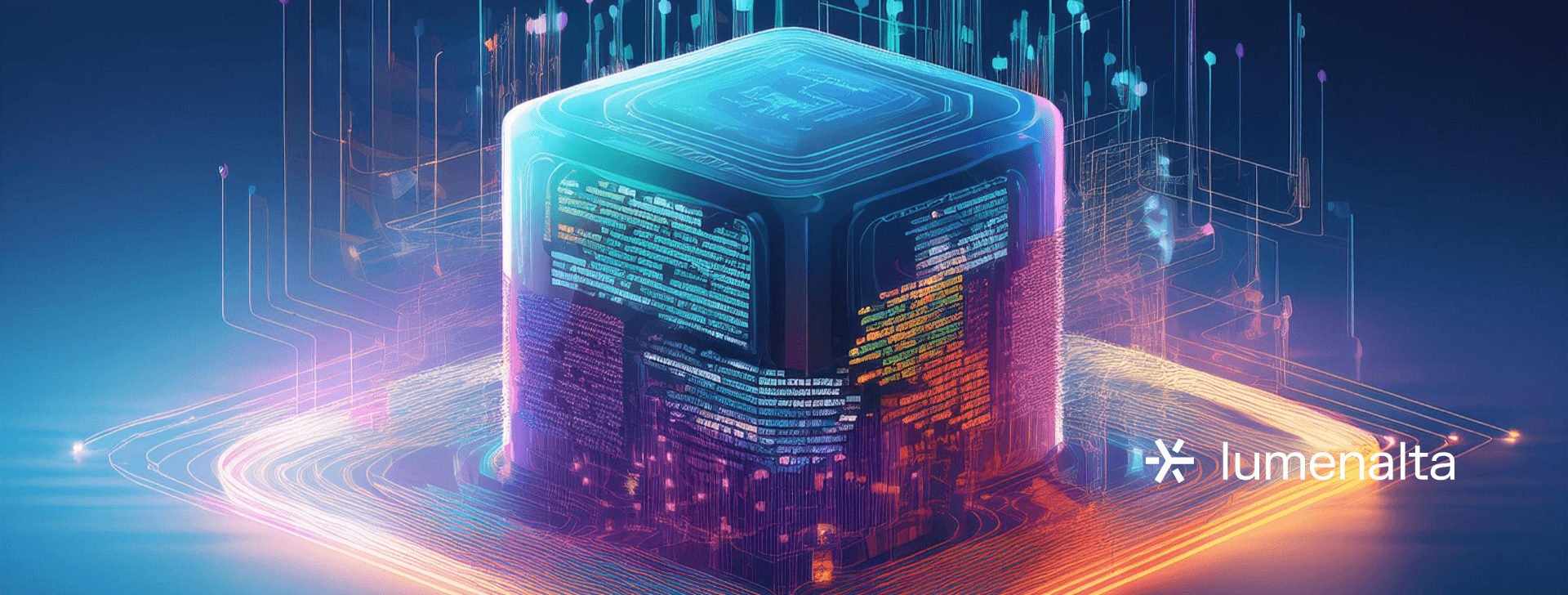
27 natural language processing use cases by industry (updated 2025)
JAN. 17, 2025
6 Min Read
Natural language processing (NLP) is redefining how businesses interact with text and speech-based data, making automation more precise and insights more accessible.
Companies across industries rely on NLP to analyze customer sentiment, optimize operations, and improve user experiences. From enhancing fraud detection in finance to streamlining clinical documentation in healthcare, NLP applications continue to expand, offering tangible efficiency gains. Organizations implementing NLP-powered solutions benefit from faster management, reduced operational costs, and more accurate data analysis. As advancements in AI refine language models, businesses must align their strategies to maximize the impact of NLP in both customer-facing and internal operations.
Key takeaways
- 1. NLP automates text and speech analysis, making processes more efficient while improving accuracy in finance, healthcare, and retail industries.
- 2. Businesses use NLP to extract insights from unstructured data, optimizing operations, and improving customer experiences.
- 3. Chatbots, sentiment analysis, and voice-enabled assistants are among the most impactful NLP applications in customer service and marketing.
- 4. NLP improves fraud detection, contract analysis, and document processing, reducing manual effort while increasing security and compliance.
- 5. Custom NLP models tailored to industry-specific needs provide businesses with measurable advantages in automation, efficiency, and data-led strategies.
What is natural language processing (NLP)?
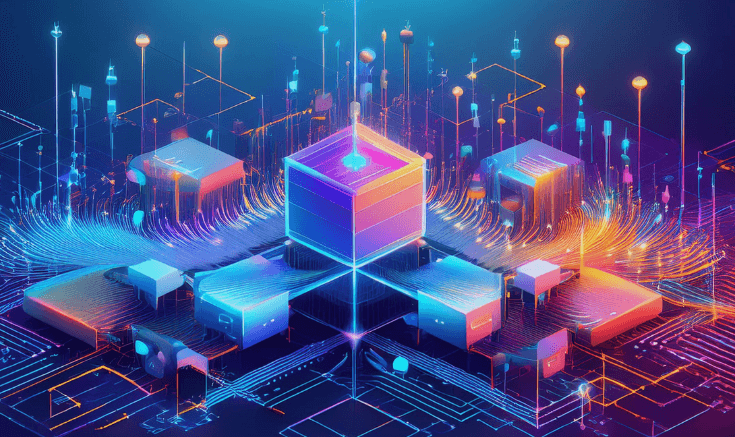
Companies use NLP to extract meaning from unstructured text, detect patterns, and structure language-based information in an actionable way. It applies machine learning and deep learning techniques to enhance accuracy in speech recognition, sentiment analysis, and text summarization. These capabilities improve operational efficiency by streamlining communication and automating data-based insights.
"Natural language processing (NLP) is redefining how businesses interact with text and speech-based data, making automation more precise and insights more accessible."
Importance of NLP in modern industries
Businesses across multiple industries are implementing NLP to reduce costs, increase efficiency, and make data easier to interpret. NLP allows the automation of routine tasks, allowing teams to focus on higher-value initiatives. Organizations that rely on vast amounts of text-based information, such as healthcare providers, financial institutions, and e-commerce platforms, benefit from NLP for tasks like document analysis, risk assessment, and customer engagement.
Retailers optimize product recommendations using NLP-powered systems that analyze customer reviews and search patterns. Financial institutions apply NLP to detect fraudulent transactions by identifying anomalies in text-based data. In healthcare, NLP improves clinical documentation by structuring physician notes into actionable insights. Marketing professionals use NLP for sentiment analysis, helping brands refine messaging based on customer perception.
NLP helps businesses extract insights, improve response times, and automate language-based operations. As adoption increases, companies find new ways to integrate NLP into existing workflows to improve accuracy, lower costs, and optimize user experiences.
27 natural language processing use cases by industry
Organizations across multiple industries use natural language processing to improve efficiency, enhance user experiences, and extract insights from unstructured data. NLP-powered systems automate text analysis, streamline communication, and optimize customer interactions, reducing manual effort and increasing scalability. Businesses apply NLP in customer support, fraud detection, personalized marketing, and healthcare documentation.
The following sections highlight how different industries use NLP to improve operations, maximize efficiency, and expose new business opportunities.
NLP in e-commerce
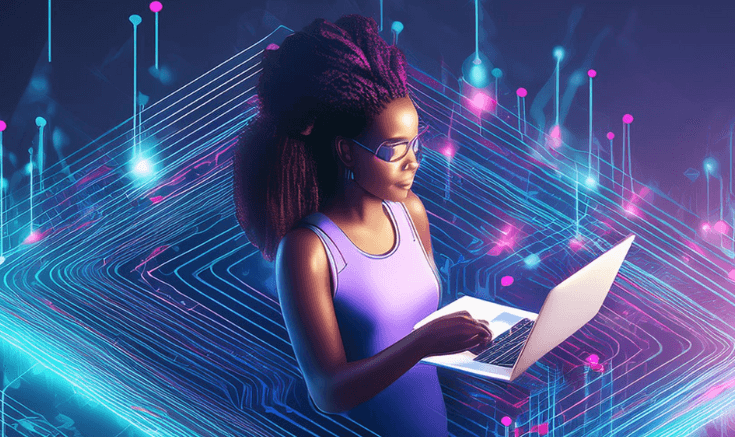
1. Personalized product recommendations
E-commerce platforms use NLP to analyze customer search queries, past purchases, and product reviews, delivering personalized recommendations tailored to individual preferences. Algorithms identify patterns in user behavior, ensuring that search results and product suggestions align with customer interests.
Shoppers receive targeted recommendations that improve their browsing experience and increase the likelihood of purchases. NLP enhances recommendation accuracy by considering sentiment from product reviews, allowing businesses to refine their suggestions based on real user feedback. This approach strengthens customer satisfaction while increasing sales conversions.
2. Chatbots for customer service
AI-powered chatbots use NLP to interpret customer inquiries and provide relevant responses in real time. These systems handle order tracking, refund requests, and product-related questions without human intervention. Advanced NLP models improve chatbot responses by understanding context, intent, and sentiment, resulting in more meaningful interactions.
Businesses reduce operational costs by automating customer service while maintaining 24/7 availability. Customers benefit from faster resolutions, and support teams can focus on complex inquiries that require human expertise.
3. Analyzing product reviews and sentiment
NLP processes large volumes of customer reviews to assess sentiment, detect recurring issues, and identify emerging trends. Businesses use this data to refine product features, adjust pricing strategies, and enhance customer experience.
Sentiment analysis categorizes feedback as positive, negative, or neutral, helping companies understand how customers perceive their offerings. This insight allows e-commerce brands to address concerns, improve product quality, and respond proactively to customer needs.
NLP in retail
4. Demand forecasting using customer feedback
Retailers apply NLP to analyze customer reviews, social media mentions, and support inquiries, identifying trends influencing purchasing behavior. This data helps businesses anticipate the need for specific products, ensuring stock levels align with market needs.
Traditional forecasting methods rely on historical sales data, but NLP enhances accuracy by incorporating sentiment analysis and contextual insights from customer feedback. Retailers adjust inventory levels and marketing strategies based on real-time sentiment shifts, reducing overstocking or shortages.
5. Voice-assisted shopping experiences
Retailers integrate NLP into voice-enabled shopping assistants that help customers search for products, place orders, and receive personalized recommendations. These systems recognize natural speech patterns, improving accuracy in voice search queries and product discovery.
Voice-enabled shopping simplifies the buying process, offering a hands-free experience for customers. Retailers benefit from higher engagement rates, as voice search allows shoppers to find relevant products faster, reducing friction in the purchasing journey.
6. Optimizing inventory management with text data
Using NLP, retailers extract valuable insights from supplier emails, purchase orders, and customer inquiries. These insights inform inventory decisions, reducing inefficiencies in stock management. Automated text analysis identifies patterns in order fulfillment, supplier performance, and customer need fluctuations.
Retailers maintain optimal inventory levels by automating the analysis of text-based data sources. NLP reduces supply chain inefficiencies, prevents stockouts, and improves responsiveness to shifting customer preferences.
NLP in healthcare
7. Processing clinical notes and records
Healthcare providers use NLP to extract structured information from unstructured clinical notes, streamlining documentation and reducing administrative burdens. Physicians and nurses generate large volumes of text-based records, including patient histories, diagnostic reports, and treatment plans. NLP automates data extraction, ensuring key details are organized and accessible.
Free-text medical records become more valuable when converted into structured formats, improving diagnosis coding, billing, and patient management accuracy. NLP enhances efficiency in electronic health record (EHR) systems, reducing paperwork and allowing medical professionals to focus more on patient care.
8. Voice-enabled patient monitoring systems
NLP powers voice-based monitoring systems that track patient symptoms, medication adherence, and general well-being. These systems analyze spoken language to detect changes in speech patterns, which may indicate health issues such as cognitive decline or respiratory conditions.
Continuous monitoring allows patients to receive proactive care without frequent in-person visits, improving accessibility to healthcare. Providers receive real-time insights into patient health, allowing for early intervention and personalized treatment adjustments.
9. Medical literature mining for drug discovery
Pharmaceutical companies and research institutions rely on NLP to analyze vast scientific literature, identifying patterns and relationships that accelerate drug discovery. Research papers, clinical trial reports, and patent filings contain critical insights that are difficult to process manually.
Automated literature reviews highlight relevant findings and reveal potential drug interactions or treatment advancements. This approach accelerates research timelines, reduces costs, and enhances the ability to identify promising drug candidates more efficiently.
NLP in marketing
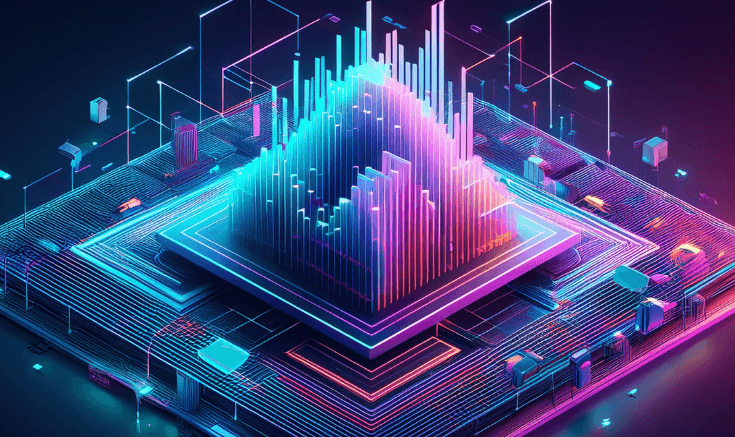
10. Analyzing social media sentiment
Marketers apply NLP to process social media posts, comments, and reviews, identifying customer sentiment toward products and campaigns. Sentiment analysis categorizes feedback as positive, negative, or neutral, helping businesses measure brand perception and detect emerging trends.
Understanding sentiment in real time allows marketing teams to adjust messaging, address concerns, and capitalize on positive feedback. Automated sentiment tracking improves campaign effectiveness and provides a deeper understanding of consumer preferences.
11. Creating personalized email campaigns
NLP enhances email marketing by analyzing customer interactions and crafting personalized messages based on past behaviors, interests, and engagement patterns. Automated systems refine subject lines, predict customer responses, and segment audiences more precisely.
Personalized email content increases open rates, boosts conversions, and improves customer retention. NLP-based automation ensures that messaging remains relevant, leading to stronger relationships between brands and consumers.
12. Identifying emerging market trends
Marketing teams use NLP to analyze industry reports, customer feedback, and news articles, revealing trends that influence consumer behavior. Automated text analysis reveals patterns in purchasing habits, dynamic positioning, and shifts in audience preferences.
Access to real-time insights gives businesses an advantage in adjusting strategies ahead of market shifts. NLP provides a data-powered approach to identifying trends, reducing reliance on manual research and allowing brands to make informed marketing decisions faster.
NLP in finance and banking
13. Fraud detection in transactions
Financial institutions use NLP to detect fraudulent activities by analyzing transaction descriptions, customer communication, and behavioral patterns. Suspicious transactions often contain subtle linguistic cues that indicate fraudulent intent, such as unusual phrasing in payment memos or inconsistencies in documentation.
NLP enhances fraud detection by identifying anomalies in text-based financial data, strengthening security measures without requiring extensive manual review. This proactive approach minimizes financial risk and improves fraud prevention accuracy.
14. Automated financial report generation
Banks and financial analysts rely on NLP to automate the creation of financial reports, extracting key figures and insights from earnings statements, regulatory filings, and market analysis documents. These systems summarize large volumes of data, providing concise and actionable reports in less time.
Automated report generation reduces manual effort, accelerates financial analysis, and ensures consistency in reporting. NLP-led insights allow decision-makers to focus on strategy rather than data processing, improving overall efficiency.
15. Enhancing chatbot-based customer service
Financial institutions integrate NLP-powered chatbots to assist customers with account inquiries, transaction history, and loan applications. These chatbots understand natural language, permitting seamless interactions without requiring customers to handle complex menus or wait for human agents.
Improved chatbot accuracy leads to faster resolutions, higher customer satisfaction, and reduced support costs. NLP ensures that responses are contextually relevant, making automated customer service more reliable and user-friendly.
NLP in recruitment and HR
16. Resume parsing for candidate screening
Recruiters use NLP to scan resumes, extracting relevant skills, experience, and qualifications to match candidates with job openings. Automated resume parsing eliminates the need for manual data entry, allowing hiring teams to focus on evaluating top talent instead of sorting through large applicant pools.
Advanced NLP models categorize resumes based on predefined job requirements, improving hiring efficiency. This approach accelerates the screening process, ensuring that qualified candidates are identified quickly and reducing time-to-hire.
17. Conducting sentiment analysis on employee feedback
HR teams analyze employee feedback from surveys, emails, and internal communication platforms using NLP to assess workplace sentiment. Understanding employee concerns helps organizations address workplace issues, improve engagement, and enhance retention strategies.
Sentiment analysis identifies patterns in employee satisfaction and reveals areas where improvements may be needed. Companies prioritizing workplace feedback gain valuable insights that contribute to a positive work setting and a stronger organizational culture.
18. Automating interview transcription and analysis
NLP-powered tools transcribe interviews in real time, allowing HR teams to focus on candidate responses instead of manual note-taking. These systems identify key topics, extract relevant information, and assess communication skills by analyzing speech patterns and tone.
Automated transcription improves hiring efficiency by providing structured interview summaries. HR teams benefit from accurate records of candidate responses, allowing for better comparisons and informed hiring decisions.
General NLP examples
19. Translating languages in real time
NLP permits instant language translation, making communication seamless across different regions and languages. Businesses use real-time translation tools to support international customer service, global collaboration, and multilingual content generation.
Improving translation accuracy allows companies to reach wider audiences without language barriers. NLP-based translation systems process complex sentence structures, dialects, and contextual nuances, ensuring messages remain clear and culturally appropriate.
20. Summarizing lengthy documents
Legal, financial, and research industries use NLP to condense long reports, contracts, and articles into concise summaries. Automated text summarization extracts key information, reducing the time required to review extensive documents.
Providing quick access to essential details improves productivity and evaluation. Professionals can focus on critical insights without manually parsing large volumes of text, streamlining workflows across various industries.
21. Extracting key information from contracts
NLP systems identify important contract clauses, obligations, and deadlines, reducing manual contract review time. Businesses use automated contract analysis to ensure compliance, detect risks, and simplify negotiations.
Enhancing contract analysis improves efficiency in legal and procurement teams. NLP reduces errors, speeds up contract processing, and highlights critical terms for review.
22. Powering virtual assistants like Siri and Alexa
NLP grants voice assistants to process spoken commands, retrieve information, and complete tasks based on user input. These AI-backed assistants recognize speech patterns, understand context, and provide accurate responses in real time.
Improving virtual assistant accuracy makes voice interactions more natural and efficient. Users rely on these systems for scheduling, search queries, and smart home control, enhancing convenience in daily tasks.
23. Enhancing search engines with semantic understanding
Search engines use NLP to interpret user queries beyond basic keyword matching. Semantic search technology understands intent, synonyms, and context, delivering more relevant user results.
Improved search accuracy helps users find information faster and more effectively. NLP enhances search engine ranking algorithms, ensuring high-quality content appears based on relevance rather than keyword density.
24. Moderating and filtering online content
Social media platforms, forums, and customer review sites use NLP to detect inappropriate content, spam, and harmful language. Automated moderation ensures compliance with community guidelines while improving user safety.
Filtering harmful or misleading content improves platform trust and engagement. NLP-powered moderation allows businesses to maintain brand integrity while reducing manual content review efforts.
25. Providing accessibility through speech-to-text systems
Speech-to-text technology powered by NLP improves accessibility for individuals with hearing impairments, allowing them to engage with audio content, meetings, and conversations in real time. Businesses implement speech-to-text tools to support inclusivity in workplaces and digital platforms.
Accurate transcription services enhance accessibility across industries, ensuring information is available to a broader audience. NLP-led speech recognition improves customer support, education, and professional communication efficiency.
26. Powering natural language question-answering systems
NLP allows AI-powered question-answering systems that retrieve precise answers from large datasets, knowledge bases, or online sources. These systems are used in chatbots, virtual assistants, and enterprise search solutions.
Providing instant responses to complex queries improves knowledge management and customer support efficiency. Businesses benefit from faster information retrieval, reducing the need for manual research and documentation review.
27. Automating customer survey analysis
NLP processes customer survey responses, identifying common themes, sentiments, and key insights from open-ended feedback. Businesses use automated analysis to measure customer satisfaction and refine products or services.
Automating survey analysis saves time and improves accuracy in extracting meaningful insights. NLP-powered tools allow companies to effectively act on customer feedback, improving overall service and customer retention.
"Financial institutions use NLP to detect fraudulent activities by analyzing transaction descriptions, customer communication, and behavioral patterns."
Implementing NLP strategies in 2025
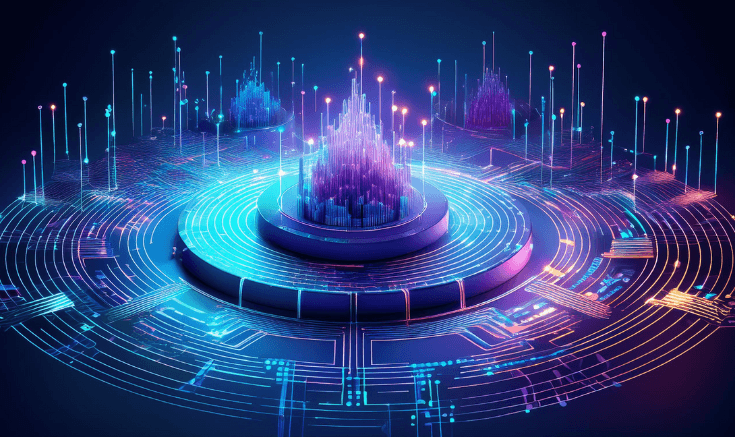
Maximizing the impact of NLP will involve automating complex text-based tasks to improve time-to-value. Real-time analytics, conversational AI, and document processing will play a more significant role in streamlining operations. Custom NLP models designed for specific business needs will allow organizations to extract meaningful insights from unstructured data while maintaining compliance and governance standards.
Seamless integration with existing systems allows NLP-backed solutions to deliver measurable results. Investing in these capabilities creates advantages such as increased efficiency, faster response times, and more effective customer interactions. As adoption continues, refining NLP strategies will remain essential for long-term scalability and sustained business impact.
Natural language processing is not just a tool—it’s a strategic advantage that improves efficiency, accuracy, and scalability in language-based automation. Extracting insights from text and speech allows businesses to improve engagement, streamline operations, and adapt faster to growing needs. At Lumenalta, we design NLP solutions that fit the needs of modern industries, ensuring organizations can focus on what matters most: delivering results. Let’s build more innovative, faster, and more adaptable systems together.
Table of contents
- What is natural language processing (NLP)?
- Importance of NLP in modern industries
- 27 natural language processing use cases by industry
- 1. Personalized product recommendations
- 2. Chatbots for customer service
- 3. Analyzing product reviews and sentiment
- 4. Demand forecasting using customer feedback
- 5. Voice-assisted shopping experiences
- 6. Optimizing inventory management with text data
- 7. Processing clinical notes and records
- 8. Voice-enabled patient monitoring systems
- 9. Medical literature mining for drug discovery
- 10. Analyzing social media sentiment
- 11. Creating personalized email campaigns
- 12. Identifying emerging market trends
- 13. Fraud detection in transactions
- 14. Automated financial report generation
- 15. Enhancing chatbot-based customer service
- 16. Resume parsing for candidate screening
- 17. Conducting sentiment analysis on employee feedback
- 18. Automating interview transcription and analysis
- 19. Translating languages in real time
- 20. Summarizing lengthy documents
- 21. Extracting key information from contracts
- 22. Powering virtual assistants like Siri and Alexa
- 23. Enhancing search engines with semantic understanding
- 24. Moderating and filtering online content
- 25. Providing accessibility through speech-to-text systems
- 26. Powering natural language question-answering systems
- 27. Automating customer survey analysis
- Implementing NLP strategies in 2025
- Common questions about NLP use cases by industry
Common questions about NLP use cases by industry
How does natural language processing improve business operations?
What are the key applications of NLP in e-commerce?
How does NLP contribute to fraud prevention in banking?
What role does NLP play in healthcare?
Why is NLP important for search engines?
Want to learn how NLP can bring more transparency and trust to your operations?