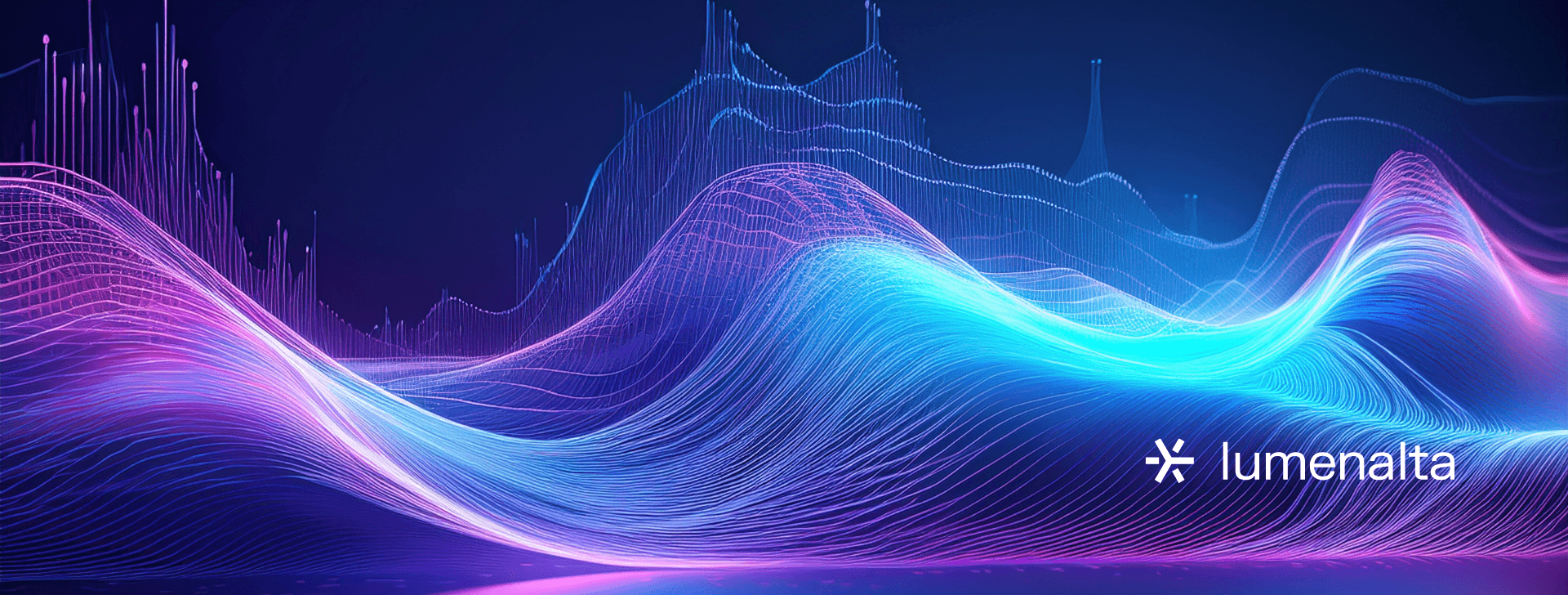
A guide to big data analytics for telecom
APR. 17, 2025
7 Min Read
Big data analytics for telecom drives strategic decisions so carriers can respond more precisely to shifting market conditions.
Telecom operators often manage massive volumes of call data records, network logs, and customer interactions. Timely analysis of this information helps you reduce churn, improve service quality, and discover untapped revenue potential. Technical leaders often find that targeted data analytics telecom solutions reduce operational costs and align teams with measurable goals. Focus on strategic outcomes converts broad data sets into actionable intelligence for BDMs. Modern analytics platforms integrate with existing IT infrastructure to expedite project delivery while maximizing returns. These innovations establish real-time insights, helping you uncover performance issues before they affect subscriber experiences. Implementing big data analytics in telecom industry settings offers an important advantage for companies seeking sustained revenue growth and dependable operational efficiency.
key-takeaways
- 1. Big data analytics for telecom bridges data silos, helping leaders track user behavior and network loads in near real time.
- 2. Data initiatives support cost reduction through targeted resource allocation and proactive service improvements.
- 3. Advanced analytics methods highlight fraud risks, improve subscriber loyalty, and generate new revenue channels.
- 4. Investments in scalable infrastructure streamline operational workflows, increasing efficiency.
- 5. ROI assessments based on clear metrics demonstrate how data analytics telecom projects drive tangible gains.
What is big data analytics in the telecom industry?
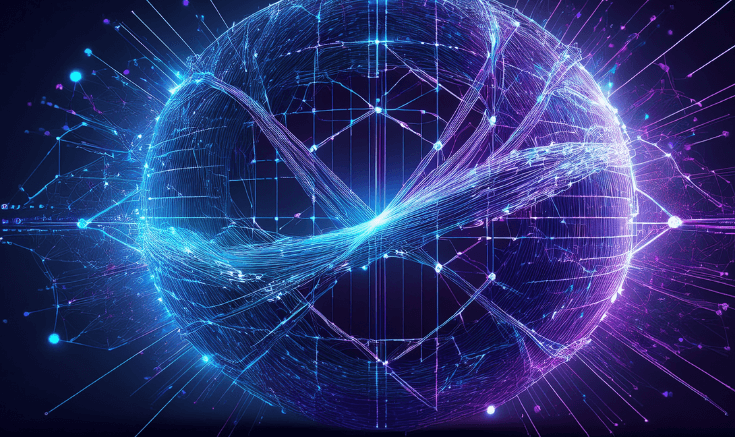
Big data analytics in telecom refers to systematically examining vast datasets drawn from call routing, customer engagement metrics, and network performance logs. The primary objective is to identify patterns and correlations that can help you address subscriber needs and optimize resource allocation. Advanced analytics techniques, including machine learning (ML), allow operators to forecast user behavior, reduce instances of fraud, and personalize service offerings. This discipline unifies data from multiple sources so decision-makers can make timely, evidence-based strategies.
Telecom providers handle dynamic network loads and unpredictable customer demands, so quick insights reduce downtime and prevent service disruptions. Big data analytics for telecom can also support more efficient budgeting, aligning investments with key performance indicators such as latency reduction and subscriber retention. This approach helps senior leaders prioritize resources, ensuring that major network deployments and expansions address real subscriber concerns. Many experts see telecom big data analytics as a strategic asset that continuously adapts to market shifts.
Key benefits of big data analytics for telecom companies
Carriers see measurable advantages when integrating advanced analytical capabilities into their daily workflows. Immediate insights into subscriber behaviors, network anomalies, and usage patterns support faster decision cycles and more cost-effective planning. Direct access to relevant metrics also boosts cooperation across finance, marketing, and engineering teams, resulting in shared accountability.
- Better subscriber experience: Tailored offers and promotions reduce churn and make service enhancements more relevant to user needs.
- Efficient resource allocation: Operators gain clarity on traffic spikes so they can plan capacity expansions at the right times.
- Reduced operational costs: Automated analysis of network patterns lowers maintenance expenses through predictive maintenance techniques.
- Quicker revenue growth: Targeted cross-selling strategies based on subscriber data accelerate acquisitions and boost overall profitability.
- Fraud prevention: Real-time detection of suspicious activities helps operators minimize financial losses and protect customer trust.
- Enhanced strategic planning: Actionable intelligence supports more agile product rollouts and more accurate budgeting.
These benefits help telecom providers refine their strategies, addressing immediate operational concerns while setting long-term priorities. Big data analytics for telecom positions senior stakeholders to track performance indicators in near real time, improving cross-department collaboration. Results often include streamlined supply chains, stronger customer loyalty, and higher margins. Strong analytics foundations also serve as a launching pad for advanced solutions like artificial intelligence and Internet of Things (IoT) platforms.
“Immediate insights into subscriber behaviors, network anomalies, and usage patterns support faster decision cycles and more cost-effective planning.”
Types of big data analytics in the telecom industry
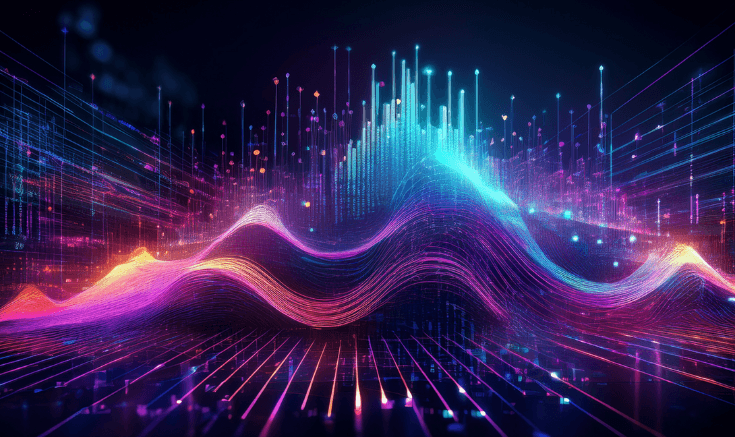
Stakeholders often categorize analytics approaches based on the type of insight they provide. This structure helps you map out how data flows from raw collection to targeted recommendations. Each methodology focuses on a distinct outcome, guiding telecom teams toward relevant metrics and patterns. Integrating several analytics types ensures a robust toolkit that adapts to different objectives.
Descriptive analytics
Descriptive analytics summarizes historical data to reveal trends and anomalies. Telecom operators track patterns in call volumes, user locations, and application usage to see which services gain traction. This baseline helps managers set performance benchmarks and measure changes over time. A clearer view of what happened fosters more precise planning for capacity expansions and service improvements.
Diagnostic analytics
Diagnostic analytics digs deeper into the root causes behind performance and usage patterns. This approach interprets historical data to explain why certain issues, such as high churn or reduced revenue in specific regions, occur. Telecom teams rely on correlation tests and statistical modeling to identify the factors that trigger these outcomes. A thorough understanding of causal relationships highlights where targeted improvements can yield immediate results.
Predictive analytics
Predictive analytics relies on machine learning algorithms and statistical techniques to forecast subscriber behavior, network performance, or product success. Telecom operators use data-powered predictions to allocate resources effectively during major events or expected surges in usage. These projections often inform marketing campaigns that align with anticipated consumer demands. Solid forecasting not only boosts profitability but also reduces wasteful spending on underutilized assets.
Prescriptive analytics
Prescriptive analytics builds on predictive models by suggesting optimal actions for specific outcomes. Telecom providers apply these methods to plan network routing strategies, fine-tune pricing models, and prioritize maintenance schedules. The system evaluates multiple scenarios, testing how each decision might impact costs and customer satisfaction. Decision-makers then choose the path that balances revenue goals with subscriber loyalty, improving ROI in a structured, data-focused way.
Common challenges in implementing big data analytics in telecom
Even with clear advantages, data initiatives often encounter operational roadblocks. Many telecoms deal with silos, outdated infrastructures, and complex compliance requirements that slow progress..
- Data quality inconsistencies: Mismatched formats or incomplete records disrupt analysis and reduce accuracy.
- Limited cross-department cooperation: Fragmented teams hamper progress when critical stakeholders are not aligned on project goals.
- Skills shortages: Hiring or training data specialists can be expensive, and inexperienced teams risk misinterpreting analytical outputs.
- Security risks: Large volumes of sensitive customer data attract cyber threats, raising the stakes for robust protection protocols.
- Complex regulatory constraints: Compliance rules around data retention and usage often change, requiring constant updates to internal policies.
Addressing these hurdles demands an organized plan that considers both technical and organizational factors. Strong communication across teams helps managers unify resources and clarify objectives. Sustainable growth in big data analytics for telecom also hinges on ongoing workforce development, ensuring staff stay informed of leading practices. Each challenge represents an opportunity to streamline processes and enhance service quality.
Strategies for optimizing big data analytics investments in telecom
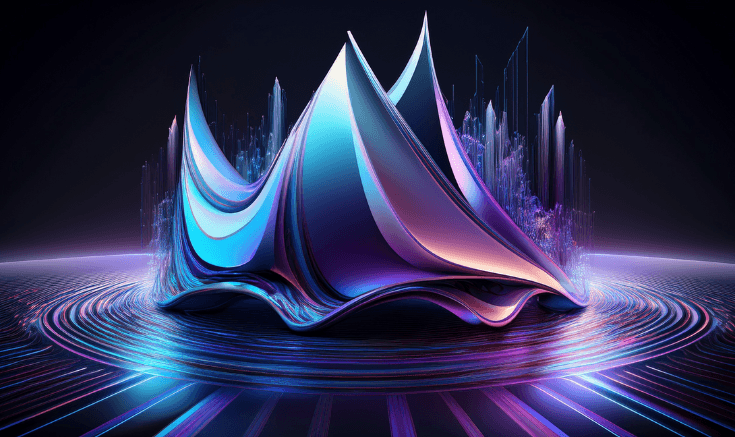
Organizations aiming to maximize returns often integrate a blend of technical and managerial strategies. Careful goal alignment ensures analytics activities match broader objectives, from cost reduction to subscriber engagement. Technology choices also matter, since selecting scalable tools positions providers for future growth. Strong collaboration across departments cements these gains, turning disparate efforts into focused results.
Align analytics goals with business outcomes
Placing business objectives at the core of analytics initiatives sets a clear direction for resource allocation. Leaders identify the most critical pain points, such as high churn or network strain, to define measurable targets. This approach helps prioritize data collection efforts, ensuring relevant metrics are available for real-time decisions. Telecom providers then avoid unnecessary spending and direct budgets toward high-impact improvements.
Adopt a scalable technology infrastructure
Legacy systems often create bottlenecks, so a scalable infrastructure is important for seamless analytics operations. Modern cloud-based solutions or on-premise clusters handle large data volumes without compromising speed. This architecture lowers the total cost of ownership by reducing maintenance overhead and providing flexible options for expansion. Consistent upgrades ensure networks remain resilient and capable of absorbing fresh data sources.
Foster cross-functional expertise
True innovation emerges when technical, financial, and marketing teams share insights. Establishing data literacy programs allows each department to interpret analytics results accurately. Collaboration also prevents conflicts over budgets or project timelines, since everyone understands the bigger business picture. Over time, this unified perspective supports more strategic conversations around advanced analytical use cases and continuous service enhancements.
Practical applications of big data analytics in telecom
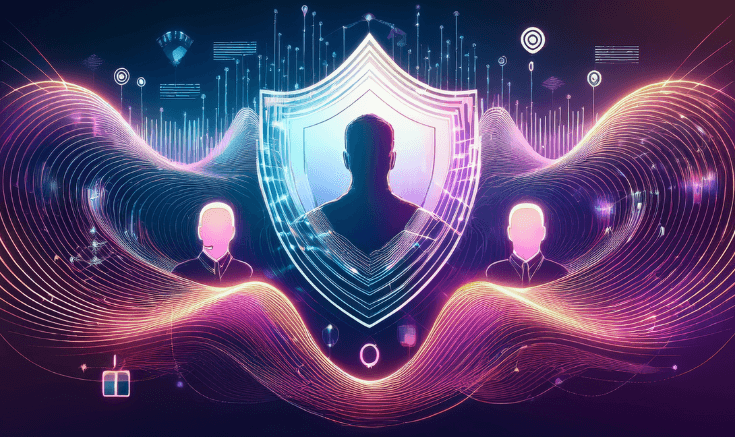
Many telecom companies apply analytics techniques to specific operations, turning raw data into measurable improvements. This includes better resource utilization, deeper customer engagement, and targeted fraud detection. These use cases serve as tangible examples of how analytics reshapes traditional workflows. Each solution incorporates real-time monitoring and agile processes, allowing operators to stay ahead of shifting subscriber demands.
Network optimization
Traffic monitoring tools process streaming data to identify congestion or outages. Rapid detection of bottlenecks helps engineers apply corrective measures before significant disruption occurs. This approach reduces customer complaints and fosters loyalty by prioritizing stable connections. Proactive maintenance becomes more efficient when analytics platforms signal hardware or software vulnerabilities.
Customer churn prevention
Operators leverage subscriber usage metrics, billing patterns, and service feedback to forecast potential churn. Algorithms segment at-risk groups based on changes in consumption or complaints, prompting targeted retention campaigns. Personalized plans and promotions often re-engage customers while boosting overall satisfaction. Significant revenue leakage is avoided when high-value subscribers remain loyal through customized service offerings.
Fraud detection
Large-scale data analysis highlights suspicious transactions or unauthorized account activities. This capability involves tracking anomalies in device location, payment patterns, and unexpected usage spikes. Machine learning flags deviations from normal behavior, prompting timely investigations that can limit financial damage. A robust fraud detection framework also preserves trust by safeguarding sensitive subscriber data.
“Rapid detection of bottlenecks helps engineers apply corrective measures before significant disruption occurs.”
Measuring ROI of telecom big data analytics strategies
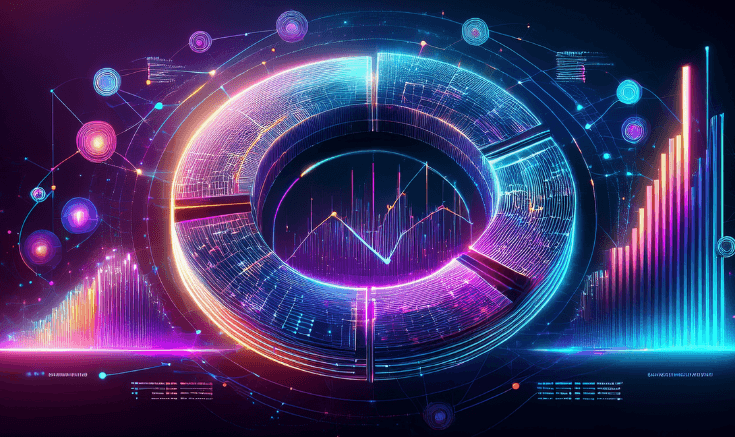
Clear metrics establish whether analytics investments yield desirable outcomes. Return on investment often hinges on factors like churn reduction, new revenue streams, or operational savings. Operators frequently track the ratio of analytics-related spending to revenue gains, comparing these figures over multiple quarters. Transparent reporting of these KPIs allows executives to justify further enhancements to analytics tools and personnel.
Profit-based models offer a tangible view of how analytics drives performance. Some companies also measure intangible benefits, including brand reputation or improved stakeholder alignment. Balancing quantitative and qualitative metrics provides a comprehensive picture of how telecom big data analytics impacts the bottom line. A well-defined ROI framework helps organizations continuously refine their strategies for sustained growth.
Big data analytics is not just a technical add-on—it provides a clear path to stronger customer relationships, resource savings, and expanded revenue opportunities for telecom providers. Focus on scalable solutions and strategic alignment empowers your teams to innovate faster without compromising reliability. At Lumenalta, we specialize in designing tailored big data analytics solutions that match your goals, ensuring you reach measurable returns. Let’s chart a brighter path together.
table-of-contents
- What is big data analytics in the telecom industry?
- Key benefits of big data analytics for telecom companies
- Types of big data analytics in the telecom industry
- Common challenges in implementing big data analytics in telecom
- Strategies for optimizing big data analytics investments in telecom
- Practical applications of big data analytics in telecom
- Measuring ROI of telecom big data analytics strategies
- Common questions about big data analytics for telecom
Common questions about big data analytics for telecom
What is the role of big data analytics for telecom in optimizing network performance?
How do telecom companies protect sensitive subscriber data when applying big data analytics?
Can big data analytics in telecom industry settings help reduce churn?
Which analytics approaches are most effective for detecting network fraud?
How do decision-makers justify big data analytics investments?
Want to learn how big data analytics can bring more transparency and trust to your operations?