performance, and market behaviors to construct and rebalance portfolios. While this method has been effective for decades, it requires significant manual effort and is subject to personal biases and processing limitations.
AI for portfolio management, in contrast, applies machine learning models, algorithmic trading, and extensive data analytics to refine investment strategies. These systems process vast datasets instantly, recognizing emerging patterns and executing trades precisely. AI-based portfolio adjustments are dynamic, responding to market changes as they happen and optimizing asset allocation with greater accuracy.
Feature | Traditional portfolio management | AI in portfolio management |
---|---|---|
Decision process | Human expertise, historical data | Algorithmic models, real-time data |
Data scope | Limited to past trends and reports | Comprehensive analysis of live and historical data |
Risk control | Based on predefined static models | Continuous adaptation using predictive analysis |
Trade execution | Slower, dependent on human intervention | Automated, immediate responses to market shifts |
Bias and limitations | Prone to subjective judgment | Data-backed assessments that reduce errors |
AI-based portfolio management provides a more scalable and precise investment approach. The ability to analyze financial indicators in real time, execute trades efficiently, and adapt to shifting market conditions offers significant advantages for investors looking to improve returns and mitigate risk. As financial markets become more complex, AI continues refining strategies, making portfolio management more data-focused and efficient.
Use cases of AI in portfolio management
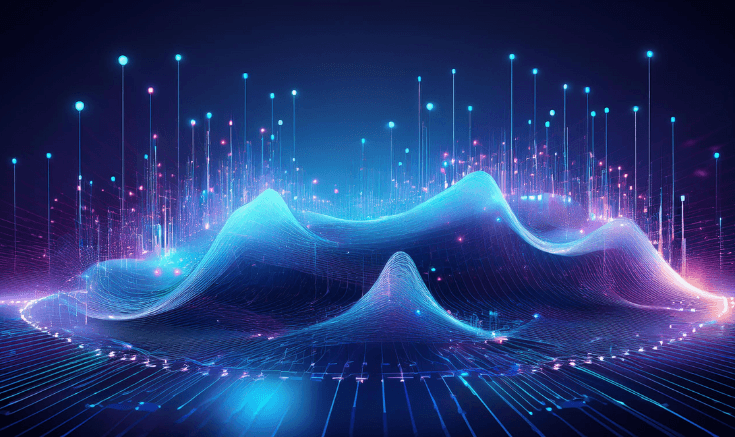
AI enhances portfolio management by streamlining processes, improving accuracy, and optimizing investments. Various applications showcase AI's practical benefits to financial evaluation and operational efficiency.
- Automated asset allocation: AI models analyze financial data to adjust portfolios based on risk tolerance and market conditions, reducing manual intervention.
- Predictive market analysis: AI-led algorithms detect trends and accurately forecast market movements, helping investors make informed choices.
- Risk assessment and mitigation: AI systems evaluate volatility, economic indicators, and historical data to recommend portfolio adjustments that align with investor goals.
- Sentiment analysis for investment decisions: AI scans financial news, earnings reports, and social media to gauge market sentiment and anticipate potential stock movements.
- Trade execution optimization: AI-powered systems automate trade execution to capitalize on optimal pricing and minimize transaction costs.
- Portfolio rebalancing automation: AI-led solutions monitor portfolio performance and reallocate assets to maintain the desired risk-return balance.
- Alternative data integration: AI incorporates non-traditional data sources, such as satellite imagery and consumer behavior patterns, to enhance investment strategies.
AI-powered tools make portfolio management more effective by automating complex tasks and refining investment decisions. These applications allow investors to reduce inefficiencies, gain deeper insights, and achieve better returns cost-effectively and scalable.
Role of AI in different types of portfolio management
AI is shaping multiple investment strategies, improving efficiency, and refining analytical capabilities. Different management styles benefit in specific ways, using AI tools to improve financial outcomes and operational execution.
Active portfolio management
Active investing relies on precise, real-time market insights to capitalize on short-term opportunities. AI-driven analytics play a critical role in this approach by continuously scanning global financial markets, identifying patterns, and making rapid trading decisions. Algorithmic trading powered by AI can execute thousands of trades in milliseconds, adjusting positions based on market sentiment, news analysis, and technical indicators.
Additionally, AI models assist in portfolio rebalancing by predicting optimal allocation shifts in response to economic events, helping investors reduce risk while maximizing returns. Advanced deep learning algorithms also integrate alternative data sources, such as social media sentiment and earnings call transcripts, to uncover hidden investment opportunities and gain an edge in volatile markets.
Passive portfolio management
Index-based investing and passive strategies benefit significantly from AI-driven automation. AI enhances asset allocation by ensuring portfolios closely track their benchmark indices with minimal tracking errors. Machine learning models optimize tax-loss harvesting strategies, dynamically identifying and selling underperforming assets to offset capital gains and improve post-tax returns.
Additionally, AI-powered tools automate dividend reinvestment and long-term performance monitoring, ensuring efficient portfolio maintenance. Robotic process automation (RPA) further streamlines administrative tasks, reducing human intervention and lowering management costs. As passive investing grows in popularity, AI-driven rebalancing ensures portfolios remain aligned with index changes, adapting to market shifts while minimizing transaction fees.
Quantitative portfolio management
Quantitative investing leverages AI to refine systematic trading strategies by analyzing vast datasets, identifying inefficiencies, and executing trades based on predictive models. AI enhances back testing by simulating how strategies would have performed under historical market conditions, allowing investors to fine-tune algorithms before deploying them in live markets.
Statistical arbitrage strategies benefit from AI’s ability to identify short-term pricing anomalies across global exchanges, executing trades that capitalize on market inefficiencies. Furthermore, AI-driven predictive analytics improve decision-making by forecasting asset price movements, volatility trends, and macroeconomic shifts. With reinforcement learning techniques, AI models continuously adjust investment strategies, ensuring they remain resilient to changing market conditions.
Factor-based portfolio management
Factor investing relies on identifying fundamental drivers of returns, such as value, momentum, and quality. AI enhances this strategy by analyzing vast datasets, assessing economic indicators, and detecting asset correlations that human analysts might overlook. Machine learning models dynamically refine factor weightings, ensuring optimal exposure to high-performing assets while mitigating concentration risks. AI-powered diversification strategies adjust holdings in real time, accounting for shifts in market conditions and macroeconomic variables. Additionally, sentiment analysis tools assess news reports, earnings transcripts, and analyst opinions to gauge investor sentiment around factor-based investments, improving decision-making accuracy. By continuously processing new information, AI ensures factor-based strategies remain adaptive and aligned with market trends.
Risk-adjusted portfolio management
AI enhances risk management by providing deep insights into market volatility, liquidity conditions, and credit ratings. Advanced machine learning models analyze historical downturns, identifying patterns that signal potential financial distress and enabling proactive risk mitigation. AI-driven stress-testing models assess portfolio resilience under different economic scenarios, allowing investors to adjust allocations to preserve targeted return levels. Portfolio stability is further enhanced through AI-powered risk control tools, which monitor real-time fluctuations and automatically adjust positions to hedge against downside risks.
Additionally, AI improves credit risk assessment by analyzing alternative datasets—such as transaction records, economic indicators, and geopolitical developments—enabling more accurate predictions of default probabilities and creditworthiness. This sophisticated risk analysis empowers investors to maintain balanced portfolios while minimizing exposure to adverse market conditions.
AI continues to expand its role across multiple investment approaches, improving financial analysis, trade execution, and risk assessment. With the ability to process vast amounts of data, refine asset allocations, and execute strategies with precision, AI improves efficiency and scalability across portfolio management practices.
Benefits of AI for portfolio management
AI reshapes portfolio management by optimizing efficiency, improving accuracy, and automating complex processes. Investors and financial institutions use AI-powered solutions to enhance risk assessment, asset allocation, and trade execution. These advancements allow for better investment strategies that adapt to market conditions while reducing manual effort and operational costs.
- Higher accuracy in portfolio analysis: AI portfolio optimization processes vast amounts of financial data precisely, minimizing miscalculations and refining asset allocation strategies.
- Faster execution of trades: Automated trading platforms execute buy and sell orders instantly, allowing investors to react to market changes without delays.
- Stronger risk mitigation: AI assesses historical data and market indicators to refine risk management techniques, helping investors avoid unnecessary exposure.
- Data-backed investment insights: AI continuously analyzes financial trends, offering actionable insights that support strategic portfolio adjustments.
- Greater scalability for asset management: AI systems handle extensive datasets efficiently, making portfolio management more effective for individual investors and large institutions.
- Reduced operational expenses: Automation decreases reliance on manual processes, lowering costs while improving accuracy and efficiency.
- Adaptive portfolio strategies: AI refines investment models based on real-time market data, helping portfolios remain aligned with developing financial goals.
Artificial intelligence portfolio management provides measurable advantages that go beyond traditional investment strategies. The ability to automate processes, refine asset selection, and execute trades precisely makes AI an essential tool for investors looking to optimize returns while minimizing risks. As AI technology progresses, its role in financial management will further expand, delivering even greater efficiency and accuracy in portfolio management.
“AI-based portfolio rebalancing improves asset allocation by adjusting investments in response to market shifts.”
Implementing AI for portfolio management
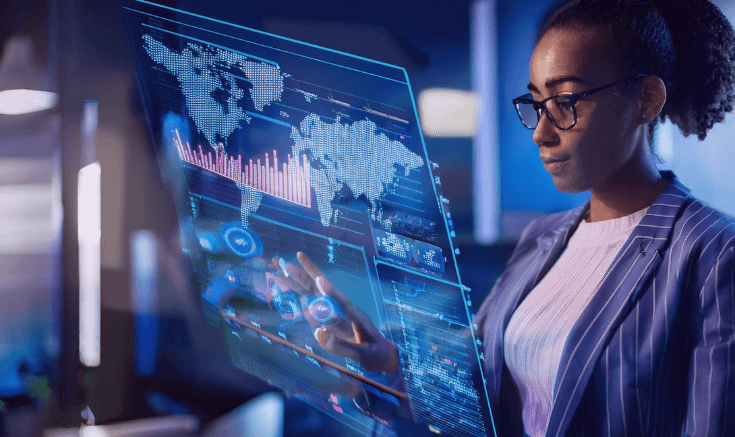
Integrating AI into portfolio management involves multiple strategic considerations to optimize its effectiveness. Financial institutions and investors must focus on selecting the right AI models, aligning investment strategies, and ensuring seamless adoption within existing frameworks. Proper implementation enhances risk analysis, improves forecasting accuracy, and automates complex tasks for better portfolio performance.
Selecting AI models for portfolio management
AI models vary in capabilities, with some excelling at predictive analytics while others focusing on automation or sentiment analysis. Investors need to identify models that align with their objectives, such as:
- Machine learning algorithms: Analyze historical and real-time market data to identify profitable investment patterns.
- Natural language processing (NLP): Extract insights from financial news, earnings reports, and market sentiment to refine investment strategies.
- Reinforcement learning models: Continuously adapt based on market conditions, making autonomous trading decisions.
- Deep learning networks: Process large volumes of data to detect patterns and forecast long-term market trends.
Integrating AI into existing investment frameworks
Seamless integration of AI tools requires aligning them with portfolio management workflows. Key considerations include:
- Data infrastructure: AI systems rely on high-quality financial data for accurate analysis, requiring robust data pipelines.
- Regulatory compliance: Investment firms must adhere to financial regulations while implementing AI-powered decision support tools.
- Customizable AI solutions: AI models should be adaptable to specific investment strategies rather than relying on generic configurations.
- Stakeholder training: To leverage them effectively, portfolio managers and analysts must understand AI-powered insights.
Automating portfolio rebalancing with AI
AI-based portfolio rebalancing improves asset allocation by adjusting investments in response to market shifts. This automation enhances investment performance through:
- Continuous monitoring: AI tracks asset performance in real-time, identifying when adjustments are necessary.
- Risk-based reallocation: AI models adjust portfolios based on growing risk tolerance and financial goals.
- Cost efficiency: Automated trading reduces manual intervention, lowering transaction costs.
- Enhanced diversification: AI optimizes asset distribution across sectors, minimizing exposure to volatility.
AI implementation in portfolio management strengthens investment strategies, enhances accuracy, and provides an edge. Financial institutions and investors adopting AI gain greater efficiency, reduced risks, and improved scalability in asset management. As AI technology progresses, its impact on portfolio management will continue to expand, reshaping investment strategies for long-term financial growth.
Choosing the right AI portfolio system
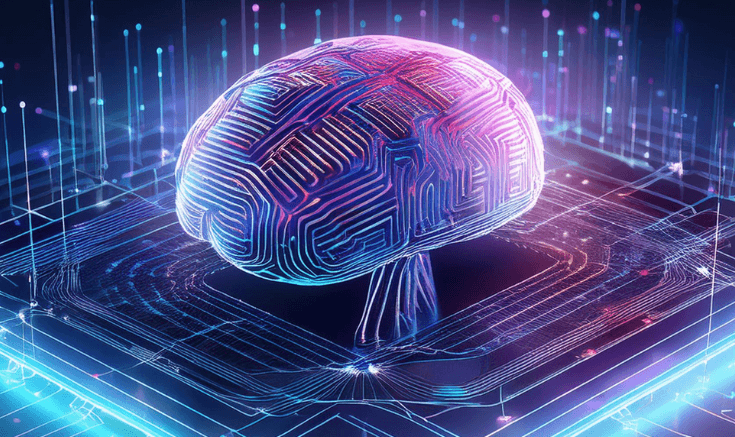
Selecting the best AI-powered portfolio management system requires a detailed evaluation of available options. Investors and financial firms must consider key factors to maximize efficiency, improve forecasting accuracy, and automate investment processes. Choosing the right system helps optimize asset allocation, reduce risks, and align portfolios with financial goals.
- Accuracy and predictive capabilities: The AI system should leverage machine learning algorithms to analyze historical and real-time market data, improving investment forecasting and risk assessments.
- Data integration and compatibility: Seamless integration with existing financial platforms and databases allows AI models to access relevant market data for optimal performance.
- Customization and adaptability: A flexible AI system should be configurable to support different investment strategies, from active trading to long-term portfolio management.
- Automation features: AI-led portfolio systems should provide automated trade execution, risk monitoring, and asset rebalancing to streamline investment operations.
- Regulatory compliance and security: The AI system must comply with financial regulations and maintain data security protocols to protect sensitive investment information.
- Scalability and performance: AI platforms should be capable of handling large volumes of financial data and expanding with an investor’s portfolio needs over time.
- User-friendly interface and insights: Portfolio managers benefit from AI systems that present actionable insights through intuitive dashboards and reports.
Choosing the most suitable AI portfolio system allows investors to automate trading, refine risk assessments, and enhance financial outcomes. With the right AI-powered solution, portfolio management becomes more efficient, accurate, and aligned with long-term investment objectives. As AI technology develops, financial institutions and individual investors can leverage these advancements to optimize asset performance and maintain a strategic advantage in the market.
AI redefines investment strategies, making portfolio management more efficient, accurate, and scalable. Integrating AI-powered tools allows investors and financial institutions to optimize asset allocation, automate trade execution, and refine risk management. At Lumenalta, we build AI-focused financial solutions to align with your investment goals, ensuring you stay ahead in a data-centric market. Let’s drive measurable results together.
table-of-contents
- How does AI in portfolio management work?
- Differences between traditional portfolio management and AI in portfolio management
- Use cases of AI in portfolio management
- Role of AI in different types of portfolio management
- Benefits of AI for portfolio management
- Implementing AI for portfolio management
- Choosing the right AI portfolio system
- Common questions about AI for portfolio management
Common questions about AI for portfolio management
How does AI improve portfolio optimization?
What are the key features of AI-powered portfolio management?
Can AI help in risk management for investments?
Is AI-based portfolio management suitable for all investors?
What should investors consider when choosing an AI portfolio system?
Want to learn how AI can bring more transparency and trust to your operations?