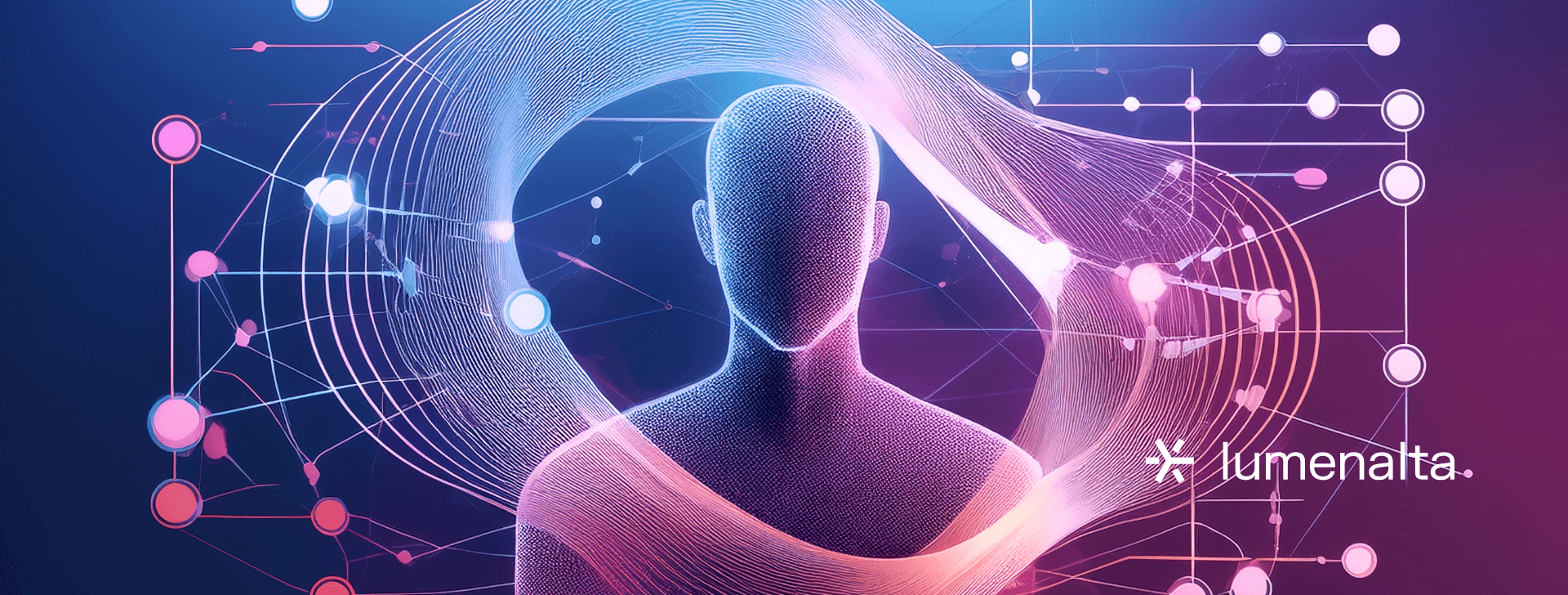
7 AI personalization examples transforming customer experiences
APR. 20, 2025
5 Min Read
AI personalization reshapes how businesses connect with audiences by serving relevant experiences that reflect each user’s needs.
Many organizations seek faster paths to value when deploying artificial intelligence, hoping for smoother integration and meaningful returns on investment. Rapid prototyping and carefully crafted data strategies often lead to greater cost-effectiveness and scalable outcomes. Stakeholders are encouraged to identify precise objectives first, then adopt metrics-powered approaches to measure how targeted recommendations or interactions impact user satisfaction. Data points such as browsing histories, purchase behaviors, and social interactions form the foundation of AI-powered features. Insights from these sources help teams innovate quickly, ensuring stakeholders feel confident in every strategic move. Operational professionals often appreciate clear guidance on implementation, especially when aiming for tangible efficiency gains. Robust governance policies and ethical guidelines also matter, supporting a transparent workflow for everyone involved.
Organizations that take advantage of well-planned AI personalization examples often achieve measurable breakthroughs in efficiency and user engagement. Predictive modeling, powered by machine learning, identifies untapped revenue streams and streamlines decision processes. As more industries experiment with advanced AI methods, the focus shifts to creating seamless experiences that resonate with different user segments. This practical mindset goes beyond technology hype, prioritizing solutions that elevate productivity and establish trust.
key-takeaways
- 1. AI-based solutions rely on user data to deliver relevant experiences across multiple touchpoints.
- 2. Predictive analytics offers guidance for inventory management, pricing decisions, and user engagement tactics.
- 3. Chatbots, virtual assistants, and email campaigns can be customized to reflect individual preferences.
- 4. Behavior analysis helps tailor interfaces that reduce friction and increase adoption rates.
- 5. Structured data strategies and periodic reviews ensure personalization initiatives remain accurate and cost-effective.
7 AI personalization examples transforming customer experiences
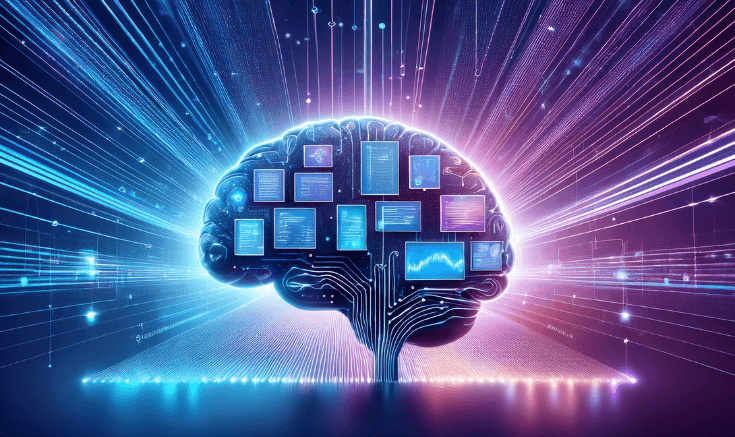
1) AI-powered chatbots enhancing customer service
Intelligent chatbots streamline conversations by analyzing user inquiries and providing quick, context-aware responses. These tools rely on natural language processing to interpret queries, reducing the need for manual intervention and accelerating resolution times. Data-led insights allow chatbots to refine responses continuously, identifying the most effective prompts for different types of interactions. This approach boosts user satisfaction and lowers operational costs linked with large-scale support teams.
Organizations benefit from this approach by offering instant customer service AI personalization that addresses user pain points immediately. Feedback loops help refine chatbot algorithms for more intuitive exchanges, forming a continuous improvement cycle. Decision-makers often value the ability to scale support services without compromising quality, especially during product launches or high-traffic events. Teams that adopt chatbots often see stronger loyalty metrics and positive word-of-mouth, reflecting the value of convenient self-service experiences.
“This approach not only boosts user satisfaction but also lowers operational costs linked with large-scale support teams.”
2) Personalized product recommendations in e-commerce
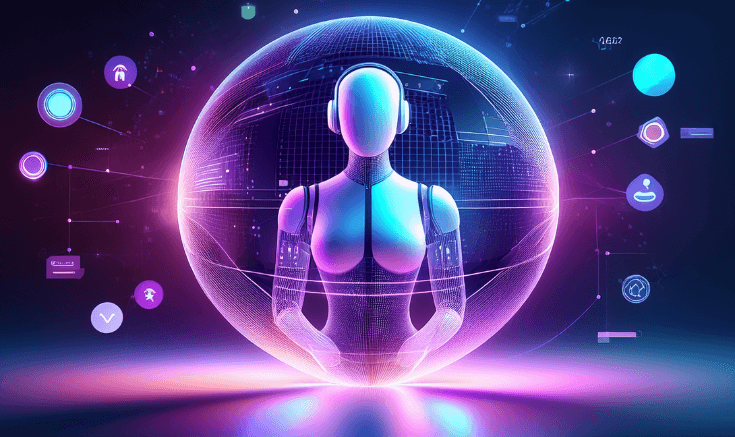
Recommendation engines rely on user preference data, browsing habits, and purchase histories to display tailor-made product suggestions. These insights integrate machine learning models that recognize similarities between users, ensuring each shopper discovers items that match personal tastes. E-commerce platforms gain agility by adapting to real-time purchasing patterns, leading to higher conversion rates and better cross-selling opportunities. A data-centric foundation allows decision-makers to adjust recommendations when consumer behaviors shift.
Shoppers often return to platforms that present thoughtful suggestions, illustrating how AI personalization examples can amplify brand stickiness. From a revenue standpoint, tailored recommendations prompt additional purchases and drive incremental upsells. Retailers benefit from advanced analytics that inform promotional strategies, minimize cart abandonment, and support better inventory management. When effectively executed, these recommendations create a shopping journey that feels welcoming and simple, resulting in improved satisfaction.
3) Dynamic content creation for targeted marketing
AI-based systems analyze user demographics, online behavior, and engagement patterns to generate marketing messages that speak directly to individual interests. These platforms can craft visual elements, headlines, or calls to action aligned with data-derived insights. Agile content delivery ensures messages adjust in real time, preventing campaigns from feeling generic or repetitive. Marketers appreciate how this refined strategy drives engagement while managing budgets efficiently.
Industries such as finance or logistics often rely on dynamic content to demonstrate value to specific segments. Streamlined processes reduce the time from ideation to deployment, creating a measurable market speed advantage. Teams leverage advanced AI personalization examples to stand apart in crowded marketplaces, catering to unique reader or viewer needs. Elevated engagement metrics prove that the right message, delivered at the right moment, resonates more effectively.
4) AI-backed email campaigns improving engagement
Email platforms equipped with intelligent algorithms assess open rates, click-through patterns, and previous user interactions to craft individualized content. Segmenting audiences based on nuanced data points allows each message to focus on specific pain points or product preferences. Automated scheduling further refines the process, ensuring users receive emails at optimal times based on past engagement patterns. This precision cuts through inbox clutter and boosts the chances of meaningful conversions.
Organizations investing in these systems often note significant gains in lead generation, especially when supported by predictive insights. Marketers regularly test various subject lines or offers, adjusting rapidly as performance metrics roll in. Scalability becomes more manageable, allowing smaller teams to handle larger subscriber bases without losing personalization quality. The final result is greater alignment between brand communications and user expectations, reinforcing the importance of customer service AI personalization.
5) Customized user interfaces based on behavior analysis
Adaptive interfaces learn from user interactions to present content layouts and navigation structures best suited for each individual. This approach often relies on A/B testing, funnel analysis, and click-path data, so the system knows how to rearrange menus or recommend shortcuts that users find most intuitive. Companies that invest in behavior-based personalization show respect for diverse user preferences, reducing friction and improving overall digital experiences. Enhanced interfaces also diminish confusion during onboarding, which can lead to faster product adoption.
Encouraging user comfort through personalized layouts produces measurable increases in engagement, including extended session durations. Teams can quickly identify which interface elements resonate with different demographics, tightening alignment between user needs and product features. Customization fosters loyalty among power users who appreciate an interface designed around their habits. Over time, refined interfaces contribute to a brand identity that values user autonomy and efficiency.
6) Predictive analytics for tailored customer experiences
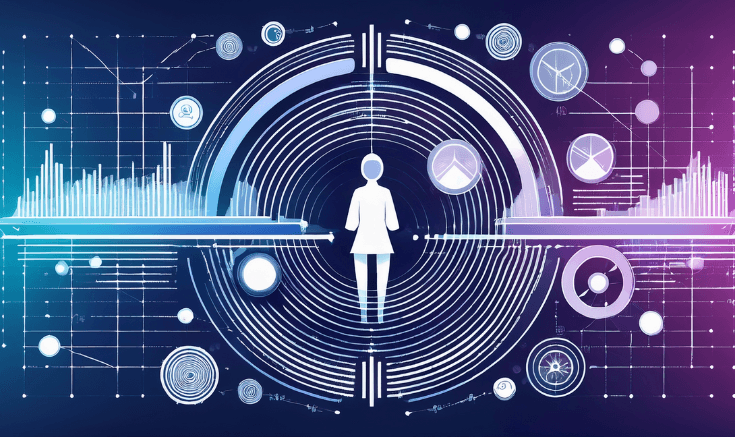
Models that forecast user needs rely on patterns found in historical and current data, such as purchase frequencies or page visits. These predictions anticipate future demands, suggesting next-best actions, promotions, or support interventions. Retailers often use this technology to inform pricing strategies, while financial services may apply it to risk assessments or personalized offers. Precision in forecasting helps stakeholders pinpoint new revenue streams and maintain better inventory control.
Managers frequently look for ways to optimize cost structures, and accurate predictions guide decisions on staffing, shipping, and production schedules. Tailored experiences stem from mapping data to specific user segments, ensuring that marketing efforts and product development stay aligned. AI personalization examples in predictive analytics underscore how small adjustments can yield significant improvements in conversion rates. Each prediction also feeds back into machine learning algorithms, making them more reliable and valuable over time.
7) AI-enhanced virtual assistants for personalized support
Virtual assistants differ from chatbots in their broader range of tasks, often serving as a hub for scheduling, resource allocation, or personal planning. These assistants collect context from voice commands, typed messages, and past behaviors, delivering a uniquely human experience. Machine learning capabilities drive continuous enhancement, refining how the assistant interprets complex queries or integrates with external apps. This comprehensive support saves users time and ensures smoother collaboration across different platforms.
Enterprises often incorporate virtual assistants to help employees manage workloads more effectively, reinforcing a culture of productivity. Higher-level tasks might include analyzing team schedules, suggesting meeting times, or reminding individuals about deadlines. Tailored recommendations based on user behavior build trust, turning virtual assistants into valuable assets rather than mere add-ons. Teams that adopt these assistants typically discover better coordination, reduced administrative tasks, and higher satisfaction among employees who need agile digital support.
Benefits of AI personalization in business
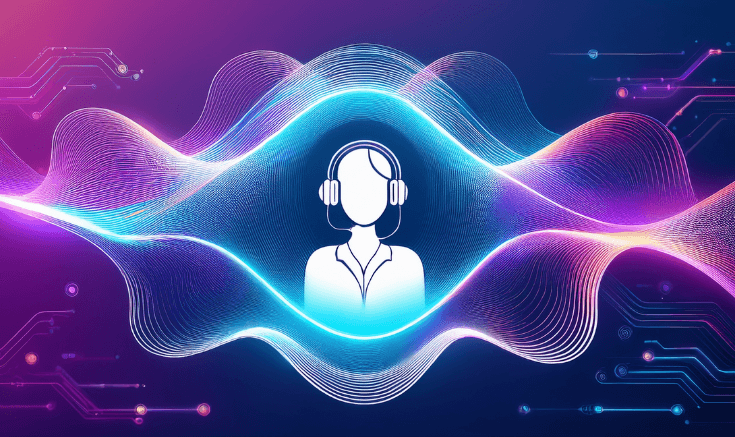
Efficient user engagement starts with a robust AI strategy that aligns with clear business objectives. Many teams embrace personalization to maximize returns, boost time to value, and reveal fresh revenue opportunities. Data intelligence guides leadership in setting practical targets, improving accuracy in marketing and operational planning. Sustainable growth emerges when personalization becomes a core function, rather than a superficial enhancement.
- Strengthened loyalty: Personalizing experiences increases user satisfaction and reduces churn.
- Optimized resource allocation: Data helps leaders assign budgets more effectively, improving productivity.
- Cost-effective results: Fewer wasted ads and streamlined workflows lead to improved profit margins.
- Faster expansions: Real-time data allows organizations to move quicker into new customer segments.
- Clear metrics: Analytics reveal which personalization tactics deliver the highest returns.
Leaders who understand these advantages plan more strategic investments, recognizing how AI personalization examples drive success. Teams often rely on iterative development, collecting frequent feedback to fine-tune each feature. When personalization efforts align with a measured approach, the entire operation benefits from steady advancements. Users appreciate consistently relevant experiences, and executives see tangible boosts in revenue, efficiency, and brand reputation.
“Data intelligence guides leadership in setting practical targets, improving accuracy in marketing and operational planning.”
Strategies for optimizing AI personalization efforts
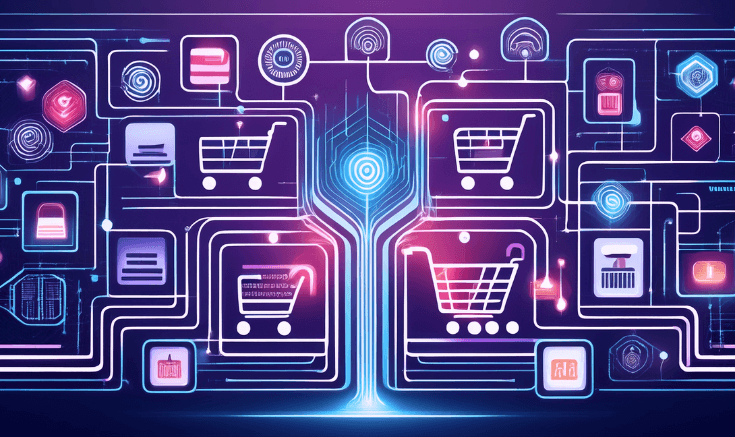
Establish clear data collection methods
Organizations often gather data from multiple sources, including e-commerce transactions, customer service platforms, and social media channels. A centralized repository ensures that each dataset remains consistent and accessible to machine learning processes. When teams prioritize transparent governance, they reduce redundancy and confusion, enabling faster iteration on personalization models. Simple metrics like conversion rates, user dwell time, and cart values help refine which data deserves priority.
Focus on transparency in algorithm design
Visibility into how algorithms function builds confidence among customers and stakeholders alike. Explaining why certain products or messages are recommended enhances user trust, encouraging repeat interactions. Continuous audits help identify biases or inaccuracies, ensuring that AI-based personalization remains fair across different demographic groups. Data scientists collaborate with compliance teams to keep user data secure, preventing privacy issues that can erode credibility.
Implement periodic review and feedback loops
AI systems learn best when they receive consistent input from users, such as star ratings or open-text feedback. Frequent reviews of performance metrics allow decision-makers to identify emerging patterns and adjust algorithms without lengthy delays. Real-time dashboards guide stakeholders in evaluating progress and deciding where to invest additional resources. This approach translates into a culture of refinement, where each iteration brings the operation closer to an optimal personalized experience.
AI personalization creates opportunities to strengthen user engagement, uncover new revenue channels, and save operational costs. Leaders who integrate advanced data practices are more equipped to detect trends, deliver relevant offerings, and reduce inefficiencies. The right solutions empower teams to adapt quickly in uncertain markets and maintain an edge. At Lumenalta, we develop customized AI strategies that align with your business priorities, ensuring that every investment drives returns. Let’s chart a brighter path together.
table-of-contents
- 7 AI personalization examples transforming customer experiences
- 1. AI-powered chatbots enhancing customer service
- 2. Personalized product recommendations in e-commerce
- 3. Dynamic content creation for targeted marketing
- 4. AI-backed email campaigns improving engagement
- 5. Customized user interfaces based on behavior analysis
- 6. Predictive analytics for tailored customer experiences
- 7. AI-enhanced virtual assistants for personalized support
- Benefits of AI personalization in business
- Strategies for optimizing AI personalization efforts
- Common questions about AI personalization examples
Common questions about AI personalization examples
How do AI personalization examples differ from traditional segmentation?
What data is essential for customer service AI personalization?
Is predictive analytics suitable for small businesses?
Can AI-led personalization reduce operational costs?
How can data privacy concerns be addressed when implementing AI personalization examples?
Want to learn how AI personalization can bring more transparency and trust to your operations?